Fenglinglwb
Models by this creator
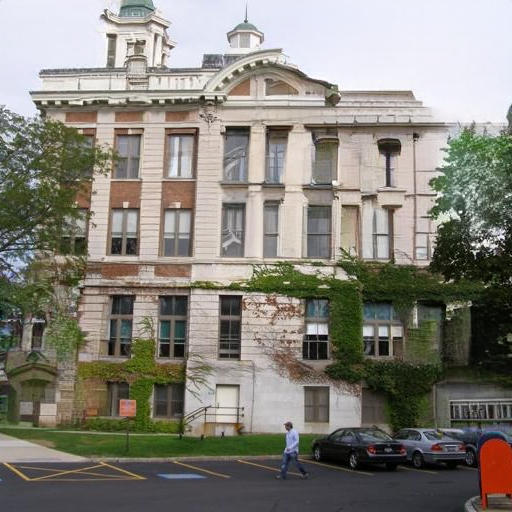
large-hole-image-inpainting

16
The large-hole-image-inpainting model, developed by fenglinglwb, is a transformer-based model called MAT (Mask-Aware Transformer) that is designed for high-fidelity and diverse large hole image inpainting. It outperforms other state-of-the-art methods like LaMa and CoModGAN on various metrics, including FID, P-IDS, and U-IDS, for both small and large mask scenarios. Model inputs and outputs The large-hole-image-inpainting model takes an input image and an optional mask as inputs. The input image must be 512x512 in size, and the mask is also expected to be 512x512, where 0 and 1 values represent the masked and remaining pixels, respectively. If no mask is provided, a random mask will be generated. The model outputs an inpainted image, where the missing regions are filled in with plausible content. Inputs Image**: The input image to be inpainted, which must be 512x512 in size. Mask**: An optional 512x512 mask, where 0 and 1 values represent the masked and remaining pixels, respectively. If not provided, a random mask will be generated. Seed**: An optional seed value for the random number generator to encourage diverse results. If set to -1, a random seed will be used. Outputs Inpainted Image**: The output image with the missing regions filled in with plausible content. Capabilities The large-hole-image-inpainting model excels at restoring high-quality and diverse images with large missing regions. Compared to other methods, it produces fewer artifacts and more photo-realistic results, as demonstrated in the provided examples. The model's transformer-based architecture allows it to effectively capture long-range dependencies and holistic image structures, leading to its strong performance on large hole inpainting tasks. What can I use it for? The large-hole-image-inpainting model can be useful in a variety of applications, such as: Restoring old or damaged photographs by filling in missing regions Removing unwanted objects or people from images Completing partial or corrupted images Enabling creative photo editing and manipulation By leveraging the model's ability to generate plausible content for large missing areas, you can explore various use cases in the fields of image editing, restoration, and creative content generation. Things to try One interesting aspect of the large-hole-image-inpainting model is its ability to generate diverse and pluralistic results. By adjusting the seed value, you can encourage the model to produce different inpainted versions of the same input, each with its own unique characteristics. This can be useful for exploring various creative possibilities or generating multiple options for a particular task. Additionally, you can experiment with different mask sizes and placements to observe how the model handles varying levels of missing information. This can provide insights into the model's strengths and limitations, and help you better understand its capabilities in different inpainting scenarios.
Updated 10/5/2024