real-esrgan-video
Maintainer: lucataco

58
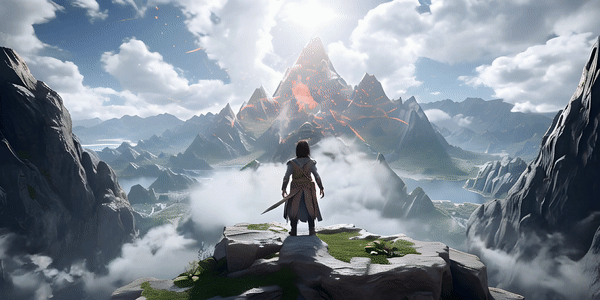
Property | Value |
---|---|
Model Link | View on Replicate |
API Spec | View on Replicate |
Github Link | View on Github |
Paper Link | View on Arxiv |
Create account to get full access
Model overview
The real-esrgan-video
model is a powerful video upscaling tool developed by lucataco. It is built on the Real-ESRGAN architecture, a state-of-the-art super-resolution model that can significantly enhance the resolution and quality of images and videos. Compared to similar models like real-esrgan, upscaler, and stable-diffusion-x4-upscaler, the real-esrgan-video
model is specifically designed for upscaling videos, offering seamless and efficient processing.
Model inputs and outputs
The real-esrgan-video
model takes a video file as input and generates an upscaled version of the video with higher resolution and improved quality. The input video can be of various formats, and the model can output the upscaled video in a range of resolutions, including 4K.
Inputs
- video_path: The input video file to be upscaled.
Outputs
- Output: The upscaled video file.
Capabilities
The real-esrgan-video
model excels at enhancing the resolution and clarity of video content. It can significantly improve the visual quality of low-resolution or compressed video, making it a valuable tool for content creators, video editors, and anyone looking to improve the presentation of their video assets.
What can I use it for?
The real-esrgan-video
model can be used in a variety of applications, such as:
- Enhancing the quality of online video content for a more professional and engaging viewing experience.
- Improving the resolution of archival or legacy video footage for preservation and restoration purposes.
- Upscaling video content for use in high-resolution displays or large-format presentations.
- Optimizing video assets for use in virtual and augmented reality applications.
Things to try
One interesting use case for the real-esrgan-video
model is to experiment with different settings and see how they affect the upscaling results. For example, you could try adjusting the resolution parameter to find the optimal balance between output quality and file size. Additionally, you could explore combining the real-esrgan-video
model with other video processing tools, such as those for video stabilization or color correction, to achieve even more impressive results.
This summary was produced with help from an AI and may contain inaccuracies - check out the links to read the original source documents!
Related Models
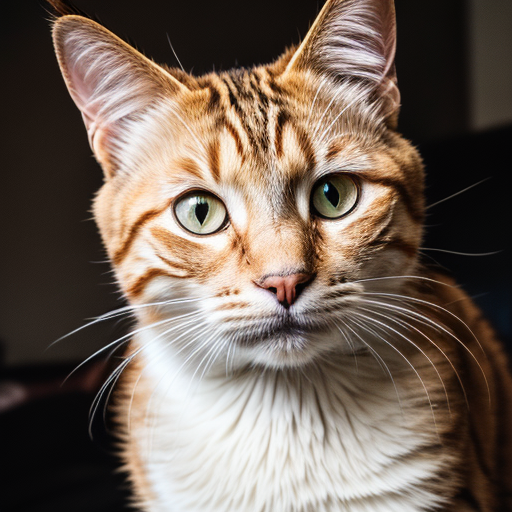
real-esrgan

26
The real-esrgan model is a powerful AI-based image upscaling and enhancement tool developed by Replicate user lucataco. It is an implementation of the Real-ESRGAN model, which aims to restore high-quality images from low-resolution inputs. This model offers optional face enhancement capabilities and allows for adjustable upscaling, making it a versatile choice for a variety of image processing tasks. Similar models include the real-esrgan-video for video upscaling, the real-esrgan model by nightmareai, and the realvisxl-v1-img2img model for image-to-image translation. Model inputs and outputs The real-esrgan model takes an input image and allows for two additional parameters: the scale factor for upscaling and a boolean flag for face enhancement. The output is a high-quality, upscaled version of the input image. Inputs Image**: The input image to be upscaled and enhanced. Scale**: The factor by which to scale the image, with a default of 4 and a range of 0 to 10. Face Enhance**: A boolean flag to enable or disable face enhancement on the output image. Outputs Output**: The upscaled and enhanced version of the input image. Capabilities The real-esrgan model is capable of producing high-quality, visually appealing upscaled images with optional face enhancement. It can effectively restore details and sharpness to low-resolution inputs, making it a valuable tool for tasks such as image restoration, photo editing, and digital art creation. What can I use it for? The real-esrgan model can be used in a variety of applications where high-quality image upscaling and enhancement are required. This includes professional photography, graphic design, video production, and even personal photo editing. By leveraging the power of this model, users can transform low-resolution images into high-resolution masterpieces, opening up new creative possibilities and improving the visual quality of their work. Things to try One interesting aspect of the real-esrgan model is its ability to handle large input images. By adjusting the scale parameter, users can upscale images to even greater resolutions, potentially unlocking new use cases in fields like medical imaging, satellite imagery, and architectural visualization. Additionally, the face enhancement feature can be a valuable tool for portrait photographers or anyone interested in improving the appearance of faces in their images.
Updated Invalid Date
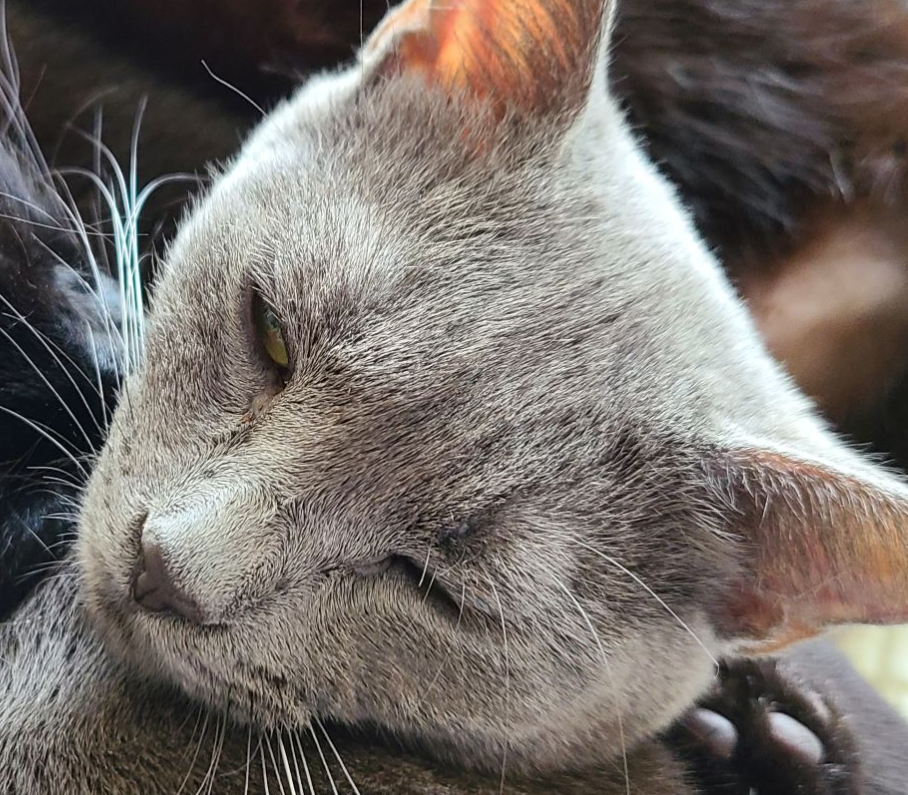
real-esrgan

49.7K
real-esrgan is a practical image restoration model developed by researchers at the Tencent ARC Lab and Shenzhen Institutes of Advanced Technology. It aims to tackle real-world blind super-resolution, going beyond simply enhancing image quality. Compared to similar models like absolutereality-v1.8.1, instant-id, clarity-upscaler, and reliberate-v3, real-esrgan is specifically focused on restoring real-world images and videos, including those with face regions. Model inputs and outputs real-esrgan takes an input image and outputs an upscaled and enhanced version of that image. The model can handle a variety of input types, including regular images, images with alpha channels, and even grayscale images. The output is a high-quality, visually appealing image that retains important details and features. Inputs Image**: The input image to be upscaled and enhanced. Scale**: The desired scale factor for upscaling the input image, typically between 2x and 4x. Face Enhance**: An optional flag to enable face enhancement using the GFPGAN model. Outputs Output Image**: The restored and upscaled version of the input image. Capabilities real-esrgan is capable of performing high-quality image upscaling and restoration, even on challenging real-world images. It can handle a variety of input types and produces visually appealing results that maintain important details and features. The model can also be used to enhance facial regions in images, thanks to its integration with the GFPGAN model. What can I use it for? real-esrgan can be useful for a variety of applications, such as: Photo Restoration**: Upscale and enhance low-quality or blurry photos to create high-resolution, visually appealing images. Video Enhancement**: Apply real-esrgan to individual frames of a video to improve the overall visual quality and clarity. Anime and Manga Upscaling**: The RealESRGAN_x4plus_anime_6B model is specifically optimized for anime and manga images, producing excellent results. Things to try Some interesting things to try with real-esrgan include: Experiment with different scale factors to find the optimal balance between quality and performance. Combine real-esrgan with other image processing techniques, such as denoising or color correction, to achieve even better results. Explore the model's capabilities on a wide range of input images, from natural photographs to detailed illustrations and paintings. Try the RealESRGAN_x4plus_anime_6B model for enhancing anime and manga-style images, and compare the results to other upscaling solutions.
Updated Invalid Date
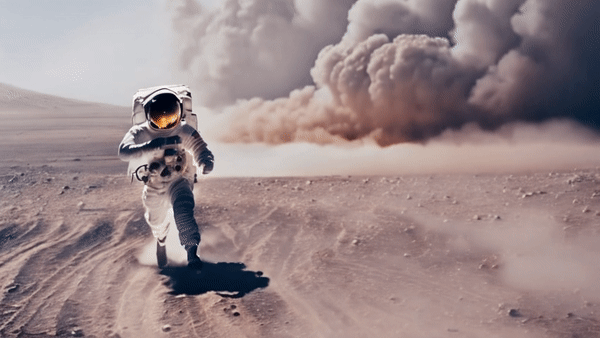
video-crafter

16
video-crafter is an open diffusion model for high-quality video generation developed by lucataco. It is similar to other diffusion-based text-to-image models like stable-diffusion but with the added capability of generating videos from text prompts. video-crafter can produce cinematic videos with dynamic scenes and movement, such as an astronaut running away from a dust storm on the moon. Model inputs and outputs video-crafter takes in a text prompt that describes the desired video and outputs a GIF file containing the generated video. The model allows users to customize various parameters like the frame rate, video dimensions, and number of steps in the diffusion process. Inputs Prompt**: The text description of the video to generate Fps**: The frames per second of the output video Seed**: The random seed to use for generation (leave blank to randomize) Steps**: The number of steps to take in the video generation process Width**: The width of the output video Height**: The height of the output video Outputs Output**: A GIF file containing the generated video Capabilities video-crafter is capable of generating highly realistic and dynamic videos from text prompts. It can produce a wide range of scenes and scenarios, from fantastical to everyday, with impressive visual quality and smooth movement. The model's versatility is evident in its ability to create videos across diverse genres, from cinematic sci-fi to slice-of-life vignettes. What can I use it for? video-crafter could be useful for a variety of applications, such as creating visual assets for films, games, or marketing campaigns. Its ability to generate unique video content from simple text prompts makes it a powerful tool for content creators and animators. Additionally, the model could be leveraged for educational or research purposes, allowing users to explore the intersection of language, visuals, and motion. Things to try One interesting aspect of video-crafter is its capacity to capture dynamic, cinematic scenes. Users could experiment with prompts that evoke a sense of movement, action, or emotional resonance, such as "a lone explorer navigating a lush, alien landscape" or "a family gathered around a crackling fireplace on a snowy evening." The model's versatility also lends itself to more abstract or surreal prompts, allowing users to push the boundaries of what is possible in the realm of generative video.
Updated Invalid Date
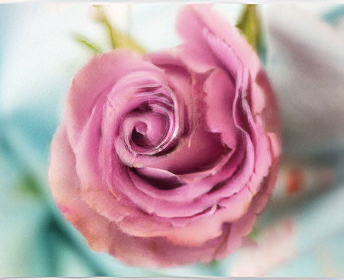
esrgan

75
The esrgan model is an image super-resolution model that can upscale low-resolution images by 4x. It was developed by researchers at Tencent and the Chinese Academy of Sciences, and is an enhancement of the SRGAN model. The esrgan model uses a deeper neural network architecture called Residual-in-Residual Dense Blocks (RRDB) without batch normalization layers, which helps it achieve superior performance compared to previous models like SRGAN. It also employs the Relativistic average GAN loss function and improved perceptual loss to further boost image quality. The esrgan model can be seen as a more advanced version of the Real-ESRGAN model, which is a practical algorithm for real-world image restoration that can also remove JPEG compression artifacts. The Real-ESRGAN model extends the original esrgan with additional features and improvements. Model inputs and outputs Inputs Image**: A low-resolution input image that the model will upscale by 4x. Outputs Image**: The output of the model is a high-resolution image that is 4 times the size of the input. Capabilities The esrgan model can effectively upscale low-resolution images while preserving important details and textures. It outperforms previous state-of-the-art super-resolution models on standard benchmarks like Set5, Set14, and BSD100 in terms of both PSNR and perceptual quality. The model is particularly adept at handling complex textures and details that can be challenging for other super-resolution approaches. What can I use it for? The esrgan model can be useful for a variety of applications that require high-quality image upscaling, such as enhancing old photos, improving the resolution of security camera footage, or generating high-res images from low-res inputs for graphic design and media production. Companies could potentially use the esrgan model to improve the visual quality of their products or services, such as by upscaling product images on an ecommerce site or enhancing the resolution of user-generated content. Things to try One interesting aspect of the esrgan model is its network interpolation capability, which allows you to smoothly transition between the high-PSNR and high-perceptual quality versions of the model. By adjusting the interpolation parameter, you can find the right balance between visual fidelity and objective image quality metrics to suit your specific needs. This can be a powerful tool for fine-tuning the model's performance for different use cases.
Updated Invalid Date