Victor-upmeet
Models by this creator
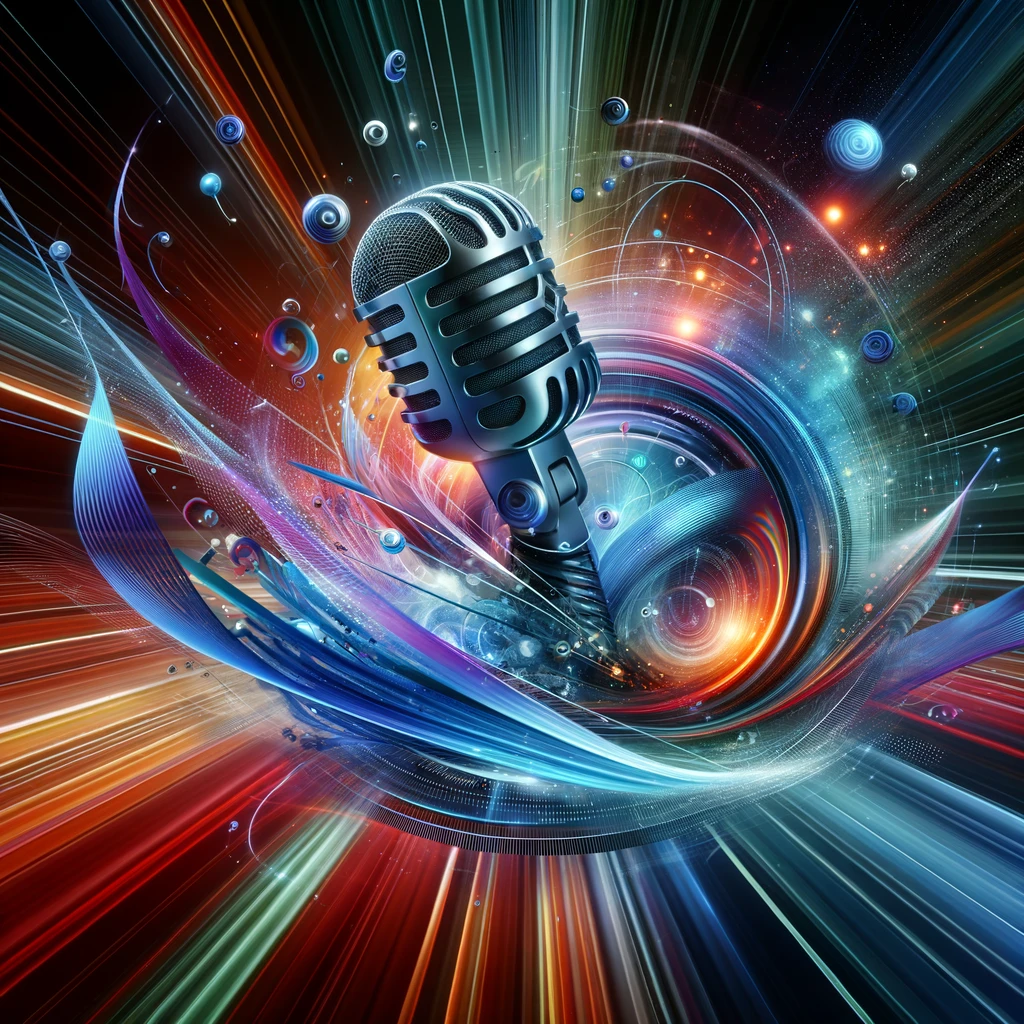
whisperx

192
whisperx is a speech transcription model developed by researchers at Upmeet. It builds upon OpenAI's Whisper model, adding features like accelerated transcription, word-level timestamps, and speaker diarization. Unlike the original Whisper, whisperx supports batching for faster processing of long-form audio. It also offers several model variants optimized for different hardware setups, including the victor-upmeet/whisperx-a40-large and victor-upmeet/whisperx-a100-80gb models. Model inputs and outputs whisperx takes an audio file as input and generates a transcript with word-level timestamps and optional speaker diarization. It can handle a variety of audio formats and supports language detection and automatic transcription of multiple languages. Inputs Audio File**: The audio file to be transcribed Language**: The ISO code of the language spoken in the audio (optional, can be automatically detected) VAD Onset/Offset**: Parameters for voice activity detection Diarization**: Whether to assign speaker ID labels Alignment**: Whether to align the transcript to get accurate word-level timestamps Speaker Limits**: Minimum and maximum number of speakers for diarization Outputs Detected Language**: The ISO code of the detected language Segments**: The transcribed text, with word-level timestamps and optional speaker IDs Capabilities whisperx provides fast and accurate speech transcription, with the ability to generate word-level timestamps and identify multiple speakers. It outperforms the original Whisper model in terms of transcription speed and timestamp accuracy, making it well-suited for use cases such as video captioning, podcast transcription, and meeting notes generation. What can I use it for? whisperx can be used in a variety of applications that require accurate speech-to-text conversion, such as: Video Captioning**: Generate captions for videos with precise timing and speaker identification. Podcast Transcription**: Automatically transcribe podcasts and audio recordings with timestamps and diarization. Meeting Notes**: Transcribe meetings and discussions, with the ability to attribute statements to individual speakers. Voice Interfaces**: Integrate whisperx into voice-based applications and services for improved accuracy and responsiveness. Things to try Consider experimenting with different model variants of whisperx to find the best fit for your hardware and use case. The victor-upmeet/whisperx model is a good starting point, but the victor-upmeet/whisperx-a40-large and victor-upmeet/whisperx-a100-80gb models may be more suitable if you encounter memory issues when dealing with long audio files or when performing alignment and diarization.
Updated 7/2/2024
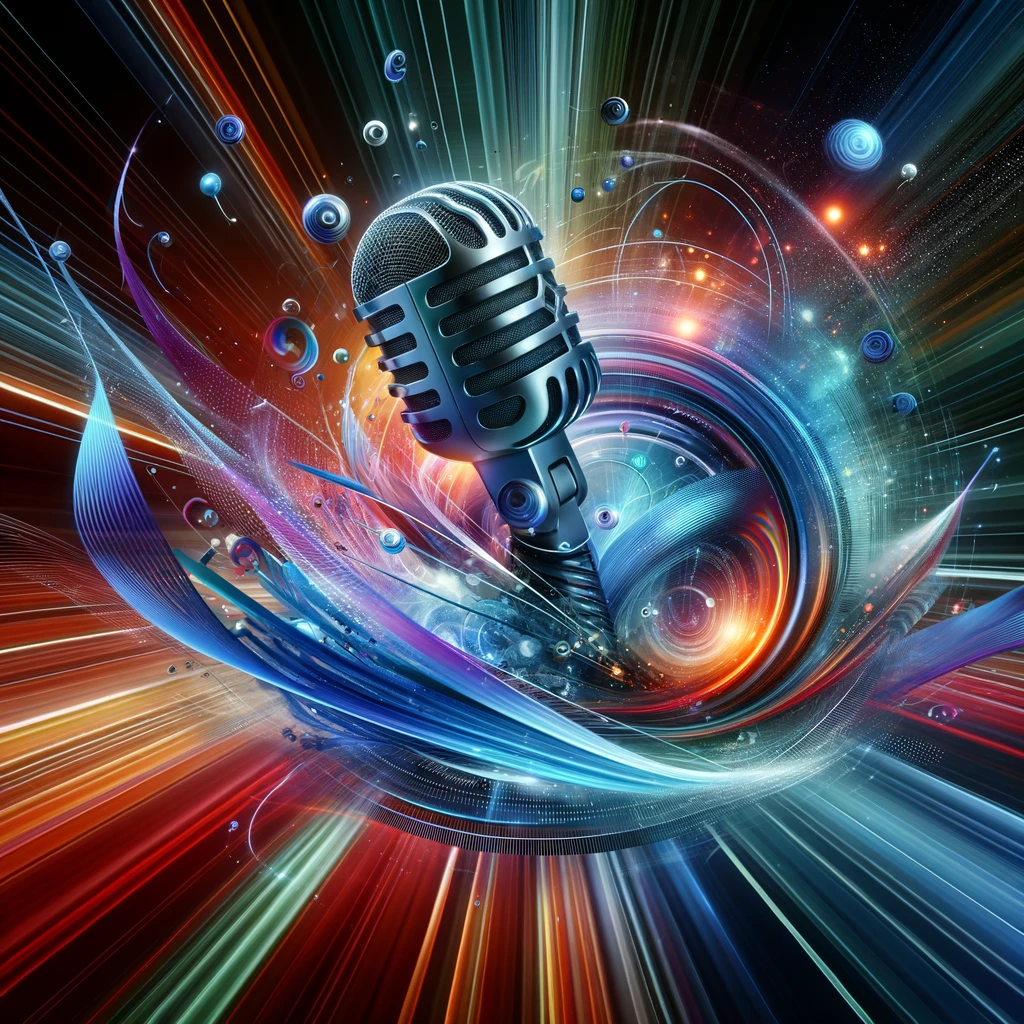
whisperx-a40-large

9
The whisperx-a40-large model is an accelerated version of the popular Whisper automatic speech recognition (ASR) model. Developed by Victor Upmeet, it provides fast transcription with word-level timestamps and speaker diarization. This model builds upon the capabilities of Whisper, which was originally created by OpenAI, and incorporates optimizations from the WhisperX project for improved performance. Similar models like whisperx, incredibly-fast-whisper, and whisperx-video-transcribe also leverage the Whisper architecture with various levels of optimization and additional features. Model inputs and outputs The whisperx-a40-large model takes an audio file as input and outputs a transcript with word-level timestamps and, optionally, speaker diarization. The model can automatically detect the language of the audio, or the language can be specified manually. Inputs Audio File**: The audio file to be transcribed. Language**: The ISO code of the language spoken in the audio. If not specified, the model will attempt to detect the language. Diarization**: A boolean flag to enable speaker diarization, which assigns speaker ID labels to the transcript. Alignment**: A boolean flag to align the Whisper output for accurate word-level timestamps. Batch Size**: The number of audio chunks to process in parallel for improved performance. Outputs Detected Language**: The language detected in the audio, if not specified manually. Segments**: The transcribed text, with word-level timestamps and speaker IDs (if diarization is enabled). Capabilities The whisperx-a40-large model excels at transcribing long-form audio with high accuracy and speed. It can handle a wide range of audio content, from interviews and lectures to podcasts and meetings. The model's ability to provide word-level timestamps and speaker diarization makes it particularly useful for applications that require detailed transcripts, such as video captioning, meeting minutes, and content indexing. What can I use it for? The whisperx-a40-large model can be used in a variety of applications that involve speech-to-text conversion, including: Automated transcription of audio and video content Real-time captioning for live events or webinars Generating meeting minutes or notes from recordings Indexing and searching audio/video archives Powering voice interfaces and chatbots As an accelerated version of the Whisper model, the whisperx-a40-large can be particularly useful for processing large audio files or handling high-volume transcription workloads. Things to try One interesting aspect of the whisperx-a40-large model is its ability to perform speaker diarization, which can be useful for analyzing multi-speaker audio recordings. Try experimenting with the diarization feature to see how it can help identify and separate different speakers in your audio content. Additionally, the model's language detection capabilities can be useful for transcribing multilingual audio or content with code-switching between languages. Test the model's performance on a variety of audio sources to see how it handles different accents, background noise, and speaking styles.
Updated 7/2/2024