Kolors-diffusers
Maintainer: Kwai-Kolors

58
🔍
Property | Value |
---|---|
Run this model | Run on HuggingFace |
API spec | View on HuggingFace |
Github link | No Github link provided |
Paper link | No paper link provided |
Create account to get full access
Model Overview
Kolors-diffusers
is a large-scale text-to-image generation model based on latent diffusion, developed by the Kuaishou Kolors team. Trained on billions of text-image pairs, Kolors
exhibits significant advantages over both open-source and proprietary models in visual quality, complex semantic accuracy, and text rendering for both Chinese and English characters. Furthermore, Kolors
supports both Chinese and English inputs, demonstrating strong performance in understanding and generating Chinese-specific content. As described in the technical report, Kolors
is an impressive model that pushes the boundaries of photorealistic text-to-image synthesis.
The Kolors
model is similar to other latent diffusion models like Kolors, kolors, and Kolors-IP-Adapter-Plus, all of which were developed by the Kuaishou Kolors team and showcase their expertise in this domain.
Model Inputs and Outputs
Inputs
- Prompt: A text description of the desired image to generate.
- Negative Prompt: An optional text description of things to exclude from the generated image.
- Guidance Scale: A parameter that controls the influence of the text prompt on the generated image.
- Number of Inference Steps: The number of diffusion steps to perform during image generation.
- Seed: An optional random seed value to control the randomness of the generated image.
Outputs
- Image: A generated image that matches the provided text prompt.
Capabilities
Kolors-diffusers
is capable of generating highly photorealistic images from text prompts, with a strong focus on preserving semantic accuracy and text rendering quality. The model excels at synthesizing complex scenes, objects, and characters, and can handle both Chinese and English inputs with ease. This makes it a versatile tool for a wide range of applications, from creative endeavors to product visualization and beyond.
What Can I Use It For?
The Kolors-diffusers
model can be used for a variety of text-to-image generation tasks, such as:
- Creative Art and Design: Generate unique, photorealistic images to use in illustrations, concept art, and other creative projects.
- Product Visualization: Create high-quality product images and renderings to showcase new designs or ideas.
- Educational and Informational Content: Generate images to supplement textual information, such as in educational materials or data visualizations.
- Marketing and Advertising: Use the model to create visually striking images for social media, advertisements, and other marketing campaigns.
Things to Try
One interesting aspect of the Kolors-diffusers
model is its ability to handle complex Chinese-specific content. Try experimenting with prompts that incorporate Chinese terms, idioms, or cultural references to see how the model handles the generation of these unique elements. Additionally, the model's strong performance on text rendering and semantic accuracy could make it a valuable tool for applications that require precise image-text alignment, such as interactive story books or data visualization tools.
This summary was produced with help from an AI and may contain inaccuracies - check out the links to read the original source documents!
Related Models
🌐
Kolors

618
Kolors is a large-scale text-to-image generation model based on latent diffusion, developed by the Kuaishou Kolors team. Trained on billions of text-image pairs, Kolors exhibits significant advantages over both open-source and proprietary models in visual quality, complex semantic accuracy, and text rendering for both Chinese and English characters. Furthermore, Kolors supports both Chinese and English inputs, demonstrating strong performance in understanding and generating Chinese-specific content. Compared to similar models like Kandinsky-3, Kolors appears to have a stronger focus on high-quality photorealistic text-to-image synthesis, particularly for Chinese and English content. The Taiyi-Stable-Diffusion-XL-3.5B model also emphasizes bilingual capabilities, but Kolors may offer superior visual quality and accuracy for Chinese text input. Model Inputs and Outputs Kolors takes text prompts as input and generates high-resolution, photorealistic images as output. The model supports both Chinese and English prompts, allowing users to generate visuals for a wide range of topics and concepts in multiple languages. Inputs Text Prompt**: A textual description of the desired image, which can include information about the subject, style, and other attributes. Outputs Image**: A high-resolution, photorealistic image generated based on the input text prompt. Capabilities Kolors is capable of generating visually stunning and semantically accurate images from text prompts, excelling in areas such as: Photorealism**: The model can produce highly realistic images that closely match the provided textual descriptions. Complex Semantics**: Kolors demonstrates strong understanding of complex visual concepts and can generate images that capture intricate details and relationships. Bilingual Support**: The model supports both Chinese and English inputs, allowing users to generate content in their preferred language. What Can I Use It For? Kolors can be a valuable tool for a variety of applications, including: Content Creation**: Generating high-quality visuals to accompany articles, blog posts, or social media content. Illustration and Design**: Creating illustrations, concept art, and design assets for various projects. Creative Exploration**: Experimenting with different text prompts to generate unique and unexpected visual ideas. Education and Training**: Using the model's capabilities to create educational materials or train other AI systems. The maintainer's profile provides additional information about the team and their work on Kolors. Things to Try One interesting aspect of Kolors is its ability to generate visuals for complex Chinese-language concepts and cultural references. Try experimenting with prompts that incorporate Chinese idioms, historical figures, or traditional artforms to see how the model handles these unique inputs. Additionally, you could explore the limits of the model's photorealistic capabilities by providing very detailed and specific prompts, and compare the generated images to real-world reference photos. This can help you understand the model's strengths and limitations in terms of visual fidelity and semantic understanding.
Updated Invalid Date
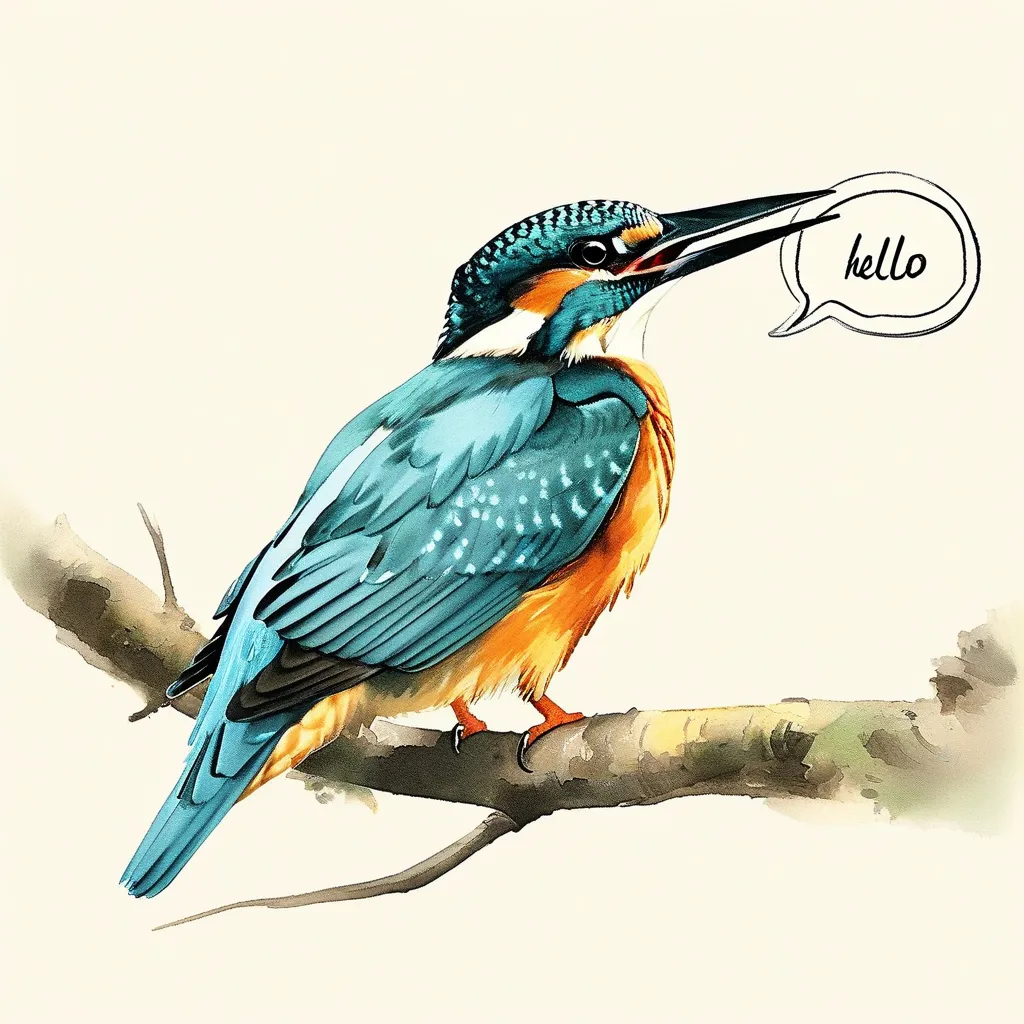
kolors

18
kolors is a large-scale text-to-image generation model based on latent diffusion, developed by the Kuaishou Kolors team. Trained on billions of text-image pairs, kolors exhibits significant advantages over both open-source and proprietary models in visual quality, complex semantic accuracy, and text rendering for both Chinese and English characters. Furthermore, kolors supports both Chinese and English inputs, demonstrating strong performance in understanding and generating Chinese-specific content. For more details, please refer to this technical report. Model inputs and outputs kolors takes a text prompt as input and generates high-quality, photorealistic images. The model supports both Chinese and English inputs, and can handle complex semantic details and text rendering. Inputs Prompt**: The text prompt that describes the desired image Width**: The width of the generated image, up to 2048 pixels Height**: The height of the generated image, up to 2048 pixels Steps**: The number of inference steps to take, up to 50 Cfg**: The guidance scale, from 0 to 20 Seed**: A seed for reproducibility (optional) Scheduler**: The diffusion scheduler to use Negative prompt**: Things you do not want to see in the image Outputs Images**: An array of generated images in the specified output format (e.g., WEBP) Capabilities kolors demonstrates strong performance in generating photorealistic images from text prompts, with advantages in visual quality, complex semantic accuracy, and text rendering compared to other models. The model's ability to understand and generate Chinese-specific content sets it apart from many open-source and proprietary alternatives. What can I use it for? kolors could be used for a variety of applications that require high-quality, photorealistic image generation from text, such as digital art creation, product design, and visual storytelling. The model's support for Chinese inputs also makes it well-suited for use cases involving Chinese-language content. Users could explore creative applications, such as illustrating stories, designing book covers, or generating concept art for games and films. Things to try One interesting aspect of kolors is its ability to generate complex, detailed images while maintaining a high level of visual quality. Users could experiment with prompts that involve intricate scenes, architectural elements, or fantastical creatures to see the model's strengths in these areas. Additionally, the model's support for both Chinese and English inputs opens up opportunities for cross-cultural applications, such as generating illustrations for bilingual children's books or visualizing traditional Chinese folklore.
Updated Invalid Date
🛸
Kolors-IP-Adapter-Plus

88
Kolors-IP-Adapter-Plus is an image-to-image model that builds upon the Kolors text-to-image generation model. The model employs a stronger image feature extractor, the Openai-CLIP-336 model, to better preserve details in reference images. It also utilizes a more diverse and high-quality training dataset to improve performance. Model inputs and outputs Kolors-IP-Adapter-Plus takes in a text prompt and a reference image, and outputs an image that combines the semantic content of the text prompt with the visual style of the reference image. Inputs Text prompt**: A natural language description of the desired image Reference image**: An image that serves as a style guide for the generated output Outputs Generated image**: An image that matches the text prompt while incorporating the visual style of the reference image Capabilities Kolors-IP-Adapter-Plus demonstrates strong performance in generating high-quality images that preserve the semantic meaning of the text prompt and faithfully represent the visual style of the reference image. It outperforms other IP-Adapter models in criteria like visual appeal, text faithfulness, and overall satisfaction according to expert evaluations. What can I use it for? The Kolors-IP-Adapter-Plus model can be useful for a variety of applications that require combining text-based descriptions with visual style references, such as: Designing product mockups or illustrations for marketing materials Creating conceptual art or visualizations based on written descriptions Generating personalized images for social media or e-commerce platforms Things to try One interesting aspect of the Kolors-IP-Adapter-Plus model is its ability to preserve details from the reference image while still faithfully representing the text prompt. You could experiment with using different types of reference images, such as abstract art or photographs, to see how the model combines them with various text prompts. Additionally, trying out prompts in different languages, such as Chinese, can help showcase the model's multilingual capabilities.
Updated Invalid Date
➖
hitokomoru-diffusion

78
hitokomoru-diffusion is a latent diffusion model that has been trained on Japanese Artist artwork, /Hitokomoru. The current model has been fine-tuned with a learning rate of 2.0e-6 for 20000 training steps/80 Epochs on 255 images collected from Danbooru. The model is trained using NovelAI Aspect Ratio Bucketing Tool so that it can be trained at non-square resolutions. Like other anime-style Stable Diffusion models, it also supports Danbooru tags to generate images. There are 4 variations of this model available, trained for different numbers of steps ranging from 5,000 to 20,000. Similar models include the hitokomoru-diffusion-v2 model, which is a continuation of this model fine-tuned from Anything V3.0, and the cool-japan-diffusion-2-1-0 model, which is a Stable Diffusion v2 model focused on Japanese art. Model inputs and outputs Inputs Text prompt**: A text description of the desired image to generate, which can include Danbooru tags like "1girl, white hair, golden eyes, beautiful eyes, detail, flower meadow, cumulonimbus clouds, lighting, detailed sky, garden". Outputs Generated image**: An image generated based on the input text prompt. Capabilities The hitokomoru-diffusion model is able to generate high-quality anime-style artwork with a focus on Japanese artistic styles. The model is particularly skilled at rendering details like hair, eyes, and natural environments. Example images showcase the model's ability to generate a variety of characters and scenes, from portraits to full-body illustrations. What can I use it for? You can use the hitokomoru-diffusion model to generate anime-inspired artwork for a variety of purposes, such as illustrations, character designs, or concept art. The model's ability to work with Danbooru tags makes it a flexible tool for creating images based on specific visual styles or themes. Some potential use cases include: Generating artwork for visual novels, manga, or anime-inspired media Creating character designs or concept art for games or other creative projects Experimenting with different artistic styles and aesthetics within the anime genre Things to try One interesting aspect of the hitokomoru-diffusion model is its support for training at non-square resolutions using the NovelAI Aspect Ratio Bucketing Tool. This allows the model to generate images with a wider range of aspect ratios, which can be useful for creating artwork intended for specific formats or platforms. Additionally, the model's ability to work with Danbooru tags provides opportunities for experimentation and fine-tuning. You could try incorporating different tags or tag combinations to see how they influence the generated output, or explore the model's capabilities for generating more complex scenes and compositions.
Updated Invalid Date