text-extract-ocr
Maintainer: abiruyt

17.3K
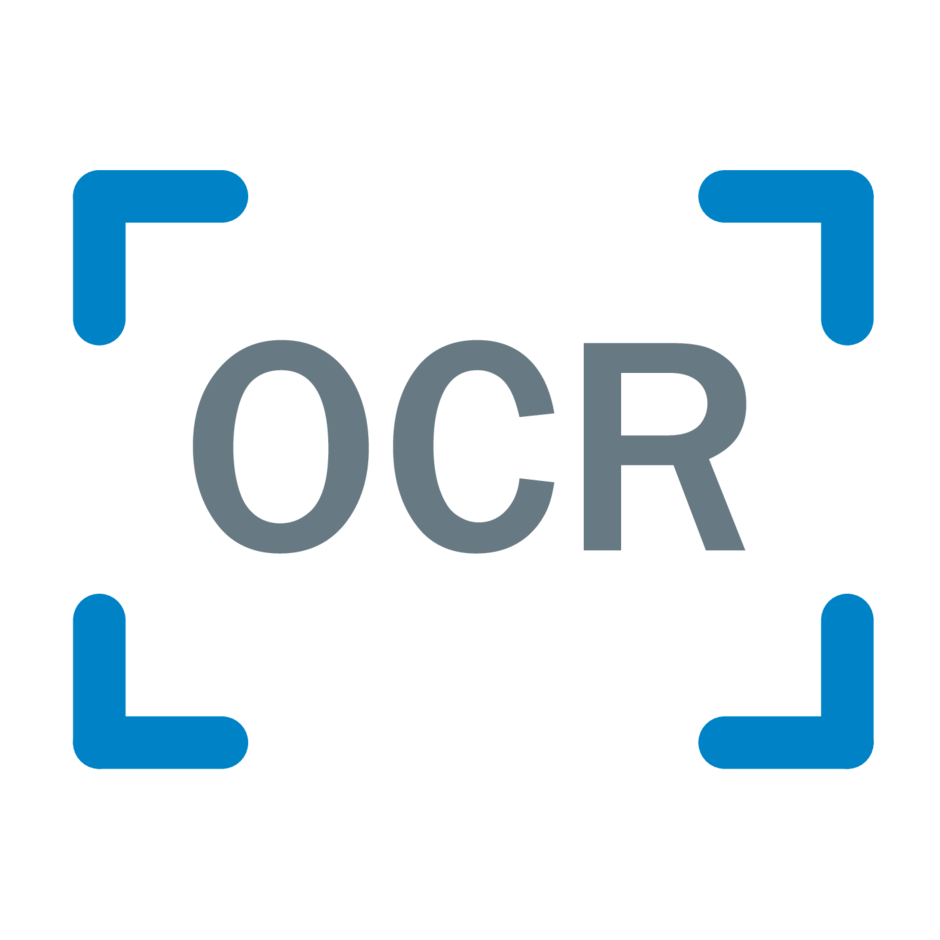
Property | Value |
---|---|
Model Link | View on Replicate |
API Spec | View on Replicate |
Github Link | No Github link provided |
Paper Link | No paper link provided |
Create account to get full access
Model overview
text-extract-ocr
is a simple OCR (Optical Character Recognition) model created by abiruyt that can easily extract text from an image. It is similar to other OCR models like ocr-surya and can be useful for a variety of text extraction tasks. Unlike more complex multimodal models like bunny-phi-2-siglip, this model focuses solely on the task of extracting text from images.
Model inputs and outputs
text-extract-ocr
takes an image as input and outputs the extracted text. The input schema specifies that the model expects a single image
parameter in the form of a URI (Uniform Resource Identifier).
Inputs
- image: The image to process and extract text from.
Outputs
- Output: The extracted text from the input image.
Capabilities
text-extract-ocr
is capable of accurately extracting text from a wide variety of image types, including scanned documents, screenshots, and photographs. It can handle multiple languages and different font styles and sizes.
What can I use it for?
You can use text-extract-ocr
for tasks like digitizing physical documents, automating data entry from forms, or extracting relevant information from images. It could be particularly useful for businesses or organizations that need to process large volumes of documents or images containing text. The model could also be integrated into broader computer vision pipelines or combined with other models like stylemc for more advanced image processing workflows.
Things to try
Some ideas for trying out text-extract-ocr
include:
- Extracting text from screenshots of web pages or mobile apps
- Digitizing physical documents like invoices, contracts, or reports
- Automating the process of extracting key information from forms or surveys
- Integrating the model into a workflow for processing large batches of images or documents
This summary was produced with help from an AI and may contain inaccuracies - check out the links to read the original source documents!
Related Models
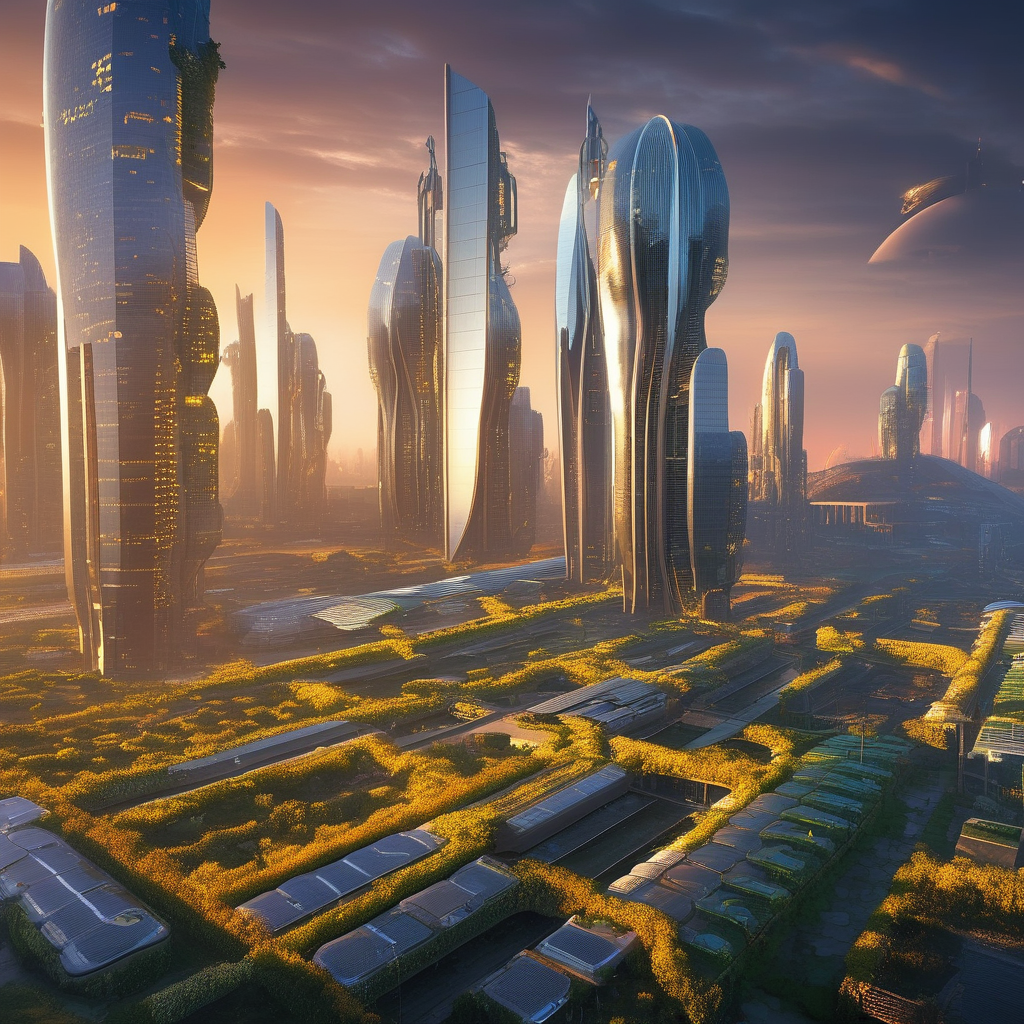
ar

1
The ar model, created by qr2ai, is a text-to-image prompt model that can generate images based on user input. It shares capabilities with similar models like outline, gfpgan, edge-of-realism-v2.0, blip-2, and rpg-v4, all of which can generate, manipulate, or analyze images based on textual input. Model inputs and outputs The ar model takes in a variety of inputs to generate an image, including a prompt, negative prompt, seed, and various settings for text and image styling. The outputs are image files in a URI format. Inputs Prompt**: The text that describes the desired image Negative Prompt**: The text that describes what should not be included in the image Seed**: A random number that initializes the image generation D Text**: Text for the first design T Text**: Text for the second design D Image**: An image for the first design T Image**: An image for the second design F Style 1**: The font style for the first text F Style 2**: The font style for the second text Blend Mode**: The blending mode for overlaying text Image Size**: The size of the generated image Final Color**: The color of the final text Design Color**: The color of the design Condition Scale**: The scale for the image generation conditioning Name Position 1**: The position of the first text Name Position 2**: The position of the second text Padding Option 1**: The padding percentage for the first text Padding Option 2**: The padding percentage for the second text Num Inference Steps**: The number of denoising steps in the image generation process Outputs Output**: An image file in URI format Capabilities The ar model can generate unique, AI-created images based on text prompts. It can combine text and visual elements in creative ways, and the various input settings allow for a high degree of customization and control over the final output. What can I use it for? The ar model could be used for a variety of creative projects, such as generating custom artwork, social media graphics, or even product designs. Its ability to blend text and images makes it a versatile tool for designers, marketers, and artists looking to create distinctive visual content. Things to try One interesting thing to try with the ar model is experimenting with different combinations of text and visual elements. For example, you could try using abstract or surreal prompts to see how the model interprets them, or play around with the various styling options to achieve unique and unexpected results.
Updated Invalid Date
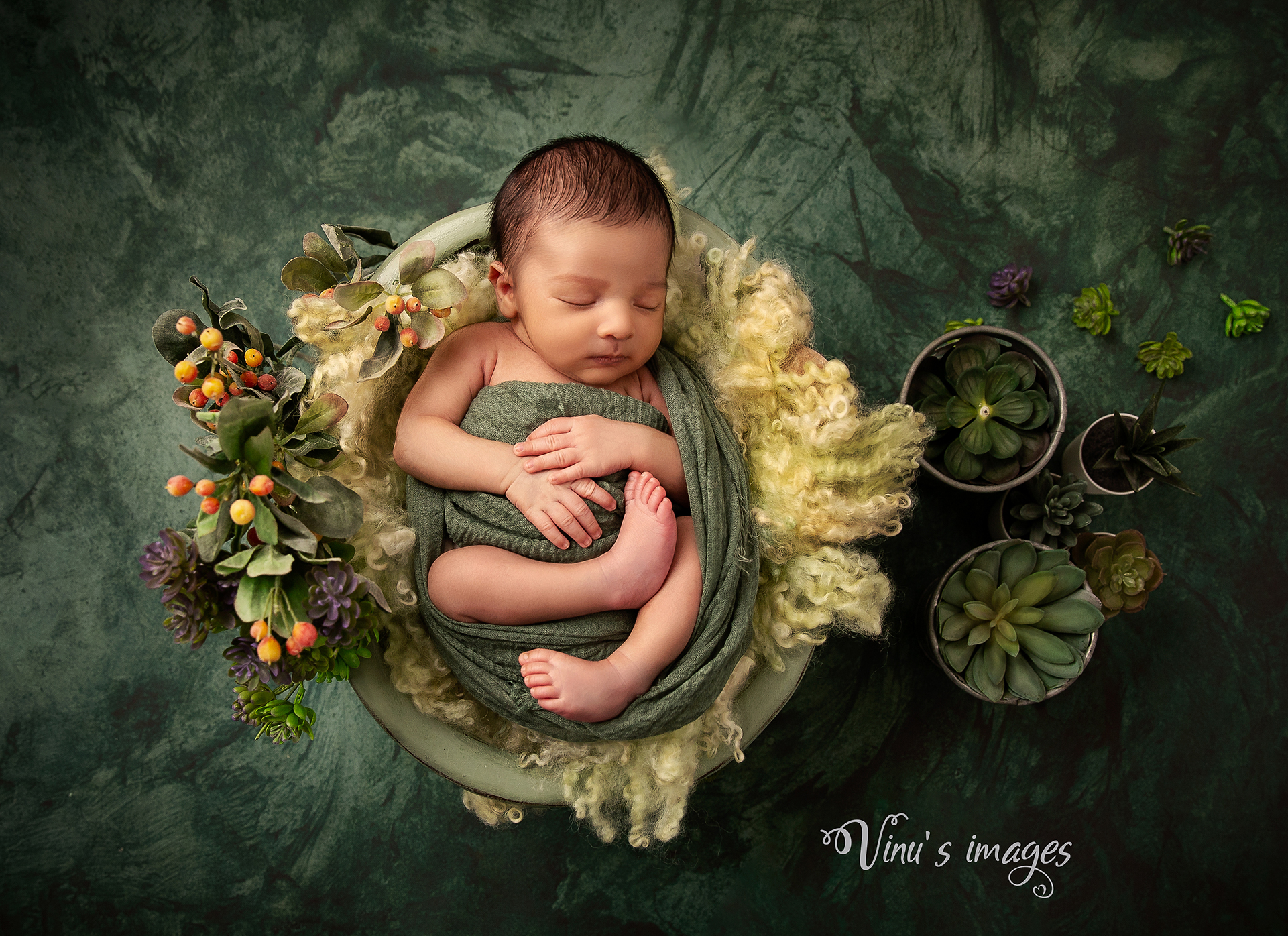
zero-shot-image-to-text

6
The zero-shot-image-to-text model is a cutting-edge AI model designed for the task of generating text descriptions from input images. Developed by researcher yoadtew, this model leverages a unique "zero-shot" approach to enable image-to-text generation without the need for task-specific fine-tuning. This sets it apart from similar models like stable-diffusion, uform-gen, and turbo-enigma which often require extensive fine-tuning for specific image-to-text tasks. Model inputs and outputs The zero-shot-image-to-text model takes in an image and produces a text description of that image. The model can handle a wide range of image types and subjects, from natural scenes to abstract concepts. Additionally, the model supports "visual-semantic arithmetic" - the ability to perform arithmetic operations on visual concepts to generate new images. Inputs Image**: The input image to be described Outputs Text Description**: A textual description of the input image Capabilities The zero-shot-image-to-text model has demonstrated impressive capabilities in generating detailed and coherent image descriptions across a diverse set of visual inputs. It can handle not only common objects and scenes, but also more complex visual reasoning tasks like understanding visual relationships and analogies. What can I use it for? The zero-shot-image-to-text model can be a valuable tool for a variety of applications, such as: Automated Image Captioning**: Generating descriptive captions for large image datasets, which can be useful for tasks like visual search, content moderation, and accessibility. Visual Question Answering**: Answering questions about the contents of an image, which can be helpful for building intelligent assistants or educational applications. Visual-Semantic Arithmetic**: Exploring and manipulating visual concepts in novel ways, which can inspire new creative applications or research directions. Things to try One interesting aspect of the zero-shot-image-to-text model is its ability to handle "visual-semantic arithmetic" - the ability to combine visual concepts in arithmetic-like operations to generate new, semantically meaningful images. For example, the model can take in images of a "woman", a "king", and a "man", and then generate a new image that represents the visual concept of "woman - king + man". This opens up fascinating possibilities for exploring the relationships between visual and semantic representations.
Updated Invalid Date
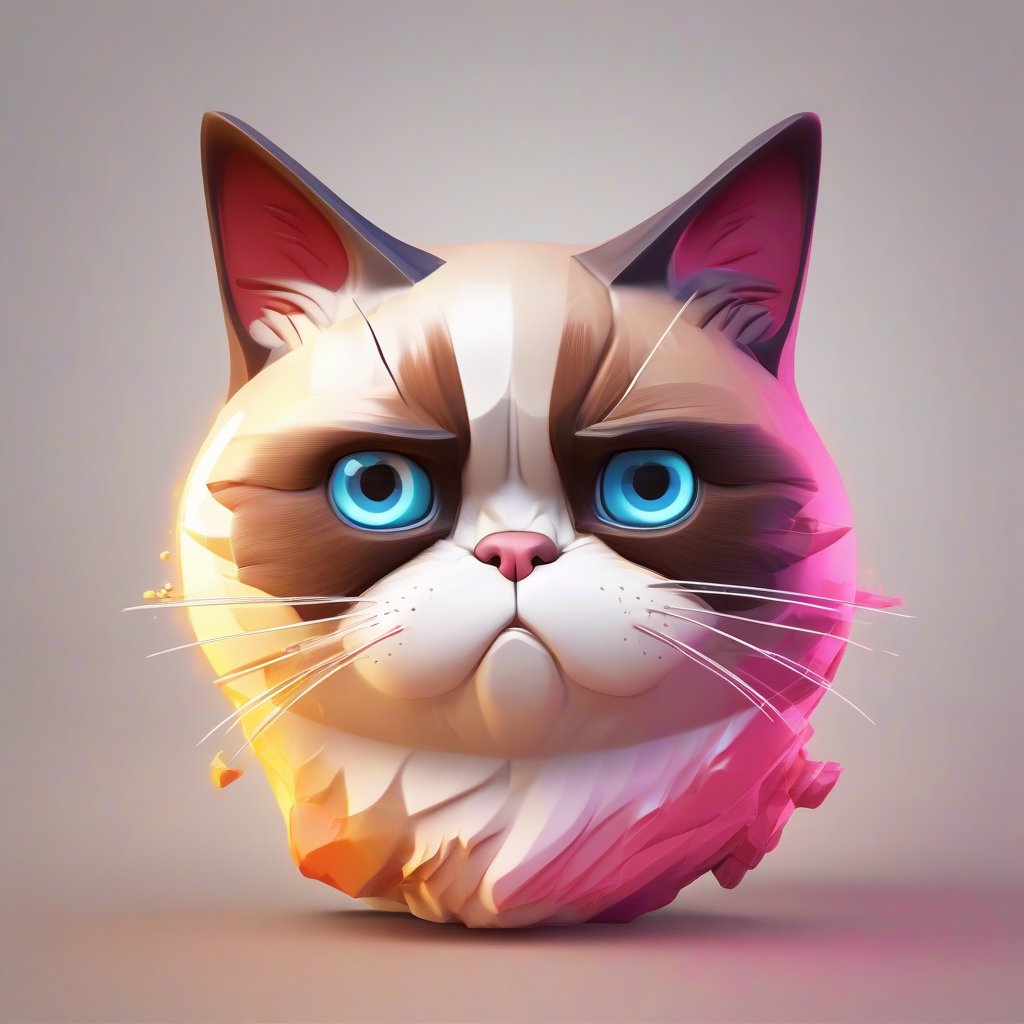
detect-ai-content

5
The detect-ai-content model is a content AI detector developed by hieunc229. This model is designed to analyze text content and detect whether it was generated by an AI system. It can be a useful tool for identifying potential AI-generated content across a variety of applications. The model shares some similarities with other large language models in the Yi series and multilingual-e5-large, as they all aim to process and analyze text data. Model inputs and outputs The detect-ai-content model takes a single input - the text content to be analyzed. The output is an array that represents the model's assessment of whether the input text was generated by an AI system. Inputs Content**: The text content to be analyzed for AI generation Outputs An array representing the model's prediction on whether the input text was AI-generated Capabilities The detect-ai-content model can be used to identify potential AI-generated content, which can be valuable for content moderation, plagiarism detection, and other applications where it's important to distinguish human-written and AI-generated text. By analyzing the characteristics and patterns of the input text, the model can provide insights into the likelihood of the content being AI-generated. What can I use it for? The detect-ai-content model can be integrated into a variety of applications and workflows to help identify AI-generated content. For example, it could be used by content creators, publishers, or social media platforms to flag potentially AI-generated content for further review or moderation. It could also be used in academic or research settings to help detect plagiarism or ensure the integrity of written work. Things to try One interesting aspect of the detect-ai-content model is its potential to evolve and improve over time as more AI-generated content is developed and analyzed. By continuously training and refining the model, it may become increasingly accurate at distinguishing human-written and AI-generated text. Users of the model could experiment with different types of content, including creative writing, technical documents, and social media posts, to better understand the model's capabilities and limitations.
Updated Invalid Date
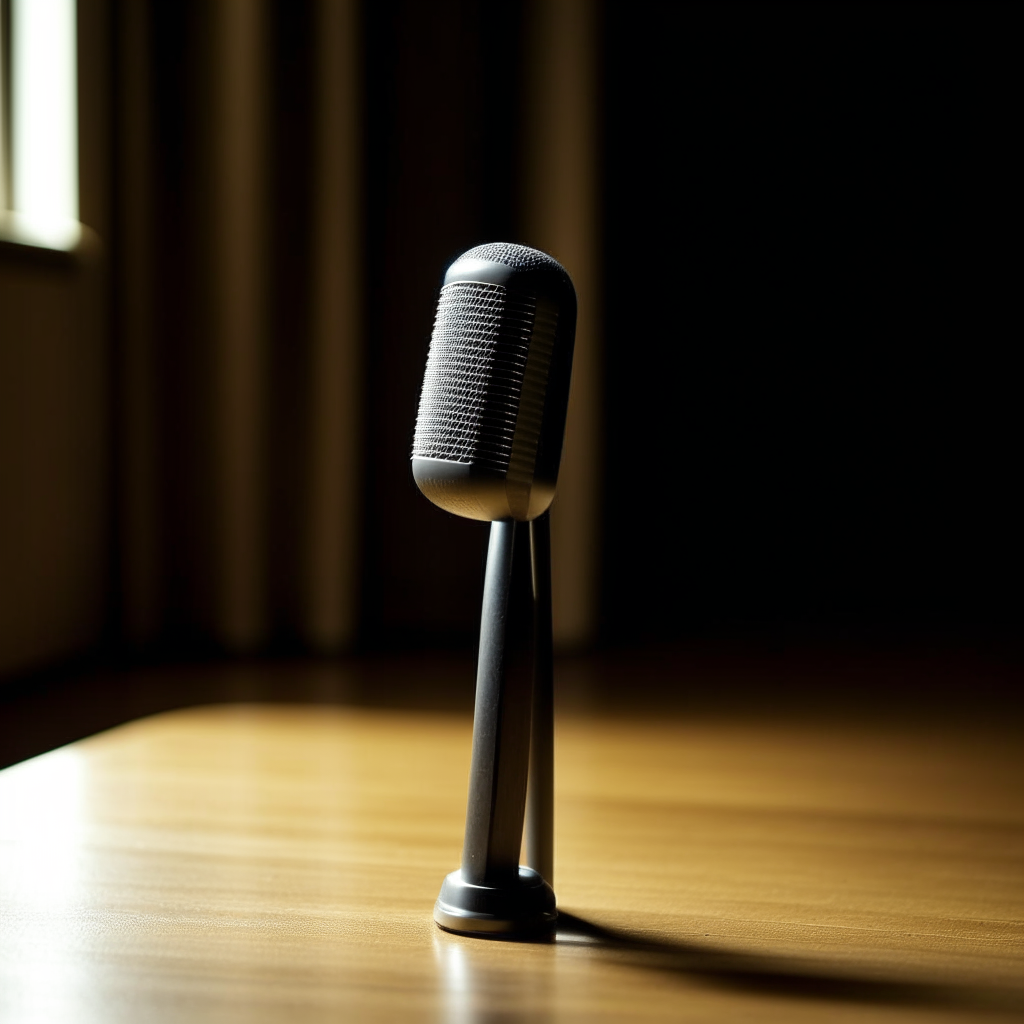
styletts2

4.2K
styletts2 is a text-to-speech (TTS) model developed by Yinghao Aaron Li, Cong Han, Vinay S. Raghavan, Gavin Mischler, and Nima Mesgarani. It leverages style diffusion and adversarial training with large speech language models (SLMs) to achieve human-level TTS synthesis. Unlike its predecessor, styletts2 models styles as a latent random variable through diffusion models, allowing it to generate the most suitable style for the text without requiring reference speech. It also employs large pre-trained SLMs, such as WavLM, as discriminators with a novel differentiable duration modeling for end-to-end training, resulting in improved speech naturalness. Model inputs and outputs styletts2 takes in text and generates high-quality speech audio. The model inputs and outputs are as follows: Inputs Text**: The text to be converted to speech. Beta**: A parameter that determines the prosody of the generated speech, with lower values sampling style based on previous or reference speech and higher values sampling more from the text. Alpha**: A parameter that determines the timbre of the generated speech, with lower values sampling style based on previous or reference speech and higher values sampling more from the text. Reference**: An optional reference speech audio to copy the style from. Diffusion Steps**: The number of diffusion steps to use in the generation process, with higher values resulting in better quality but longer generation time. Embedding Scale**: A scaling factor for the text embedding, which can be used to produce more pronounced emotion in the generated speech. Outputs Audio**: The generated speech audio in the form of a URI. Capabilities styletts2 is capable of generating human-level TTS synthesis on both single-speaker and multi-speaker datasets. It surpasses human recordings on the LJSpeech dataset and matches human performance on the VCTK dataset. When trained on the LibriTTS dataset, styletts2 also outperforms previous publicly available models for zero-shot speaker adaptation. What can I use it for? styletts2 can be used for a variety of applications that require high-quality text-to-speech generation, such as audiobook production, voice assistants, language learning tools, and more. The ability to control the prosody and timbre of the generated speech, as well as the option to use reference audio, makes styletts2 a versatile tool for creating personalized and expressive speech output. Things to try One interesting aspect of styletts2 is its ability to perform zero-shot speaker adaptation on the LibriTTS dataset. This means that the model can generate speech in the style of speakers it has not been explicitly trained on, by leveraging the diverse speech synthesis offered by the diffusion model. Developers could explore the limits of this zero-shot adaptation and experiment with fine-tuning the model on new speakers to further improve the quality and diversity of the generated speech.
Updated Invalid Date