Zust-ai
Models by this creator
🔍
zust-diffusion

61
zust-diffusion is an AI model developed by zust-ai that is based on the auto1111_ds8 version. It shares similarities with other text-to-image diffusion models like kandinsky-2.2, cog-a1111-ui, uform-gen, turbo-enigma, and animagine-xl-3.1 in its ability to generate images from text prompts. Model inputs and outputs zust-diffusion takes a variety of inputs related to image generation, including prompts, image URLs, and various parameters that control the output. The key inputs are: Inputs Prompt**: The text description of the image to generate Width/Height**: The dimensions of the output image Subjects**: URLs for images that will be used as subjects in the output Pipe Type**: The type of image generation pipeline to use (e.g. SAM, photoshift, zust_fashion, etc.) Upscale By**: The factor to upscale the output image by The model outputs one or more URLs pointing to the generated image(s). Capabilities zust-diffusion is capable of generating a wide variety of images based on textual prompts, including scenes with specific objects, people, and environments. It can also perform various image manipulation tasks like upscaling, enhancing, and cleaning up images. What can I use it for? zust-diffusion could be useful for creative projects, product visualization, and research applications that require generating or manipulating images from text. For example, a company could use it to create product visualizations for their e-commerce site, or a designer could use it to explore creative ideas quickly. Things to try Some interesting things to try with zust-diffusion could include experimenting with different prompts to see the variety of images it can generate, or testing its capabilities for specific tasks like generating product images or enhancing existing images. The model's ability to handle a range of image manipulation tasks could also be an interesting area to explore further.
Updated 9/18/2024
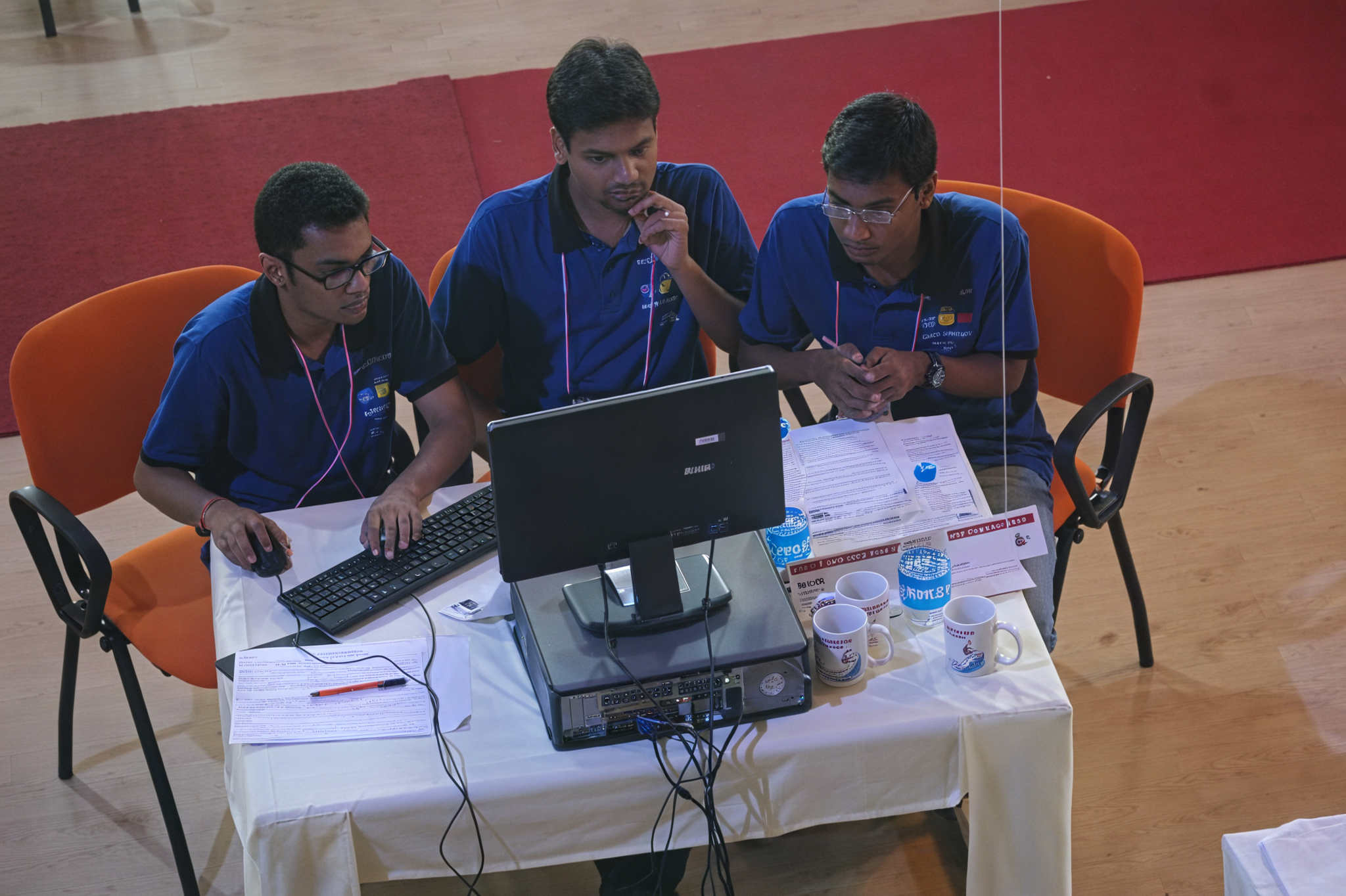
supir

32
SUPIR is a image restoration model developed by zust-ai that aims to produce high-quality, photo-realistic images from low-quality inputs. It builds on similar models like zust-diffusion, SUPIR-v0F, and SUPIR-v0Q, which also focus on image restoration and enhancement. Model inputs and outputs SUPIR takes in a low-quality input image and various parameters to control the image generation process, such as guidance scale, churn, noise, and upscaling ratio. It then outputs a high-quality, photo-realistic image. Inputs Image**: Low quality input image Seed**: Random seed (leave blank to randomize) S Cfg**: Classifier-free guidance scale for prompts S Churn**: Original churn parameter of EDM S Noise**: Original noise parameter of EDM Upscale**: Upsampling ratio of the input image A Prompt**: Additive positive prompt for the inputs Min Size**: Minimum resolution of output images N Prompt**: Negative prompt for the inputs S Stage1**: Control Strength of Stage1 (negative means invalid) S Stage2**: Control Strength of Stage2 Edm Steps**: Number of steps for EDM Sampling Schedule Use Llava**: Use LLaVA model to get captions Linear Cfg**: Linearly (with sigma) increase CFG from 'spt_linear_CFG' to s_cfg Model Name**: Choose a model (SUPIR-v0Q is the default) Color Fix Type**: Color Fixing Type Spt Linear Cfg**: Start point of linearly increasing CFG Linear S Stage2**: Linearly (with sigma) increase s_stage2 from 'spt_linear_s_stage2' to s_stage2 Spt Linear S Stage2**: Start point of linearly increasing s_stage2 Outputs Output**: A high-quality, photo-realistic image Capabilities SUPIR is capable of taking low-quality input images and restoring them to high-quality, photo-realistic outputs. It can handle a variety of image types and degradations, and the various input parameters allow for fine-tuning the generation process. What can I use it for? SUPIR can be used for a variety of image restoration and enhancement tasks, such as upscaling low-resolution images, removing noise and artifacts, and improving the overall quality of images. It could be particularly useful for professional photography, video production, and other creative industries that require high-quality visual assets. Things to try Some interesting things to try with SUPIR include experimenting with different combinations of input parameters to see how they affect the output quality, using it in conjunction with other image processing tools or workflows, and exploring its capabilities for specific use cases or creative applications.
Updated 9/18/2024