sd-inpaint
Maintainer: zf-kbot

1.3K
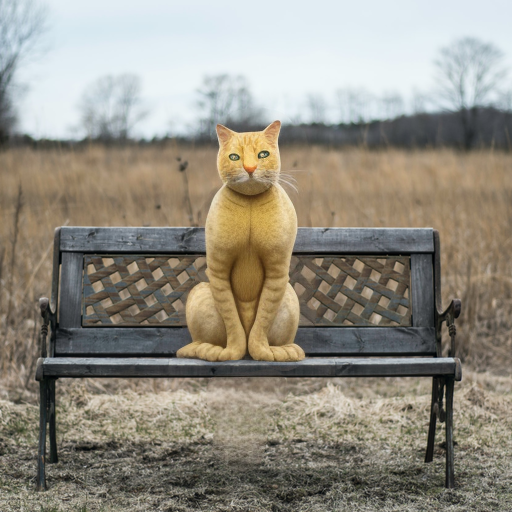
Property | Value |
---|---|
Run this model | Run on Replicate |
API spec | View on Replicate |
Github link | No Github link provided |
Paper link | No paper link provided |
Create account to get full access
Model overview
The sd-inpaint
model is a powerful AI tool developed by zf-kbot that allows users to fill in masked parts of images using Stable Diffusion. It is similar to other inpainting models like stable-diffusion-inpainting, stable-diffusion-wip, and flux-dev-inpainting, all of which aim to provide users with the ability to modify and enhance existing images.
Model inputs and outputs
The sd-inpaint
model takes a number of inputs, including the input image, a mask, a prompt, and various settings like the seed, guidance scale, and scheduler. The model then generates one or more output images that fill in the masked areas based on the provided prompt and settings.
Inputs
- Image: The input image to be inpainted
- Mask: The mask that defines the areas to be inpainted
- Prompt: The text prompt that guides the inpainting process
- Seed: The random seed to use for the image generation
- Guidance Scale: The scale for the classifier-free guidance
- Scheduler: The scheduler to use for the image generation
Outputs
- Output Images: One or more images that have been inpainted based on the input prompt and settings
Capabilities
The sd-inpaint
model is capable of generating high-quality inpainted images that seamlessly blend the generated content with the original image. This can be useful for a variety of applications, such as removing unwanted elements from photos, completing partially obscured images, or creating new content within existing images.
What can I use it for?
The sd-inpaint
model can be used for a wide range of creative and practical applications. For example, you could use it to remove unwanted objects from photos, fill in missing portions of an image, or even create new art by generating content within a specified mask. The model's versatility makes it a valuable tool for designers, artists, and content creators who need to modify and enhance existing images.
Things to try
One interesting thing to try with the sd-inpaint
model is to experiment with different prompts and settings to see how they affect the generated output. You could try varying the prompt complexity, adjusting the guidance scale, or using different schedulers to see how these factors influence the inpainting results. Additionally, you could explore using the model in combination with other image processing tools to create more complex and sophisticated image manipulations.
This summary was produced with help from an AI and may contain inaccuracies - check out the links to read the original source documents!
Related Models
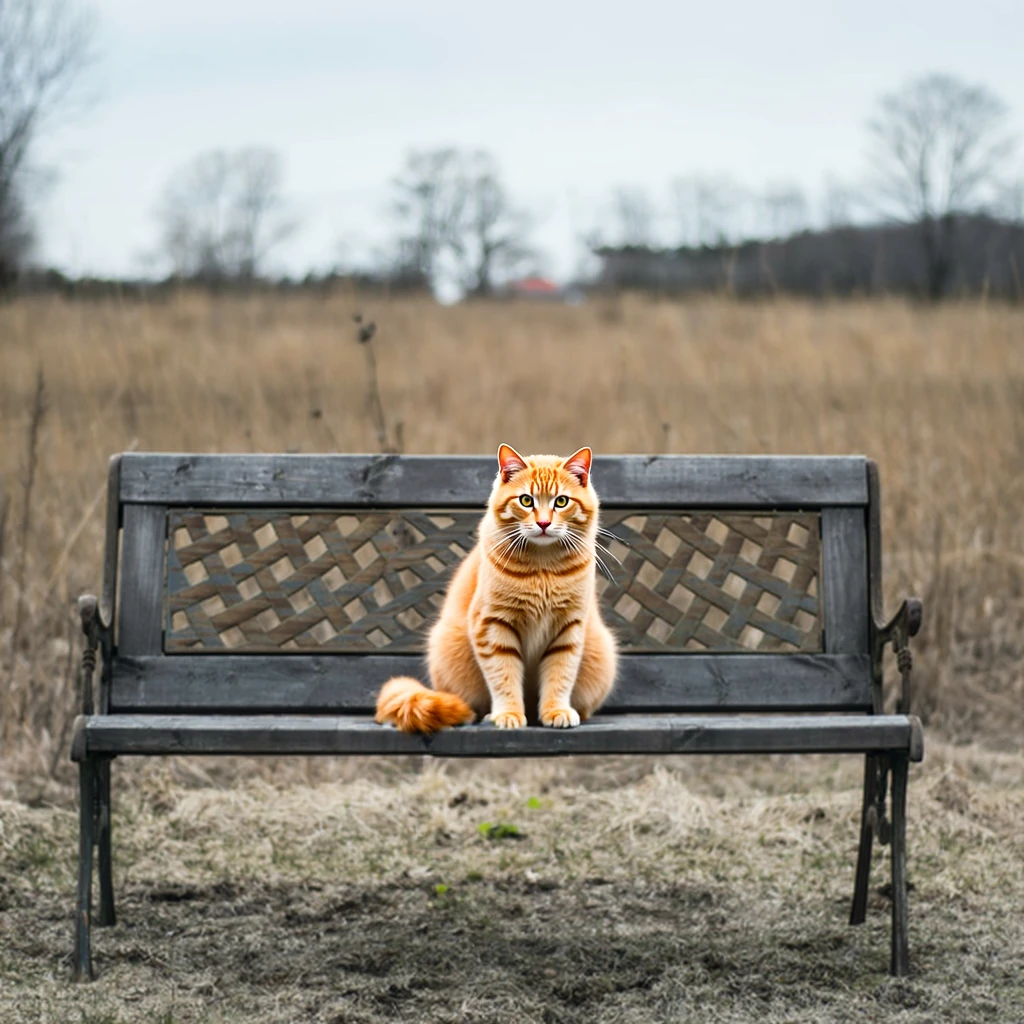
flux-dev-inpainting

18
flux-dev-inpainting is an AI model developed by zsxkib that can fill in masked parts of images. This model is similar to other inpainting models like stable-diffusion-inpainting, sdxl-inpainting, and inpainting-xl, which use Stable Diffusion or other diffusion models to generate content that fills in missing regions of an image. Model inputs and outputs The flux-dev-inpainting model takes several inputs to control the inpainting process: Inputs Mask**: The mask image that defines the region to be inpainted Image**: The input image to be inpainted Prompt**: The text prompt that guides the inpainting process Strength**: The strength of the inpainting, ranging from 0 to 1 Seed**: The random seed to use for the inpainting process Output Format**: The format of the output image (e.g. WEBP) Output Quality**: The quality of the output image, from 0 to 100 Outputs Output**: The inpainted image Capabilities The flux-dev-inpainting model can generate realistic and visually coherent content to fill in masked regions of an image. It can handle a wide range of image types and prompts, and produces high-quality output. The model is particularly adept at preserving the overall style and composition of the original image while seamlessly integrating the inpainted content. What can I use it for? You can use flux-dev-inpainting for a variety of image editing and manipulation tasks, such as: Removing unwanted objects or elements from an image Filling in missing or damaged parts of an image Creating new image content by inpainting custom prompts Experimenting with different inpainting techniques and styles The model's capabilities make it a powerful tool for creative projects, photo editing, and visual content production. You can also explore using flux-dev-inpainting in combination with other FLUX-based models for more advanced image-to-image workflows. Things to try Try experimenting with different input prompts and masks to see how the model handles various inpainting challenges. You can also play with the strength and seed parameters to generate diverse output and explore the model's creative potential. Additionally, consider combining flux-dev-inpainting with other image processing techniques, such as segmentation or style transfer, to create unique visual effects and compositions.
Updated Invalid Date
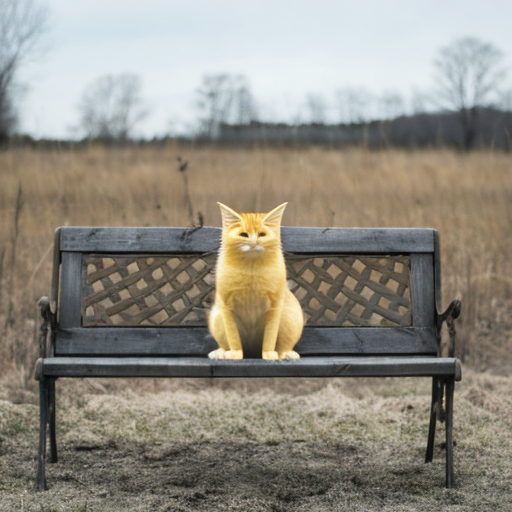
stable-diffusion-inpainting

18.2K
stable-diffusion-inpainting is a model created by Stability AI that can fill in masked parts of images using the Stable Diffusion text-to-image model. It is built on top of the Diffusers Stable Diffusion v2 model and can be used to edit and manipulate images in a variety of ways. This model is similar to other inpainting models like GFPGAN, which focuses on face restoration, and Real-ESRGAN, which can enhance the resolution of images. Model inputs and outputs The stable-diffusion-inpainting model takes in an initial image, a mask indicating which parts of the image to inpaint, and a prompt describing the desired output. It then generates a new image with the masked areas filled in based on the given prompt. The model can produce multiple output images based on a single input. Inputs Prompt**: A text description of the desired output image. Image**: The initial image to be inpainted. Mask**: A black and white image used to indicate which parts of the input image should be inpainted. Seed**: An optional random seed to control the generated output. Scheduler**: The scheduling algorithm to use during the diffusion process. Guidance Scale**: A value controlling the trade-off between following the prompt and staying close to the original image. Negative Prompt**: A text description of things to avoid in the generated image. Num Inference Steps**: The number of denoising steps to perform during the diffusion process. Disable Safety Checker**: An option to disable the safety checker, which can be useful for certain applications. Outputs Image(s)**: One or more new images with the masked areas filled in based on the provided prompt. Capabilities The stable-diffusion-inpainting model can be used to edit and manipulate images in a variety of ways. For example, you could use it to remove unwanted objects or people from a photograph, or to fill in missing parts of an image. The model can also be used to generate entirely new images based on a text prompt, similar to other text-to-image models like Kandinsky 2.2. What can I use it for? The stable-diffusion-inpainting model can be useful for a variety of applications, such as: Photo editing**: Removing unwanted elements, fixing blemishes, or enhancing photos. Creative projects**: Generating new images based on text prompts or combining elements from different images. Content generation**: Producing visuals for articles, social media posts, or other digital content. Prototype creation**: Quickly mocking up designs or visualizing concepts. Companies could potentially monetize this model by offering image editing and manipulation services, or by incorporating it into creative tools or content generation platforms. Things to try One interesting thing to try with the stable-diffusion-inpainting model is to use it to remove or replace specific elements in an image, such as a person or object. You could then generate a new image that fills in the masked area based on the prompt, creating a seamless edit. Another idea is to use the model to combine elements from different images, such as placing a castle in a forest scene or adding a dragon to a cityscape.
Updated Invalid Date
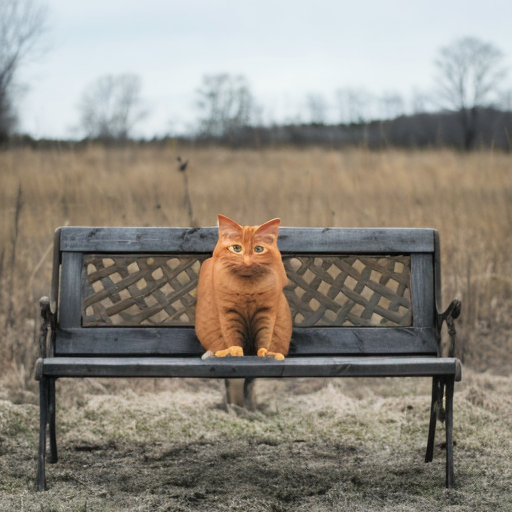
stable-diffusion-v2-inpainting

62
stable-diffusion-v2-inpainting is a text-to-image AI model that can generate variations of an image while preserving specific regions. This model builds on the capabilities of the Stable Diffusion model, which can generate photo-realistic images from text prompts. The stable-diffusion-v2-inpainting model adds the ability to inpaint, or fill in, specific areas of an image while preserving the rest of the image. This can be useful for tasks like removing unwanted objects, filling in missing details, or even creating entirely new content within an existing image. Model inputs and outputs The stable-diffusion-v2-inpainting model takes several inputs to generate new images: Inputs Prompt**: The text prompt that describes the desired image. Image**: The initial image to generate variations of. Mask**: A black and white image used to define the areas of the initial image that should be inpainted. Seed**: A random number that controls the randomness of the generated images. Guidance Scale**: A value that controls the influence of the text prompt on the generated images. Prompt Strength**: A value that controls how much the initial image is modified by the text prompt. Number of Inference Steps**: The number of denoising steps used to generate the final image. Outputs Output images**: One or more images generated based on the provided inputs. Capabilities The stable-diffusion-v2-inpainting model can be used to modify existing images in a variety of ways. For example, you could use it to remove unwanted objects from a photo, fill in missing details, or even create entirely new content within an existing image. The model's ability to preserve the structure and perspective of the original image while generating new content is particularly impressive. What can I use it for? The stable-diffusion-v2-inpainting model could be useful for a wide range of creative and practical applications. For example, you could use it to enhance photos by removing blemishes or unwanted elements, generate concept art for games or movies, or even create custom product images for e-commerce. The model's versatility and ease of use make it a powerful tool for anyone working with visual content. Things to try One interesting thing to try with the stable-diffusion-v2-inpainting model is to use it to create alternative versions of existing artworks or photographs. By providing the model with an initial image and a prompt that describes a desired modification, you can generate unique variations that preserve the original composition while introducing new elements. This could be a fun way to explore creative ideas or generate content for personal projects.
Updated Invalid Date
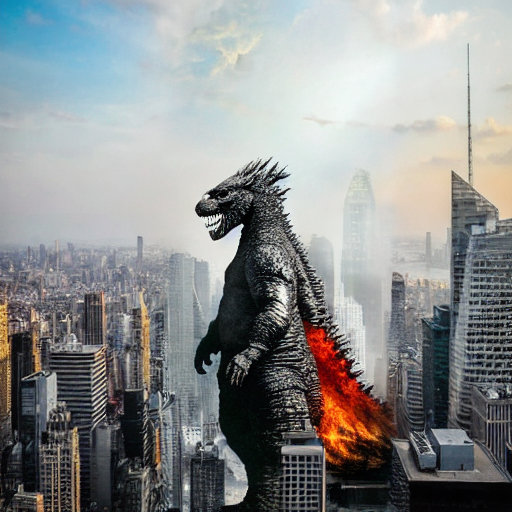
stable-diffusion-wip

13
stable-diffusion-wip is an experimental inpainting model based on the popular Stable Diffusion AI. This model allows you to take an existing image and fill in masked regions with new content generated by the model. It is developed by andreasjansson, who has also created other Stable Diffusion-based models like stable-diffusion-animation. Unlike the production-ready stable-diffusion-inpainting model, this is a work-in-progress version with experimental features. Model inputs and outputs stable-diffusion-wip takes in a variety of inputs to control the inpainting process, including an initial image, a mask image, a text prompt, and various parameters to adjust the output. The model then generates one or more new images based on the provided inputs. Inputs Prompt**: The text prompt that describes the content you want the model to generate. Init Image**: The initial image that you want the model to generate variations of. Mask**: A black and white image used to define the regions of the init image that should be inpainted. Seed**: A random seed value to control the stochastic output of the model. Width/Height**: The desired dimensions of the output image. Num Outputs**: The number of images to generate. Guidance Scale**: A parameter that controls the strength of the text prompt in the generation process. Prompt Strength**: A parameter that controls how much the init image should be preserved in the output. Num Inference Steps**: The number of denoising steps to use during the generation process. Outputs Output Images**: One or more images generated by the model based on the provided inputs. Capabilities stable-diffusion-wip is capable of generating photorealistic images based on a text prompt, while using an existing image as a starting point. The model can fill in masked regions of the image with new content that matches the overall style and composition. This can be useful for tasks like object removal, image editing, and creative visual generation. What can I use it for? With stable-diffusion-wip, you can experiment with inpainting and image editing tasks. For example, you could use it to remove unwanted objects from a photograph, fill in missing parts of an image, or generate new variations of an existing artwork. The model's capabilities can be particularly useful for creative professionals, such as digital artists, designers, and photographers, who are looking to enhance and manipulate their visual content. Things to try One interesting thing to try with stable-diffusion-wip is to experiment with the prompt strength parameter. By adjusting this value, you can control the balance between preserving the original image and generating new content. Lower prompt strength values will result in output that is closer to the init image, while higher values will lead to more dramatic changes. This can be a useful technique for gradually transitioning an image towards a desired style or composition.
Updated Invalid Date