realistic
Maintainer: zhouzhengjun

5
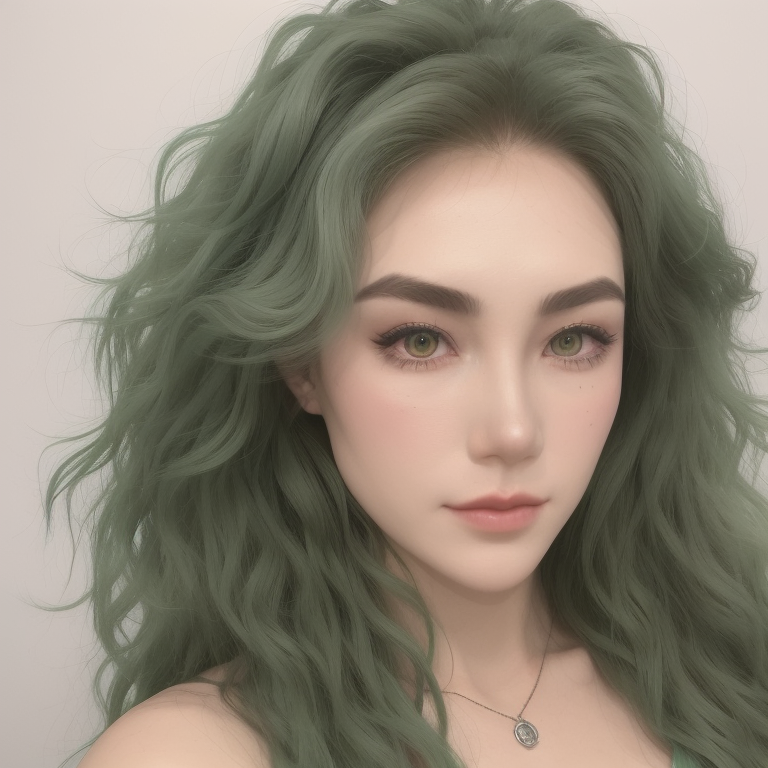
Property | Value |
---|---|
Model Link | View on Replicate |
API Spec | View on Replicate |
Github Link | No Github link provided |
Paper Link | No paper link provided |
Create account to get full access
Model overview
realistic
is an AI model developed by zhouzhengjun, a contributor on the Replicate platform. This model is part of a suite of AI models created by zhouzhengjun, including gfpgan, lora_inpainting, lora_openjourney_v4, and real-esrgan. The model's purpose is to generate realistic images based on text prompts.
Model inputs and outputs
The realistic
model takes in a variety of inputs, including a text prompt, image seed, and various parameters like image size, number of outputs, and guidance scale. The outputs are an array of image URIs representing the generated images.
Inputs
- Prompt: The text prompt that describes what the model should generate.
- Image: An optional initial image to use as a starting point for generation.
- Width/Height: The desired width and height of the output images.
- Number of outputs: The number of images to generate.
- Guidance scale: A parameter that controls the balance between the text prompt and the initial image.
- Negative prompt: Text that describes what the model should avoid generating.
Outputs
- Array of image URIs: The generated images as a list of URIs.
Capabilities
The realistic
model is capable of generating highly detailed and photorealistic images based on text prompts. It can create a wide variety of scenes, objects, and characters, including some that may be challenging for other text-to-image models, such as complex landscapes or intricate details.
What can I use it for?
The realistic
model could be used for a variety of creative projects, such as generating concept art, illustrations, or even product visualizations. Its ability to create photorealistic images may also make it useful for tasks like image restoration or enhancement. As with any powerful text-to-image model, it's important to consider the ethical implications of its use, such as potential biases or the creation of misleading imagery.
Things to try
One interesting aspect of the realistic
model is its ability to incorporate additional context through the use of LoRA (Low-Rank Adaptation) models. By providing URLs for pre-trained LoRA models, users can fine-tune the model's outputs to align with specific styles or subject matter. This could be a powerful way to customize the model's capabilities for your specific needs.
This summary was produced with help from an AI and may contain inaccuracies - check out the links to read the original source documents!
Related Models
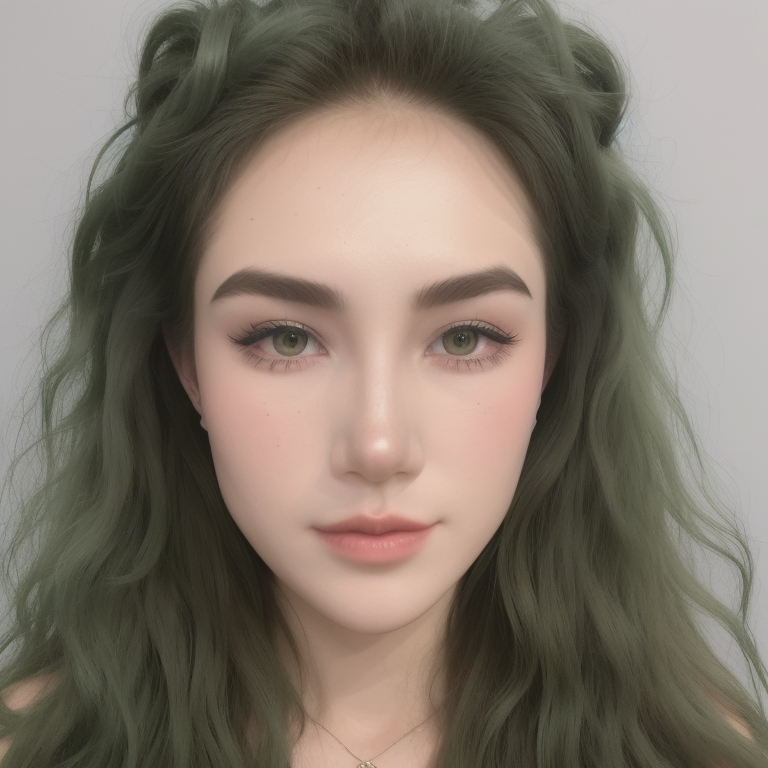
lora_inpainting

14
lora_inpainting is a powerful AI model developed by zhouzhengjun that can perform inpainting on images. It is an improved version of the SDRV_2.0 model. lora_inpainting can be used to seamlessly fill in missing or damaged areas of an image, making it a valuable tool for tasks like photo restoration, image editing, and creative content generation. While similar to models like LAMA, ad-inpaint, and sdxl-outpainting-lora, lora_inpainting offers its own unique capabilities and use cases. Model inputs and outputs lora_inpainting takes in an image, a mask, and various optional parameters like a prompt, guidance scale, and seed. The model then generates a new image with the specified areas inpainted, preserving the original content and seamlessly blending in the generated elements. The output is an array of one or more images, allowing users to choose the best result or experiment with different variations. Inputs Image**: The initial image to generate variations of. This can be used for Img2Img tasks. Mask**: A black and white image used to specify the areas to be inpainted. Prompt**: The input prompt, which can use tags like , , etc. to specify LoRA concepts. Negative Prompt**: Specify things the model should not include in the output. Num Outputs**: The number of images to generate. Guidance Scale**: The scale for classifier-free guidance. Num Inference Steps**: The number of denoising steps to perform. Scheduler**: The scheduling algorithm to use. LoRA URLs**: A list of URLs for LoRA model weights to be applied. LoRA Scales**: A list of scales for the LoRA models. Seed**: The random seed to use. Outputs An array of one or more images, with the specified areas inpainted. Capabilities lora_inpainting excels at seamlessly filling in missing or damaged areas of an image while preserving the original content and style. This makes it a powerful tool for tasks like photo restoration, image editing, and content generation. The model can handle a wide range of image types and styles, and the ability to apply LoRA models adds even more flexibility and customization options. What can I use it for? lora_inpainting can be used for a variety of applications, such as: Photo Restoration**: Repair old, damaged, or incomplete photos by inpainting missing or corrupted areas. Image Editing**: Seamlessly remove unwanted elements from images or add new content to existing scenes. Creative Content Generation**: Generate unique and compelling images by combining input prompts with LoRA models. Product Advertising**: Create professional-looking product images by inpainting over backgrounds or adding promotional elements. Things to try One interesting aspect of lora_inpainting is its ability to blend in generated content with the original image in a very natural and unobtrusive way. This can be especially useful for tasks like photo restoration, where the model can fill in missing details or repair damaged areas without disrupting the overall composition and style of the image. Experiment with different prompts, LoRA models, and parameter settings to see how the model responds and the range of results it can produce.
Updated Invalid Date
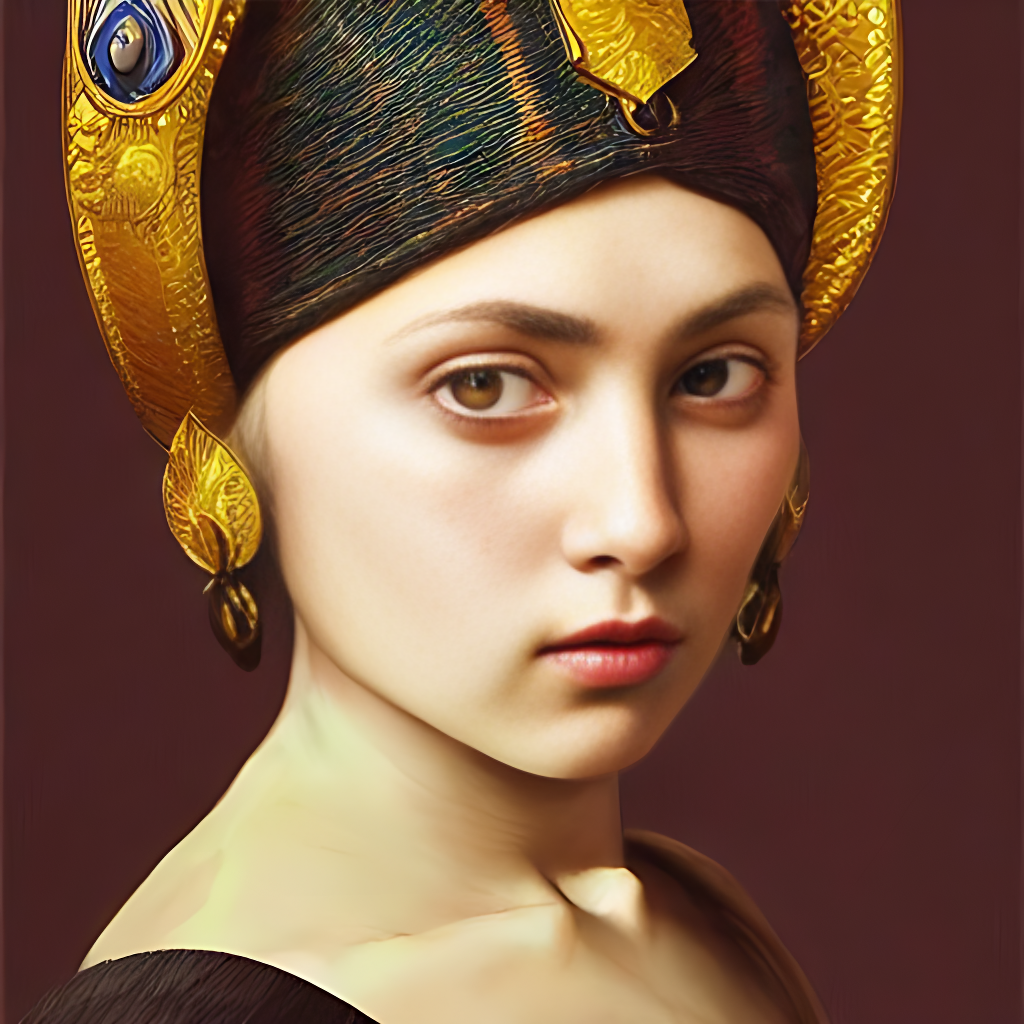
gfpgan

76.7K
gfpgan is a practical face restoration algorithm developed by the Tencent ARC team. It leverages the rich and diverse priors encapsulated in a pre-trained face GAN (such as StyleGAN2) to perform blind face restoration on old photos or AI-generated faces. This approach contrasts with similar models like Real-ESRGAN, which focuses on general image restoration, or PyTorch-AnimeGAN, which specializes in anime-style photo animation. Model inputs and outputs gfpgan takes an input image and rescales it by a specified factor, typically 2x. The model can handle a variety of face images, from low-quality old photos to high-quality AI-generated faces. Inputs Img**: The input image to be restored Scale**: The factor by which to rescale the output image (default is 2) Version**: The gfpgan model version to use (v1.3 for better quality, v1.4 for more details and better identity) Outputs Output**: The restored face image Capabilities gfpgan can effectively restore a wide range of face images, from old, low-quality photos to high-quality AI-generated faces. It is able to recover fine details, fix blemishes, and enhance the overall appearance of the face while preserving the original identity. What can I use it for? You can use gfpgan to restore old family photos, enhance AI-generated portraits, or breathe new life into low-quality images of faces. The model's capabilities make it a valuable tool for photographers, digital artists, and anyone looking to improve the quality of their facial images. Additionally, the maintainer tencentarc offers an online demo on Replicate, allowing you to try the model without setting up the local environment. Things to try Experiment with different input images, varying the scale and version parameters, to see how gfpgan can transform low-quality or damaged face images into high-quality, detailed portraits. You can also try combining gfpgan with other models like Real-ESRGAN to enhance the background and non-facial regions of the image.
Updated Invalid Date
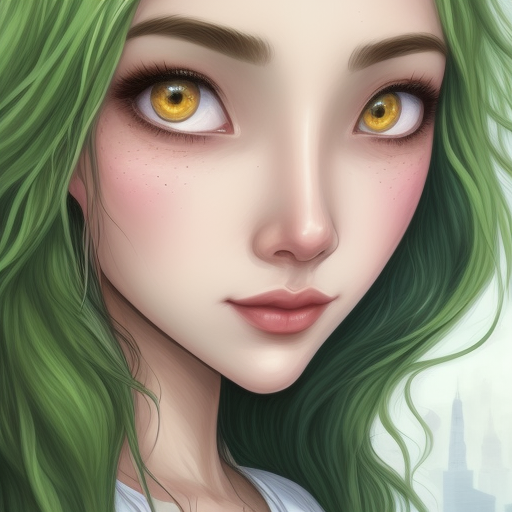
lora_openjourney_v4

18
lora_openjourney_v4 is a powerful AI model developed by zhouzhengjun, as detailed on their creator profile. This model builds upon the capabilities of the openjourney model, incorporating LoRA (Low-Rank Adaptation) techniques to enhance its performance. It is designed to generate high-quality, creative images based on textual prompts. The lora_openjourney_v4 model shares similarities with other LoRA-based models such as lora_inpainting, Style-lora-all, open-dalle-1.1-lora, and Genshin-lora-all, all of which leverage LoRA techniques to enhance their image generation capabilities. Model inputs and outputs The lora_openjourney_v4 model accepts a variety of inputs, including a text prompt, an optional image for inpainting, and various parameters to control the output, such as the image size, number of outputs, and guidance scale. The model then generates one or more images based on the provided inputs. Inputs Prompt**: The text prompt that describes the desired image. Image**: An optional image to be used as a starting point for inpainting. Seed**: A random seed to control the generation process. Width and Height**: The desired dimensions of the output image. Number of Outputs**: The number of images to generate. Guidance Scale**: A value to control the balance between the prompt and the model's own biases. Negative Prompt**: Text to specify things that should not be present in the output. LoRA URLs and Scales**: URLs and scales for LoRA models to be applied. Scheduler**: The algorithm used to generate the output images. Outputs The model outputs one or more images as specified by the "Num Outputs" input parameter. The output images are returned as a list of URIs. Capabilities The lora_openjourney_v4 model is capable of generating high-quality, creative images based on text prompts. It can handle a wide range of subject matter, from fantastical scenes to realistic portraits, and it is particularly adept at incorporating LoRA-based techniques to enhance the visual fidelity and coherence of the output. What can I use it for? The lora_openjourney_v4 model can be used for a variety of creative and artistic applications, such as concept art, illustration, and product design. Its ability to generate unique and compelling images based on textual prompts makes it a valuable tool for artists, designers, and creative professionals who need to quickly generate visual ideas. Additionally, the model's versatility and customization options (such as the ability to apply LoRA models) make it a flexible solution for businesses and individuals who want to create visually striking content for their products, services, or marketing campaigns. Things to try Experiment with different prompts to see the range of images the lora_openjourney_v4 model can generate. Try combining the model with other LoRA-based models, such as those mentioned earlier, to explore the synergies and unique capabilities that can arise from these combinations. Additionally, consider using the model's inpainting functionality to seamlessly incorporate existing images into new, imaginative compositions. The ability to fine-tune the model's output through parameters like guidance scale and negative prompts can also be a valuable tool for refining and optimizing the generated images.
Updated Invalid Date
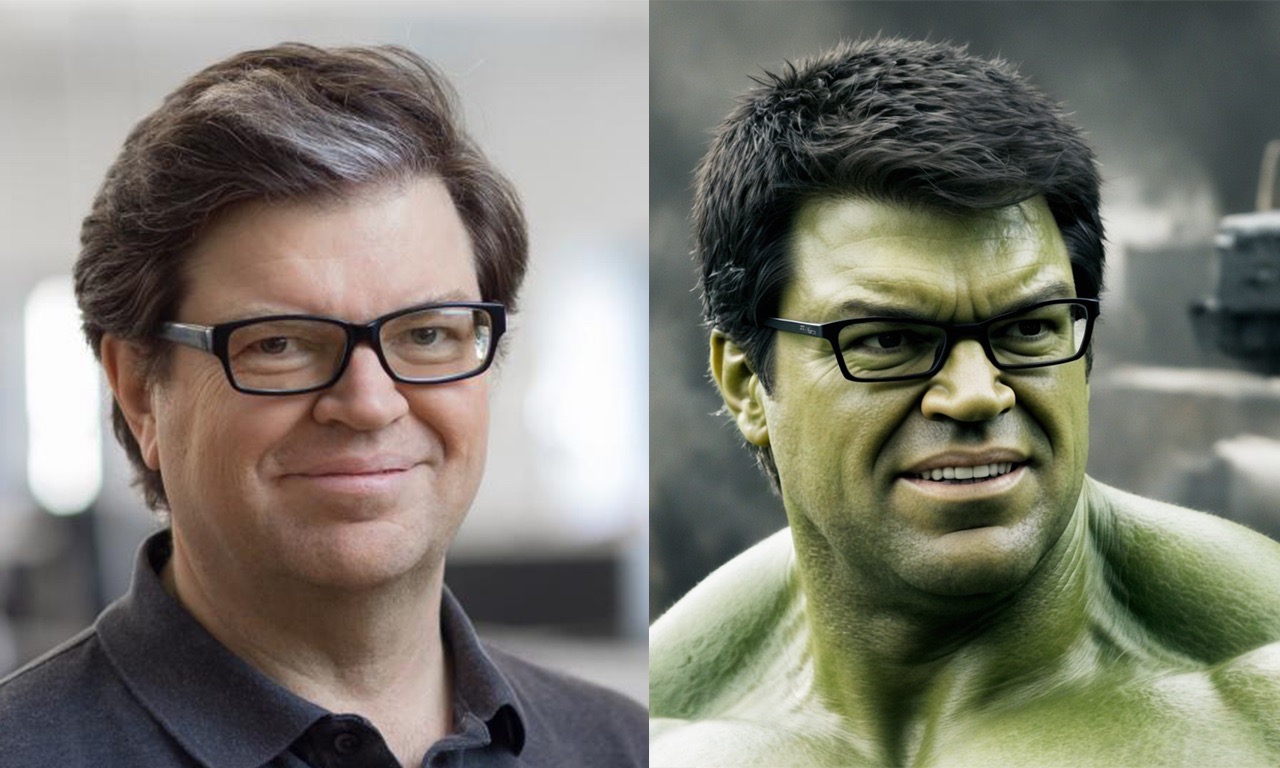
instant-id

487
instant-id is a state-of-the-art AI model developed by the InstantX team that can generate realistic images of real people instantly. It utilizes a tuning-free approach to achieve identity-preserving generation with only a single input image. The model is capable of various downstream tasks such as stylized synthesis, where it can blend the facial features and style of the input image. Compared to similar models like AbsoluteReality V1.8.1, Reliberate v3, Stable Diffusion, Photomaker, and Photomaker Style, instant-id achieves better fidelity and retains good text editability, allowing the generated faces and styles to blend more seamlessly. Model inputs and outputs instant-id takes a single input image of a face and a text prompt, and generates one or more realistic images that preserve the identity of the input face while incorporating the desired style and content from the text prompt. The model utilizes a novel identity-preserving generation technique that allows it to generate high-quality, identity-preserving images in a matter of seconds. Inputs Image**: The input face image used as a reference for the generated images. Prompt**: The text prompt describing the desired style and content of the generated images. Seed** (optional): A random seed value to control the randomness of the generated images. Pose Image** (optional): A reference image used to guide the pose of the generated images. Outputs Images**: One or more realistic images that preserve the identity of the input face while incorporating the desired style and content from the text prompt. Capabilities instant-id is capable of generating highly realistic images of people in a variety of styles and settings, while preserving the identity of the input face. The model can seamlessly blend the facial features and style of the input image, allowing for unique and captivating results. This makes the model a powerful tool for a wide range of applications, from creative content generation to virtual avatars and character design. What can I use it for? instant-id can be used for a variety of applications, such as: Creative Content Generation**: Quickly generate unique and realistic images for use in art, design, and multimedia projects. Virtual Avatars**: Create personalized virtual avatars that can be used in games, social media, or other digital environments. Character Design**: Develop realistic and expressive character designs for use in animation, films, or video games. Augmented Reality**: Integrate generated images into augmented reality experiences, allowing for the seamless blending of real and virtual elements. Things to try With instant-id, you can experiment with a wide range of text prompts and input images to generate unique and captivating results. Try prompts that explore different styles, genres, or themes, and see how the model can blend the facial features and aesthetics in unexpected ways. You can also experiment with different input images, from close-up portraits to more expressive or stylized faces, to see how the model adapts and responds. By pushing the boundaries of what's possible with identity-preserving generation, you can unlock a world of creative possibilities.
Updated Invalid Date