instant-style
Maintainer: jyoung105

1
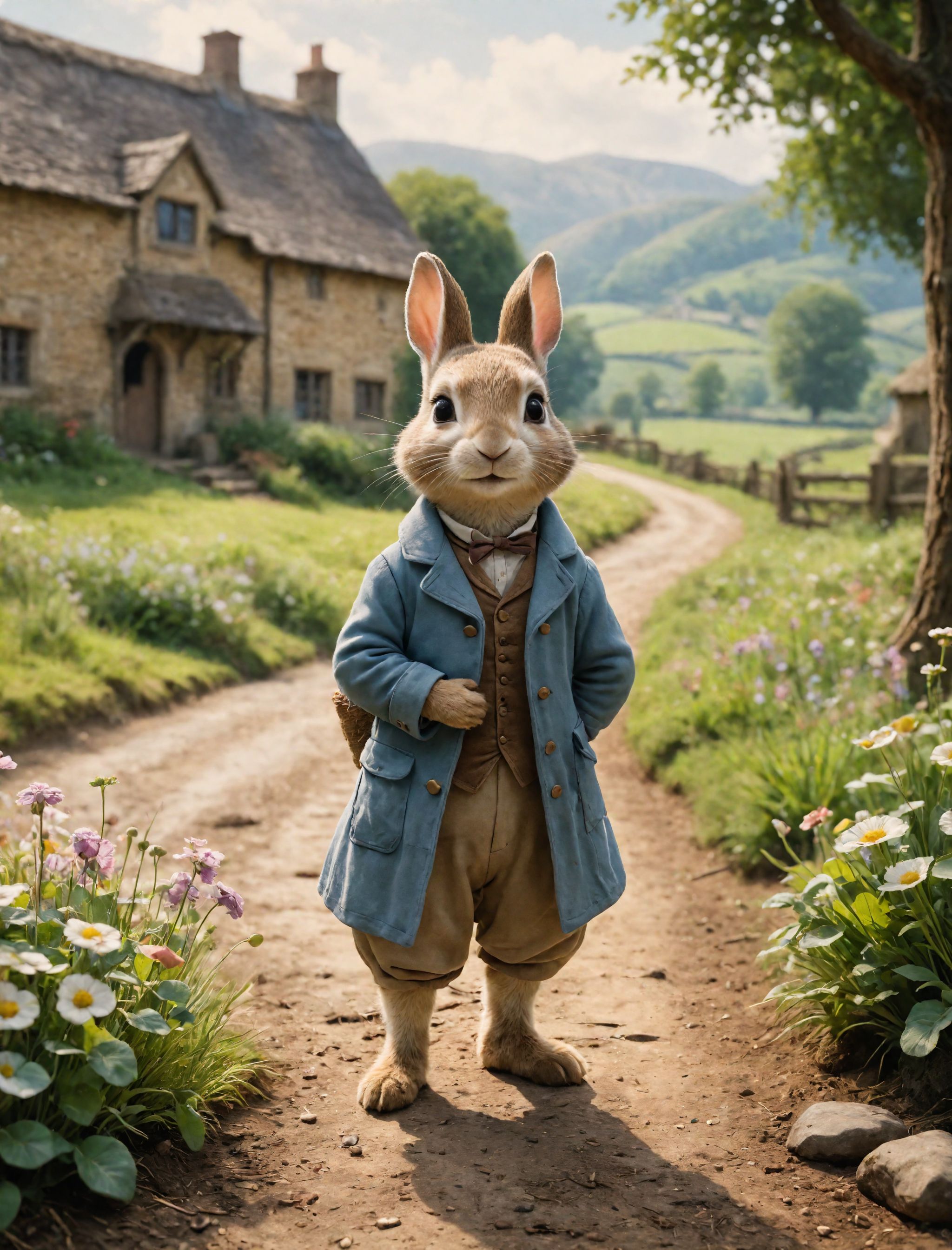
Property | Value |
---|---|
Run this model | Run on Replicate |
API spec | View on Replicate |
Github link | View on Github |
Paper link | View on Arxiv |
Create account to get full access
Model overview
instant-style
is a general framework developed by the InstantX team that employs two straightforward yet potent techniques for achieving an effective disentanglement of style and content from reference images. The key insights are separating content from the image by subtracting the content text features from the image features, and injecting the style into specific attention layers. This strategy is quite effective in mitigating content leakage compared to previous works like StyleMC and StyleCLIP.
Model inputs and outputs
instant-style
takes in a text prompt, an optional style image, and various configuration options to generate images that preserve the style of the reference image while following the content of the text prompt. The model outputs one or more generated images.
Inputs
- Prompt: The text prompt describing the desired image content.
- Style Image: An optional reference image to guide the style of the generated image.
- Seed: A random seed for reproducibility.
- Width/Height: The desired dimensions of the output image.
- Num Outputs: The number of images to generate.
- Guidance Scale: The scale for classifier-free guidance.
- Num Inference Steps: The number of denoising steps.
- Block Mode: The mode to reference the image: original, style with or without layout.
- Adapter Mode: The mode to reference the image: high flexibility but low fidelity or low flexibility but high fidelity.
- Style Strength: The conditioning scale for the IP-Adapter.
- Negative Prompt: The text prompt for content to exclude.
- Negative Content: The text prompt for style to exclude.
- Negative Content Strength: The conditioning scale for content to exclude.
Outputs
- Generated Images: One or more images generated based on the input prompt and style.
Capabilities
instant-style
can generate images that preserve the style of a reference image while following the content of a text prompt. It is particularly effective at maintaining the color, material, atmosphere, and spatial layout of the reference image. The model can also selectively control the style and layout components, allowing for fine-grained stylization.
What can I use it for?
instant-style
can be useful for a variety of applications, such as:
- Artistic Image Generation: Create visually striking images by combining a text prompt with a reference style image.
- Stylized Product Visualization: Generate product images with a desired aesthetic by providing a reference style.
- Augmented Reality and Virtual Try-On: Quickly generate stylized images of products or avatars for immersive experiences.
Things to try
Some interesting things to try with instant-style
include:
- Experimenting with different combinations of text prompts and style images to see how the model handles various types of content and styles.
- Trying different block and adapter modes to find the right balance between style preservation and content fidelity.
- Leveraging the selective style and layout control to create unique hybrid styles.
- Exploring the use of negative prompts to exclude certain style or content elements.
Overall, instant-style
provides a powerful and flexible framework for generating visually compelling images that preserve the desired style while following the provided text prompt.
This summary was produced with help from an AI and may contain inaccuracies - check out the links to read the original source documents!
Related Models
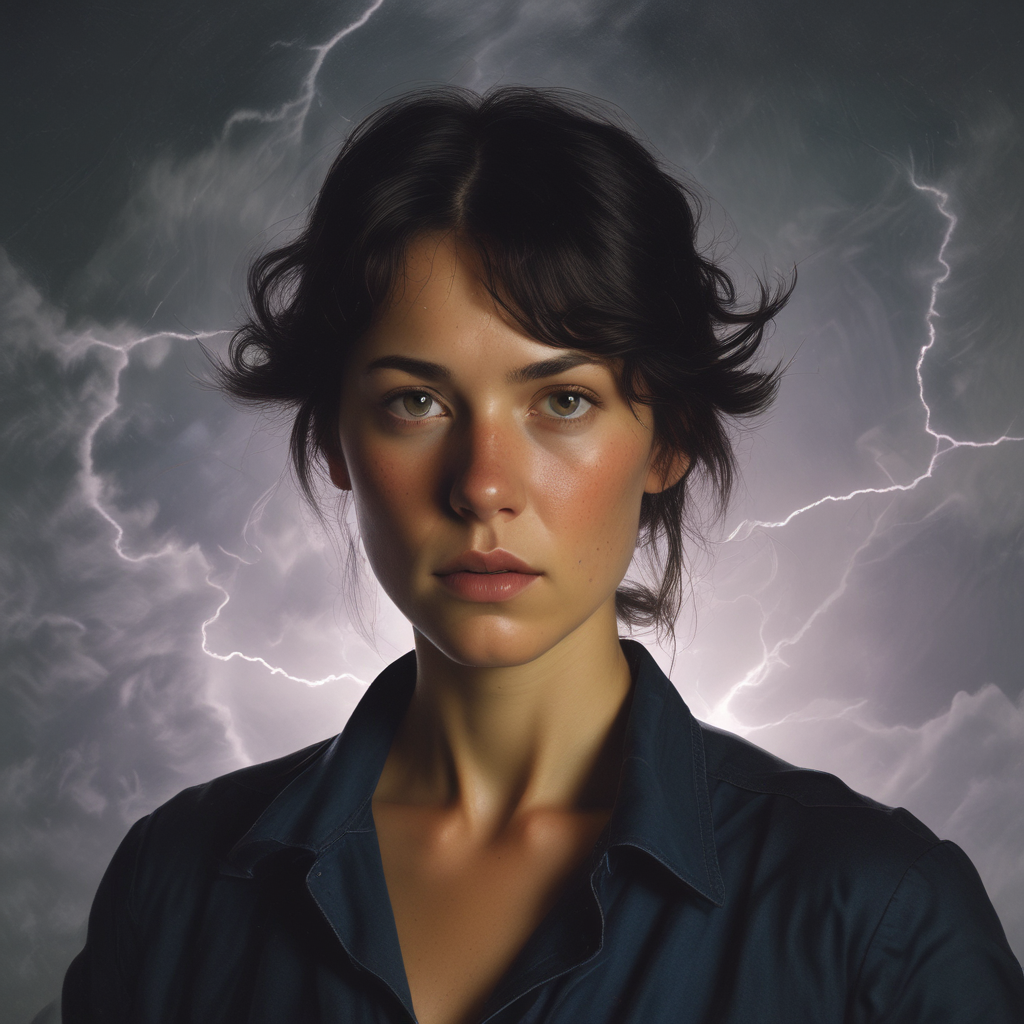
sdxl-lightning-4step

453.2K
sdxl-lightning-4step is a fast text-to-image model developed by ByteDance that can generate high-quality images in just 4 steps. It is similar to other fast diffusion models like AnimateDiff-Lightning and Instant-ID MultiControlNet, which also aim to speed up the image generation process. Unlike the original Stable Diffusion model, these fast models sacrifice some flexibility and control to achieve faster generation times. Model inputs and outputs The sdxl-lightning-4step model takes in a text prompt and various parameters to control the output image, such as the width, height, number of images, and guidance scale. The model can output up to 4 images at a time, with a recommended image size of 1024x1024 or 1280x1280 pixels. Inputs Prompt**: The text prompt describing the desired image Negative prompt**: A prompt that describes what the model should not generate Width**: The width of the output image Height**: The height of the output image Num outputs**: The number of images to generate (up to 4) Scheduler**: The algorithm used to sample the latent space Guidance scale**: The scale for classifier-free guidance, which controls the trade-off between fidelity to the prompt and sample diversity Num inference steps**: The number of denoising steps, with 4 recommended for best results Seed**: A random seed to control the output image Outputs Image(s)**: One or more images generated based on the input prompt and parameters Capabilities The sdxl-lightning-4step model is capable of generating a wide variety of images based on text prompts, from realistic scenes to imaginative and creative compositions. The model's 4-step generation process allows it to produce high-quality results quickly, making it suitable for applications that require fast image generation. What can I use it for? The sdxl-lightning-4step model could be useful for applications that need to generate images in real-time, such as video game asset generation, interactive storytelling, or augmented reality experiences. Businesses could also use the model to quickly generate product visualization, marketing imagery, or custom artwork based on client prompts. Creatives may find the model helpful for ideation, concept development, or rapid prototyping. Things to try One interesting thing to try with the sdxl-lightning-4step model is to experiment with the guidance scale parameter. By adjusting the guidance scale, you can control the balance between fidelity to the prompt and diversity of the output. Lower guidance scales may result in more unexpected and imaginative images, while higher scales will produce outputs that are closer to the specified prompt.
Updated Invalid Date
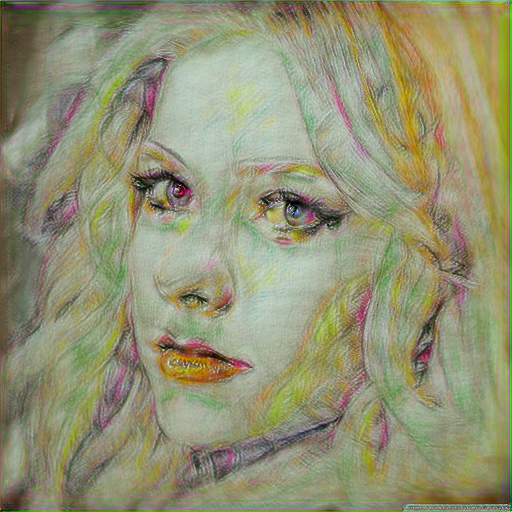
clipstyler

25
clipstyler is an AI model developed by Gihyun Kwon and Jong Chul Ye that enables image style transfer with a single text condition. It is similar to models like stable-diffusion, styleclip, and style-clip-draw that leverage text-to-image generation capabilities. However, clipstyler is unique in its ability to transfer the style of an image based on a single text prompt, rather than relying on a reference image. Model inputs and outputs The clipstyler model takes two inputs: an image and a text prompt. The image is used as the content that will have its style transferred, while the text prompt specifies the desired style. The model then outputs the stylized image, where the content of the input image has been transformed to match the requested style. Inputs Image**: The input image that will have its style transferred Text**: A text prompt describing the desired style to be applied to the input image Outputs Image**: The output image with the input content stylized according to the provided text prompt Capabilities clipstyler is capable of transferring the style of an image based on a single text prompt, without requiring a reference image. This allows for more flexibility and creativity in the style transfer process, as users can experiment with a wide range of styles by simply modifying the text prompt. The model leverages the CLIP text-image encoder to learn the relationship between textual style descriptions and visual styles, enabling it to produce high-quality stylized images. What can I use it for? The clipstyler model can be used for a variety of creative applications, such as: Artistic image generation**: Quickly generate stylized versions of your own images or photos, experimenting with different artistic styles and techniques. Concept visualization**: Bring your ideas to life by generating images that match a specific textual description, useful for designers, artists, and product developers. Content creation**: Enhance your digital content, such as blog posts, social media graphics, or marketing materials, by applying unique and custom styles to your images. Things to try One interesting aspect of clipstyler is its ability to produce diverse and unexpected results by experimenting with different text prompts. Try prompts that combine multiple styles or emotions, or explore abstract concepts like "surreal" or "futuristic" to see how the model interprets and translates these ideas into visual form. The variety of outcomes can spark new creative ideas and inspire you to push the boundaries of what's possible with text-driven style transfer.
Updated Invalid Date
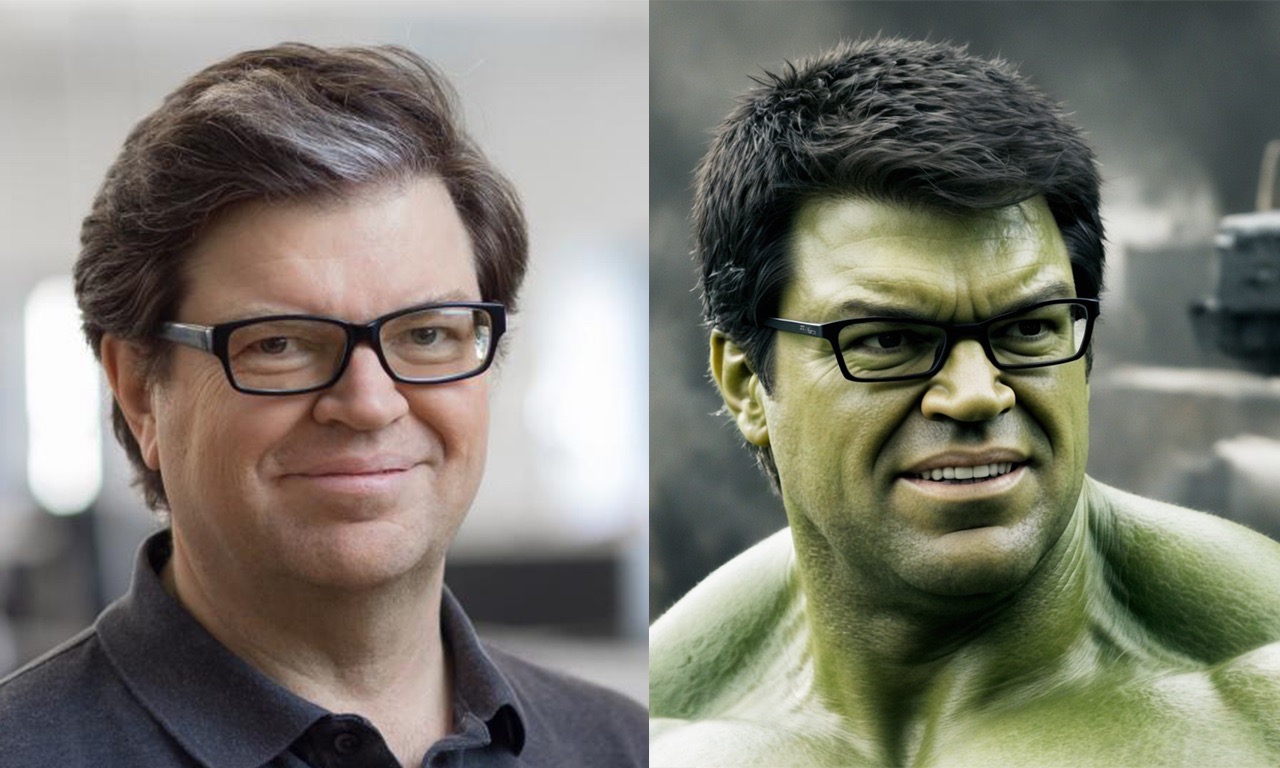
instant-id

609
instant-id is a state-of-the-art AI model developed by the InstantX team that can generate realistic images of real people instantly. It utilizes a tuning-free approach to achieve identity-preserving generation with only a single input image. The model is capable of various downstream tasks such as stylized synthesis, where it can blend the facial features and style of the input image. Compared to similar models like AbsoluteReality V1.8.1, Reliberate v3, Stable Diffusion, Photomaker, and Photomaker Style, instant-id achieves better fidelity and retains good text editability, allowing the generated faces and styles to blend more seamlessly. Model inputs and outputs instant-id takes a single input image of a face and a text prompt, and generates one or more realistic images that preserve the identity of the input face while incorporating the desired style and content from the text prompt. The model utilizes a novel identity-preserving generation technique that allows it to generate high-quality, identity-preserving images in a matter of seconds. Inputs Image**: The input face image used as a reference for the generated images. Prompt**: The text prompt describing the desired style and content of the generated images. Seed** (optional): A random seed value to control the randomness of the generated images. Pose Image** (optional): A reference image used to guide the pose of the generated images. Outputs Images**: One or more realistic images that preserve the identity of the input face while incorporating the desired style and content from the text prompt. Capabilities instant-id is capable of generating highly realistic images of people in a variety of styles and settings, while preserving the identity of the input face. The model can seamlessly blend the facial features and style of the input image, allowing for unique and captivating results. This makes the model a powerful tool for a wide range of applications, from creative content generation to virtual avatars and character design. What can I use it for? instant-id can be used for a variety of applications, such as: Creative Content Generation**: Quickly generate unique and realistic images for use in art, design, and multimedia projects. Virtual Avatars**: Create personalized virtual avatars that can be used in games, social media, or other digital environments. Character Design**: Develop realistic and expressive character designs for use in animation, films, or video games. Augmented Reality**: Integrate generated images into augmented reality experiences, allowing for the seamless blending of real and virtual elements. Things to try With instant-id, you can experiment with a wide range of text prompts and input images to generate unique and captivating results. Try prompts that explore different styles, genres, or themes, and see how the model can blend the facial features and aesthetics in unexpected ways. You can also experiment with different input images, from close-up portraits to more expressive or stylized faces, to see how the model adapts and responds. By pushing the boundaries of what's possible with identity-preserving generation, you can unlock a world of creative possibilities.
Updated Invalid Date
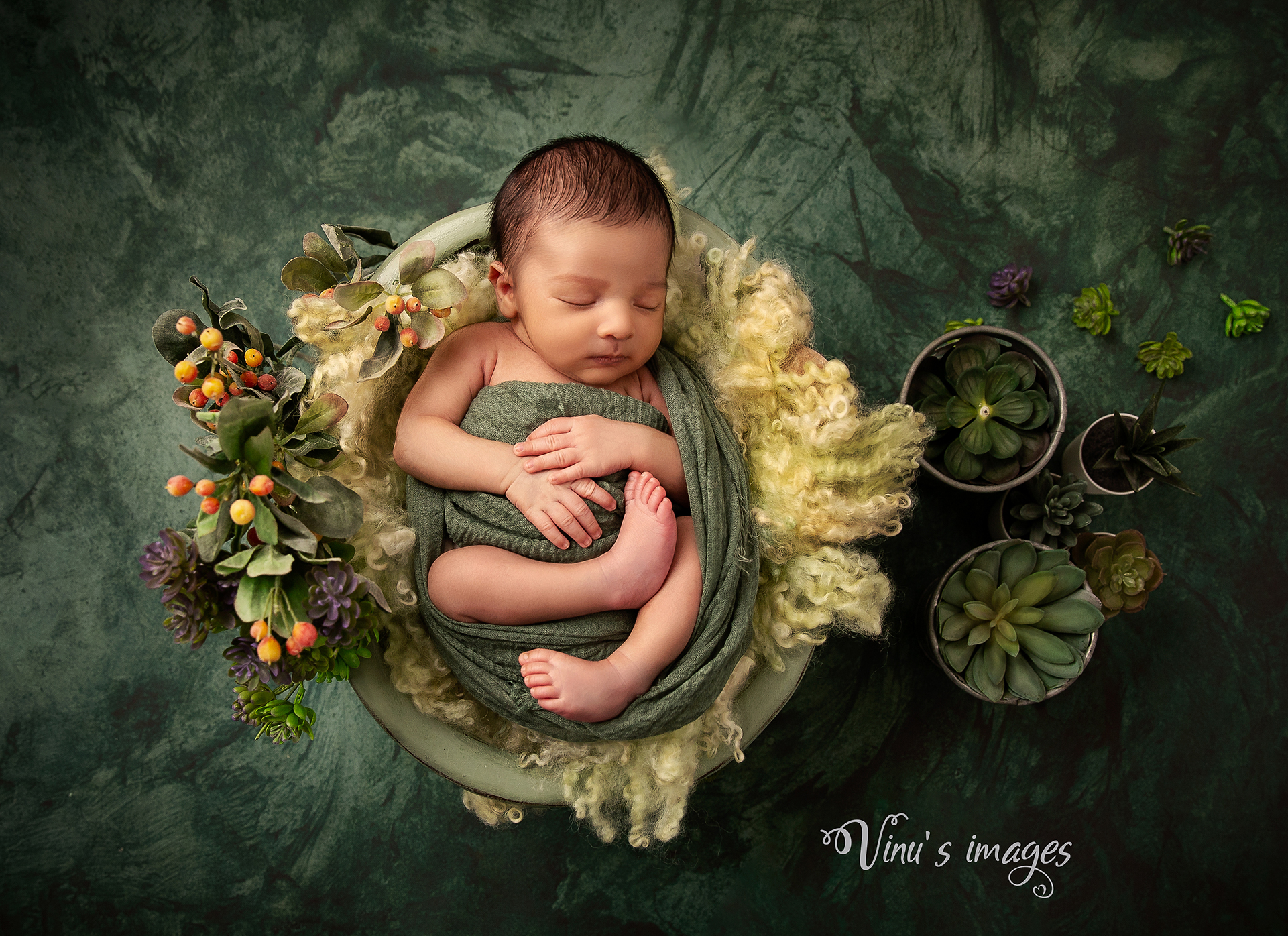
zero-shot-image-to-text

6
The zero-shot-image-to-text model is a cutting-edge AI model designed for the task of generating text descriptions from input images. Developed by researcher yoadtew, this model leverages a unique "zero-shot" approach to enable image-to-text generation without the need for task-specific fine-tuning. This sets it apart from similar models like stable-diffusion, uform-gen, and turbo-enigma which often require extensive fine-tuning for specific image-to-text tasks. Model inputs and outputs The zero-shot-image-to-text model takes in an image and produces a text description of that image. The model can handle a wide range of image types and subjects, from natural scenes to abstract concepts. Additionally, the model supports "visual-semantic arithmetic" - the ability to perform arithmetic operations on visual concepts to generate new images. Inputs Image**: The input image to be described Outputs Text Description**: A textual description of the input image Capabilities The zero-shot-image-to-text model has demonstrated impressive capabilities in generating detailed and coherent image descriptions across a diverse set of visual inputs. It can handle not only common objects and scenes, but also more complex visual reasoning tasks like understanding visual relationships and analogies. What can I use it for? The zero-shot-image-to-text model can be a valuable tool for a variety of applications, such as: Automated Image Captioning**: Generating descriptive captions for large image datasets, which can be useful for tasks like visual search, content moderation, and accessibility. Visual Question Answering**: Answering questions about the contents of an image, which can be helpful for building intelligent assistants or educational applications. Visual-Semantic Arithmetic**: Exploring and manipulating visual concepts in novel ways, which can inspire new creative applications or research directions. Things to try One interesting aspect of the zero-shot-image-to-text model is its ability to handle "visual-semantic arithmetic" - the ability to combine visual concepts in arithmetic-like operations to generate new, semantically meaningful images. For example, the model can take in images of a "woman", a "king", and a "man", and then generate a new image that represents the visual concept of "woman - king + man". This opens up fascinating possibilities for exploring the relationships between visual and semantic representations.
Updated Invalid Date