SD-Kurzgesagt-style-finetune
Maintainer: questcoast

45
🤖
Property | Value |
---|---|
Run this model | Run on HuggingFace |
API spec | View on HuggingFace |
Github link | No Github link provided |
Paper link | No paper link provided |
Create account to get full access
Model overview
The SD-Kurzgesagt-style-finetune
model is a DreamBooth fine-tune of the Stable Diffusion v1.5 model, trained on a collection of stills from the popular Kurzgesagt YouTube channel. This model can generate images with a distinct visual style reminiscent of the Kurzgesagt aesthetic, adding a unique flavor to the outputs of the Stable Diffusion system.
Similar models like MagicPrompt-Stable-Diffusion, Future-Diffusion, and Ghibli-Diffusion also fine-tune Stable Diffusion for specific visual styles, showing the versatility and customizability of this powerful text-to-image model.
Model inputs and outputs
The SD-Kurzgesagt-style-finetune
model takes text prompts as input and generates corresponding images. The text prompts can include the token _kurzgesagt style_
to invoke the specialized visual style learned during the fine-tuning process.
Inputs
- Text prompts, which can include the
_kurzgesagt style_
token to specify the desired visual style
Outputs
- Images generated based on the input text prompts, with a distinctive Kurzgesagt-inspired visual style
Capabilities
The SD-Kurzgesagt-style-finetune
model can generate a wide variety of images in the Kurzgesagt style, including illustrations, diagrams, and visualizations of scientific concepts. The model's capabilities are showcased in the provided samples, which depict informative graphics and whimsical scenes with the recognizable Kurzgesagt aesthetic.
What can I use it for?
The SD-Kurzgesagt-style-finetune
model can be particularly useful for creators and content producers looking to generate visuals with a Kurzgesagt-inspired look and feel. This could include creating assets for educational videos, informative graphics, or even concept art and illustrations for various projects. The model's ability to generate high-quality images in the Kurzgesagt style can save time and effort compared to manual illustration or other more labor-intensive methods.
Things to try
Experiment with different prompts that incorporate the _kurzgesagt style_
token to see the range of visuals the model can produce. Try combining the Kurzgesagt style with other elements, such as specific subjects, themes, or artistic styles, to create unique and compelling images. Additionally, consider exploring the capabilities of other fine-tuned Stable Diffusion models, such as Future-Diffusion and Ghibli-Diffusion, to see how they can be utilized for different creative projects.
This summary was produced with help from an AI and may contain inaccuracies - check out the links to read the original source documents!
Related Models
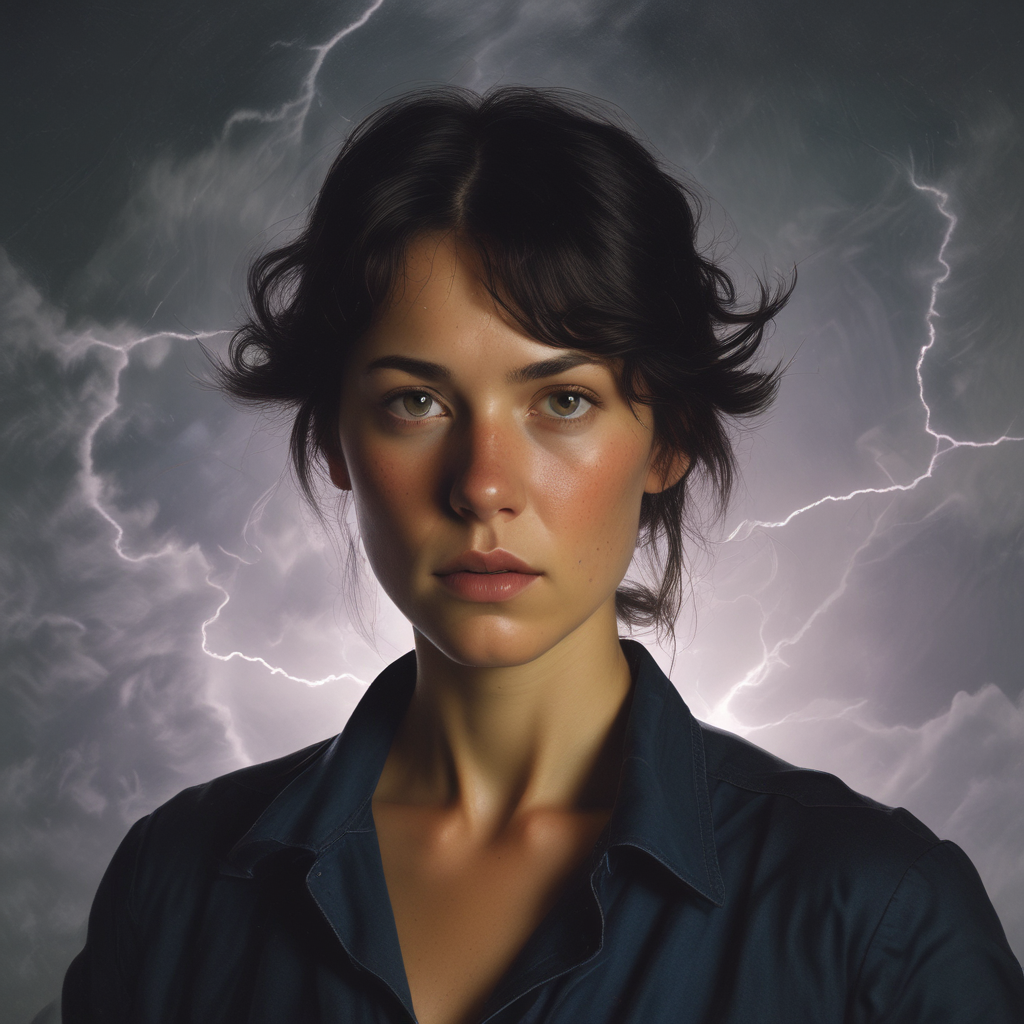
sdxl-lightning-4step

417.0K
sdxl-lightning-4step is a fast text-to-image model developed by ByteDance that can generate high-quality images in just 4 steps. It is similar to other fast diffusion models like AnimateDiff-Lightning and Instant-ID MultiControlNet, which also aim to speed up the image generation process. Unlike the original Stable Diffusion model, these fast models sacrifice some flexibility and control to achieve faster generation times. Model inputs and outputs The sdxl-lightning-4step model takes in a text prompt and various parameters to control the output image, such as the width, height, number of images, and guidance scale. The model can output up to 4 images at a time, with a recommended image size of 1024x1024 or 1280x1280 pixels. Inputs Prompt**: The text prompt describing the desired image Negative prompt**: A prompt that describes what the model should not generate Width**: The width of the output image Height**: The height of the output image Num outputs**: The number of images to generate (up to 4) Scheduler**: The algorithm used to sample the latent space Guidance scale**: The scale for classifier-free guidance, which controls the trade-off between fidelity to the prompt and sample diversity Num inference steps**: The number of denoising steps, with 4 recommended for best results Seed**: A random seed to control the output image Outputs Image(s)**: One or more images generated based on the input prompt and parameters Capabilities The sdxl-lightning-4step model is capable of generating a wide variety of images based on text prompts, from realistic scenes to imaginative and creative compositions. The model's 4-step generation process allows it to produce high-quality results quickly, making it suitable for applications that require fast image generation. What can I use it for? The sdxl-lightning-4step model could be useful for applications that need to generate images in real-time, such as video game asset generation, interactive storytelling, or augmented reality experiences. Businesses could also use the model to quickly generate product visualization, marketing imagery, or custom artwork based on client prompts. Creatives may find the model helpful for ideation, concept development, or rapid prototyping. Things to try One interesting thing to try with the sdxl-lightning-4step model is to experiment with the guidance scale parameter. By adjusting the guidance scale, you can control the balance between fidelity to the prompt and diversity of the output. Lower guidance scales may result in more unexpected and imaginative images, while higher scales will produce outputs that are closer to the specified prompt.
Updated Invalid Date
🤷
MagicPrompt-Stable-Diffusion

658
The MagicPrompt-Stable-Diffusion model is a GPT-2 model trained to generate prompt texts for the Stable Diffusion text-to-image generation model. The model was trained on a dataset of 80,000 prompts extracted from the Lexica.art image search engine, which was filtered for relevant and engaging prompts. This allows the MagicPrompt-Stable-Diffusion model to generate high-quality prompts that can be used to produce impressive images with Stable Diffusion. Model inputs and outputs The MagicPrompt-Stable-Diffusion model takes no direct inputs. Instead, it generates novel text prompts that can be used as inputs to the Stable Diffusion text-to-image model. The outputs of the MagicPrompt-Stable-Diffusion model are the generated text prompts, which can then be used to produce images with Stable Diffusion. Inputs No direct inputs to the MagicPrompt-Stable-Diffusion model Outputs Text prompts for use with the Stable Diffusion text-to-image model Capabilities The MagicPrompt-Stable-Diffusion model can generate a wide variety of engaging and creative text prompts for Stable Diffusion. Examples include prompts for fantastical scenes, photorealistic portraits, and surreal artworks. By using the MagicPrompt-Stable-Diffusion model, users can more easily access the full potential of the Stable Diffusion text-to-image generation capabilities. What can I use it for? The MagicPrompt-Stable-Diffusion model can be used to enhance the capabilities of the Stable Diffusion text-to-image model. Users can leverage the generated prompts to produce a wide variety of high-quality images for use in creative projects, artistic endeavors, and more. The model can also be used as a research tool to better understand the interplay between text prompts and image generation. Things to try One interesting thing to try with the MagicPrompt-Stable-Diffusion model is to use it to generate prompts that explore the limits of the Stable Diffusion model. For example, you could try generating prompts that push the boundaries of realism, complexity, or abstraction, and then see how the Stable Diffusion model responds. This can help uncover the strengths and weaknesses of both text-to-image models, and lead to new insights and discoveries.
Updated Invalid Date
⛏️
Future-Diffusion

402
Future-Diffusion is a fine-tuned version of the Stable Diffusion 2.0 base model, trained by nitrosocke on high-quality 3D images with a futuristic sci-fi theme. This model allows users to generate images with a distinct "future style" by incorporating the future style token into their prompts. Compared to similar models like redshift-diffusion-768, Future-Diffusion has a 512x512 resolution, while the redshift model has a higher 768x768 resolution. The Ghibli-Diffusion and Arcane-Diffusion models, on the other hand, are fine-tuned on anime and Arcane-themed images respectively, producing outputs with those distinct visual styles. Model inputs and outputs Future-Diffusion is a text-to-image model, taking text prompts as input and generating corresponding images as output. The model was trained using the diffusers-based dreambooth training approach with prior-preservation loss and the train-text-encoder flag. Inputs Text prompts**: Users provide text descriptions to guide the image generation, such as future style [subject] Negative Prompt: duplicate heads bad anatomy for character generation or future style city market street level at night Negative Prompt: blurry fog soft for landscapes. Outputs Images**: The model generates 512x512 or 1024x576 pixel images based on the provided text prompts, with a futuristic sci-fi style. Capabilities Future-Diffusion can generate a wide range of images with a distinct futuristic aesthetic, including human characters, animals, vehicles, and landscapes. The model's ability to capture this specific style sets it apart from more generic text-to-image models. What can I use it for? The Future-Diffusion model can be useful for various creative and commercial applications, such as: Generating concept art for science fiction stories, games, or films Designing futuristic product visuals or packaging Creating promotional materials or marketing assets with a futuristic flair Exploring and experimenting with novel visual styles and aesthetics Things to try One interesting aspect of Future-Diffusion is the ability to combine the "future style" token with other style tokens, such as those from the Ghibli-Diffusion or Arcane-Diffusion models. This can result in unique and unexpected hybrid styles, allowing users to expand their creative possibilities.
Updated Invalid Date
📶
herge-style

70
The herge-style model is a Stable Diffusion model fine-tuned on the Herge style concept using Dreambooth. This allows the model to generate images in the distinctive visual style of the Herge's Tintin comic books. The model was created by maderix and is part of the sd-dreambooth-library collection. Other related models include the Disco Diffusion style and Midjourney style models, which have been fine-tuned on those respective art styles. The Ghibli Diffusion model is another related example, trained on Studio Ghibli anime art. Model inputs and outputs Inputs instance_prompt**: A prompt specifying "a photo of sks herge_style" to generate images in the Herge style. Outputs High-quality, photorealistic images in the distinctive visual style of Herge's Tintin comic books. Capabilities The herge-style model can generate a wide variety of images in the Herge visual style, from portraits and characters to environments and scenes. The model is able to capture the clean lines, exaggerated features, and vibrant colors that define the Tintin art style. What can I use it for? The herge-style model could be used to create comic book-inspired illustrations, character designs, and concept art. It would be particularly well-suited for projects related to Tintin or similar European comic book aesthetics. The model could also be fine-tuned further on additional Herge-style artwork to expand its capabilities. Things to try One interesting aspect of the herge-style model is its ability to blend the Herge visual style with other elements. For example, you could try generating images that combine the Tintin art style with science fiction, fantasy, or other genres to create unique and unexpected results. Experimenting with different prompts and prompt engineering techniques could unlock a wide range of creative possibilities.
Updated Invalid Date