K-amir
Models by this creator
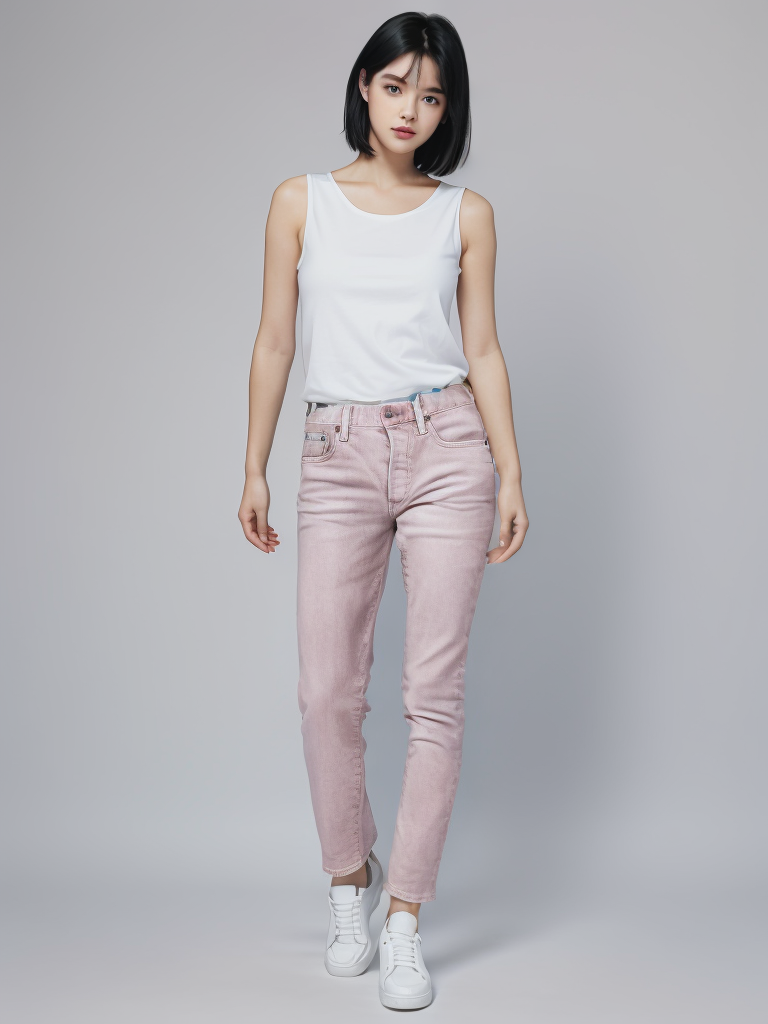
ootdifussiondc

4.9K
The ootdifussiondc model, created by maintainer k-amir, is a virtual dressing room model that allows users to try on clothing in a full-body setting. This model is similar to other virtual try-on models like oot_diffusion, which provide a dressing room experience, as well as stable-diffusion, a powerful text-to-image diffusion model. Model inputs and outputs The ootdifussiondc model takes in several key inputs, including an image of the user's model, an image of the garment to be tried on, and various parameters like the garment category, number of steps, and image scale. The model then outputs a new image showing the user wearing the garment. Inputs vton_img**: The image of the user's model garm_img**: The image of the garment to be tried on category**: The category of the garment (upperbody, lowerbody, or dress) n_steps**: The number of steps for the diffusion process n_samples**: The number of samples to generate image_scale**: The scale factor for the output image seed**: The seed for random number generation Outputs Output**: A new image showing the user wearing the selected garment Capabilities The ootdifussiondc model is capable of generating realistic-looking images of users wearing various garments, allowing for a virtual try-on experience. It can handle both half-body and full-body models, and supports different garment categories. What can I use it for? The ootdifussiondc model can be used to build virtual dressing room applications, allowing customers to try on clothes online before making a purchase. This can help reduce the number of returns and improve the overall shopping experience. Additionally, the model could be used in fashion design and styling applications, where users can experiment with different outfit combinations. Things to try Some interesting things to try with the ootdifussiondc model include experimenting with different garment categories, adjusting the number of steps and image scale, and generating multiple samples to explore variations. You could also try combining the model with other AI tools, such as GFPGAN for face restoration or k-diffusion for further image refinement.
Updated 9/18/2024