Nateraw
Models by this creator
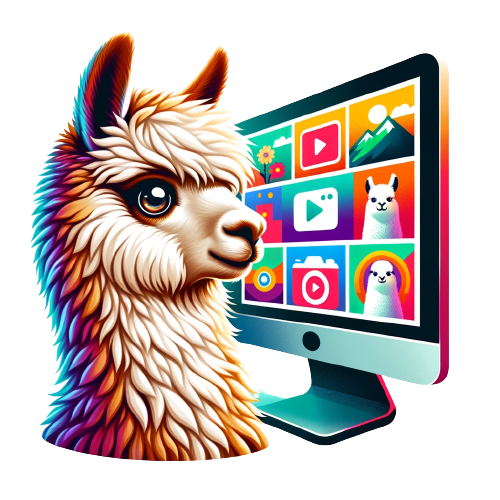
video-llava

466
Video-LLaVA is a powerful AI model developed by the PKU-YuanGroup that exhibits remarkable interactive capabilities between images and videos. The model is built upon the foundations of LLaVA, an efficient large language and vision assistant, and it showcases significant superiority when compared to models specifically designed for either images or videos. The key innovation of Video-LLaVA lies in its ability to learn a united visual representation by aligning it with the language feature space before projection. This approach enables the model to perform visual reasoning capabilities on both images and videos simultaneously, despite the absence of image-video pairs in the dataset. The extensive experiments conducted by the researchers demonstrate the complementarity of modalities, highlighting the model's remarkable performance across a wide range of tasks. Model Inputs and Outputs Video-LLaVA is a versatile model that can handle both image and video inputs, allowing for a diverse range of applications. The model's inputs and outputs are as follows: Inputs Image Path**: The path to an image file that the model can process and analyze. Video Path**: The path to a video file that the model can process and analyze. Text Prompt**: A natural language prompt that the model can use to generate relevant responses based on the provided image or video. Outputs Output**: The model's response to the provided text prompt, which can be a description, analysis, or other relevant information about the input image or video. Capabilities Video-LLaVA exhibits remarkable capabilities in both image and video understanding tasks. The model can perform various visual reasoning tasks, such as answering questions about the content of an image or video, generating captions, and even engaging in open-ended conversations about the visual information. One of the key highlights of Video-LLaVA is its ability to leverage the complementarity of image and video modalities. The model's unified visual representation allows it to excel at tasks that require cross-modal understanding, such as zero-shot video question-answering, where it outperforms models designed specifically for either images or videos. What Can I Use It For? Video-LLaVA can be a valuable tool in a wide range of applications, from content creation and analysis to educational and research purposes. Some potential use cases include: Video Summarization and Captioning**: The model can generate concise summaries or detailed captions for video content, making it useful for video indexing, search, and recommendation systems. Visual Question Answering**: Video-LLaVA can answer questions about the content of images and videos, enabling interactive and informative experiences for users. Video-based Dialogue Systems**: The model's capabilities in understanding and reasoning about visual information can be leveraged to build more engaging and contextual conversational agents. Multimodal Content Generation**: Video-LLaVA can be used to generate creative and coherent content that seamlessly combines visual and textual elements, such as illustrated stories or interactive educational materials. Things to Try With Video-LLaVA's impressive capabilities, there are many exciting possibilities to explore. Here are a few ideas to get you started: Experiment with different text prompts**: Try asking the model a wide range of questions about images and videos, from simple factual queries to more open-ended, creative prompts. Observe how the model's responses vary and how it leverages the visual information. Combine image and video inputs**: Explore the model's ability to reason about and synthesize information from both image and video inputs. See how the model's understanding and responses change when provided with multiple modalities. Fine-tune the model**: If you have domain-specific data or task requirements, consider fine-tuning Video-LLaVA to further enhance its performance in your area of interest. Integrate the model into your applications**: Leverage Video-LLaVA's capabilities to build innovative, multimodal applications that can provide enhanced user experiences or automate visual-based tasks. By exploring the capabilities of Video-LLaVA, you can unlock new possibilities in the realm of large language and vision models, pushing the boundaries of what's possible in the field of artificial intelligence.
Updated 9/18/2024
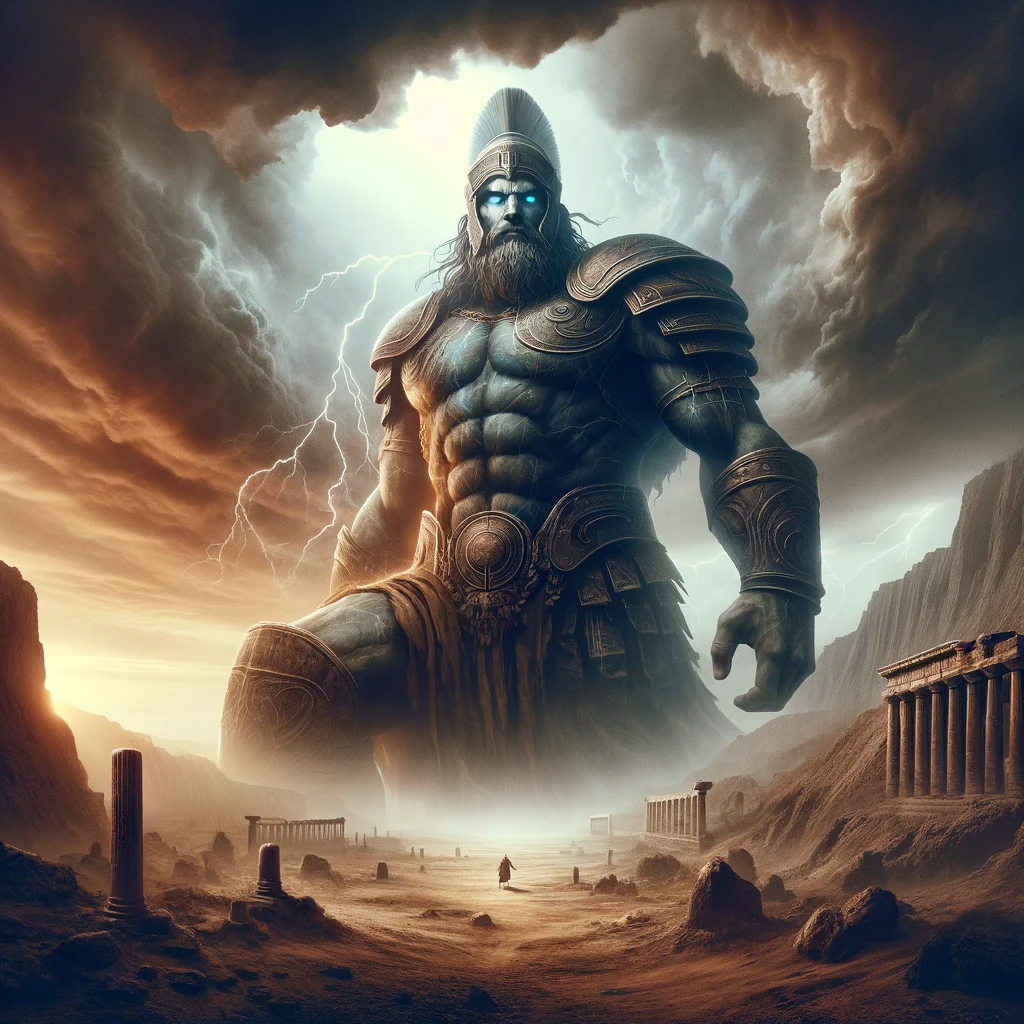
goliath-120b

235
goliath-120b is an auto-regressive causal language model created by combining two fine-tuned Llama-2 70B models into one. Developed by Nateraw, this large language model (LLM) represents an advancement in the Llama 2 line of models, offering increased capability and scale. Similar models in this space include the Mixtral-8x7B and various CodeLlama models, which focus on coding and conversational abilities. Model inputs and outputs goliath-120b is a text-to-text generative model, taking in a prompt as input and generating a response as output. The model allows for customization of several key parameters, including temperature, top-k and top-p filtering, maximum new tokens, and presence and frequency penalties. Inputs Prompt**: The text prompt that the model will use to generate a response. Temperature**: A value used to modulate the next token probabilities, controlling the "creativity" of the model's output. Top K**: The number of highest probability tokens to consider for generating the output. Top P**: A probability threshold for generating the output, using nucleus filtering. Max New Tokens**: The maximum number of tokens the model should generate as output. Outputs Generated Text**: The model's response, generated based on the provided input prompt and parameters. Capabilities goliath-120b is a powerful language model capable of a wide range of text generation tasks, from creative writing to task-oriented dialogue. The model's large size and fine-tuning allow it to produce coherent, contextually-appropriate text with high quality. What can I use it for? goliath-120b can be used for various natural language processing applications, such as chatbots, content generation, and language modeling. The model's versatility makes it a valuable tool for businesses and developers looking to incorporate advanced language capabilities into their products or services. Things to try Experiment with different prompts and parameter settings to see the model's full capabilities. Try using goliath-120b for tasks like story generation, question answering, or code completion to explore its strengths and limitations. The model's large scale and fine-tuning can produce impressive results, but it's important to carefully monitor the outputs and ensure they align with your intended use case.
Updated 9/18/2024
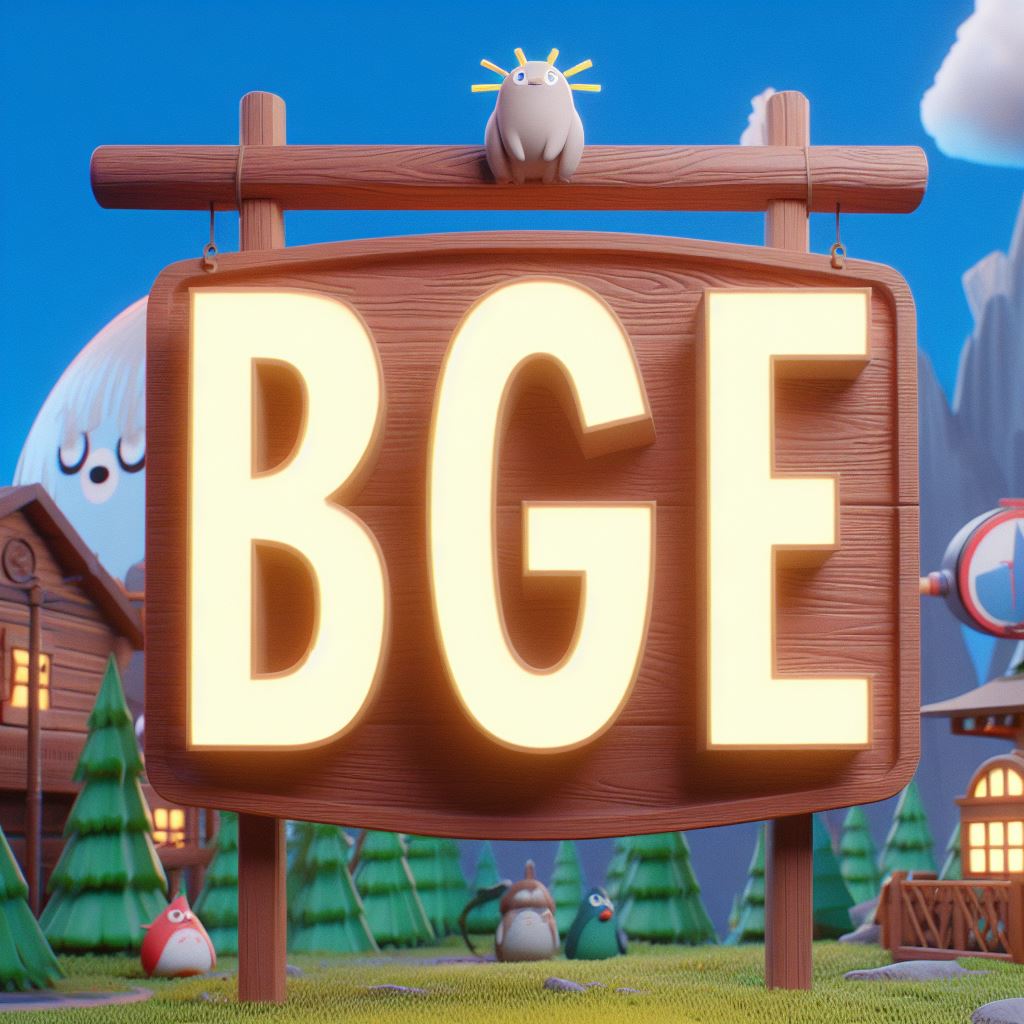
bge-large-en-v1.5

202
The bge-large-en-v1.5 is a text embedding model created by BAAI (Beijing Academy of Artificial Intelligence). It is designed to generate high-quality embeddings for text sequences in English. This model builds upon BAAI's previous work on the bge-reranker-base and multilingual-e5-large models, which have shown strong performance on various language tasks. The bge-large-en-v1.5 model offers enhanced capabilities and is well-suited for a range of natural language processing applications. Model inputs and outputs The bge-large-en-v1.5 model takes text sequences as input and generates corresponding embeddings. Users can provide the text either as a path to a file containing JSONL data with a 'text' field, or as a JSON list of strings. The model also accepts a batch size parameter to control the processing of the input data. Additionally, users can choose to normalize the output embeddings and convert the results to a NumPy format. Inputs Path**: Path to a file containing text as JSONL with a 'text' field or a valid JSON string list. Texts**: Text to be embedded, formatted as a JSON list of strings. Batch Size**: Batch size to use when processing the text data. Convert To Numpy**: Option to return the output as a NumPy file instead of JSON. Normalize Embeddings**: Option to normalize the generated embeddings. Outputs The model outputs the text embeddings, which can be returned either as a JSON array or as a NumPy file, depending on the user's preference. Capabilities The bge-large-en-v1.5 model is capable of generating high-quality text embeddings that capture the semantic and contextual meaning of the input text. These embeddings can be utilized in a wide range of natural language processing tasks, such as text classification, semantic search, and content recommendation. The model's performance has been demonstrated in various benchmarks and real-world applications. What can I use it for? The bge-large-en-v1.5 model can be a valuable tool for developers and researchers working on natural language processing projects. The text embeddings generated by the model can be used as input features for downstream machine learning models, enabling more accurate and efficient text-based applications. For example, the embeddings could be used in sentiment analysis, topic modeling, or to power personalized content recommendations. Things to try To get the most out of the bge-large-en-v1.5 model, you can experiment with different input text formats, batch sizes, and normalization options to find the configuration that works best for your specific use case. You can also explore how the model's performance compares to other similar models, such as the bge-reranker-base and multilingual-e5-large models, to determine the most suitable approach for your needs.
Updated 9/18/2024
🌿
musicgen-songstarter-v0.2

115
musicgen-songstarter-v0.2 is a large, stereo MusicGen model fine-tuned by nateraw on a dataset of melody loops from their Splice sample library. It is intended to be a useful tool for music producers to generate song ideas. Compared to the previous version musicgen-songstarter-v0.1, this new model was trained on 3x more unique, manually-curated samples and is double the size, using a larger large transformer language model. Similar models include the original musicgen from Meta, which can generate music from a prompt or melody, as well as other fine-tuned versions like musicgen-fine-tuner and musicgen-stereo-chord. Model inputs and outputs musicgen-songstarter-v0.2 takes a variety of inputs to control the generated music, including a text prompt, audio file, and various parameters to adjust the sampling and normalization. The model outputs stereo audio at 32kHz. Inputs Prompt**: A description of the music you want to generate Input Audio**: An audio file that will influence the generated music Continuation**: Whether the generated music should continue from the provided audio file or mimic its melody Continuation Start/End**: The start and end times of the audio file to use for continuation Duration**: The duration of the generated audio in seconds Sampling Parameters**: Controls like top_k, top_p, temperature, and classifier_free_guidance to adjust the diversity and influence of the inputs Outputs Audio**: Stereo audio samples in the requested format (e.g. WAV) Capabilities musicgen-songstarter-v0.2 can generate a variety of musical styles and genres based on the provided prompt, including genres like hip hop, soul, jazz, and more. It can also continue or mimic the melody of an existing audio file, making it useful for music producers looking to build on existing ideas. What can I use it for? musicgen-songstarter-v0.2 is a great tool for music producers looking to generate song ideas and sketches. By providing a textual prompt and/or an existing audio file, the model can produce new musical ideas that can be used as a starting point for further development. The model's ability to generate in stereo and mimic existing melodies makes it particularly useful for quickly prototyping new songs. Things to try One interesting capability of musicgen-songstarter-v0.2 is its ability to generate music that adheres closely to the provided inputs, thanks to the "classifier free guidance" parameter. By increasing this value, you can produce outputs that are less diverse but more closely aligned with the desired style and melody. This can be useful for quickly generating variations on a theme or refining a specific musical idea.
Updated 5/30/2024
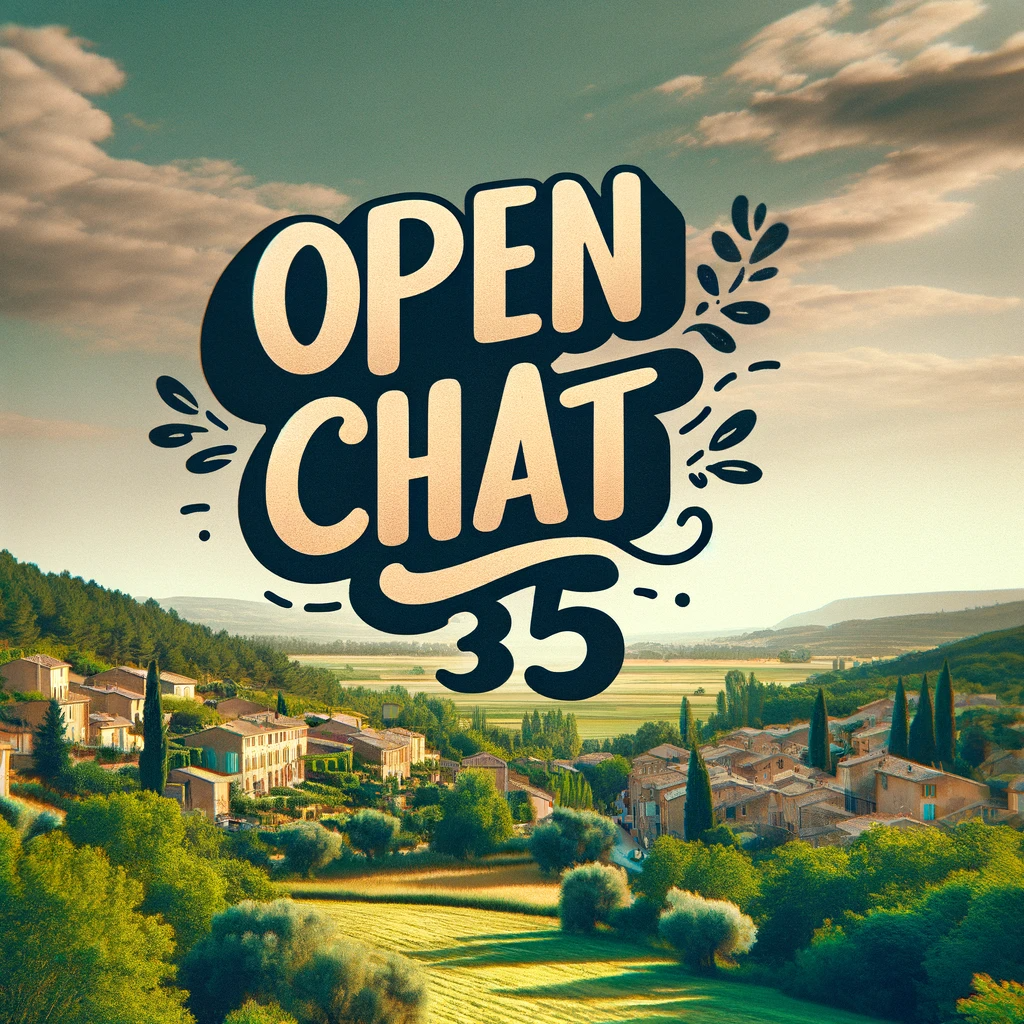
openchat_3.5-awq

101
openchat_3.5-awq is an innovative open-source language model developed by Replicate's nateraw. It is part of the OpenChat library, which includes a series of high-performing models fine-tuned using a strategy called C-RLFT (Contextual Reinforcement Learning from Feedback). This approach allows the models to learn from mixed-quality data without explicit preference labels, delivering exceptional performance on par with ChatGPT despite being a relatively compact 7B model. The OpenChat models outperform other open-source alternatives like OpenHermes 2.5, OpenOrca Mistral, and Zephyr-β on various benchmarks, including reasoning, coding, and mathematical tasks. The latest version, openchat_3.5-0106, even surpasses the capabilities of ChatGPT (March) and Grok-1 on several key metrics. Model Inputs and Outputs Inputs prompt**: The input text prompt for the model to generate a response. max_new_tokens**: The maximum number of tokens the model should generate as output. temperature**: The value used to modulate the next token probabilities. top_p**: A probability threshold for generating the output. If = top_p (nucleus filtering). top_k**: The number of highest probability tokens to consider for generating the output. If > 0, only keep the top k tokens with highest probability (top-k filtering). prompt_template**: The template used to format the prompt. The input prompt is inserted into the template using the {prompt} placeholder. presence_penalty**: The penalty applied to tokens based on their presence in the generated text. frequency_penalty**: The penalty applied to tokens based on their frequency in the generated text. Outputs The model generates a sequence of tokens as output, which can be concatenated to form the model's response. Capabilities openchat_3.5-awq demonstrates strong performance in a variety of tasks, including: Reasoning and Coding**: The model outperforms ChatGPT (March) and other open-source alternatives on coding and reasoning benchmarks like HumanEval, BBH MC, and AGIEval. Mathematical Reasoning**: The model achieves state-of-the-art results on mathematical reasoning tasks like GSM8K, showcasing its ability to tackle complex numerical problems. General Language Understanding**: The model performs well on MMLU, a broad benchmark for general language understanding, indicating its versatility in handling diverse language tasks. What Can I Use It For? The openchat_3.5-awq model can be leveraged for a wide range of applications, such as: Conversational AI**: The model can be deployed as a conversational agent, engaging users in natural language interactions and providing helpful responses. Content Generation**: The model can be used to generate high-quality text, such as articles, stories, or creative writing, by fine-tuning on specific domains or datasets. Task-oriented Dialogue**: The model can be fine-tuned for task-oriented dialogues, such as customer service, technical support, or virtual assistance. Code Generation**: The model's strong performance on coding tasks makes it a valuable tool for automating code generation, programming assistance, or code synthesis. Things to Try Here are some ideas for what you can try with openchat_3.5-awq: Explore the model's capabilities**: Test the model on a variety of tasks, such as open-ended conversations, coding challenges, or mathematical problems, to understand its strengths and limitations. Fine-tune the model**: Leverage the model's strong foundation by fine-tuning it on your specific dataset or domain to create a customized language model for your applications. Combine with other technologies**: Integrate the model with other AI or automation tools, such as voice interfaces or robotic systems, to create more comprehensive and intelligent solutions. Contribute to the open-source ecosystem**: As an open-source model, you can explore ways to improve or extend the OpenChat library, such as by contributing to the codebase, providing feedback, or collaborating on research and development.
Updated 9/18/2024
🛠️
vit-age-classifier

86
The vit-age-classifier is a Vision Transformer (ViT) model that has been fine-tuned to classify the age of a person's face in an image. This model builds upon the Vision Transformer (base-sized model) and the Vision Transformer (base-sized model) pre-trained on ImageNet-21k, which are general-purpose pre-trained image classification models. The vit-age-classifier model has been further trained on a proprietary dataset of facial images to specialize in age prediction. Similar models include the Fine-Tuned Vision Transformer (ViT) for NSFW Image Classification, which can be used for content moderation, and the CLIP model, which can be used for zero-shot image classification. However, the vit-age-classifier is unique in its specialization for facial age prediction. Model inputs and outputs Inputs Image**: The model takes a single image as input, which should contain a human face. Outputs Age prediction**: The model outputs a predicted age for the person in the input image. Capabilities The vit-age-classifier model can be used to accurately predict the age of a person's face in an image. This can be useful for applications such as age-based content filtering, demographic analysis, or user interface customization. The model has been trained on a diverse dataset, so it should perform well on a variety of facial images. What can I use it for? The vit-age-classifier model could be used in a variety of applications that require age-based analysis of facial images. For example, it could be integrated into a content moderation system to filter out age-inappropriate content, or used to provide age-targeted recommendations in a media platform. It could also be used to analyze demographic trends in a dataset of facial images. To use the model, you can load it directly from the Hugging Face model hub using the provided code examples. You can then pass in new facial images and get age predictions for the people in those images. Things to try One interesting thing to try with the vit-age-classifier model would be to evaluate its performance on a diverse dataset of facial images, including people of different ages, genders, and ethnicities. This could help understand any potential biases or limitations in the model's predictions. You could also try fine-tuning the model on your own dataset of facial images to see if you can improve its accuracy for your specific use case. The provided code examples should give you a good starting point for integrating the model into your own applications.
Updated 5/27/2024
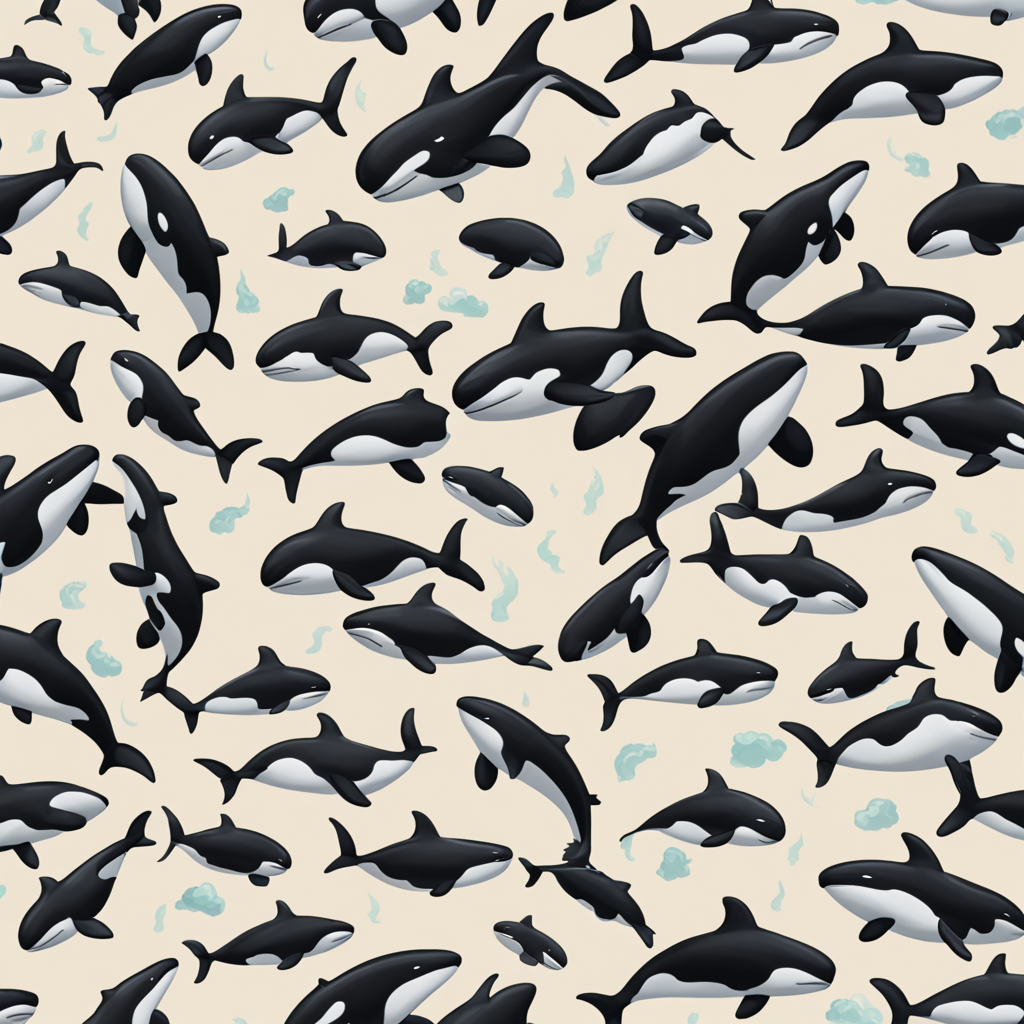
mistral-7b-openorca

65
The mistral-7b-openorca is a large language model developed by Mistral AI and fine-tuned on the OpenOrca dataset. It is a 7 billion parameter model that has been trained to engage in open-ended dialogue and assist with a variety of tasks. This model can be seen as a successor to the Mistral-7B-v0.1 and Dolphin-2.1-Mistral-7B models, which were also based on the Mistral-7B architecture but fine-tuned on different datasets. Model inputs and outputs The mistral-7b-openorca model takes a text prompt as input and generates a response as output. The input prompt can be on any topic and the model will attempt to provide a relevant and coherent response. The output is returned as a list of string tokens. Inputs Prompt**: The text prompt that the model will use to generate a response. Max new tokens**: The maximum number of tokens the model should generate as output. Temperature**: The value used to modulate the next token probabilities. Top K**: The number of highest probability tokens to consider for generating the output. Top P**: A probability threshold for generating the output, using nucleus filtering. Presence penalty**: A penalty applied to tokens based on their previous appearance in the output. Frequency penalty**: A penalty applied to tokens based on their overall frequency in the output. Prompt template**: A template used to format the input prompt, with a placeholder for the actual prompt text. Outputs Output**: A list of string tokens representing the generated response. Capabilities The mistral-7b-openorca model is capable of engaging in open-ended dialogue on a wide range of topics. It can be used for tasks such as answering questions, providing summaries, and generating creative content. The model's performance is likely comparable to similar large language models, such as the Dolphin-2.2.1-Mistral-7B and Mistral-7B-Instruct-v0.2 models, which share the same underlying architecture. What can I use it for? The mistral-7b-openorca model can be used for a variety of applications, such as: Chatbots and virtual assistants: The model's ability to engage in open-ended dialogue makes it well-suited for building conversational interfaces. Content generation: The model can be used to generate creative writing, blog posts, or other types of textual content. Question answering: The model can be used to answer questions on a wide range of topics. Summarization: The model can be used to summarize long passages of text. Things to try One interesting aspect of the mistral-7b-openorca model is its ability to provide step-by-step reasoning for its responses. By using the provided prompt template, users can instruct the model to "Write out your reasoning step-by-step to be sure you get the right answers!" This can be a useful feature for understanding the model's decision-making process and for educational or analytical purposes.
Updated 9/18/2024
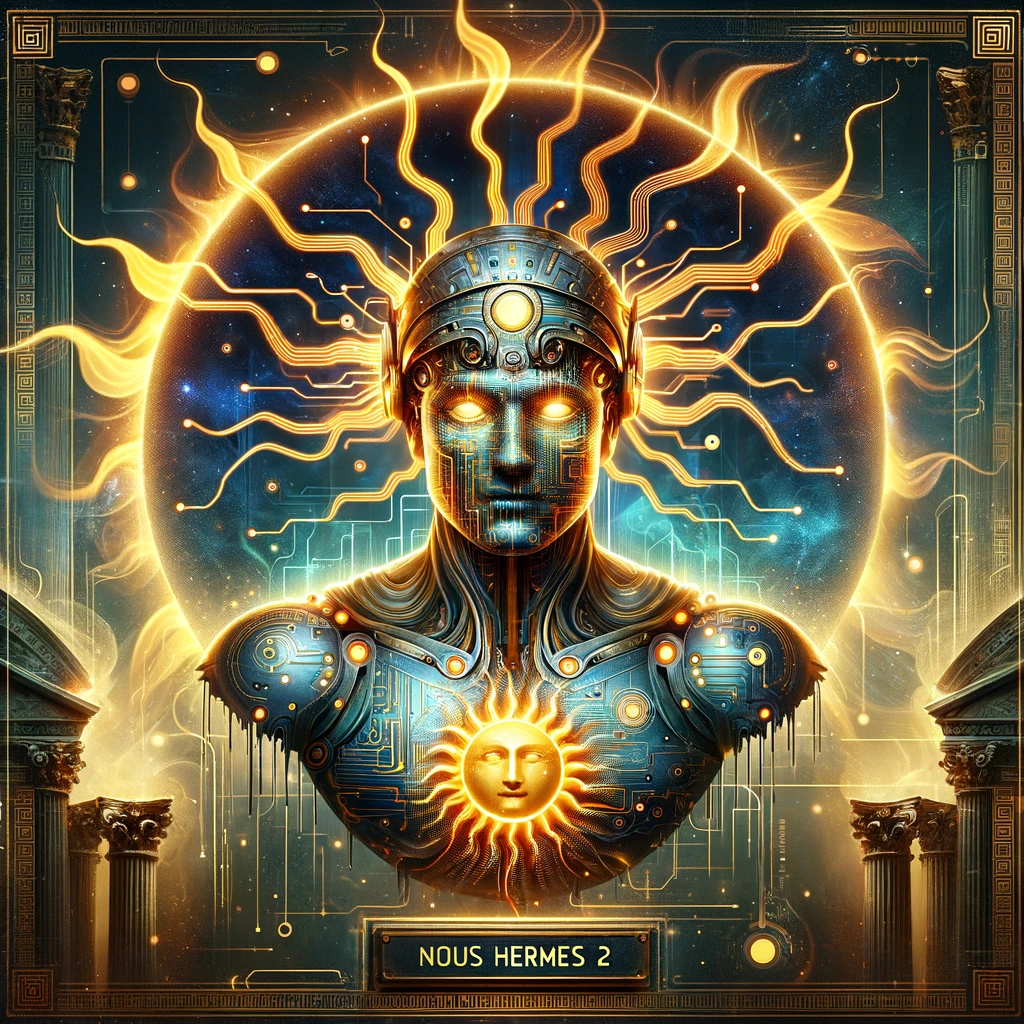
nous-hermes-2-solar-10.7b

62
nous-hermes-2-solar-10.7b is the flagship model of Nous Research, built on the SOLAR 10.7B base model. It is a powerful language model with a wide range of capabilities. While it shares some similarities with other Nous Research models like nous-hermes-2-yi-34b-gguf, nous-hermes-2-solar-10.7b has its own unique strengths and specialized training. Model inputs and outputs nous-hermes-2-solar-10.7b is a text generation model that takes a prompt as input and generates relevant and coherent text as output. The model's inputs and outputs are detailed below: Inputs Prompt**: The text that the model will use to generate a response. Top K**: The number of highest probability tokens to consider for generating the output. Top P**: A probability threshold for generating the output, used in nucleus filtering. Temperature**: A value used to modulate the next token probabilities. Max New Tokens**: The maximum number of tokens the model should generate as output. Prompt Template**: A template used to format the prompt, with a placeholder for the input prompt. Presence Penalty**: A penalty applied to the score of tokens based on their previous occurrences in the generated text. Frequency Penalty**: A penalty applied to the score of tokens based on their overall frequency in the generated text. Outputs The model generates a list of strings as output, representing the text it has generated based on the provided input. Capabilities nous-hermes-2-solar-10.7b is a highly capable language model that can be used for a variety of tasks, such as text generation, question answering, and language understanding. It has been trained on a vast amount of data and can produce human-like responses on a wide range of topics. What can I use it for? nous-hermes-2-solar-10.7b can be used for a variety of applications, including: Content generation**: The model can be used to generate original text, such as stories, articles, or poems. Chatbots and virtual assistants**: The model's natural language processing capabilities make it well-suited for building conversational AI agents. Language understanding**: The model can be used to analyze and interpret text, such as for sentiment analysis or topic classification. Question answering**: The model can be used to answer questions on a wide range of subjects, drawing from its extensive knowledge base. Things to try There are many interesting things you can try with nous-hermes-2-solar-10.7b. For example, you could experiment with different input prompts to see how the model responds, or you could try using the model in combination with other AI tools or datasets to unlock new capabilities.
Updated 9/18/2024
👁️
stable-diffusion-videos

58
stable-diffusion-videos is a model that generates videos by interpolating the latent space of Stable Diffusion, a popular text-to-image diffusion model. This model was created by nateraw, who has developed several other Stable Diffusion-based models. Unlike the stable-diffusion-animation model, which animates between two prompts, stable-diffusion-videos allows for interpolation between multiple prompts, enabling more complex video generation. Model inputs and outputs The stable-diffusion-videos model takes in a set of prompts, random seeds, and various configuration parameters to generate an interpolated video. The output is a video file that seamlessly transitions between the provided prompts. Inputs Prompts**: A set of text prompts, separated by the | character, that describe the desired content of the video. Seeds**: Random seeds, also separated by |, that control the stochastic elements of the video generation. Leaving this blank will randomize the seeds. Num Steps**: The number of interpolation steps to generate between prompts. Guidance Scale**: A parameter that controls the balance between the input prompts and the model's own creativity. Num Inference Steps**: The number of diffusion steps used to generate each individual image in the video. Fps**: The desired frames per second for the output video. Outputs Video File**: The generated video file, which can be saved to a specified output directory. Capabilities The stable-diffusion-videos model is capable of generating highly realistic and visually striking videos by smoothly transitioning between different text prompts. This can be useful for a variety of creative and commercial applications, such as generating animated artwork, product demonstrations, or even short films. What can I use it for? The stable-diffusion-videos model can be used for a wide range of creative and commercial applications, such as: Animated Art**: Generate dynamic, evolving artwork by transitioning between different visual concepts. Product Demonstrations**: Create captivating videos that showcase products or services by seamlessly blending different visuals. Short Films**: Experiment with video storytelling by generating visually impressive sequences that transition between different scenes or moods. Commercials and Advertisements**: Leverage the model's ability to generate engaging, high-quality visuals to create compelling marketing content. Things to try One interesting aspect of the stable-diffusion-videos model is its ability to incorporate audio to guide the video interpolation. By providing an audio file along with the text prompts, the model can synchronize the video transitions to the beat and rhythm of the music, creating a truly immersive and synergistic experience. Another interesting approach is to experiment with the model's various configuration parameters, such as the guidance scale and number of inference steps, to find the optimal balance between adhering to the input prompts and allowing the model to explore its own creative possibilities.
Updated 9/17/2024
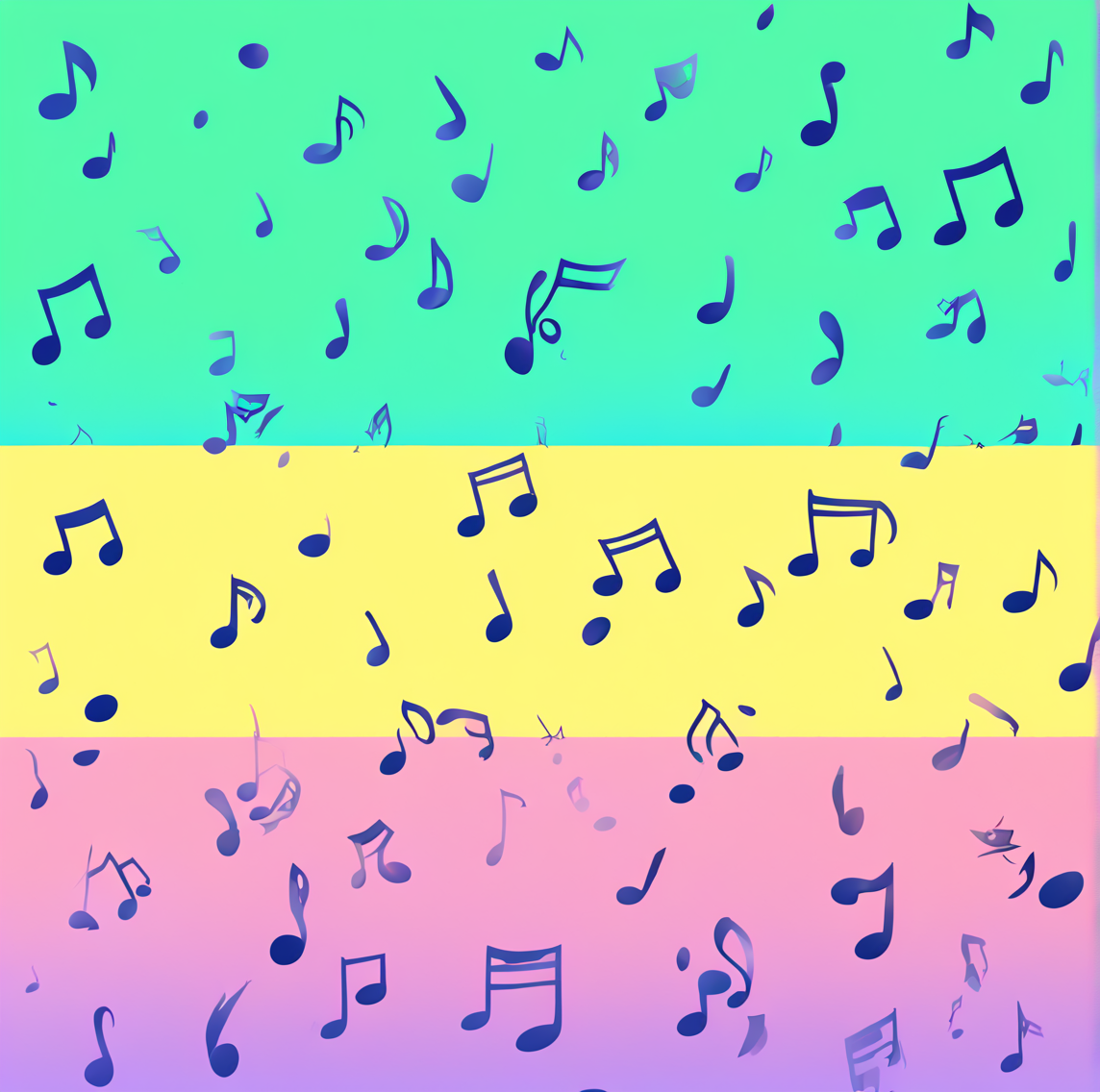
audio-super-resolution

46
audio-super-resolution is a versatile audio super-resolution model developed by Replicate creator nateraw. It is capable of upscaling various types of audio, including music, speech, and environmental sounds, to higher fidelity across different sampling rates. This model can be seen as complementary to other audio-focused models like whisper-large-v3, which focuses on speech recognition, and salmonn, which handles a broader range of audio tasks. Model inputs and outputs audio-super-resolution takes in an audio file and generates an upscaled version of the input. The model supports both single file processing and batch processing of multiple audio files. Inputs Input Audio File**: The audio file to be upscaled, which can be in various formats. Input File List**: A file containing a list of audio files to be processed in batch. Outputs Upscaled Audio File**: The super-resolved version of the input audio, saved in the specified output directory. Capabilities audio-super-resolution can handle a wide variety of audio types, from music and speech to environmental sounds, and it can work with different sampling rates. The model is capable of enhancing the fidelity and quality of the input audio, making it a useful tool for tasks such as audio restoration, content creation, and audio post-processing. What can I use it for? The audio-super-resolution model can be leveraged in various applications where high-quality audio is required, such as music production, podcast editing, sound design, and audio archiving. By upscaling lower-quality audio files, users can create more polished and professional-sounding audio content. Additionally, the model's versatility makes it suitable for use in creative projects, content creation workflows, and audio-related research and development. Things to try To get started with audio-super-resolution, you can experiment with processing both individual audio files and batches of files. Try using the model on a variety of audio types, such as music, speech, and environmental sounds, to see how it performs. Additionally, you can adjust the model's parameters, such as the DDIM steps and guidance scale, to explore the trade-offs between audio quality and processing time.
Updated 9/18/2024