sdxl-controlnet-lora-inpaint
Maintainer: batouresearch

1
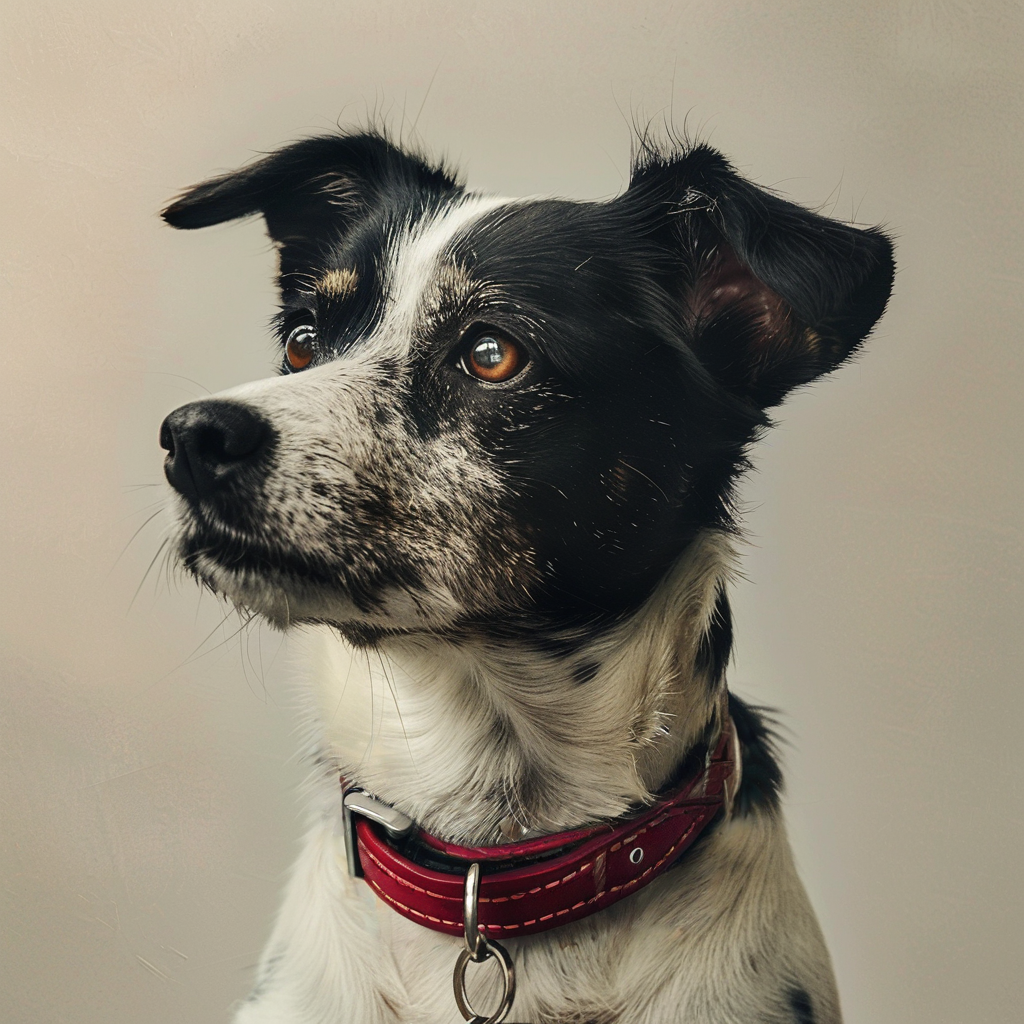
Property | Value |
---|---|
Run this model | Run on Replicate |
API spec | View on Replicate |
Github link | No Github link provided |
Paper link | No paper link provided |
Create account to get full access
Model overview
The sdxl-controlnet-lora-inpaint
model is a combination of several powerful AI techniques - ControlNet, LORA, and inpainting. This model builds upon similar models like [object Object], [object Object], and [object Object] by the same creator, batouresearch. It aims to provide a convenient all-in-one solution for image generation and inpainting.
Model inputs and outputs
The sdxl-controlnet-lora-inpaint
model takes in several inputs to generate images, including a prompt, image, mask, and various settings like seed, guidance scale, and number of inference steps. The generated images are output as a list of image URLs.
Inputs
- Prompt: The text prompt that describes the desired image.
- Image: An input image for img2img or inpaint mode.
- Mask: A mask image for the inpaint mode, where black areas will be preserved and white areas will be inpainted.
- Seed: A random seed value to control the image generation.
- Scheduler: The scheduler algorithm to use for the diffusion process.
- LoRA Scale: The additive scale for the LoRA weights.
- Num Outputs: The number of images to generate.
- LoRA Weights: The Replicate LoRA weights to use.
- Guidance Scale: The scale for classifier-free guidance.
- Condition Scale: The scale for how much the ControlNet should interfere.
- Negative Prompt: A text prompt to describe undesired elements in the image.
- Prompt Strength: The strength of the input prompt, where 1 means total destruction of the input image.
- Num Inference Steps: The number of denoising steps to perform during image generation.
Outputs
- Generated Images: A list of URLs for the generated images.
Capabilities
The sdxl-controlnet-lora-inpaint
model combines the power of ControlNet, LORA, and inpainting to provide a versatile image generation tool. It can generate images based on a text prompt, while also allowing for the use of input images and masks to guide the generation process. This makes it useful for a variety of tasks, such as creating concept art, enhancing existing images, and fixing or modifying specific areas of an image.
What can I use it for?
The sdxl-controlnet-lora-inpaint
model can be used for a wide range of creative and practical applications. Artists and designers can use it to generate concept art, explore new ideas, and refine existing images. Marketers can use it to create visuals for advertising and branding. Hobbyists can use it to generate unique and personalized images for their projects. Additionally, the inpainting capabilities of the model can be useful for tasks like photo restoration, object removal, and image editing.
Things to try
One interesting aspect of the sdxl-controlnet-lora-inpaint
model is the ability to fine-tune the generated images using the various input parameters. Experimenting with different prompt strengths, guidance scales, and condition scales can result in subtle or dramatic changes to the output, allowing you to explore a wide range of artistic possibilities. Additionally, the combination of ControlNet and inpainting techniques opens up new ways to manipulate and refine images, such as selectively modifying specific areas or features within an image.
This summary was produced with help from an AI and may contain inaccuracies - check out the links to read the original source documents!
Related Models
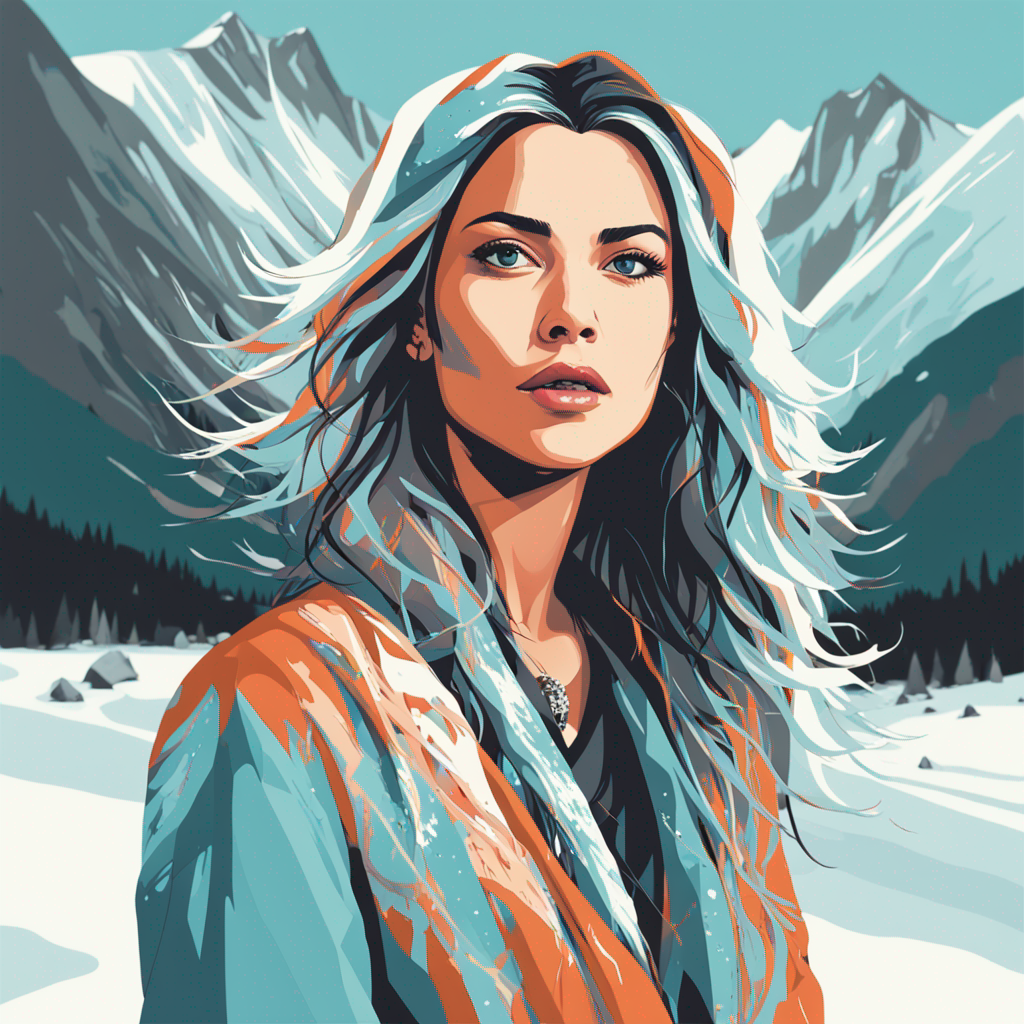
sdxl-controlnet-lora

646
The sdxl-controlnet-lora model is an implementation of Stability AI's SDXL text-to-image model with support for ControlNet and Replicate's LoRA technology. This model is developed and maintained by batouresearch, and is similar to other SDXL-based models like instant-id-multicontrolnet and sdxl-lightning-4step. The key difference is the addition of ControlNet, which allows the model to generate images based on a provided control image, such as a Canny edge map. Model inputs and outputs The sdxl-controlnet-lora model takes a text prompt, an optional input image, and various settings as inputs. It outputs one or more generated images based on the provided prompt and settings. Inputs Prompt**: The text prompt describing the image to generate. Image**: An optional input image to use as a control or base image for the generation process. Seed**: A random seed value to use for generation. Img2Img**: A flag to enable the img2img generation pipeline, which uses the input image as both the control and base image. Strength**: The strength of the img2img denoising process, ranging from 0 to 1. Negative Prompt**: An optional negative prompt to guide the generation away from certain undesired elements. Num Inference Steps**: The number of denoising steps to take during the generation process. Guidance Scale**: The scale for classifier-free guidance, which controls the influence of the text prompt on the generated image. Scheduler**: The scheduler algorithm to use for the generation process. LoRA Scale**: The additive scale for the LoRA weights, which can be used to fine-tune the model's behavior. LoRA Weights**: The URL of the Replicate LoRA weights to use for the generation. Outputs Generated Images**: One or more images generated based on the provided inputs. Capabilities The sdxl-controlnet-lora model is capable of generating high-quality, photorealistic images based on text prompts. The addition of ControlNet support allows the model to generate images based on a provided control image, such as a Canny edge map, enabling more precise control over the generated output. The LoRA technology further enhances the model's flexibility by allowing for easy fine-tuning and customization. What can I use it for? The sdxl-controlnet-lora model can be used for a variety of image generation tasks, such as creating concept art, product visualizations, or custom illustrations. The ability to use a control image can be particularly useful for tasks like image inpainting, where the model can generate content to fill in missing or damaged areas of an image. Additionally, the fine-tuning capabilities enabled by LoRA can make the model well-suited for specialized applications or personalized use cases. Things to try One interesting thing to try with the sdxl-controlnet-lora model is experimenting with different control images and LoRA weight sets to see how they affect the generated output. You could, for example, try using a Canny edge map, a depth map, or a segmentation mask as the control image, and see how the model's interpretation of the prompt changes. Additionally, you could explore using LoRA to fine-tune the model for specific styles or subject matter, and see how that impacts the generated images.
Updated Invalid Date
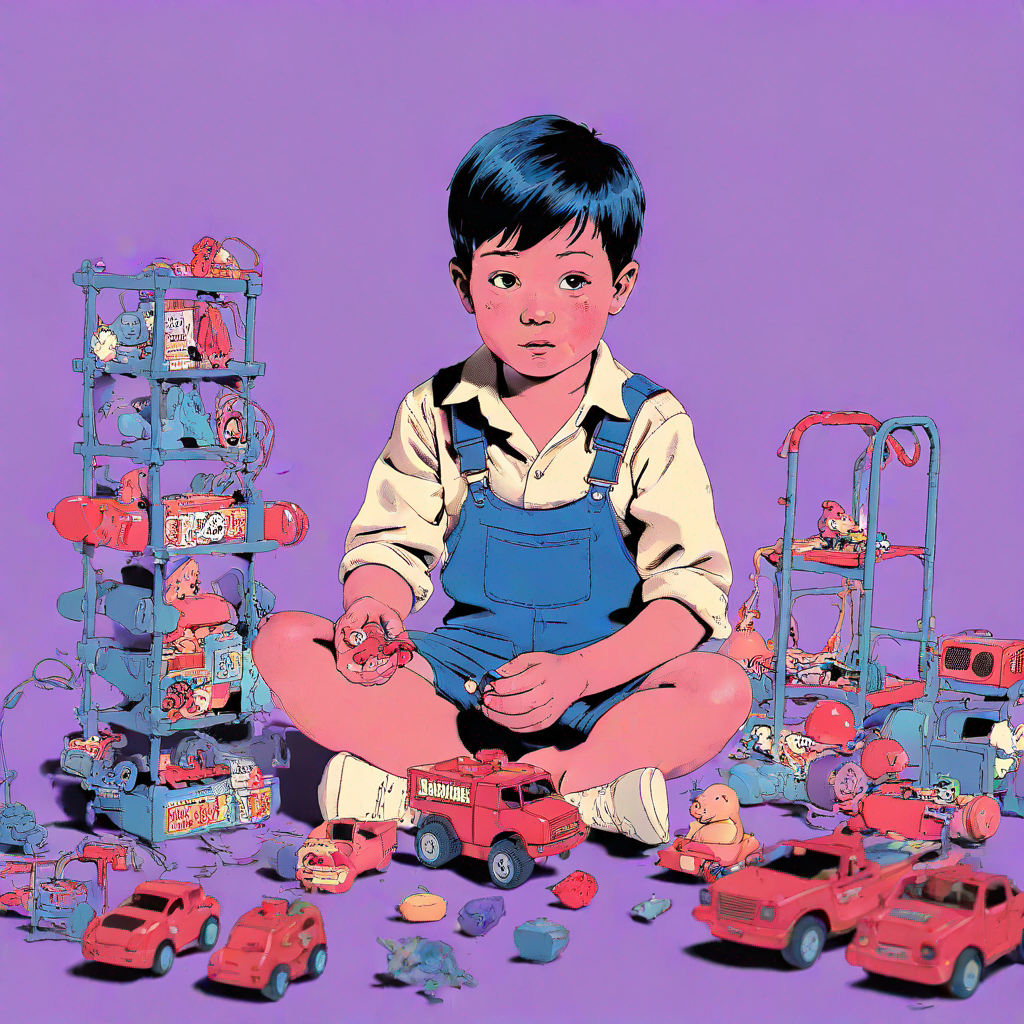
sdxl-lcm-lora-controlnet

12
The sdxl-lcm-lora-controlnet model is an all-in-one AI model developed by batouresearch that combines Stability AI's SDXL model with LCM LoRA for faster inference and ControlNet capabilities. This model builds upon similar models like the sdxl-controlnet-lora, open-dalle-1.1-lora, and sdxl-multi-controlnet-lora to provide an efficient and versatile all-in-one solution for text-to-image generation. Model inputs and outputs The sdxl-lcm-lora-controlnet model accepts a variety of inputs, including a text prompt, an optional input image, a seed value, and various settings to control the output, such as resolution, guidance scale, and LoRA scale. The model can generate one or more images based on the provided inputs. Inputs Prompt**: The text prompt that describes the desired output image. Image**: An optional input image that can be used for img2img or inpaint mode. Seed**: A random seed value that can be used to generate reproducible outputs. Resolution**: The desired width and height of the output image. Scheduler**: The scheduler to use for the diffusion process, with the default being LCM. Number of outputs**: The number of images to generate. LoRA scale**: The additive scale for the LoRA weights. ControlNet image**: An optional input image that will be converted to a Canny edge image and used as a conditioning input. Outputs Images**: One or more generated images that match the provided prompt. Capabilities The sdxl-lcm-lora-controlnet model is capable of generating high-quality images from text prompts, leveraging the power of the SDXL model combined with the efficiency of LCM LoRA and the control provided by ControlNet. This model excels at generating a wide range of image types, from realistic scenes to fantastical and imaginative creations. What can I use it for? The sdxl-lcm-lora-controlnet model can be used for a variety of applications, including: Creative content generation**: Produce unique, high-quality images for use in art, advertising, or entertainment. Prototyping and visualization**: Generate visual concepts and mockups to aid in the design and development process. Educational and research purposes**: Explore the capabilities of text-to-image AI models and experiment with different prompts and settings. Things to try With the sdxl-lcm-lora-controlnet model, you can explore the power of combining SDXL, LCM LoRA, and ControlNet by trying different prompts, input images, and settings. Experiment with the LoRA scale and condition scale to see how they affect the output, or use the ControlNet input to guide the generation process in specific ways.
Updated Invalid Date
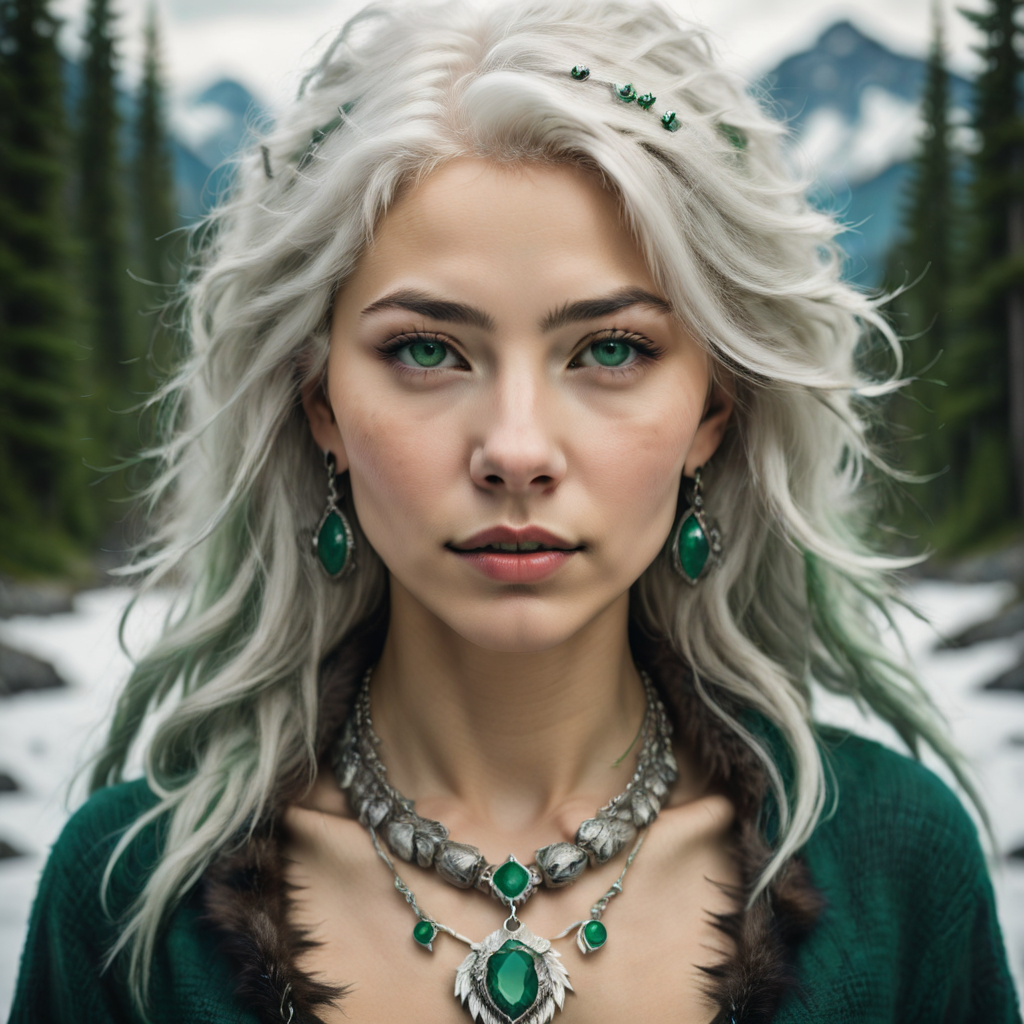
open-dalle-1.1-lora

120
The open-dalle-1.1-lora model, created by batouresearch, is an improved text-to-image generation model that builds upon the capabilities of the original DALL-E model. This model is particularly adept at prompt adherence and generating high-quality images, surpassing the performance of the SDXL model in these areas. Similar models from batouresearch include the sdxl-controlnet-lora, sdxl-outpainting-lora, and magic-image-refiner. Model inputs and outputs The open-dalle-1.1-lora model accepts a variety of inputs, including an input prompt, image, and mask for inpainting tasks. Users can also specify parameters like image size, seed, and scheduler. The model outputs one or more generated images as image URIs. Inputs Prompt**: The input prompt describing the desired image Negative Prompt**: An optional prompt describing elements to exclude from the generated image Image**: An input image for img2img or inpaint mode Mask**: An input mask for inpaint mode, where black areas will be preserved and white areas will be inpainted Seed**: A random seed, which can be left blank to randomize Width/Height**: The desired dimensions of the output image Num Outputs**: The number of images to generate (up to 4) Scheduler**: The scheduler algorithm to use for image generation Guidance Scale**: The scale for classifier-free guidance Num Inference Steps**: The number of denoising steps to perform Outputs Output Images**: One or more generated images as image URIs Capabilities The open-dalle-1.1-lora model excels at generating high-quality images that closely adhere to the provided prompt. Compared to the SDXL model, it produces images with improved detail, coherence, and faithfulness to the input text. This model can be particularly useful for tasks like illustration, product visualization, and conceptual art generation. What can I use it for? The open-dalle-1.1-lora model can be used for a variety of creative and commercial applications. For example, you could use it to generate concept art for a new product, illustrate a children's book, or create unique digital art pieces. The model's strong prompt adherence and image quality make it a valuable tool for designers, artists, and content creators looking to quickly and easily generate high-quality visuals. Additionally, the sdxl-controlnet-lora and sdxl-outpainting-lora models from batouresearch offer additional capabilities for tasks like image-to-image translation and outpainting. Things to try One interesting aspect of the open-dalle-1.1-lora model is its ability to generate images that capture subtle details and nuances specified in the input prompt. For example, you could try using the model to create detailed, fantastical scenes that blend realistic elements with imaginative, whimsical components. Experimenting with different prompts and prompt engineering techniques can help you unlock the full potential of this powerful text-to-image generation tool.
Updated Invalid Date
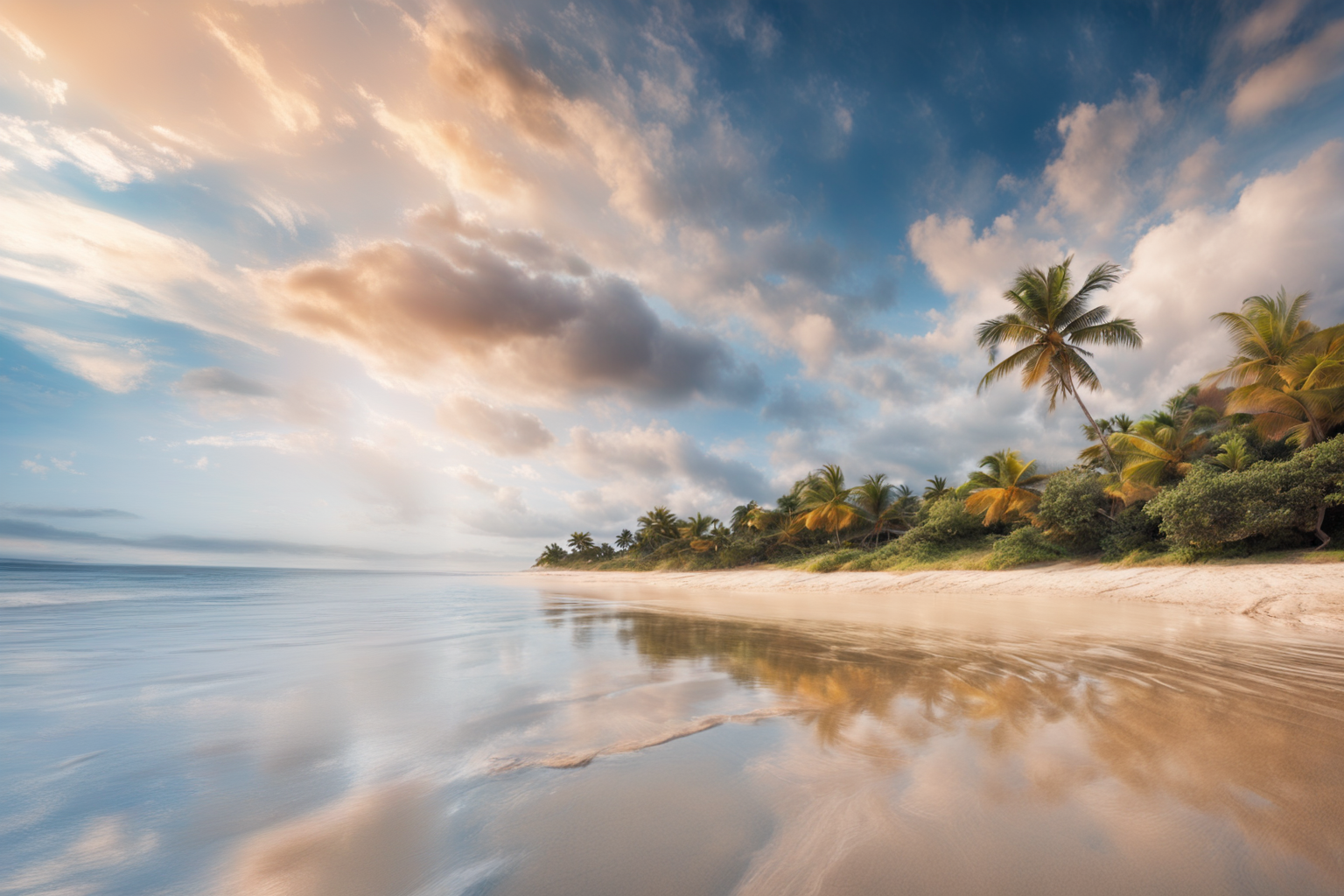
sdxl-outpainting-lora

57
The sdxl-outpainting-lora model is an improved version of Stability AI's SDXL outpainting model, which supports LoRA (Low-Rank Adaptation) for fine-tuning the model. This model uses PatchMatch, an algorithm that improves the quality of the generated mask, allowing for more seamless outpainting. The model is implemented as a Cog model, making it easy to use as a cloud API. Model inputs and outputs The sdxl-outpainting-lora model takes a variety of inputs, including a prompt, an input image, a seed, and various parameters to control the outpainting and generation process. The model outputs one or more generated images that extend the input image in the specified direction. Inputs Prompt**: The text prompt that describes the desired output image. Image**: The input image to be outpainted. Seed**: The random seed to use for generation, allowing for reproducible results. Scheduler**: The scheduler algorithm to use for the diffusion process. LoRA Scale**: The scale to apply to the LoRA weights, which can be used to fine-tune the model. Num Outputs**: The number of output images to generate. LoRA Weights**: The LoRA weights to use, which must be from the Replicate platform. Outpaint Size**: The size of the outpainted region, in pixels. Guidance Scale**: The scale to apply to the classifier-free guidance, which controls the balance between the prompt and the input image. Apply Watermark**: Whether to apply a watermark to the generated images. Condition Scale**: The scale to apply to the ControlNet guidance, which controls the influence of the input image. Negative Prompt**: An optional negative prompt to guide the generation away from certain outputs. Outpaint Direction**: The direction in which to outpaint the input image. Outputs Generated Images**: The one or more output images that extend the input image in the specified direction. Capabilities The sdxl-outpainting-lora model is capable of seamlessly outpainting input images in a variety of directions, using the PatchMatch algorithm to improve the quality of the generated mask. The model can be fine-tuned using LoRA, allowing for customization and adaptation to specific use cases. What can I use it for? The sdxl-outpainting-lora model can be used for a variety of applications, such as: Image Editing**: Extending the canvas of existing images to create new compositions or add additional context. Creative Expression**: Generating unique and imaginative outpainted images based on user prompts. Architectural Visualization**: Extending architectural renderings or product images to showcase more of the environment or surroundings. Things to try Some interesting things to try with the sdxl-outpainting-lora model include: Experimenting with different LoRA scales to see how it affects the output quality and fidelity. Trying out various prompts and input images to see the range of outputs the model can generate. Combining the outpainting capabilities with other AI models, such as GFPGAN for face restoration or stable-diffusion-inpainting for more advanced inpainting.
Updated Invalid Date