bshm-portrait
Maintainer: twn39

1
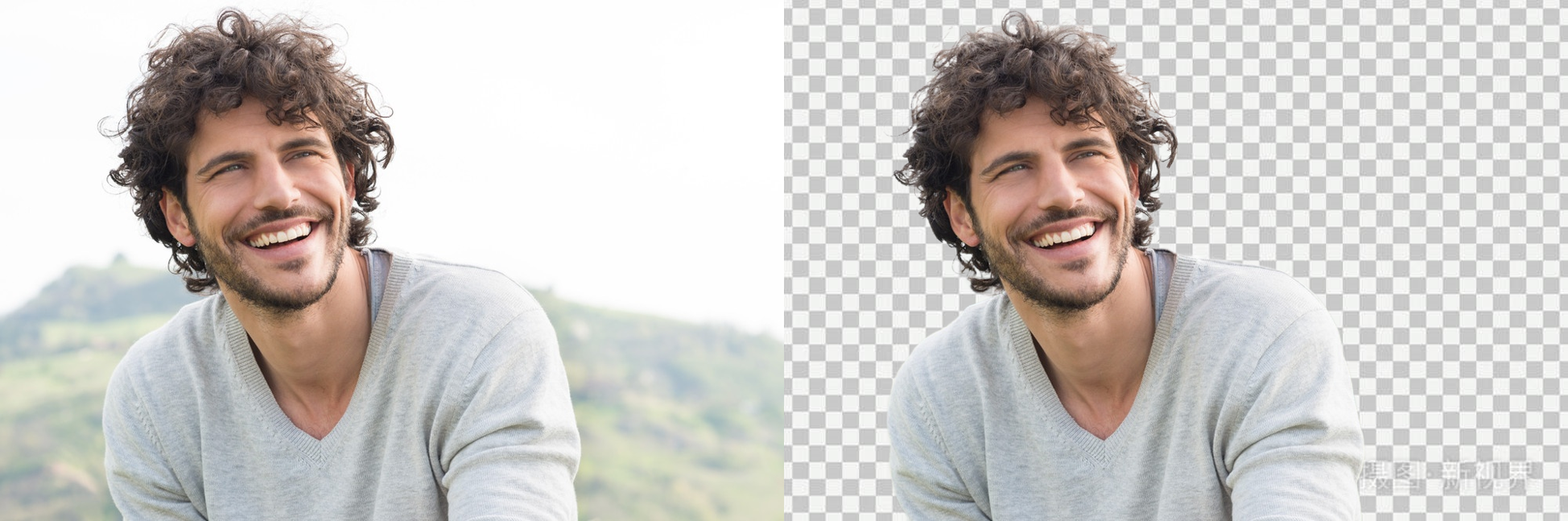
Property | Value |
---|---|
Run this model | Run on Replicate |
API spec | View on Replicate |
Github link | No Github link provided |
Paper link | No paper link provided |
Create account to get full access
Model overview
The bshm-portrait
model is a portrait segmentation AI model developed by twn39. This model can be used to extract the subject from a portrait image, separating the person from the background. It can be a useful tool for tasks like image editing, compositing, or creating high-quality portrait photos.
The bshm-portrait
model is similar to other portrait and image segmentation models like gfpgan, real-esrgan, lama, supir, and rembg-enhance. These models all aim to improve or manipulate portrait and image content in various ways.
Model inputs and outputs
The bshm-portrait
model takes a single input - an image. The output is also an image, which is the original input image with the background removed, leaving only the portrait subject.
Inputs
- Image: The input image, which should be a portrait or headshot of a person.
Outputs
- Segmented Image: The original input image with the background removed, leaving only the portrait subject.
Capabilities
The bshm-portrait
model can effectively extract the subject from a portrait image, separating the person from the background. This can be useful for a variety of image editing and compositing tasks, such as creating high-quality portrait photos, removing unwanted backgrounds, or placing subjects into new scenes.
What can I use it for?
The bshm-portrait
model can be a valuable tool for photographers, designers, and content creators who work with portrait images. Some potential use cases include:
- Removing distracting backgrounds from portrait photos to create a more focused and professional-looking image
- Extracting portrait subjects to composite them into new backgrounds or scenes
- Preparing portrait images for further editing, such as retouching or applying special effects
- Creating high-quality portrait assets for use in graphic design, web design, or multimedia projects
Things to try
With the bshm-portrait
model, you can experiment with various portrait images to see how well the model can extract the subject. Try using the model on a range of portrait styles and subjects, from formal headshots to more casual, candid photos. You can also try combining the bshm-portrait
model with other image editing tools and techniques to further refine and enhance your portrait photos.
This summary was produced with help from an AI and may contain inaccuracies - check out the links to read the original source documents!
Related Models
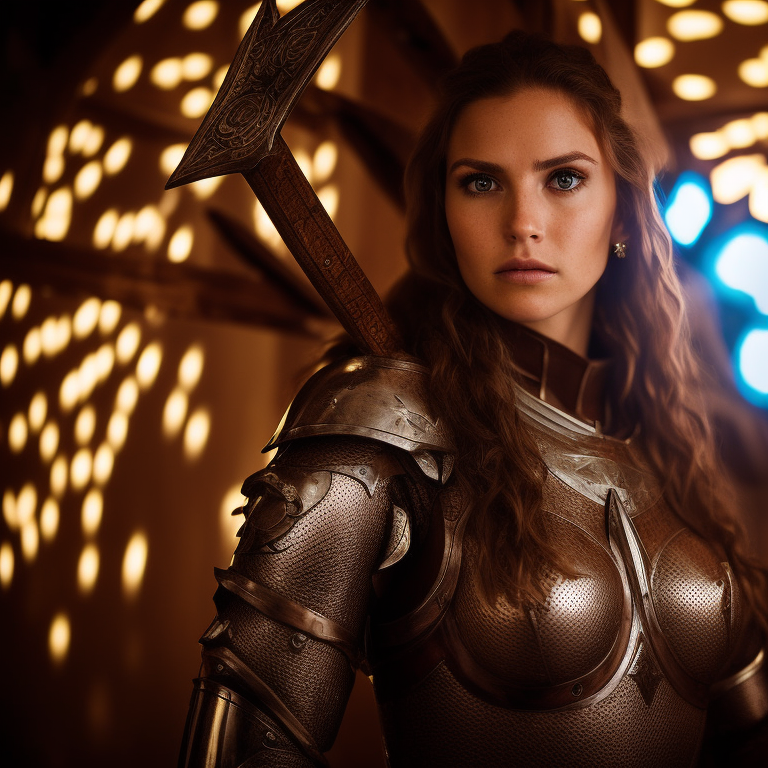
portraitplus

23
portraitplus is a model developed by Replicate user cjwbw that focuses on generating high-quality portraits in the "portrait+" style. It is similar to other Stable Diffusion models created by cjwbw, such as stable-diffusion-v2-inpainting, stable-diffusion-2-1-unclip, analog-diffusion, and anything-v4.0. These models aim to produce highly detailed and realistic images, often with a particular artistic style. Model inputs and outputs portraitplus takes a text prompt as input and generates one or more images as output. The input prompt can describe the desired portrait, including details about the subject, style, and other characteristics. The model then uses this prompt to create a corresponding image. Inputs Prompt**: The text prompt describing the desired portrait Seed**: A random seed value to control the initial noise used for image generation Width and Height**: The desired dimensions of the output image Scheduler**: The algorithm used to control the diffusion process Guidance Scale**: The amount of guidance the model should use to adhere to the provided prompt Negative Prompt**: Text describing what the model should avoid including in the generated image Outputs Image(s)**: One or more images generated based on the input prompt Capabilities portraitplus can generate highly detailed and realistic portraits in a variety of styles, from photorealistic to more stylized or artistic renderings. The model is particularly adept at capturing the nuances of facial features, expressions, and lighting to create compelling and lifelike portraits. What can I use it for? portraitplus could be used for a variety of applications, such as digital art, illustration, concept design, and even personalized portrait commissions. The model's ability to generate unique and expressive portraits can make it a valuable tool for creative professionals or hobbyists looking to explore new artistic avenues. Things to try One interesting aspect of portraitplus is its ability to generate portraits with a diverse range of subjects and styles. You could experiment with prompts that describe historical figures, fictional characters, or even abstract concepts to see how the model interprets and visualizes them. Additionally, you could try adjusting the input parameters, such as the guidance scale or number of inference steps, to find the optimal settings for your desired output.
Updated Invalid Date
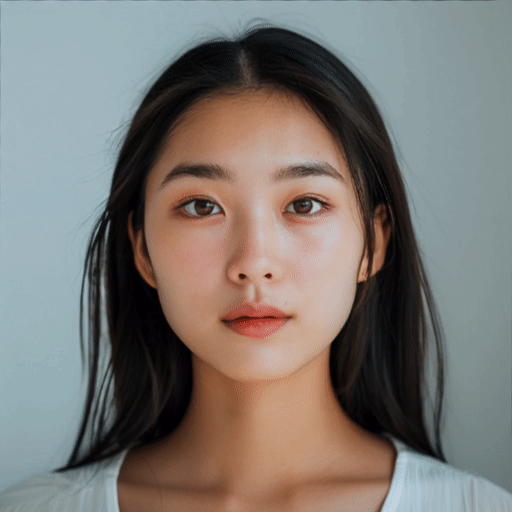
live-portrait

6
The live-portrait model is a unique AI tool that can create dynamic, audio-driven portrait animations. It combines an input image and video to produce a captivating animated portrait that reacts to the accompanying audio. This model builds upon similar portrait animation models like live-portrait-fofr, livespeechportraits-yuanxunlu, and aniportrait-audio2vid-cjwbw, each with its own distinct capabilities. Model inputs and outputs The live-portrait model takes two inputs: an image and a video. The image serves as the base for the animated portrait, while the video provides the audio that drives the facial movements and expressions. The output is an array of image URIs representing the animated portrait sequence. Inputs Image**: An input image that forms the base of the animated portrait Video**: An input video that provides the audio to drive the facial animations Outputs An array of image URIs representing the animated portrait sequence Capabilities The live-portrait model can create compelling, real-time animations that seamlessly blend a static portrait with dynamic facial expressions and movements. This can be particularly useful for creating lively, engaging content for video, presentations, or other multimedia applications. What can I use it for? The live-portrait model could be used to bring portraits to life, adding a new level of dynamism and engagement to a variety of projects. For example, you could use it to create animated avatars for virtual events, generate personalized video messages, or add animated elements to presentations and videos. The model's ability to sync facial movements to audio also makes it a valuable tool for creating more expressive and lifelike digital characters. Things to try One interesting aspect of the live-portrait model is its potential to capture the nuances of human expression and movement. By experimenting with different input images and audio sources, you can explore how the model responds to various emotional tones, speech patterns, and physical gestures. This could lead to the creation of unique and captivating animated portraits that convey a wide range of human experiences.
Updated Invalid Date
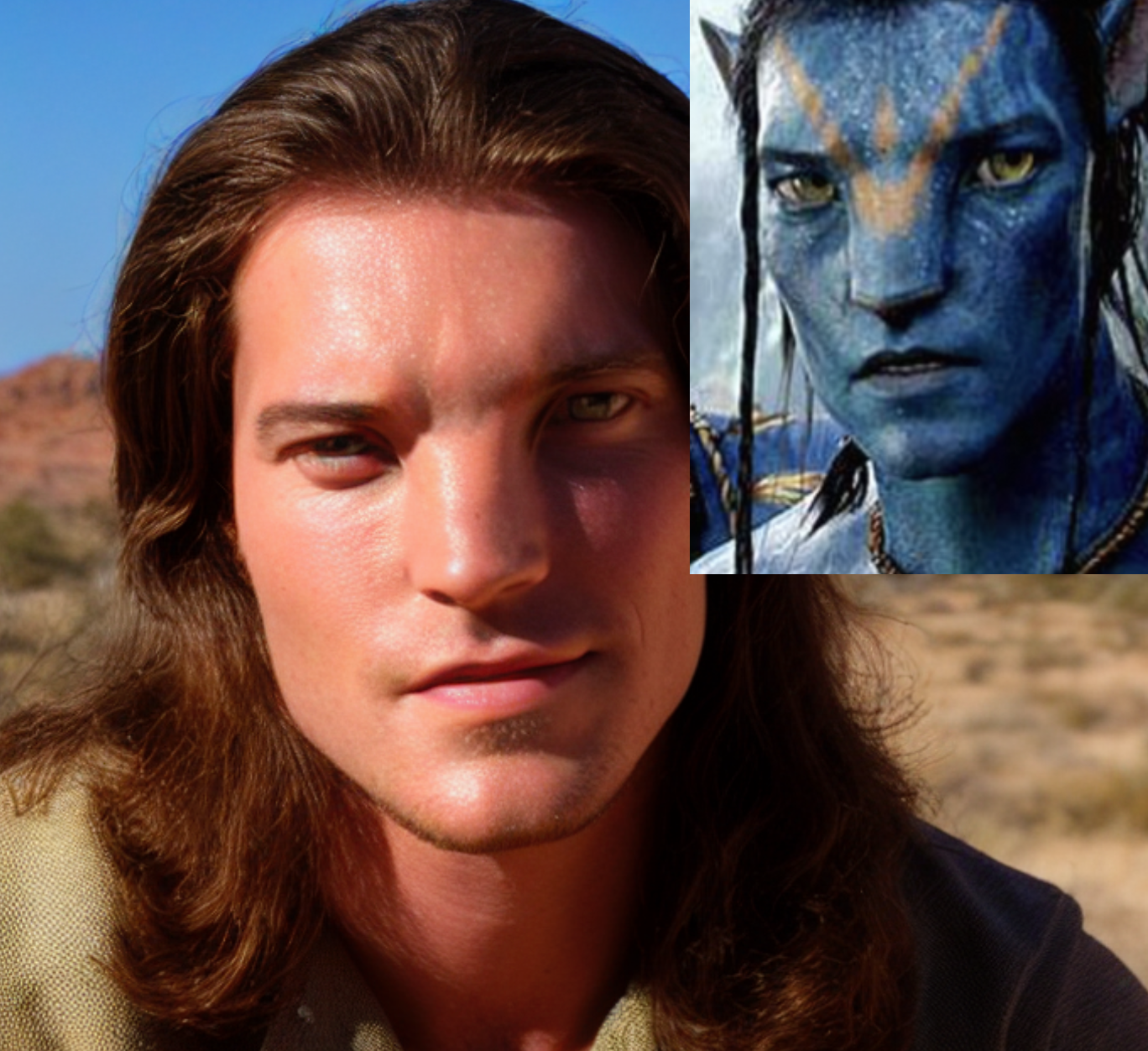
flash-face

3
flash-face is a powerful AI model developed by zsxkib that can generate highly realistic and personalized human images. It is similar to other models like GFPGAN, Instant-ID, and Stable Diffusion, which are also focused on creating photorealistic images of people. Model Inputs and Outputs The flash-face model takes in a variety of inputs, including positive and negative prompts, reference face images, and various parameters to control the output. The outputs are high-quality images of realistic-looking people, which can be generated in different formats and quality levels. Inputs Positive Prompt**: The text description of the desired image. Negative Prompt**: Text to exclude from the generated image. Reference Face Images**: Up to 4 face images to use as references for the generated image. Face Bounding Box**: The coordinates of the face region in the generated image. Text Control Scale**: The strength of the text guidance during image generation. Face Guidance**: The strength of the reference face guidance during image generation. Lamda Feature**: The strength of the reference feature guidance during image generation. Steps**: The number of steps to run the image generation process. Num Sample**: The number of images to generate. Seed**: The random seed to use for image generation. Output Format**: The format of the generated images (e.g., WEBP). Output Quality**: The quality level of the generated images (from 1 to 100). Outputs Generated Images**: An array of high-quality, realistic-looking images of people. Capabilities The flash-face model excels at generating personalized human images with high-fidelity identity preservation. It can create images that closely resemble real people, while still maintaining a sense of artistic creativity and uniqueness. The model's ability to blend reference face images with text-based prompts makes it a powerful tool for a wide range of applications, from art and design to entertainment and marketing. What Can I Use It For? The flash-face model can be used for a variety of applications, including: Creative Art and Design**: Generate unique, personalized portraits and character designs for use in illustration, animation, and other creative projects. Entertainment and Media**: Create realistic-looking avatars or virtual characters for use in video games, movies, and other media. Marketing and Advertising**: Generate personalized, high-quality images for use in marketing campaigns, product packaging, and other promotional materials. Education and Research**: Use the model to create diverse, representative datasets for training and testing computer vision and image processing algorithms. Things to Try One interesting aspect of the flash-face model is its ability to blend multiple reference face images together to create a unique, composite image. You could try experimenting with different combinations of reference faces and prompts to see how the model responds and what kind of unique results it can produce. Additionally, you could explore the model's ability to generate images with specific emotional expressions or poses by carefully crafting your prompts and reference images.
Updated Invalid Date
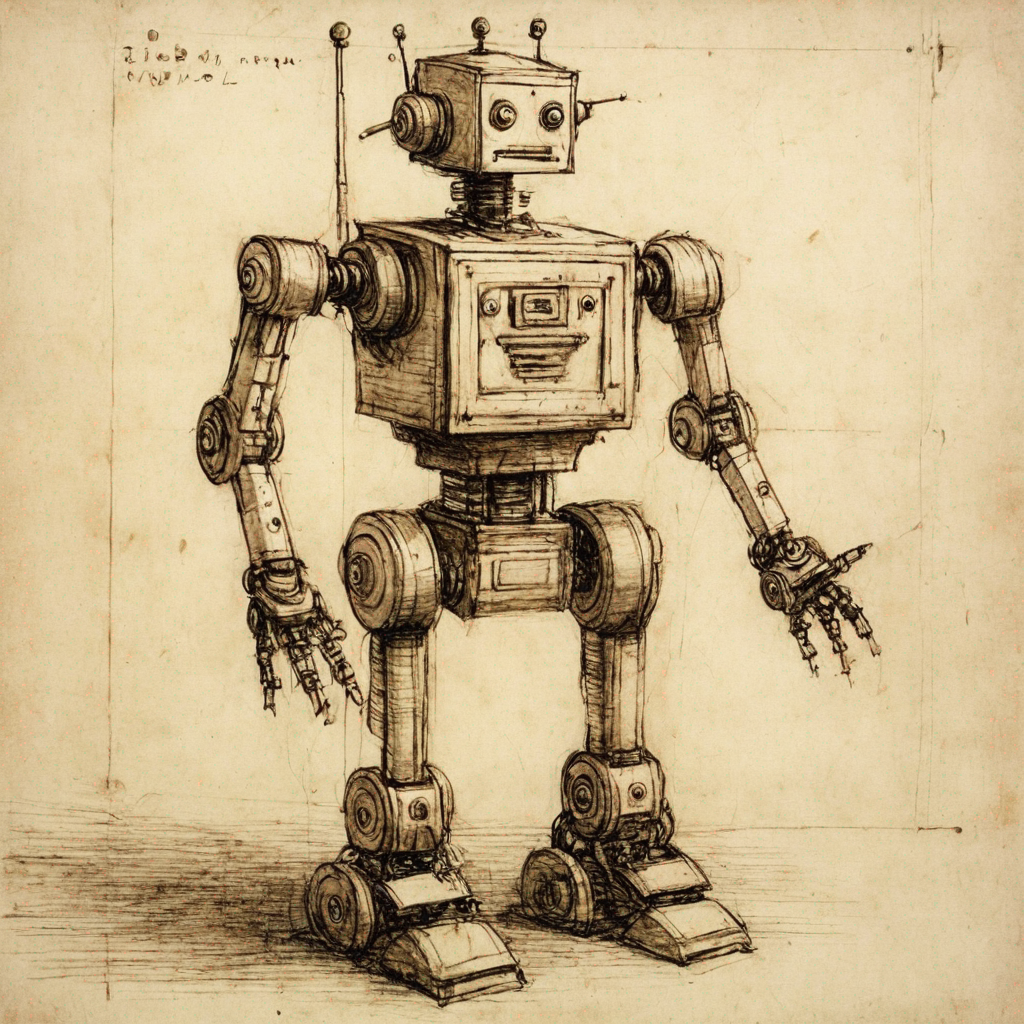
sdxl-davinci

5
sdxl-davinci is a fine-tuned version of the SDXL model, created by cbh123, that has been trained on Davinci drawings. This model is similar to other SDXL models like sdxl-allaprima, sdxl-shining, sdxl-money, sdxl-victorian-illustrations, and sdxl-2004, which have been fine-tuned on specific datasets to capture unique artistic styles and visual characteristics. Model inputs and outputs The sdxl-davinci model accepts a variety of inputs, including an image, prompt, and various parameters to control the output. The model can generate images based on the provided prompt, or perform tasks like image inpainting and refinement. The output is an array of one or more generated images. Inputs Prompt**: The text prompt that describes the desired image Image**: An input image to be used for tasks like img2img or inpainting Mask**: An input mask for the inpaint mode, where black areas will be preserved and white areas will be inpainted Width/Height**: The desired dimensions of the output image Seed**: A random seed value to control the image generation Refine**: The type of refinement to apply to the generated image Scheduler**: The scheduler algorithm to use for image generation LoRA Scale**: The scale to apply to any LoRA components Num Outputs**: The number of images to generate Refine Steps**: The number of refinement steps to apply Guidance Scale**: The scale for classifier-free guidance Apply Watermark**: Whether to apply a watermark to the generated image High Noise Frac**: The fraction of high noise to use for the expert_ensemble_refiner Negative Prompt**: An optional negative prompt to guide the image generation Outputs An array of one or more generated images Capabilities sdxl-davinci can generate a variety of artistic and illustrative images based on the provided prompt. The model's fine-tuning on Davinci drawings allows it to capture a unique and expressive style in the generated outputs. The model can also perform image inpainting and refinement tasks, allowing users to modify or enhance existing images. What can I use it for? The sdxl-davinci model can be used for a range of creative and artistic applications, such as generating illustrations, concept art, and digital paintings. Its ability to work with input images and masks makes it suitable for tasks like image editing, restoration, and enhancement. Additionally, the model's varied capabilities allow for experimentation and exploration of different artistic styles and compositions. Things to try One interesting aspect of the sdxl-davinci model is its ability to capture the expressive and dynamic qualities of Davinci's drawing style. Users can experiment with different prompts and input parameters to see how the model interprets and translates these artistic elements into unique and visually striking outputs. Additionally, the model's inpainting and refinement capabilities can be used to transform or enhance existing images, opening up opportunities for creative image manipulation and editing.
Updated Invalid Date