Mv-lab
Models by this creator
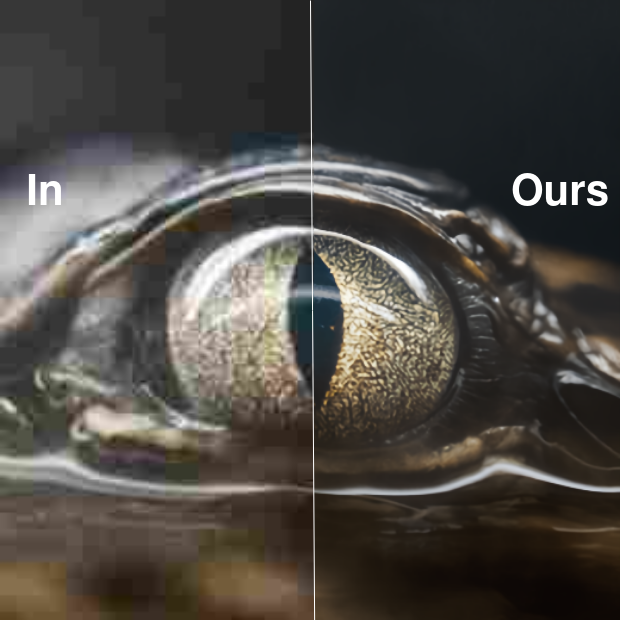
swin2sr

3.5K
swin2sr is a state-of-the-art AI model for photorealistic image super-resolution and restoration, developed by the mv-lab research team. It builds upon the success of the SwinIR model by incorporating the novel Swin Transformer V2 architecture, which improves training convergence and performance, especially for compressed image super-resolution tasks. The model outperforms other leading solutions in classical, lightweight, and real-world image super-resolution, JPEG compression artifact reduction, and compressed input super-resolution. It was a top-5 solution in the "AIM 2022 Challenge on Super-Resolution of Compressed Image and Video". Similar models in the image restoration and enhancement space include supir, stable-diffusion, instructir, gfpgan, and seesr. Model inputs and outputs swin2sr takes low-quality, low-resolution JPEG compressed images as input and generates high-quality, high-resolution images as output. The model can upscale the input by a factor of 2, 4, or other scales, depending on the task. Inputs Low-quality, low-resolution JPEG compressed images Outputs High-quality, high-resolution images with reduced compression artifacts and enhanced visual details Capabilities swin2sr can effectively tackle various image restoration and enhancement tasks, including: Classical image super-resolution Lightweight image super-resolution Real-world image super-resolution JPEG compression artifact reduction Compressed input super-resolution The model's excellent performance is achieved through the use of the Swin Transformer V2 architecture, which improves training stability and data efficiency compared to previous transformer-based approaches like SwinIR. What can I use it for? swin2sr can be particularly useful in applications where image quality and resolution are crucial, such as: Enhancing images for high-resolution displays and printing Improving image quality for streaming services and video conferencing Restoring old or damaged photos Generating high-quality images for virtual reality and gaming The model's ability to handle compressed input super-resolution makes it a valuable tool for efficient image and video transmission and storage in bandwidth-limited systems. Things to try One interesting aspect of swin2sr is its potential to be used in combination with other image processing and generation models, such as instructir or stable-diffusion. By integrating swin2sr into a workflow that starts with text-to-image generation or semantic-aware image manipulation, users can achieve even more impressive and realistic results. Additionally, the model's versatility in handling various image restoration tasks makes it a valuable tool for researchers and developers working on computational photography, low-level vision, and image signal processing applications.
Updated 6/29/2024
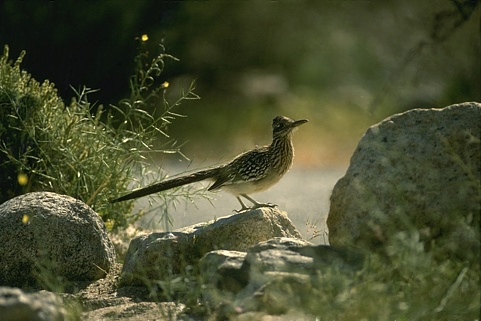
instructir

404
InstructIR is a high-quality image restoration model that can recover clean images from various types of degradation, such as noise, rain, blur, and haze. The model takes a degraded image and a human-written prompt as input, and generates a restored, high-quality output image. This approach is unique compared to similar models like gfpgan, which focuses on face restoration, or supir, which uses a large language model for image restoration. InstructIR is designed to be a versatile, all-in-one image restoration tool that can handle a wide range of degradation types. Model inputs and outputs InstructIR takes two inputs: an image and a human-written instruction prompt. The image can be any type of degraded or low-quality image, and the prompt should describe the desired restoration or enhancement. The model then generates a high-quality, restored output image that follows the instruction. Inputs Image**: The degraded or low-quality input image Prompt**: A human-written instruction describing the desired restoration or enhancement Outputs Image**: The restored, high-quality output image Capabilities InstructIR can perform a variety of image restoration tasks, including denoising, deraining, deblurring, dehazing, and low-light enhancement. The model achieves state-of-the-art results on several benchmarks, outperforming previous all-in-one restoration methods by over 1 dB. This makes InstructIR a powerful tool for a wide range of applications, from computational photography to image editing and restoration. What can I use it for? InstructIR can be used for a variety of image-related tasks, such as: Restoring old or damaged photos Enhancing low-light or hazy images Removing unwanted elements like rain, snow, or lens flare Sharpening blurry images Improving the quality of AI-generated images The model's ability to follow human instructions makes it a versatile tool for both professional and amateur users. For example, a photographer could use InstructIR to quickly remove unwanted elements from their images, while a designer could use it to enhance the visual quality of their work. Things to try One interesting aspect of InstructIR is its ability to handle different types of image degradation simultaneously. For example, you could try inputting an image with both noise and blur, and then providing a prompt like "Remove the noise and sharpen the image." The model should be able to restore the image, addressing both issues in a single step. Another thing to try is experimenting with more creative or subjective prompts. For instance, you could try prompts like "Make this image look like a professional portrait" or "Apply a cinematic style to this landscape." The model's ability to understand and respond to these types of instructions is an exciting area of research and development.
Updated 6/29/2024