CounterfeitXL
Maintainer: gsdf

91
🏋️
Property | Value |
---|---|
Run this model | Run on HuggingFace |
API spec | View on HuggingFace |
Github link | No Github link provided |
Paper link | No paper link provided |
Create account to get full access
Model overview
CounterfeitXL
is an AI model developed by gsdf, a creator on Hugging Face. It is a text-to-text model that can generate anime-style images. Similar models include Counterfeit-V2.0, Counterfeit-V3.0, sdxl-niji-se, counterfeit-xl-v2, and animagine-xl-3.1. These models share a focus on generating anime-inspired imagery.
Model inputs and outputs
CounterfaitXL
takes text prompts as input and generates anime-style images as output. The model has been trained on a dataset of anime-style illustrations, allowing it to produce visuals with a distinct aesthetic.
Inputs
- Text prompts describing the desired image
Outputs
- Generated anime-style images
Capabilities
CounterfaitXL
can generate a variety of anime-inspired scenes and characters, ranging from solo portraits to more complex compositions with multiple figures. The model demonstrates a strong grasp of anime-style elements such as expressive facial features, dynamic poses, and distinctive clothing.
What can I use it for?
CounterfaitXL
could be useful for artists, illustrators, and content creators looking to incorporate anime-style visuals into their projects. The generated images could be used as standalone artworks, as references for traditional drawing and painting, or as assets in various digital media applications.
Things to try
Experiment with different text prompts to see the range of visual styles and compositions CounterfaitXL
can produce. Try prompts that explore specific anime genres, character archetypes, or narrative themes to see how the model interprets and renders them.
This summary was produced with help from an AI and may contain inaccuracies - check out the links to read the original source documents!
Related Models
🔍
Counterfeit-V2.5

1.5K
The Counterfeit-V2.5 model is an anime-style text-to-image AI model created by maintainer gsdf. It builds upon the Counterfeit-V2.0 model, which is an anime-style Stable Diffusion model that utilizes DreamBooth, Merge Block Weights, and Merge LoRA. The V2.5 update focuses on improving the ease of use for anime-style image generation. The model also includes a related negative prompt embedding called EasyNegative that can be used for generating higher-quality anime-style images. Model inputs and outputs Inputs Text prompts that describe the desired anime-style image Negative prompts to filter out undesirable image elements Outputs Anime-style images generated based on the provided text prompts Capabilities The Counterfeit-V2.5 model excels at generating high-quality, expressive anime-style images. It can produce a wide range of character types, settings, and scenes with a focus on aesthetics and composition. The model's capabilities are showcased in the provided examples, which include images of characters in various poses, environments, and outfits. What can I use it for? The Counterfeit-V2.5 model can be used for a variety of anime-themed creative projects, such as: Illustrations for light novels, manga, or web novels Character designs for anime-inspired video games or animation Concept art for anime-style worldbuilding or storytelling Profile pictures, avatars, or other social media content Anime-style fan art or commissions Things to try One interesting aspect of the Counterfeit-V2.5 model is its focus on ease of use for anime-style image generation. Experimenting with different prompt combinations, negative prompts, and the provided EasyNegative embedding can help you quickly generate a wide range of unique and expressive anime-inspired images.
Updated Invalid Date
🖼️
Counterfeit-V2.0

460
Counterfeit-V2.0 is an anime-style Stable Diffusion model created by gsdf. It is based on the Stable Diffusion model and incorporates techniques like DreamBooth, Merge Block Weights, and Merge LoRA to produce anime-inspired images. This model can be a useful alternative to the counterfeit-xl-v2 model, which also focuses on anime-style generation. Model inputs and outputs Inputs Text prompts that describe the desired image, including details like characters, settings, and styles Negative prompts to specify what should be avoided in the generated image Outputs Anime-style images generated based on the input prompts The model can produce images in a variety of aspect ratios and resolutions, including portrait, landscape, and square formats Capabilities The Counterfeit-V2.0 model is capable of generating high-quality anime-style images with impressive attention to detail and stylistic elements. The examples provided showcase the model's ability to create images with characters, settings, and accessories that are consistent with the anime aesthetic. What can I use it for? The Counterfeit-V2.0 model could be useful for a variety of applications, such as: Generating anime-inspired artwork or character designs for games, animation, or other media Creating concept art or illustrations for anime-themed projects Producing unique and visually striking images for social media, websites, or other digital content Things to try One interesting aspect of the Counterfeit-V2.0 model is its ability to generate images with a wide range of styles and settings, from indoor scenes to outdoor environments. Experimenting with different prompts and settings can lead to diverse and unexpected results, allowing users to explore the full potential of this anime-focused model.
Updated Invalid Date
👀
Counterfeit-V3.0

497
The Counterfeit-V3.0 model is a version of the Counterfeit anime-style Stable Diffusion model developed by the maintainer gsdf. This model builds upon the previous Counterfeit-V2.0 by incorporating BLIP-2 into the training process, which the maintainer claims may result in more effective natural language prompts. The model prioritizes expressive freedom in composition, which the maintainer notes may come at the cost of increased anatomical errors. Additionally, the maintainer has provided a new Negative Embedding that was trained alongside Counterfeit-V3.0, stating that there is no clear superiority between this and the previous embedding, so users are free to choose based on preference. Similar anime-style Stable Diffusion models include Replicant-V2.0 and OctaFuzz, which offer their own unique approaches and characteristics. Model inputs and outputs Inputs Text prompts to guide the image generation process Outputs High-quality, anime-style images based on the provided text prompts Capabilities The Counterfeit-V3.0 model excels at generating detailed, expressive anime-style images. It can produce a wide range of characters, scenes, and compositions, showcasing a high level of artistic flair. However, as noted by the maintainer, the model may occasionally exhibit anatomical errors or inconsistencies due to its prioritization of creative freedom. What can I use it for? The Counterfeit-V3.0 model can be a powerful tool for artists, illustrators, and anyone interested in creating high-quality anime-inspired artwork. Its versatility allows for the generation of character designs, background scenes, and even complex narrative compositions. Some potential use cases include: Concept art and character design for anime, manga, or video games Illustrations and fan art for online communities Visualizations and artwork for storytelling or worldbuilding projects Generating unique and personalized images for various creative projects Things to try One interesting aspect of the Counterfeit-V3.0 model is the inclusion of a new Negative Embedding, which the maintainer suggests offers different trade-offs compared to the previous embedding. Experimenting with both the standard and negative embeddings can provide insight into the model's capabilities and limitations, allowing users to find the optimal approach for their specific needs. Additionally, leveraging natural language prompts with the BLIP-2 integration may yield intriguing results, potentially leading to more cohesive and well-composed images. Exploring the nuances of prompt engineering can be a fruitful avenue for users to unlock the full potential of this anime-focused Stable Diffusion model.
Updated Invalid Date
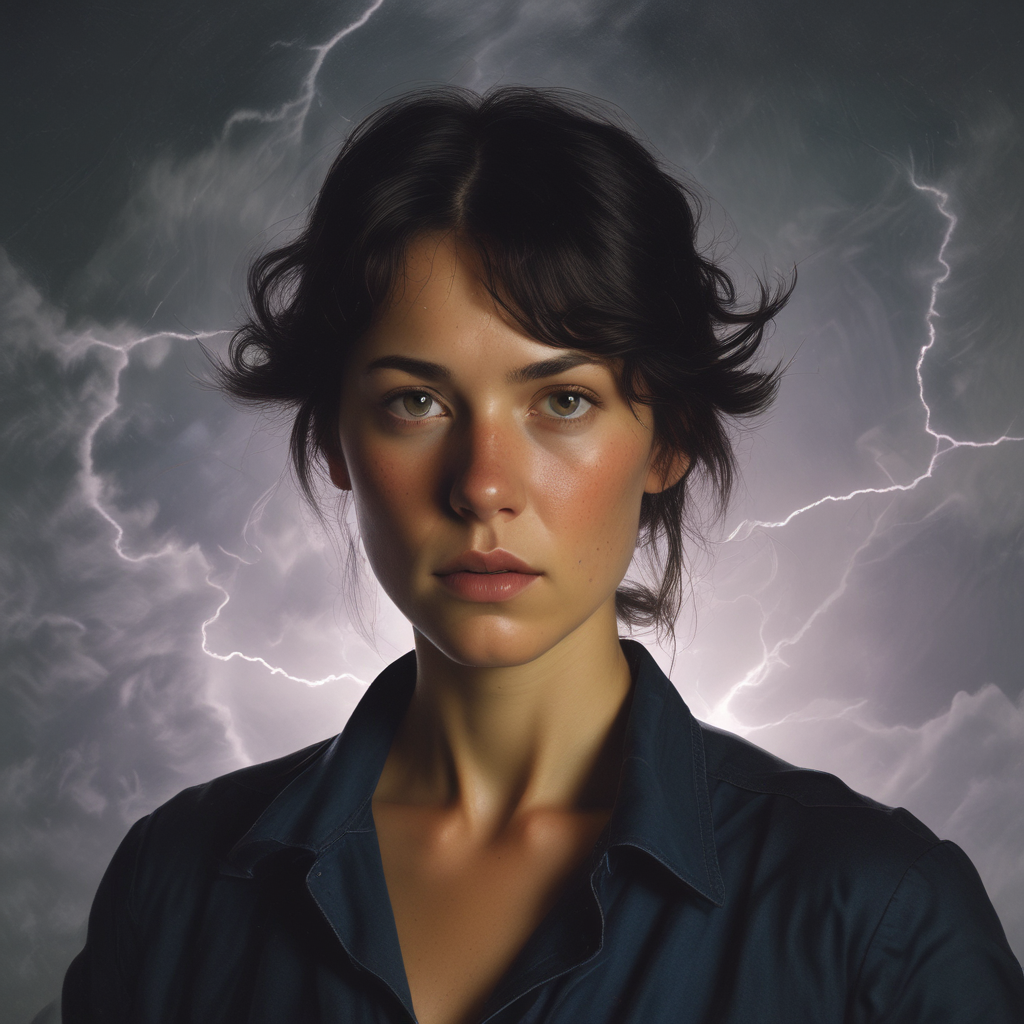
sdxl-lightning-4step

417.0K
sdxl-lightning-4step is a fast text-to-image model developed by ByteDance that can generate high-quality images in just 4 steps. It is similar to other fast diffusion models like AnimateDiff-Lightning and Instant-ID MultiControlNet, which also aim to speed up the image generation process. Unlike the original Stable Diffusion model, these fast models sacrifice some flexibility and control to achieve faster generation times. Model inputs and outputs The sdxl-lightning-4step model takes in a text prompt and various parameters to control the output image, such as the width, height, number of images, and guidance scale. The model can output up to 4 images at a time, with a recommended image size of 1024x1024 or 1280x1280 pixels. Inputs Prompt**: The text prompt describing the desired image Negative prompt**: A prompt that describes what the model should not generate Width**: The width of the output image Height**: The height of the output image Num outputs**: The number of images to generate (up to 4) Scheduler**: The algorithm used to sample the latent space Guidance scale**: The scale for classifier-free guidance, which controls the trade-off between fidelity to the prompt and sample diversity Num inference steps**: The number of denoising steps, with 4 recommended for best results Seed**: A random seed to control the output image Outputs Image(s)**: One or more images generated based on the input prompt and parameters Capabilities The sdxl-lightning-4step model is capable of generating a wide variety of images based on text prompts, from realistic scenes to imaginative and creative compositions. The model's 4-step generation process allows it to produce high-quality results quickly, making it suitable for applications that require fast image generation. What can I use it for? The sdxl-lightning-4step model could be useful for applications that need to generate images in real-time, such as video game asset generation, interactive storytelling, or augmented reality experiences. Businesses could also use the model to quickly generate product visualization, marketing imagery, or custom artwork based on client prompts. Creatives may find the model helpful for ideation, concept development, or rapid prototyping. Things to try One interesting thing to try with the sdxl-lightning-4step model is to experiment with the guidance scale parameter. By adjusting the guidance scale, you can control the balance between fidelity to the prompt and diversity of the output. Lower guidance scales may result in more unexpected and imaginative images, while higher scales will produce outputs that are closer to the specified prompt.
Updated Invalid Date