GFPGANv1
Maintainer: TencentARC

47
↗️
Property | Value |
---|---|
Run this model | Run on HuggingFace |
API spec | View on HuggingFace |
Github link | No Github link provided |
Paper link | No paper link provided |
Create account to get full access
Model overview
GFPGANv1
is an AI model developed by TencentARC that aims to restore and enhance facial details in images. It is similar to other face restoration models like gfpgan and gfpgan which are also created by TencentARC. These models are designed to work on both old photos and AI-generated faces to improve their visual quality.
Model inputs and outputs
GFPGANv1
takes an image as input and outputs an enhanced version of the same image with improved facial details. The model is particularly effective at addressing common issues in AI-generated faces, such as blurriness or lack of realism.
Inputs
- Images containing human faces
Outputs
- Enhanced images with more realistic and detailed facial features
Capabilities
GFPGANv1
can significantly improve the visual quality of faces in images, making them appear more natural and lifelike. This can be particularly useful for enhancing the results of other AI models that generate faces, such as T2I-Adapter and arc_realistic_models.
What can I use it for?
You can use GFPGANv1
to improve the visual quality of AI-generated faces or to restore and enhance old, low-quality photos. This can be useful in a variety of applications, such as creating more realistic virtual avatars, improving the appearance of characters in video games, or restoring family photos. The model's ability to address common issues in AI-generated faces also makes it a valuable tool for researchers and developers working on text-to-image generation models like sdxl-lightning-4step.
Things to try
One interesting aspect of GFPGANv1
is its ability to work on a wide range of facial images, from old photographs to AI-generated faces. You could experiment with feeding the model different types of facial images and observe how it enhances the details and realism in each case. Additionally, you could try combining GFPGANv1
with other AI models that generate or manipulate images to see how the combined outputs can be further improved.
This summary was produced with help from an AI and may contain inaccuracies - check out the links to read the original source documents!
Related Models
🤔
T2I-Adapter

770
The T2I-Adapter is a text-to-image generation model developed by TencentARC that provides additional conditioning to the Stable Diffusion model. The T2I-Adapter is designed to work with the StableDiffusionXL (SDXL) base model, and there are several variants of the T2I-Adapter that accept different types of conditioning inputs, such as sketch, canny edge detection, and depth maps. The T2I-Adapter model is built on top of the Stable Diffusion model and aims to provide more controllable and expressive text-to-image generation capabilities. The model was trained on 3 million high-resolution image-text pairs from the LAION-Aesthetics V2 dataset. Model inputs and outputs Inputs Text prompt**: A natural language description of the desired image. Control image**: A conditioning image, such as a sketch or depth map, that provides additional guidance to the model during the generation process. Outputs Generated image**: The resulting image generated by the model based on the provided text prompt and control image. Capabilities The T2I-Adapter model can generate high-quality and detailed images based on text prompts, with the added control provided by the conditioning input. The model's ability to generate images from sketches or depth maps can be particularly useful for applications such as digital art, concept design, and product visualization. What can I use it for? The T2I-Adapter model can be used for a variety of applications, such as: Digital art and illustration**: Generate custom artwork and illustrations based on text prompts and sketches. Product design and visualization**: Create product renderings and visualizations by providing depth maps or sketches as input. Concept design**: Quickly generate visual concepts and ideas based on textual descriptions. Education and research**: Explore the capabilities of text-to-image generation models and experiment with different conditioning inputs. Things to try One interesting aspect of the T2I-Adapter model is its ability to generate images from different types of conditioning inputs, such as sketches, depth maps, and edge maps. Try experimenting with these different conditioning inputs and see how they affect the generated images. You can also try combining the T2I-Adapter with other AI models, such as GFPGAN, to further enhance the quality and realism of the generated images.
Updated Invalid Date
🌐
arc_realistic_models

48
arc_realistic_models is an AI model designed for image-to-image tasks. It is similar to models like animelike2d, photorealistic-fuen-v1, iroiro-lora, sd-webui-models, and doll774, which also focus on image-to-image tasks. This model was created by the Hugging Face user GRS0024. Model inputs and outputs arc_realistic_models takes image data as input and generates transformed images as output. The model can be used to create photorealistic renders, stylize images, and perform other image-to-image transformations. Inputs Image data Outputs Transformed image data Capabilities arc_realistic_models can be used to perform a variety of image-to-image tasks, such as creating photorealistic renders, stylizing images, and generating new images from existing ones. The model's capabilities are similar to those of other image-to-image models, but the specific outputs may vary. What can I use it for? arc_realistic_models can be used for a variety of creative and professional applications, such as generating product visualizations, creating art assets, and enhancing existing images. The model's ability to generate photorealistic outputs makes it particularly useful for product design and visualization projects. Things to try Experiment with different input images and see how the model transforms them. Try using the model to create stylized versions of your own photographs or to generate new images from scratch. The model's versatility means there are many possibilities to explore.
Updated Invalid Date
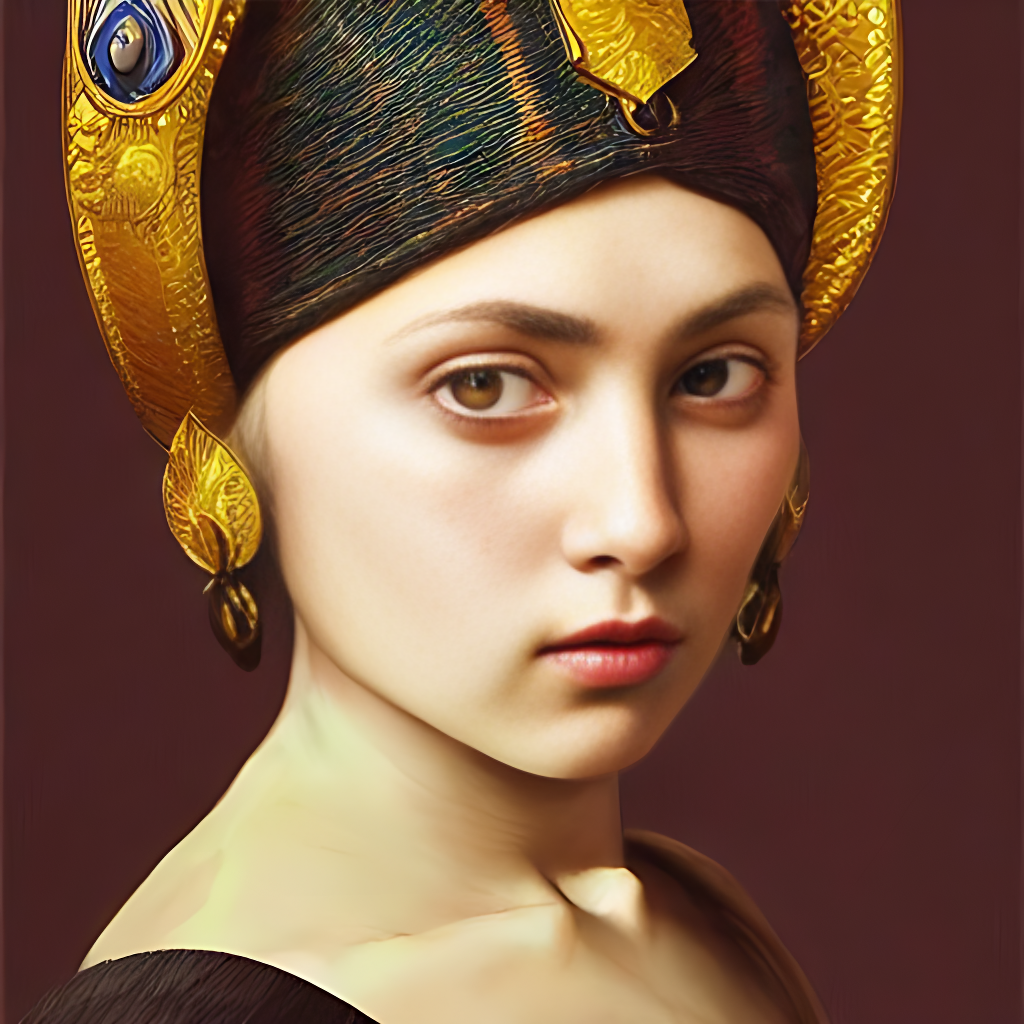
gfpgan

80.1K
gfpgan is a practical face restoration algorithm developed by the Tencent ARC team. It leverages the rich and diverse priors encapsulated in a pre-trained face GAN (such as StyleGAN2) to perform blind face restoration on old photos or AI-generated faces. This approach contrasts with similar models like Real-ESRGAN, which focuses on general image restoration, or PyTorch-AnimeGAN, which specializes in anime-style photo animation. Model inputs and outputs gfpgan takes an input image and rescales it by a specified factor, typically 2x. The model can handle a variety of face images, from low-quality old photos to high-quality AI-generated faces. Inputs Img**: The input image to be restored Scale**: The factor by which to rescale the output image (default is 2) Version**: The gfpgan model version to use (v1.3 for better quality, v1.4 for more details and better identity) Outputs Output**: The restored face image Capabilities gfpgan can effectively restore a wide range of face images, from old, low-quality photos to high-quality AI-generated faces. It is able to recover fine details, fix blemishes, and enhance the overall appearance of the face while preserving the original identity. What can I use it for? You can use gfpgan to restore old family photos, enhance AI-generated portraits, or breathe new life into low-quality images of faces. The model's capabilities make it a valuable tool for photographers, digital artists, and anyone looking to improve the quality of their facial images. Additionally, the maintainer tencentarc offers an online demo on Replicate, allowing you to try the model without setting up the local environment. Things to try Experiment with different input images, varying the scale and version parameters, to see how gfpgan can transform low-quality or damaged face images into high-quality, detailed portraits. You can also try combining gfpgan with other models like Real-ESRGAN to enhance the background and non-facial regions of the image.
Updated Invalid Date
🔗
DragGan-Models

42
DragGan-Models is a text-to-image AI model. Similar models include sdxl-lightning-4step, GhostMix, DynamiCrafter_pruned, and DGSpitzer-Art-Diffusion. These models all focus on generating images from text prompts, with varying levels of quality, speed, and specialization. Model inputs and outputs The DragGan-Models accepts text prompts as input and generates corresponding images as output. The model can produce a wide variety of images based on the provided prompts, from realistic scenes to abstract and fantastical visualizations. Inputs Text prompts:** The model takes in text descriptions that describe the desired image. Outputs Generated images:** The model outputs images that match the provided text prompts. Capabilities DragGan-Models can generate high-quality images from text prompts, with the ability to capture detailed scenes, textures, and stylistic elements. The model has been trained on a vast dataset of images and text, allowing it to understand and translate language into visual representations. What can I use it for? You can use DragGan-Models to create custom images for a variety of applications, such as social media content, marketing materials, or even as a tool for creative expression. The model's ability to generate unique visuals based on text prompts makes it a versatile tool for those looking to explore the intersection of language and imagery. Things to try Experiment with different types of text prompts to see the range of images that DragGan-Models can generate. Try prompts that describe specific scenes, objects, or artistic styles, and see how the model interprets and translates them into visual form. Explore the model's capabilities by pushing the boundaries of what it can create, and use the results to inspire new ideas and creative projects.
Updated Invalid Date