deepfashionsdxl
Maintainer: omniedgeio

1
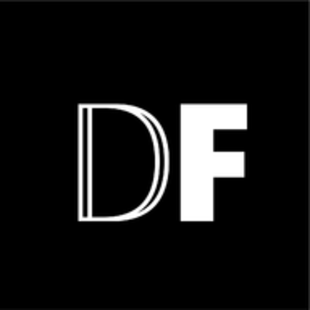
Property | Value |
---|---|
Run this model | Run on Replicate |
API spec | View on Replicate |
Github link | No Github link provided |
Paper link | No paper link provided |
Create account to get full access
Model overview
The deepfashionsdxl
model is a high-resolution image generation AI developed by omniedgeio. It is similar to other SDXL-based models like sdxl-lightning-4step and fashion-design, which are also focused on generating high-quality images. The deepfashionsdxl
model is particularly well-suited for fashion-related image generation tasks.
Model inputs and outputs
The deepfashionsdxl
model takes a variety of inputs, including a text prompt, an optional input image, and various parameters to control the output. The model can generate high-resolution images up to 1024x1024 pixels in size.
Inputs
- Prompt: The text prompt that describes the desired image.
- Image: An optional input image that can be used as a starting point for the generation process.
- Width/Height: The desired width and height of the output image.
- Num Outputs: The number of images to generate (up to 4).
- Strength: The strength of the denoising process when using an input image.
- Guidance Scale: The scale for the classifier-free guidance.
- Num Inference Steps: The number of denoising steps to perform.
Outputs
- Image: One or more high-resolution images generated based on the provided inputs.
Capabilities
The deepfashionsdxl
model is capable of generating high-quality, photorealistic images related to fashion and clothing. It can create images of models wearing various outfits, accessories, and fashion-forward designs. The model is particularly good at capturing intricate details and textures, making it well-suited for fashion-focused applications.
What can I use it for?
The deepfashionsdxl
model could be useful for a variety of fashion-related applications, such as generating product images for e-commerce websites, visualizing clothing designs, or creating fashion editorials and lookbooks. It could also be used to generate concept art or inspiration for fashion designers and stylists. Additionally, the model's ability to generate high-resolution images makes it a valuable tool for creating marketing materials, social media content, and other visual assets related to the fashion industry.
Things to try
With the deepfashionsdxl
model, you could experiment with different clothing styles, accessories, and fashion trends to see how the model interprets and generates these elements. You could also try using the model to create unique and unexpected fashion combinations or to explore the boundaries of what is possible in fashion-focused image generation.
This summary was produced with help from an AI and may contain inaccuracies - check out the links to read the original source documents!
Related Models
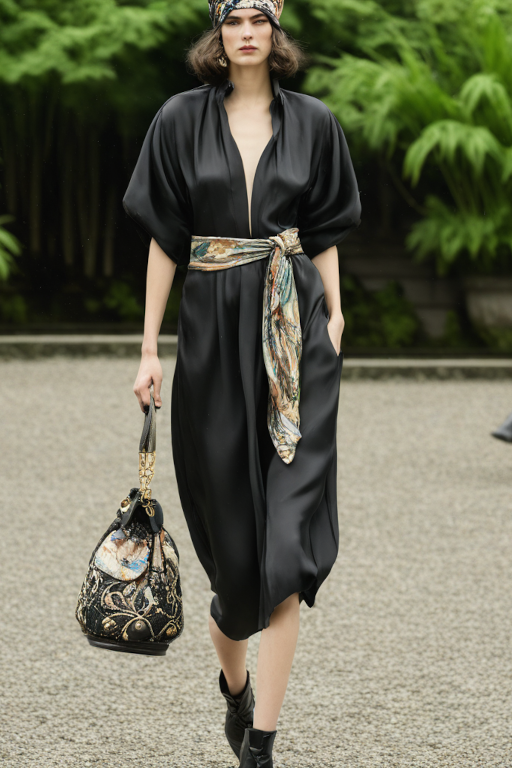
fashion-design

5
The fashion-design model by DeepFashion is a powerful AI tool designed to assist with fashion design and creation. This model can be compared to similar models like fashion-ai and lookbook, which also focus on clothing and fashion-related tasks. The fashion-design model stands out with its ability to generate and manipulate fashion designs, making it a valuable resource for designers, artists, and anyone interested in the fashion industry. Model inputs and outputs The fashion-design model accepts a variety of inputs, including an image, a prompt, and various parameters to control the output. The output is an array of generated images, which can be used as inspiration or as the basis for further refinement and development. Inputs Image**: An input image for the img2img or inpaint mode. Prompt**: A text prompt describing the desired fashion design. Mask**: An input mask for the inpaint mode, where black areas will be preserved and white areas will be inpainted. Seed**: A random seed to control the output. Width and Height**: The dimensions of the output image. Refine**: The refine style to use. Scheduler**: The scheduler to use for the diffusion process. LoRA Scale**: The additive scale for LoRA (Low-Rank Adaptation), which is only applicable on trained models. Num Outputs**: The number of images to generate. Refine Steps**: The number of steps to refine the image, used for the base_image_refiner. Guidance Scale**: The scale for classifier-free guidance. Apply Watermark**: A toggle to apply a watermark to the generated images. High Noise Frac**: The fraction of noise to use for the expert_ensemble_refiner. Negative Prompt**: An optional negative prompt to guide the image generation. Prompt Strength**: The strength of the prompt when using img2img or inpaint modes. Replicate Weights**: The LoRA weights to use, which can be left blank to use the default weights. Num Inference Steps**: The number of denoising steps to perform during the diffusion process. Outputs Array of Image URIs**: The model outputs an array of generated image URIs, which can be used for further processing or display. Capabilities The fashion-design model can be used to generate and manipulate fashion designs, including clothing, accessories, and other fashion-related elements. It can be particularly useful for designers, artists, and anyone working in the fashion industry who needs to quickly generate new ideas or explore different design concepts. What can I use it for? The fashion-design model can be used for a variety of purposes, including: Generating new fashion designs and concepts Exploring different styles and aesthetics Customizing and personalizing clothing and accessories Creating mood boards and inspiration for fashion collections Collaborating with fashion designers and brands Visualizing and testing new product ideas Things to try One interesting thing to try with the fashion-design model is exploring the different refine styles and scheduler options. By adjusting these parameters, you can generate a wide range of fashion designs, from realistic to abstract and experimental. You can also experiment with different prompts and negative prompts to see how they affect the output. Another idea is to use the fashion-design model in conjunction with other AI-powered tools, such as the fashion-ai or lookbook models, to create a more comprehensive fashion design workflow. By combining the strengths of multiple models, you can unlock even more creative possibilities and streamline your design process.
Updated Invalid Date
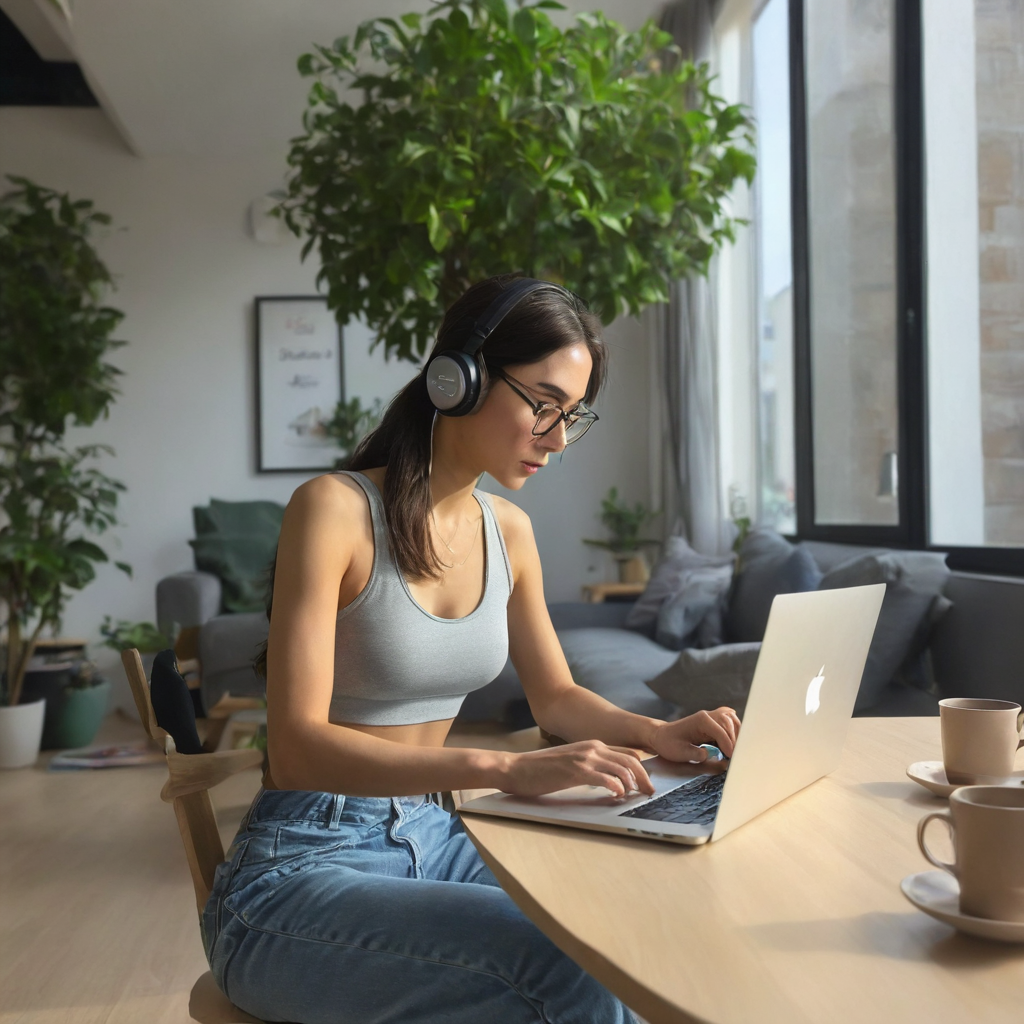
fitnessme

1
The fitnessme model is an AI-powered gym goddess generator created by omniedgeio. It is similar to other AI models for image generation, such as gfpgan, upscaler, real-esrgan, playground-v2.5-1024px-aesthetic, and instant-id-photorealistic. Model inputs and outputs The fitnessme model takes in a variety of inputs, including an image, a prompt, a seed, and various settings for the image generation process. The output is an array of generated images. Inputs Prompt**: The text prompt that describes the desired image Image**: An input image to be used for image-to-image generation or inpainting Mask**: A mask for the input image, used for inpainting Seed**: A random seed to ensure reproducibility Width/Height**: The desired dimensions of the output image Num Outputs**: The number of images to generate Guidance Scale**: The scale for classifier-free guidance Num Inference Steps**: The number of denoising steps Outputs Output Images**: An array of generated image URLs Capabilities The fitnessme model is capable of generating photorealistic images of gym goddesses based on a text prompt. It can be used to create visually stunning and highly detailed images of muscular female figures in various fitness-related poses and settings. What can I use it for? The fitnessme model could be useful for a variety of applications, such as creating images for fitness-related content, social media, or marketing. It could also be used to generate stock images or custom illustrations for fitness-focused businesses or individuals. Things to try Some interesting things to try with the fitnessme model include experimenting with different prompts to generate a variety of gym goddess styles, exploring the effect of the guidance scale and number of inference steps on the output, and using the model in combination with other image editing or upscaling tools to further enhance the generated images.
Updated Invalid Date
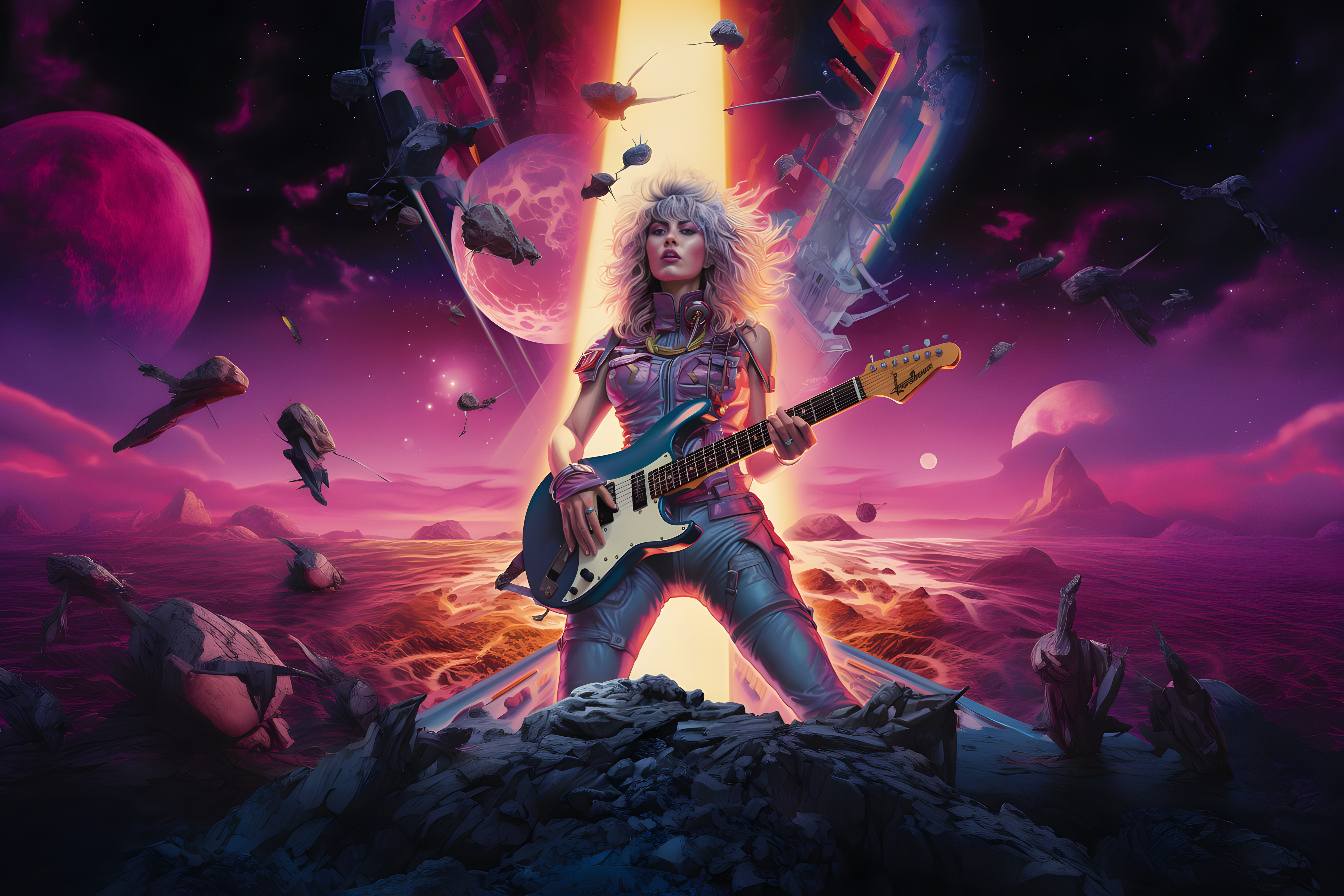
facerestoration

2
The facerestoration model is a tool for restoring and enhancing faces in images. It can be used to improve the quality of old photos or AI-generated faces. This model is similar to other face restoration models like GFPGAN, which is designed for old photos, and Real-ESRGAN, which offers face correction and upscaling. However, the facerestoration model has its own unique capabilities. Model inputs and outputs The facerestoration model takes an image as input and can optionally scale the image by a factor of up to 10x. It also has a "face enhance" toggle that can be used to further improve the quality of the faces in the image. Inputs Image**: The input image Scale**: The factor to scale the image by, from 0 to 10 Face Enhance**: A toggle to enable face enhancement Outputs Output**: The restored and enhanced image Capabilities The facerestoration model can improve the quality of faces in images, making them appear sharper and more detailed. It can be used to restore old photos or to enhance the faces in AI-generated images. What can I use it for? The facerestoration model can be a useful tool for various applications, such as photo restoration, creating high-quality portraits, or improving the visual fidelity of AI-generated images. For example, a photographer could use this model to restore and enhance old family photos, or a designer could use it to create more realistic-looking character portraits for a game or animation. Things to try One interesting way to use the facerestoration model is to experiment with the different scale and face enhancement settings. By adjusting these parameters, you can achieve a range of different visual effects, from subtle improvements to more dramatic transformations.
Updated Invalid Date
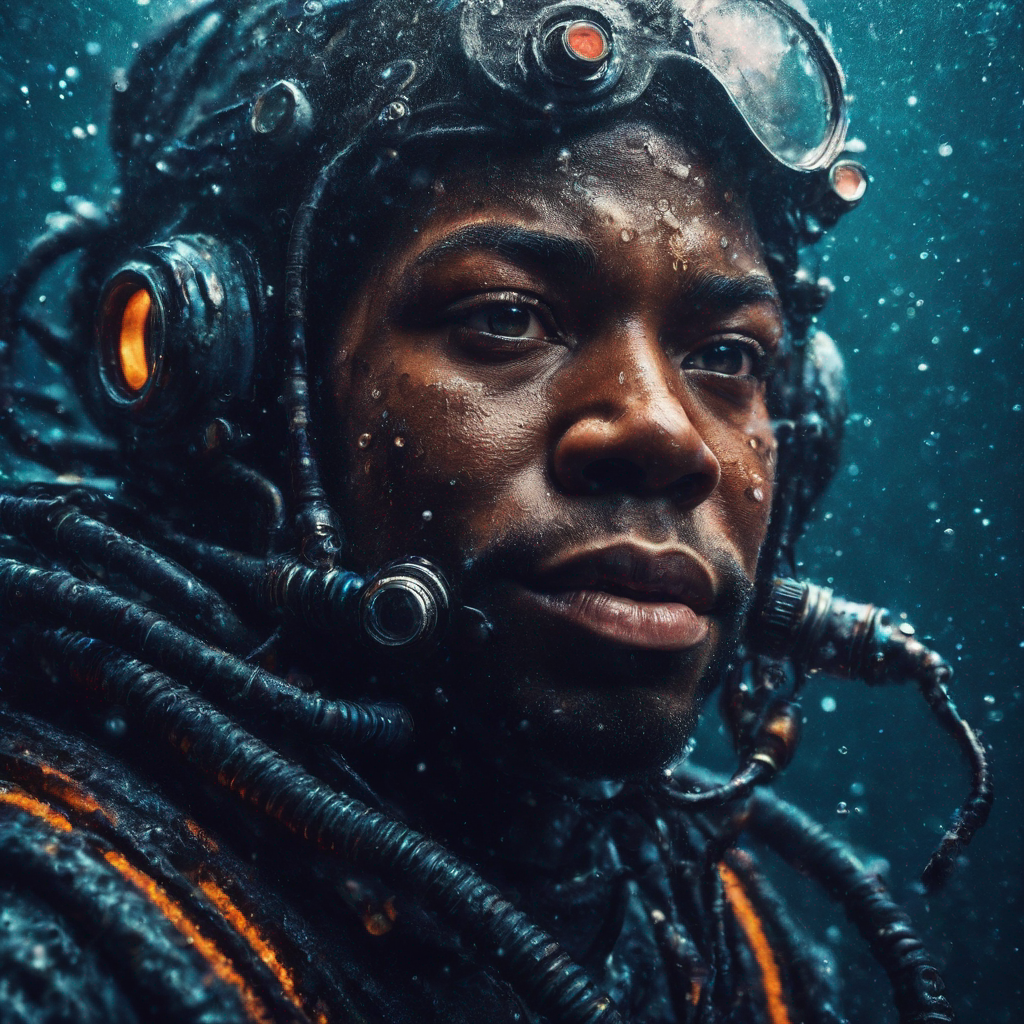
sdxl-deep-down

59
sdxl-deep-down is an SDXL model fine-tuned by fofr on underwater imagery. This model is part of a series of SDXL models created by fofr, including sdxl-black-light, sdxl-fresh-ink, sdxl-energy-drink, and sdxl-toy-story-people. The sdxl-deepcache model created by lucataco is another related SDXL model. Model inputs and outputs sdxl-deep-down takes a variety of inputs, including a prompt, image, mask, and various parameters to control the output. The model can generate images based on the provided prompt, or can perform inpainting on an input image using the provided mask. Inputs Prompt**: The text prompt that describes the desired output image. Image**: An input image for img2img or inpaint mode. Mask**: A mask for inpaint mode, where black areas will be preserved and white areas will be inpainted. Seed**: A random seed for generating the output. Width/Height**: The desired dimensions of the output image. Refine**: The refine style to use. Scheduler**: The scheduler to use for the diffusion process. LoRA Scale**: The additive scale for LoRA, applicable only on trained models. Num Outputs**: The number of images to output. Refine Steps**: The number of steps to refine for the base_image_refiner. Guidance Scale**: The scale for classifier-free guidance. Apply Watermark**: Whether to apply a watermark to the generated image. High Noise Frac**: The fraction of noise to use for the expert_ensemble_refiner. Negative Prompt**: An optional negative prompt to guide the output. Prompt Strength**: The strength of the prompt when using img2img or inpaint. Replicate Weights**: Optional LoRA weights to use. Num Inference Steps**: The number of denoising steps to perform. Outputs Images**: One or more generated images based on the provided inputs. Capabilities sdxl-deep-down can generate high-quality images based on provided text prompts, as well as perform inpainting on input images using a provided mask. The model is particularly adept at creating underwater and oceanic-themed imagery, building on the fine-tuning data it was trained on. What can I use it for? sdxl-deep-down could be useful for a variety of applications, such as creating concept art for underwater-themed video games or films, designing promotional materials for marine conservation organizations, or generating stock imagery for websites and publications focused on aquatic themes. The model's ability to perform inpainting could also be leveraged for tasks like restoring damaged underwater photographs or creating digital artwork inspired by the ocean. Things to try Experiment with different prompts and input images to see the range of outputs the sdxl-deep-down model can produce. Try combining the model with other AI-powered tools, such as those for 3D modeling or animation, to create more complex and immersive underwater scenes. You can also experiment with the various input parameters, such as the guidance scale and number of inference steps, to find the settings that work best for your specific use case.
Updated Invalid Date