Cswry
Models by this creator
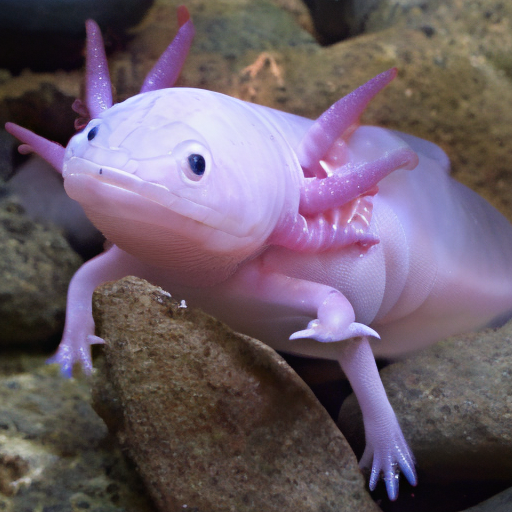
seesr

24
seesr is an AI model developed by cswry that aims to perform semantics-aware real-world image super-resolution. It builds upon the Stable Diffusion model and incorporates additional components to enhance the quality of real-world image upscaling. Unlike similar models like supir, supir-v0q, supir-v0f, real-esrgan, and gfpgan, seesr focuses on leveraging semantic information to improve the fidelity and perception of the upscaled images. Model inputs and outputs seesr takes in a low-resolution real-world image and generates a high-resolution version of the same image, aiming to preserve the semantic content and visual quality. The model can handle a variety of input images, from natural scenes to portraits and close-up shots. Inputs Image**: The input low-resolution real-world image Outputs Output image**: The high-resolution version of the input image, with improved fidelity and perception Capabilities seesr demonstrates the ability to perform semantics-aware real-world image super-resolution, preserving the semantic content and visual quality of the input images. It can handle a diverse range of real-world scenes, from buildings and landscapes to people and animals, and produces high-quality upscaled results. What can I use it for? seesr can be used for a variety of applications that require high-resolution real-world images, such as photo editing, digital art, and content creation. Its semantic awareness allows for more faithful and visually pleasing upscaling, making it a valuable tool for professionals and enthusiasts alike. Additionally, the model can be utilized in applications where high-quality image assets are needed, such as virtual reality, gaming, and architectural visualization. Things to try One interesting aspect of seesr is its ability to balance the trade-off between fidelity and perception in the upscaled images. Users can experiment with the various parameters, such as the number of inference steps and the guidance scale, to find the right balance for their specific use cases. Additionally, users can try manually specifying prompts to further enhance the quality of the results, as the automatic prompt extraction by the DAPE component may not always be perfect.
Updated 6/29/2024