llamaguard-7b
Maintainer: tomasmcm

540
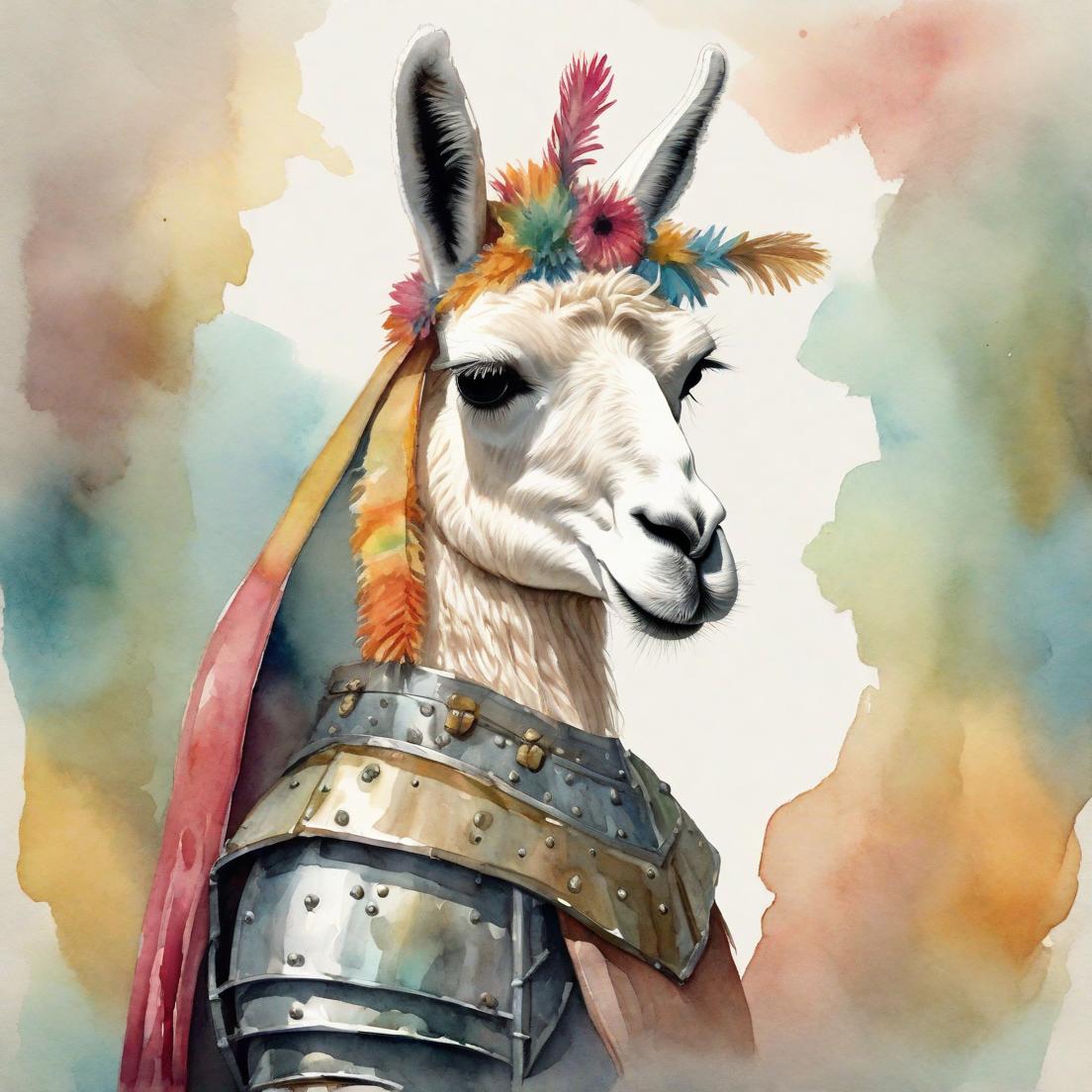
Property | Value |
---|---|
Run this model | Run on Replicate |
API spec | View on Replicate |
Github link | No Github link provided |
Paper link | View on Arxiv |
Create account to get full access
Model overview
llamaguard-7b
is a 7 billion parameter Llama 2-based model developed by tomasmcm that serves as an input-output safeguard. It is similar in size and capabilities to models like codellama-7b, codellama-7b-instruct, and other Llama-based models. The key focus of llamaguard-7b
is on providing input-output safeguards to help ensure safe and responsible language model usage.
Model inputs and outputs
llamaguard-7b
takes a text prompt as input and generates a text output. The model provides various input parameters to control the generation, including:
Inputs
- Prompt: The text prompt to send to the model.
- Max Tokens: The maximum number of tokens to generate per output sequence.
- Temperature: A float that controls the randomness of the sampling, with lower values making the model more deterministic and higher values making it more random.
- Presence Penalty: A float that penalizes new tokens based on whether they appear in the generated text so far, encouraging the use of new tokens.
- Frequency Penalty: A float that penalizes new tokens based on their frequency in the generated text so far, also encouraging the use of new tokens.
- Top K: An integer that controls the number of top tokens to consider, with -1 meaning to consider all tokens.
- Top P: A float that controls the cumulative probability of the top tokens to consider, with values between 0 and 1.
- Stop: A list of strings that, if generated, will stop the generation.
Outputs
- Output: The generated text output from the model.
Capabilities
llamaguard-7b
is a capable language model that can be used for a variety of natural language processing tasks, such as text generation, summarization, and translation. It is particularly well-suited for applications that require safe and responsible language model usage, as it includes various safeguards and controls to help ensure the generated output is appropriate and aligned with the user's intentions.
What can I use it for?
llamaguard-7b
can be used in a wide range of applications, including content creation, chatbots, virtual assistants, and even creative writing. Its input-output safeguards make it well-suited for use cases where the generated text needs to be carefully controlled, such as in educational, medical, or financial contexts. Additionally, the model's flexibility allows it to be fine-tuned or adapted for more specialized use cases, such as code generation or programming assistance.
Things to try
One interesting aspect of llamaguard-7b
is its ability to generate text with a high degree of coherence and logical consistency, while still maintaining a sense of creativity and originality. Users can experiment with adjusting the model's input parameters, such as temperature and top-k/top-p values, to explore the range of outputs and find the sweet spot that works best for their specific use case. Additionally, users can try providing the model with different types of prompts, from creative writing exercises to task-oriented instructions, to see how it responds and adapts to different contexts.
This summary was produced with help from an AI and may contain inaccuracies - check out the links to read the original source documents!
Related Models
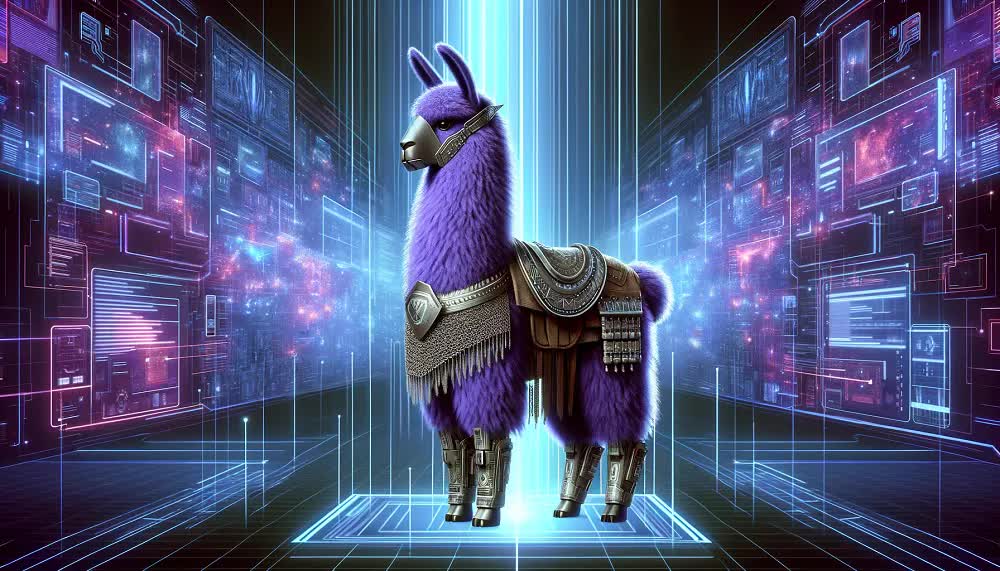
meta-llama-guard-2-8b

142
meta-llama-guard-2-8b is a language model based on the Llama-3 architecture, developed by Meta. This model is specifically designed for moderation and safeguarding tasks, aimed at detecting and filtering potentially harmful or unsafe content. It builds upon the capabilities of the base Llama-3 model, with additional fine-tuning and training focused on content moderation. Model inputs and outputs meta-llama-guard-2-8b takes user-generated text as input and provides an assessment of the safety and appropriateness of the content. The model is designed to identify and flag potentially harmful or abusive language, explicit content, or instructions related to illegal activities. Inputs User-generated text, such as comments, messages, or prompts Outputs Prediction of the safety and appropriateness of the input text Flagging of potential issues or concerns Capabilities meta-llama-guard-2-8b is capable of analyzing text inputs and determining whether they contain potentially harmful or inappropriate content. This includes identifying explicit language, violent or hateful speech, and instructions related to illegal activities. The model is trained to provide reliable and accurate assessments to help ensure a safe and constructive online environment. What can I use it for? meta-llama-guard-2-8b can be integrated into a variety of applications and services that involve user-generated content, such as social media platforms, chat systems, or content moderation platforms. By integrating this model, developers can automate the process of detecting and filtering potentially harmful or inappropriate content, improving the overall user experience and promoting a safer online environment. Things to try You can experiment with meta-llama-guard-2-8b by providing it with different types of text inputs, ranging from harmless conversations to potentially unsafe or illegal content. Observe how the model responds and assess its ability to accurately identify and flag problematic content. This can help you understand the model's capabilities and limitations, and how it could be applied in your specific use case.
Updated Invalid Date
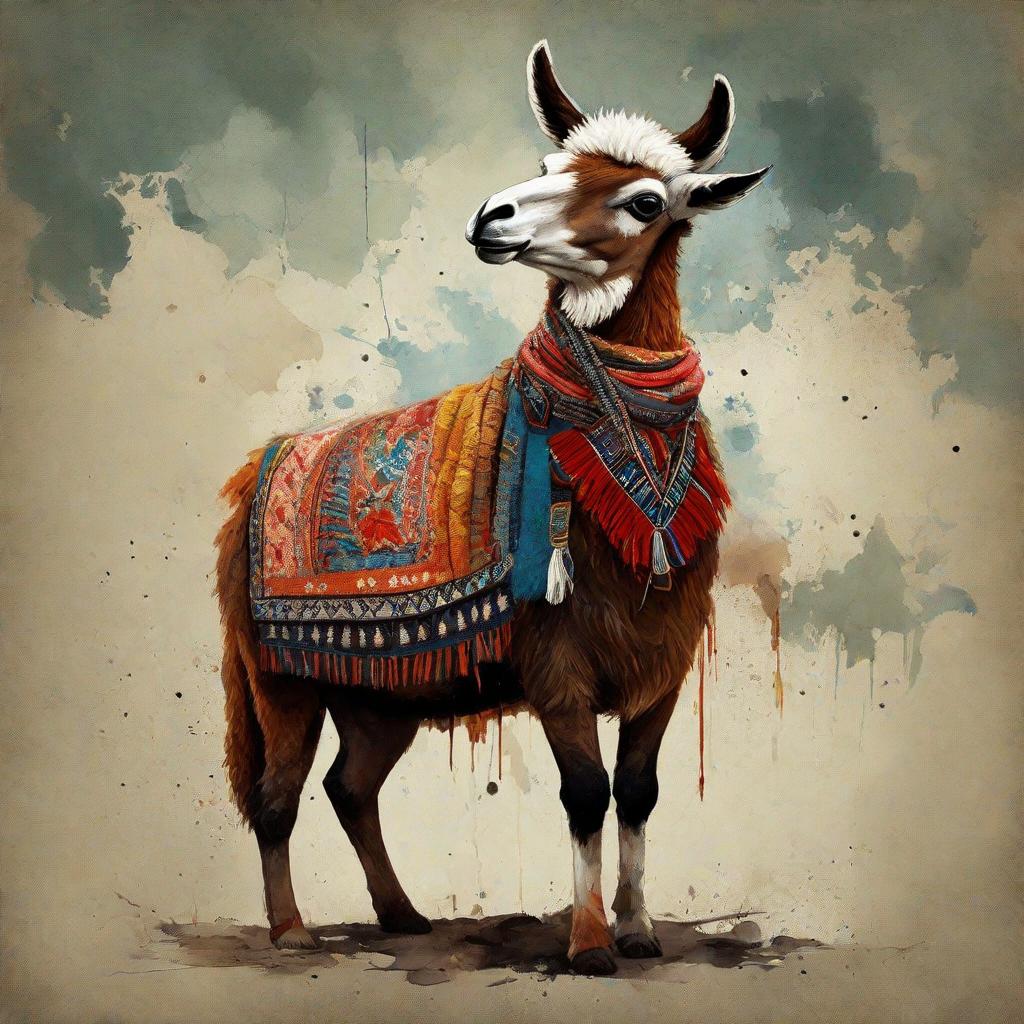
llama2-13b-tiefighter

166
llama2-13b-tiefighter is a large language model developed by the KoboldAI community. It is a merged model created by combining multiple pre-existing models, including LLaMA2-13B-Tiefighter and LLaMA2-13B-Tiefighter-GGUF. The model aims to be a creative and versatile tool, capable of tasks like story writing, persona-based chatbots, and interactive adventures. Model inputs and outputs Inputs prompt**: The text prompt to send to the model. max_tokens**: The maximum number of tokens to generate per output sequence. temperature**: A float that controls the randomness of the sampling, with lower values making the model more deterministic and higher values making it more random. top_k**: An integer that controls the number of top tokens to consider during generation. top_p**: A float that controls the cumulative probability of the top tokens to consider, must be between 0 and 1. presence_penalty**: A float that penalizes new tokens based on whether they appear in the generated text so far. frequency_penalty**: A float that penalizes new tokens based on their frequency in the generated text so far. stop**: A list of strings that will stop the generation when they are generated. Outputs Output**: The generated text output from the model. Capabilities llama2-13b-tiefighter is a highly capable model that can engage in a variety of creative tasks. It excels at story writing, allowing users to either continue an existing story or generate a new one from scratch. The model is also well-suited for persona-based chatbots, where it can improvise believable conversations based on simple prompts or character descriptions. What can I use it for? This model is an excellent choice for anyone looking to explore the creative potential of large language models. Writers, roleplayers, and interactive fiction enthusiasts can all find use for llama2-13b-tiefighter. The model's ability to generate coherent and engaging narratives makes it a valuable tool for world-building, character development, and open-ended storytelling. Things to try One interesting aspect of llama2-13b-tiefighter is its integration of adventure game-style mechanics. By using the > prefix for user commands, you can engage the model in interactive adventure scenarios, where it will respond based on its training on choose-your-own-adventure datasets. Experimenting with different prompts and command styles can lead to unique and unpredictable narrative experiences.
Updated Invalid Date
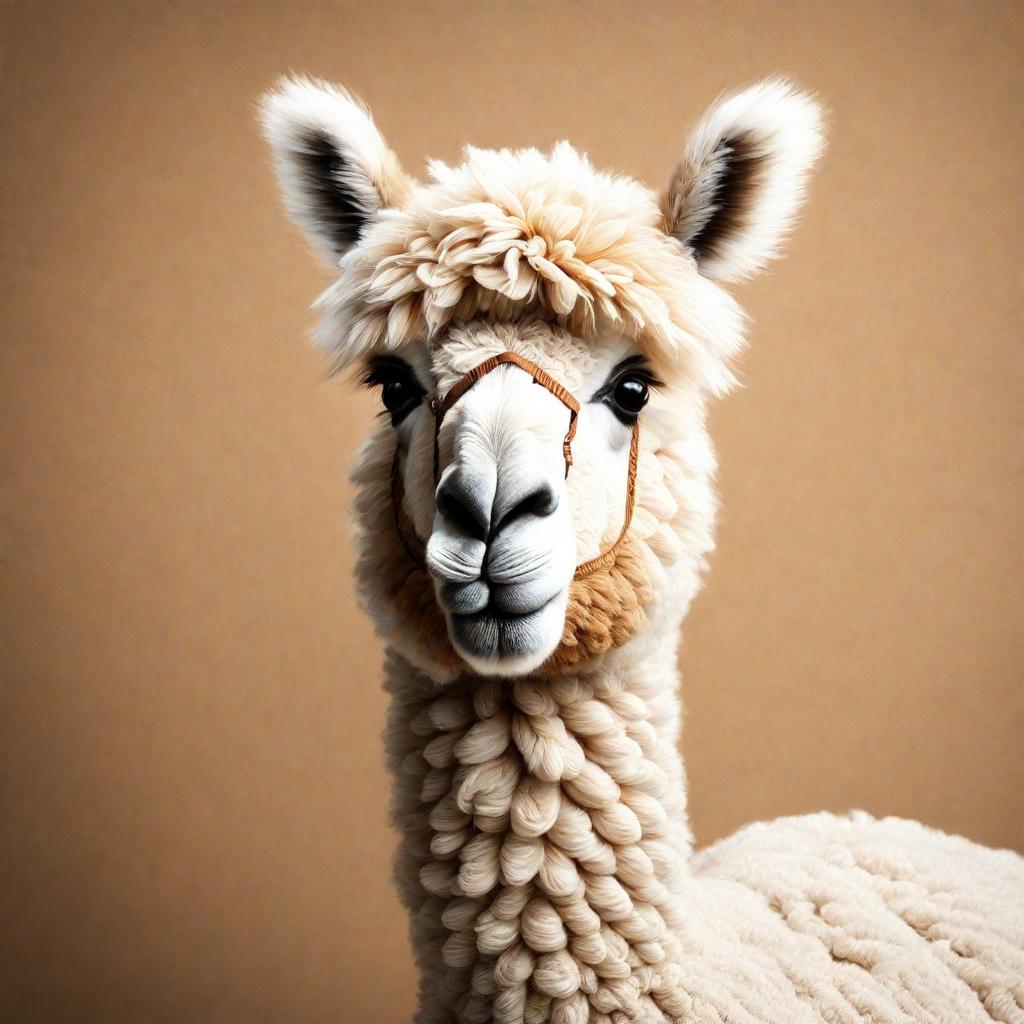
claude2-alpaca-13b

3
claude2-alpaca-13b is a large language model developed by Replicate and the UMD-Zhou-Lab. It is a fine-tuned version of Meta's Llama-2 model, using the Claude2 Alpaca dataset. This model shares similarities with other Llama-based models like llama-2-7b-chat, codellama-34b-instruct, and codellama-13b, which are also designed for tasks like coding, conversation, and instruction-following. However, claude2-alpaca-13b is uniquely trained on the Claude2 Alpaca dataset, which may give it distinct capabilities compared to these other models. Model inputs and outputs claude2-alpaca-13b is a text-to-text generation model, taking in a text prompt as input and generating relevant text as output. The model supports configurable parameters like top_k, top_p, temperature, presence_penalty, and frequency_penalty to control the sampling process and the diversity of the generated output. Inputs Prompt**: The text prompt to send to the model. Max Tokens**: The maximum number of tokens to generate per output sequence. Temperature**: A float that controls the randomness of the sampling, with lower values making the model more deterministic and higher values making it more random. Presence Penalty**: A float that penalizes new tokens based on whether they appear in the generated text so far, encouraging the model to use new tokens. Frequency Penalty**: A float that penalizes new tokens based on their frequency in the generated text so far, also encouraging the model to use new tokens. Outputs Output**: The text generated by the model in response to the input prompt. Capabilities The claude2-alpaca-13b model is capable of generating coherent and relevant text across a wide range of domains, from creative writing to task-oriented instructions. Its training on the Claude2 Alpaca dataset may give it particular strengths in areas like conversation, open-ended problem-solving, and task-completion. What can I use it for? The versatile capabilities of claude2-alpaca-13b make it suitable for a variety of applications, such as: Content Generation**: Producing engaging and informative text for blogs, articles, or social media posts. Conversational AI**: Building chatbots and virtual assistants that can engage in natural, human-like dialogue. Task-oriented Assistants**: Developing applications that can help users with various tasks, from research to analysis to creative projects. The model's large size and specialized training data mean it may be particularly well-suited for monetization by companies looking to integrate advanced language AI into their products or services. Things to try Some interesting things to explore with claude2-alpaca-13b include: Prompting the model with open-ended questions or scenarios to see how it responds creatively. Experimenting with the model's configuration parameters to generate more or less diverse, deterministic, or novel output. Comparing the model's performance to other Llama-based models like llama-2-13b-chat and codellama-13b-instruct to understand its unique strengths and weaknesses. By pushing the boundaries of what claude2-alpaca-13b can do, you can uncover new and exciting applications for this powerful language model.
Updated Invalid Date
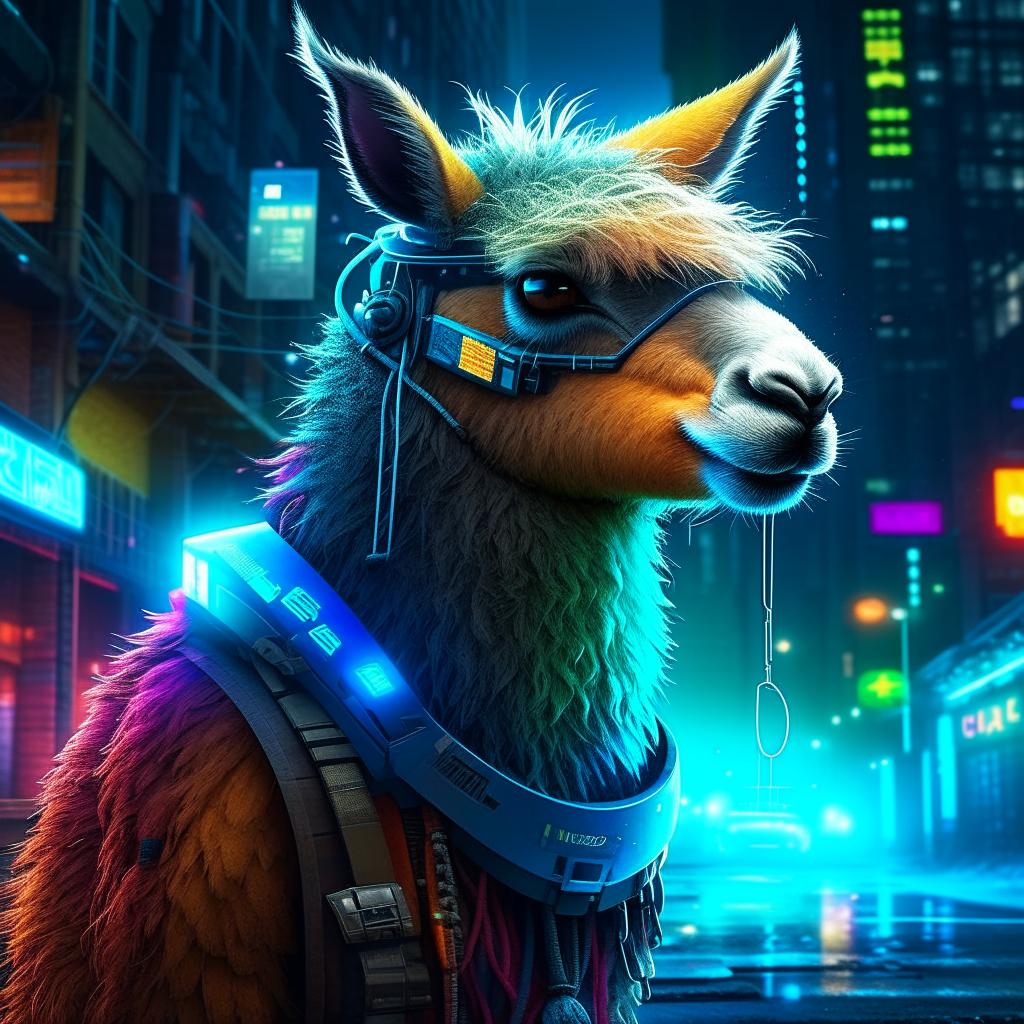
llama-2-7b-chat

20
The llama-2-7b-chat is a version of Meta's Llama 2 language model with 7 billion parameters, fine-tuned specifically for chat completions. It is part of a family of Llama 2 models created by Meta, including the base Llama 2 7B model, the Llama 2 13B model, and the Llama 2 13B chat model. These models demonstrate Meta's continued advancement in large language models. Model inputs and outputs The llama-2-7b-chat model takes several input parameters to govern the text generation process: Inputs Prompt**: The initial text that the model will use to generate additional content. System Prompt**: A prompt that helps guide the system's behavior, instructing it to be helpful, respectful, honest, and avoid harmful content. Max New Tokens**: The maximum number of new tokens the model will generate. Temperature**: Controls the randomness of the output, with higher values resulting in more varied and creative text. Top P**: Specifies the percentage of the most likely tokens to consider during sampling, allowing the model to focus on the most relevant options. Repetition Penalty**: Adjusts the likelihood of the model repeating words or phrases, encouraging more diverse output. Outputs Output Text**: The text generated by the model based on the provided input parameters. Capabilities The llama-2-7b-chat model is capable of generating human-like text responses to a wide range of prompts. Its fine-tuning on chat data allows it to engage in more natural and contextual conversations compared to the base Llama 2 7B model. The model can be used for tasks such as question answering, task completion, and open-ended dialogue. What can I use it for? The llama-2-7b-chat model can be used in a variety of applications that require natural language generation, such as chatbots, virtual assistants, and content creation tools. Its strong performance on chat-related tasks makes it well-suited for building conversational AI systems that can engage in more realistic and meaningful dialogues. Additionally, the model's smaller size compared to the 13B version may make it more accessible for certain use cases or deployment environments. Things to try One interesting aspect of the llama-2-7b-chat model is its ability to adapt its tone and style based on the provided system prompt. By adjusting the system prompt, you can potentially guide the model to generate responses that are more formal, casual, empathetic, or even playful. Experimenting with different system prompts can reveal the model's versatility and help uncover new use cases.
Updated Invalid Date