Mixtral-SlimOrca-8x7B
Maintainer: Open-Orca

50
🔮
Property | Value |
---|---|
Run this model | Run on HuggingFace |
API spec | View on HuggingFace |
Github link | No Github link provided |
Paper link | No paper link provided |
Create account to get full access
Model overview
The Mixtral-SlimOrca-8x7B
is a large language model developed by the Open-Orca team. It is a finetuned version of the Mistral-8x7B model, which is a sparse mixture of experts model that outperforms GPT-4 on many benchmarks. Open-Orca used their own OpenOrca dataset to finetune the Mistral-8x7B, aiming to reproduce the dataset from the Orca Research Paper. This model is part of their effort to create capable, open-source AI models that can run on consumer hardware.
Similar models include the Mistral-7B-OpenOrca from nateraw, the Mistral-7B-OpenOrca-GPTQ from TheBloke, and the original Mixtral-8x7B-v0.1 from Mistral AI.
Model inputs and outputs
Inputs
- Natural language text prompts
Outputs
- Generated text continuations of the input prompts
Capabilities
The Mixtral-SlimOrca-8x7B
model has strong text generation capabilities, able to produce coherent and relevant responses to a wide variety of prompts. It demonstrates impressive performance on benchmarks like the HuggingFace Leaderboard, AGIEval, and BigBench-Hard, outperforming many larger models. The model's capabilities make it well-suited for tasks like language modeling, question answering, and open-ended text generation.
What can I use it for?
The Mixtral-SlimOrca-8x7B
model could be used for a variety of natural language processing applications, such as:
- Chatbots and virtual assistants
- Content generation for blogs, articles, or creative writing
- Question answering systems
- Summarization and text simplification
- Language modeling and pretraining for downstream tasks
The model's strong performance and ability to run on consumer hardware make it an attractive option for developers and researchers looking to build capable, open-source language models.
Things to try
One interesting aspect of the Mixtral-SlimOrca-8x7B
model is its sparse mixture of experts architecture, which allows it to outperform models of similar size. Developers could experiment with prompts that leverage this capability, such as asking the model to provide multiple, diverse response options or to break down complex tasks into steps. Additionally, the model's strong performance on benchmarks suggests it may be a good starting point for further finetuning or prompt engineering to tailor it to specific use cases.
This summary was produced with help from an AI and may contain inaccuracies - check out the links to read the original source documents!
Related Models
🔍
Mistral-7B-OpenOrca

657
The Mistral-7B-OpenOrca model is a powerful language model developed by the Open-Orca team. It is built on top of the Mistral 7B base model and fine-tuned using the OpenOrca dataset, which is an attempt to reproduce the dataset generated for Microsoft Research's Orca Paper. The model uses OpenChat packing and was trained with the Axolotl framework. This release is trained on a curated filtered subset of the OpenOrca dataset, which is the same data used for the OpenOrcaxOpenChat-Preview2-13B model. Evaluation results place this 7B model as the top performer among models smaller than 30B at the time of release, outperforming other 7B and 13B models. Model inputs and outputs Inputs Natural language text prompts for the model to continue or generate. Outputs Continued or generated text based on the input prompt. Capabilities The Mistral-7B-OpenOrca model demonstrates strong performance across a variety of benchmarks, making it a capable generalist language model. It is able to engage in open-ended conversation, answer questions, and generate human-like text on a wide range of topics. What can I use it for? The Mistral-7B-OpenOrca model can be used for a variety of natural language processing tasks, such as: Open-ended conversation and dialogue Question answering Text generation (e.g. stories, articles, code) Summarization Sentiment analysis And more The model's strong performance and ability to run efficiently on consumer GPUs make it a compelling choice for a wide range of applications and projects. Things to try Some interesting things to try with the Mistral-7B-OpenOrca model include: Engaging the model in open-ended conversation and observing its ability to maintain coherence and context over multiple turns. Prompting the model to generate creative writing, such as short stories or poetry, and analyzing the results. Exploring the model's knowledge and reasoning capabilities by asking it questions on a variety of topics, from science and history to current events and trivia. Utilizing the model's accelerated performance on consumer GPUs to integrate it into real-time applications and services. The versatility and strong performance of the Mistral-7B-OpenOrca model make it a valuable tool for a wide range of AI and natural language processing applications.
Updated Invalid Date
📶
Mistral-7B-OpenOrca-GPTQ

100
The Mistral-7B-OpenOrca-GPTQ is a large language model created by OpenOrca and quantized to GPTQ format by TheBloke. This model is based on OpenOrca's Mistral 7B OpenOrca and provides multiple GPTQ parameter options to allow for optimizing performance based on hardware constraints and quality requirements. Similar models include the Mistral-7B-OpenOrca-GGUF and Mixtral-8x7B-v0.1-GPTQ, all of which provide quantized versions of large language models for efficient inference. Model inputs and outputs Inputs Text prompts**: The model takes in text prompts to generate continuations. System messages**: The model can receive system messages as part of a conversational prompt template. Outputs Generated text**: The primary output of the model is the generation of continuation text based on the provided prompts. Capabilities The Mistral-7B-OpenOrca-GPTQ model demonstrates high performance on a variety of benchmarks, including HuggingFace Leaderboard, AGIEval, BigBench-Hard, and GPT4ALL. It can be used for a wide range of natural language tasks such as open-ended text generation, question answering, and summarization. What can I use it for? The Mistral-7B-OpenOrca-GPTQ model can be used for many different applications, such as: Content generation**: The model can be used to generate engaging, human-like text for blog posts, articles, stories, and more. Chatbots and virtual assistants**: With its strong conversational abilities, the model can power chatbots and virtual assistants to provide helpful and natural responses. Research and experimentation**: The quantized model files provided by TheBloke allow for efficient inference on a variety of hardware, making it suitable for research and experimentation. Things to try One interesting thing to try with the Mistral-7B-OpenOrca-GPTQ model is to experiment with the different GPTQ parameter options provided. Each option offers a different trade-off between model size, inference speed, and quality, allowing you to find the best fit for your specific use case and hardware constraints. Another idea is to use the model in combination with other AI tools and frameworks, such as LangChain or ctransformers, to build more complex applications and workflows.
Updated Invalid Date
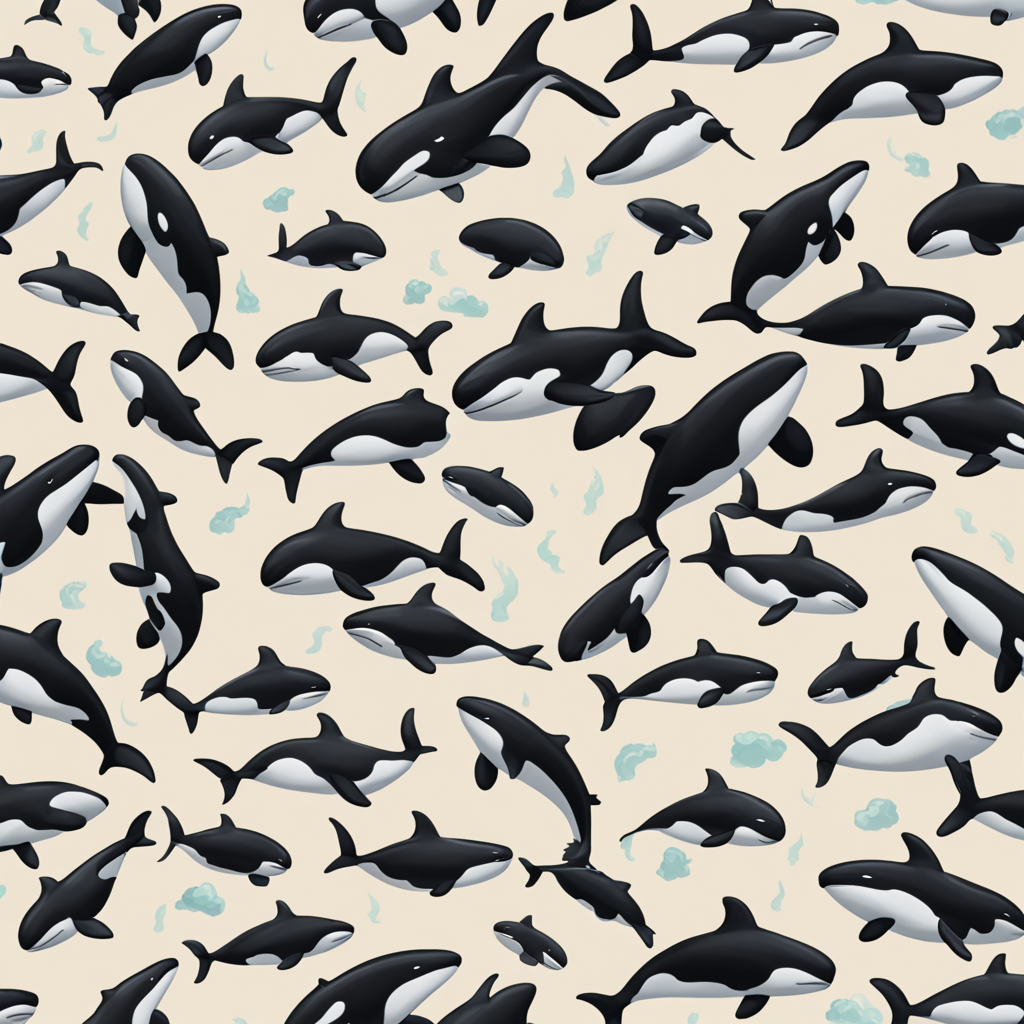
mistral-7b-openorca

65
The mistral-7b-openorca is a large language model developed by Mistral AI and fine-tuned on the OpenOrca dataset. It is a 7 billion parameter model that has been trained to engage in open-ended dialogue and assist with a variety of tasks. This model can be seen as a successor to the Mistral-7B-v0.1 and Dolphin-2.1-Mistral-7B models, which were also based on the Mistral-7B architecture but fine-tuned on different datasets. Model inputs and outputs The mistral-7b-openorca model takes a text prompt as input and generates a response as output. The input prompt can be on any topic and the model will attempt to provide a relevant and coherent response. The output is returned as a list of string tokens. Inputs Prompt**: The text prompt that the model will use to generate a response. Max new tokens**: The maximum number of tokens the model should generate as output. Temperature**: The value used to modulate the next token probabilities. Top K**: The number of highest probability tokens to consider for generating the output. Top P**: A probability threshold for generating the output, using nucleus filtering. Presence penalty**: A penalty applied to tokens based on their previous appearance in the output. Frequency penalty**: A penalty applied to tokens based on their overall frequency in the output. Prompt template**: A template used to format the input prompt, with a placeholder for the actual prompt text. Outputs Output**: A list of string tokens representing the generated response. Capabilities The mistral-7b-openorca model is capable of engaging in open-ended dialogue on a wide range of topics. It can be used for tasks such as answering questions, providing summaries, and generating creative content. The model's performance is likely comparable to similar large language models, such as the Dolphin-2.2.1-Mistral-7B and Mistral-7B-Instruct-v0.2 models, which share the same underlying architecture. What can I use it for? The mistral-7b-openorca model can be used for a variety of applications, such as: Chatbots and virtual assistants: The model's ability to engage in open-ended dialogue makes it well-suited for building conversational interfaces. Content generation: The model can be used to generate creative writing, blog posts, or other types of textual content. Question answering: The model can be used to answer questions on a wide range of topics. Summarization: The model can be used to summarize long passages of text. Things to try One interesting aspect of the mistral-7b-openorca model is its ability to provide step-by-step reasoning for its responses. By using the provided prompt template, users can instruct the model to "Write out your reasoning step-by-step to be sure you get the right answers!" This can be a useful feature for understanding the model's decision-making process and for educational or analytical purposes.
Updated Invalid Date
✨
Mistral-7B-OpenOrca-GGUF

241
Mistral-7B-OpenOrca-GGUF is a large language model created by OpenOrca, which fine-tuned the Mistral 7B model on the OpenOrca dataset. This dataset aims to reproduce the dataset from the Orca Paper. The model is available in a variety of quantized GGUF formats, which are compatible with tools like llama.cpp, text-generation-webui, and KoboldCpp. Model Inputs and Outputs Inputs The model accepts text prompts as input. Outputs The model generates coherent and contextual text output in response to the input prompt. Capabilities The Mistral-7B-OpenOrca-GGUF model demonstrates strong performance on a variety of benchmarks, outperforming other 7B and 13B models. It performs well on tasks like commonsense reasoning, world knowledge, reading comprehension, and math. The model also exhibits strong safety characteristics, with low toxicity and high truthfulness scores. What Can I Use It For? The Mistral-7B-OpenOrca-GGUF model can be used for a variety of natural language processing tasks, such as: Content Generation**: The model can be used to generate coherent and contextual text, making it useful for tasks like story writing, article creation, or dialogue generation. Question Answering**: The model's strong performance on benchmarks like NaturalQuestions and TriviaQA suggests it could be used for question answering applications. Conversational AI**: The model's chat-oriented fine-tuning makes it well-suited for developing conversational AI assistants. Things to Try One interesting aspect of the Mistral-7B-OpenOrca-GGUF model is its use of the GGUF format, which offers advantages over the older GGML format used by earlier language models. Experimenting with the different quantization levels provided in the model repository can allow you to find the right balance between model size, performance, and resource requirements for your specific use case.
Updated Invalid Date