multidiffusion
Maintainer: omerbt

2

Property | Value |
---|---|
Run this model | Run on Replicate |
API spec | View on Replicate |
Github link | View on Github |
Paper link | View on Arxiv |
Create account to get full access
Model overview
MultiDiffusion
is a unified framework that enables versatile and controllable image generation using a pre-trained text-to-image diffusion model, without any further training or fine-tuning. Developed by omerbt, this approach binds together multiple diffusion generation processes with a shared set of parameters or constraints, allowing for high-quality and diverse images that adhere to user-provided controls. Unlike recent text-to-image generation models like stable-diffusion which can struggle with user controllability, MultiDiffusion
provides a versatile solution for tasks such as generating images with desired aspect ratios (e.g., panoramas) or incorporating spatial guiding signals.
Model inputs and outputs
MultiDiffusion
takes in prompts, seeds, image dimensions, and other parameters to generate high-resolution images. The model outputs an array of generated images that match the user's specifications.
Inputs
- Prompt: The text prompt describing the desired image
- Seed: A random seed value to control the image generation process
- Width/Height: The desired dimensions of the output image
- Number of outputs: The number of images to generate
- Guidance scale: The scale for classifier-free guidance, controlling the trade-off between sample quality and sample diversity
- Negative prompt: Text prompts to guide the image generation away from undesired content
Outputs
- Array of images: The generated images matching the user's input prompts and parameters
Capabilities
MultiDiffusion
can generate high-quality, diverse images that adhere to user-provided controls, such as desired aspect ratio (e.g., panoramas) and spatial guiding signals. Unlike standard text-to-image models, MultiDiffusion
does not require further training or fine-tuning to achieve this level of control and versatility.
What can I use it for?
The MultiDiffusion
framework can be used for a variety of creative and practical applications, such as generating panoramic landscape images, incorporating semi-transparent effects (e.g., smoke, fire, snow) into scenes, and more. The model's ability to generate images based on spatial constraints makes it a powerful tool for tasks like product visualization, architectural design, and digital art.
Things to try
One interesting aspect of MultiDiffusion
is its ability to generate images with desired aspect ratios, such as panoramas. This can be useful for creating visually striking landscape images or immersive virtual environments. Additionally, the model's spatial control capabilities allow for the incorporation of specific elements or effects into the generated images, opening up possibilities for creative and practical applications.
This summary was produced with help from an AI and may contain inaccuracies - check out the links to read the original source documents!
Related Models
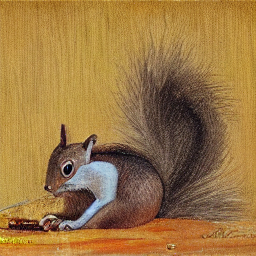
latent-diffusion-text2img

4
The latent-diffusion-text2img model is a text-to-image AI model developed by cjwbw, a creator on Replicate. It uses latent diffusion, a technique that allows for high-resolution image synthesis from text prompts. This model is similar to other text-to-image models like stable-diffusion, stable-diffusion-v2, and stable-diffusion-2-1-unclip, which are also capable of generating photo-realistic images from text. Model inputs and outputs The latent-diffusion-text2img model takes a text prompt as input and generates an image as output. The text prompt can describe a wide range of subjects, from realistic scenes to abstract concepts, and the model will attempt to generate a corresponding image. Inputs Prompt**: A text description of the desired image. Seed**: An optional seed value to enable reproducible sampling. Ddim steps**: The number of diffusion steps to use during sampling. Ddim eta**: The eta parameter for the DDIM sampler, which controls the amount of noise injected during sampling. Scale**: The unconditional guidance scale, which controls the balance between the text prompt and the model's own prior. Plms**: Whether to use the PLMS sampler instead of the default DDIM sampler. N samples**: The number of samples to generate for each prompt. Outputs Image**: A high-resolution image generated from the input text prompt. Capabilities The latent-diffusion-text2img model is capable of generating a wide variety of photo-realistic images from text prompts. It can create scenes with detailed objects, characters, and environments, as well as more abstract and surreal imagery. The model's ability to capture the essence of a text prompt and translate it into a visually compelling image makes it a powerful tool for creative expression and visual storytelling. What can I use it for? You can use the latent-diffusion-text2img model to create custom images for various applications, such as: Illustrations and artwork for books, magazines, or websites Concept art for games, films, or other media Product visualization and design Social media content and marketing assets Personal creative projects and artistic exploration The model's versatility allows you to experiment with different text prompts and see how they are interpreted visually, opening up new possibilities for artistic expression and collaboration between text and image. Things to try One interesting aspect of the latent-diffusion-text2img model is its ability to generate images that go beyond the typical 256x256 resolution. By adjusting the H and W arguments, you can instruct the model to generate larger images, up to 384x1024 or more. This can result in intriguing and unexpected visual outcomes, as the model tries to scale up the generated imagery while maintaining its coherence and detail. Another thing to try is using the model's "retrieval-augmented" mode, which allows you to condition the generation on both the text prompt and a set of related images retrieved from a database. This can help the model better understand the context and visual references associated with the prompt, potentially leading to more interesting and faithful image generation.
Updated Invalid Date
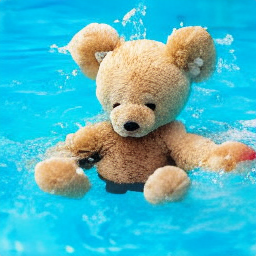
vq-diffusion

20
vq-diffusion is a text-to-image synthesis model developed by cjwbw. It is similar to other diffusion models like stable-diffusion, stable-diffusion-v2, latent-diffusion-text2img, clip-guided-diffusion, and van-gogh-diffusion, all of which are capable of generating photorealistic images from text prompts. The key innovation in vq-diffusion is the use of vector quantization to improve the quality and coherence of the generated images. Model inputs and outputs vq-diffusion takes in a text prompt and various parameters to control the generation process. The outputs are one or more high-quality images that match the input prompt. Inputs prompt**: The text prompt describing the desired image. image_class**: The ImageNet class label to use for generation (if generation_type is set to ImageNet class label). guidance_scale**: A value that controls the strength of the text guidance during sampling. generation_type**: Specifies whether to generate from in-the-wild text, MSCOCO datasets, or ImageNet class labels. truncation_rate**: A value between 0 and 1 that controls the amount of truncation applied during sampling. Outputs An array of generated images that match the input prompt. Capabilities vq-diffusion can generate a wide variety of photorealistic images from text prompts, spanning scenes, objects, and abstract concepts. It uses vector quantization to improve the coherence and fidelity of the generated images compared to other diffusion models. What can I use it for? vq-diffusion can be used for a variety of creative and commercial applications, such as visual art, product design, marketing, and entertainment. For example, you could use it to generate concept art for a video game, create unique product visuals for an e-commerce store, or produce promotional images for a new service or event. Things to try One interesting aspect of vq-diffusion is its ability to generate images that mix different visual styles and concepts. For example, you could try prompting it to create a "photorealistic painting of a robot in the style of Van Gogh" and see the results. Experimenting with different prompts and parameter settings can lead to some fascinating and unexpected outputs.
Updated Invalid Date
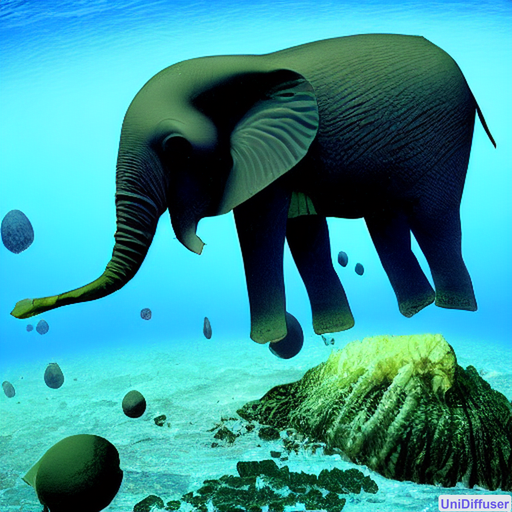
unidiffuser

1
unidiffuser is a unified diffusion framework developed by cjwbw that can fit all distributions relevant to a set of multi-modal data in a single model. Unlike traditional diffusion models that are trained for a single task, unidiffuser is able to perform image, text, text-to-image, image-to-text, and image-text pair generation by setting proper timesteps without any additional overhead. The key insight behind unidiffuser is that learning diffusion models for marginal, conditional, and joint distributions can be unified as predicting the noise in the perturbed data, where the perturbation levels (i.e. timesteps) can be different for different modalities. Inspired by this unified view, unidiffuser learns all distributions simultaneously with a minimal modification to the original diffusion model - it perturbs data in all modalities instead of a single modality, inputs individual timesteps in different modalities, and predicts the noise of all modalities instead of a single modality. unidiffuser is parameterized by a transformer model called U-ViT to handle input types of different modalities. It also utilizes a pretrained image autoencoder from Stable Diffusion, a pretrained image ViT-B/32 CLIP encoder, a pretrained text ViT-L CLIP encoder, and a GPT-2 text decoder finetuned by the researchers. Compared to similar models like Stable Diffusion, ScaleCrafter, and TokenFlow, unidiffuser is a more general-purpose model that can handle multi-modal tasks without additional overhead. Its quantitative results are also comparable to specialized models in representative tasks. Model inputs and outputs unidiffuser is a multi-modal AI model that can handle a variety of input types and generate corresponding outputs. The model takes in either text prompts, images, or both, and can produce images, text, or both as output. Inputs Prompt**: A text prompt describing the desired image or text generation. Image**: An input image for tasks like image-to-text generation or image variation. Outputs Generated Image**: The model can generate a photorealistic image based on a text prompt. Generated Text**: The model can generate relevant text descriptions for a given input image. Joint Generation**: The model can generate both an image and corresponding text description simultaneously. Capabilities unidiffuser is a highly capable multi-modal AI model that can handle a variety of tasks. It is able to produce perceptually realistic samples in all tasks, including image generation, text generation, text-to-image generation, image-to-text generation, and joint image-text generation. Its quantitative results, such as Fréchet Inception Distance (FID) and CLIP score, are not only superior to existing general-purpose models but also comparable to specialized models like Stable Diffusion and DALL-E 2 in representative tasks. What can I use it for? unidiffuser is a versatile model that can be used for a wide range of applications. Some potential use cases include: Content Creation**: Generate photorealistic images or relevant text descriptions based on prompts, helpful for tasks like graphic design, illustration, and content creation. Multimodal Understanding**: Use the model's ability to understand and generate both images and text to build applications that require deep multi-modal understanding, such as visual question answering or image captioning. Creative Exploration**: Leverage the model's open-ended generation capabilities to explore creative ideas and inspirations, such as conceptual art, storytelling, or imaginative world-building. Things to try One interesting thing to try with unidiffuser is its ability to perform image and text variation tasks. By first generating an image or text output, and then using that as input to generate a new version, the model can create novel and creative variations on the original. This can be a powerful tool for exploring ideas and expanding the creative potential of the model. Another intriguing aspect is the model's unified approach to handling different modalities and distributions. By learning a single model that can seamlessly switch between tasks like image generation, text generation, and cross-modal generation, unidiffuser demonstrates the potential for more flexible and efficient multi-modal AI systems. Experimenting with this unified framework could lead to valuable insights about the underlying connections between different modalities and how they can be best leveraged for AI applications.
Updated Invalid Date
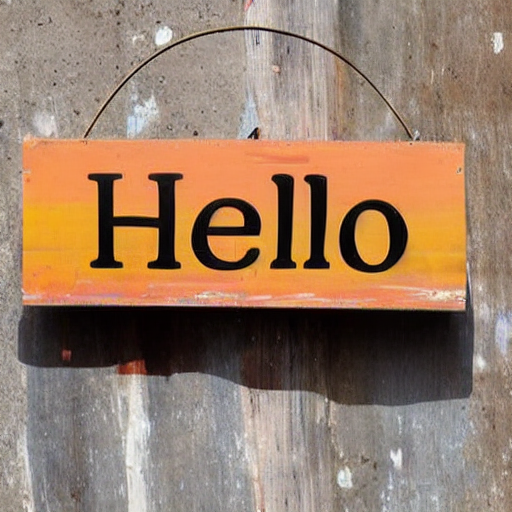
textdiffuser

1
textdiffuser is a diffusion model created by Replicate contributor cjwbw. It is similar to other powerful text-to-image models like stable-diffusion, latent-diffusion-text2img, and stable-diffusion-v2. These models use diffusion techniques to transform text prompts into detailed, photorealistic images. Model inputs and outputs The textdiffuser model takes a text prompt as input and generates one or more corresponding images. The key input parameters are: Inputs Prompt**: The text prompt describing the desired image Seed**: A random seed value to control the image generation Guidance Scale**: A parameter that controls the influence of the text prompt on the generated image Num Inference Steps**: The number of denoising steps to perform during image generation Outputs Output Images**: One or more generated images corresponding to the input text prompt Capabilities textdiffuser can generate a wide variety of photorealistic images from text prompts, ranging from scenes and objects to abstract art and stylized depictions. The quality and fidelity of the generated images are highly impressive, often rivaling or exceeding human-created artwork. What can I use it for? textdiffuser and similar diffusion models have a wealth of potential applications, from creative tasks like art and illustration to product visualization, scene generation for games and films, and much more. Businesses could use these models to rapidly prototype product designs, create promotional materials, or generate custom images for marketing campaigns. Creatives could leverage them to ideate and explore new artistic concepts, or to bring their visions to life in novel ways. Things to try One interesting aspect of textdiffuser and related models is their ability to capture and reproduce specific artistic styles, as demonstrated by the van-gogh-diffusion model. Experimenting with different styles, genres, and creative prompts can yield fascinating and unexpected results. Additionally, the clip-guided-diffusion model offers a unique approach to image generation that could be worth exploring further.
Updated Invalid Date