sdxl-panoramic
Maintainer: lucataco

6
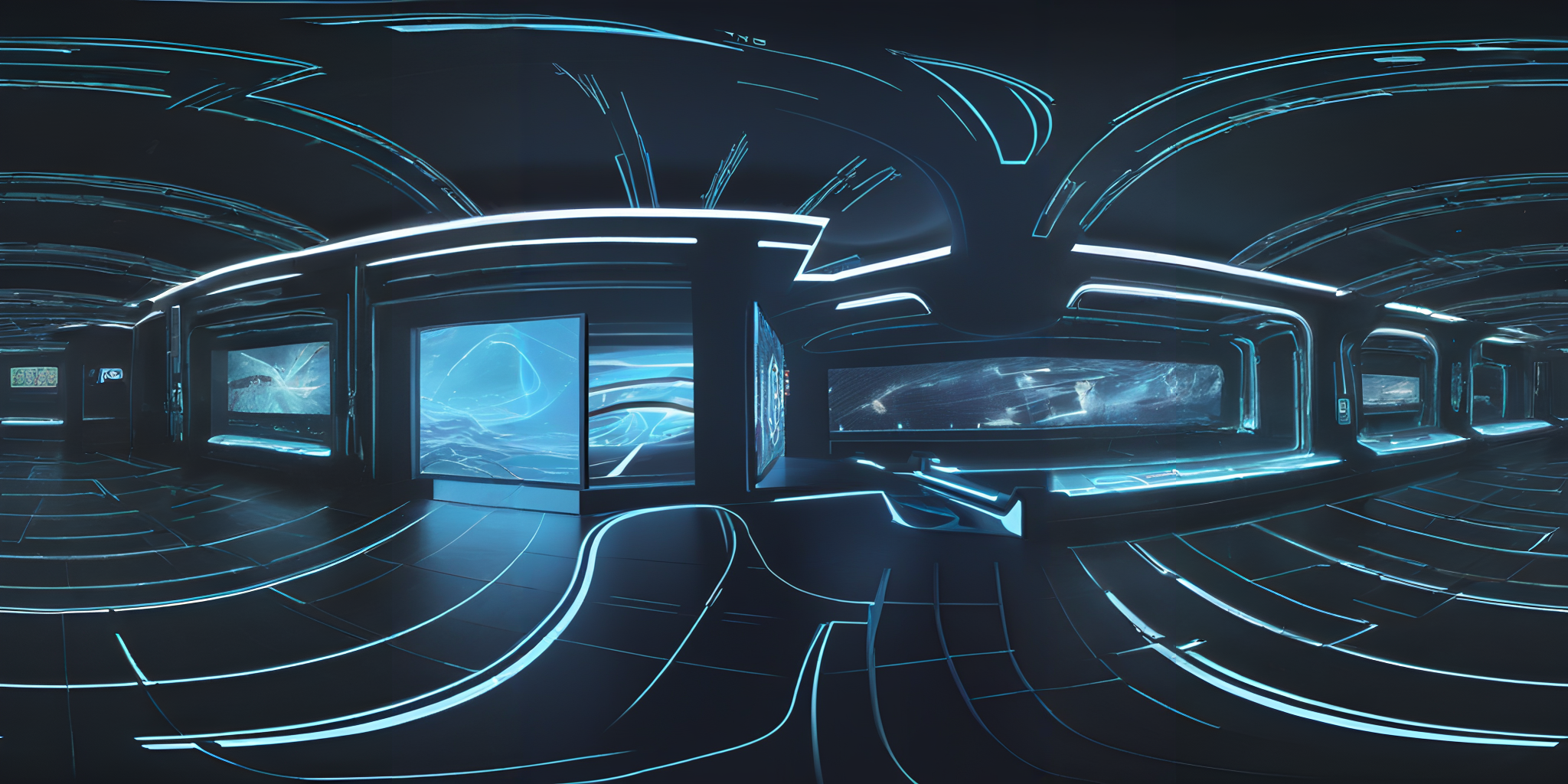
Property | Value |
---|---|
Run this model | Run on Replicate |
API spec | View on Replicate |
Github link | View on Github |
Paper link | No paper link provided |
Create account to get full access
Model overview
The sdxl-panoramic
is a custom AI model developed by Replicate that generates seamless 360-degree panoramic images. It builds upon Replicate's previous work on the [object Object], [object Object], and [object Object] models, incorporating techniques like GFPGAN upscaling and image inpainting to create a high-quality, panoramic output.
Model inputs and outputs
The sdxl-panoramic
model takes a text prompt as its input, and generates a 360-degree panoramic image as output. The input prompt can describe the desired scene or content, and the model will generate an image that matches the prompt.
Inputs
- Prompt: A text description of the desired panoramic image.
- Seed: An optional integer value to control the random number generator and produce consistent outputs.
Outputs
- Image: A seamless 360-degree panoramic image, generated based on the input prompt.
Capabilities
The sdxl-panoramic
model is capable of generating a wide variety of panoramic scenes, from futuristic cityscapes to fantastical landscapes. It can handle complex prompts and produce highly detailed, immersive images. The model's ability to seamlessly stitch together the panoramic output is a key feature that sets it apart from other text-to-image models.
What can I use it for?
The sdxl-panoramic
model could be used to create visually stunning backgrounds or environments for various applications, such as virtual reality experiences, video game environments, or architectural visualizations. Its panoramic output could also be used in marketing, advertising, or social media content to capture a sense of scale and immersion.
Things to try
Try experimenting with different prompts that describe expansive, panoramic scenes, such as "a sprawling cyberpunk city at night" or "a lush, alien world with towering mountains and flowing rivers." The model's ability to handle complex, detailed prompts and produce cohesive, 360-degree images is a key strength to explore.
This summary was produced with help from an AI and may contain inaccuracies - check out the links to read the original source documents!
Related Models
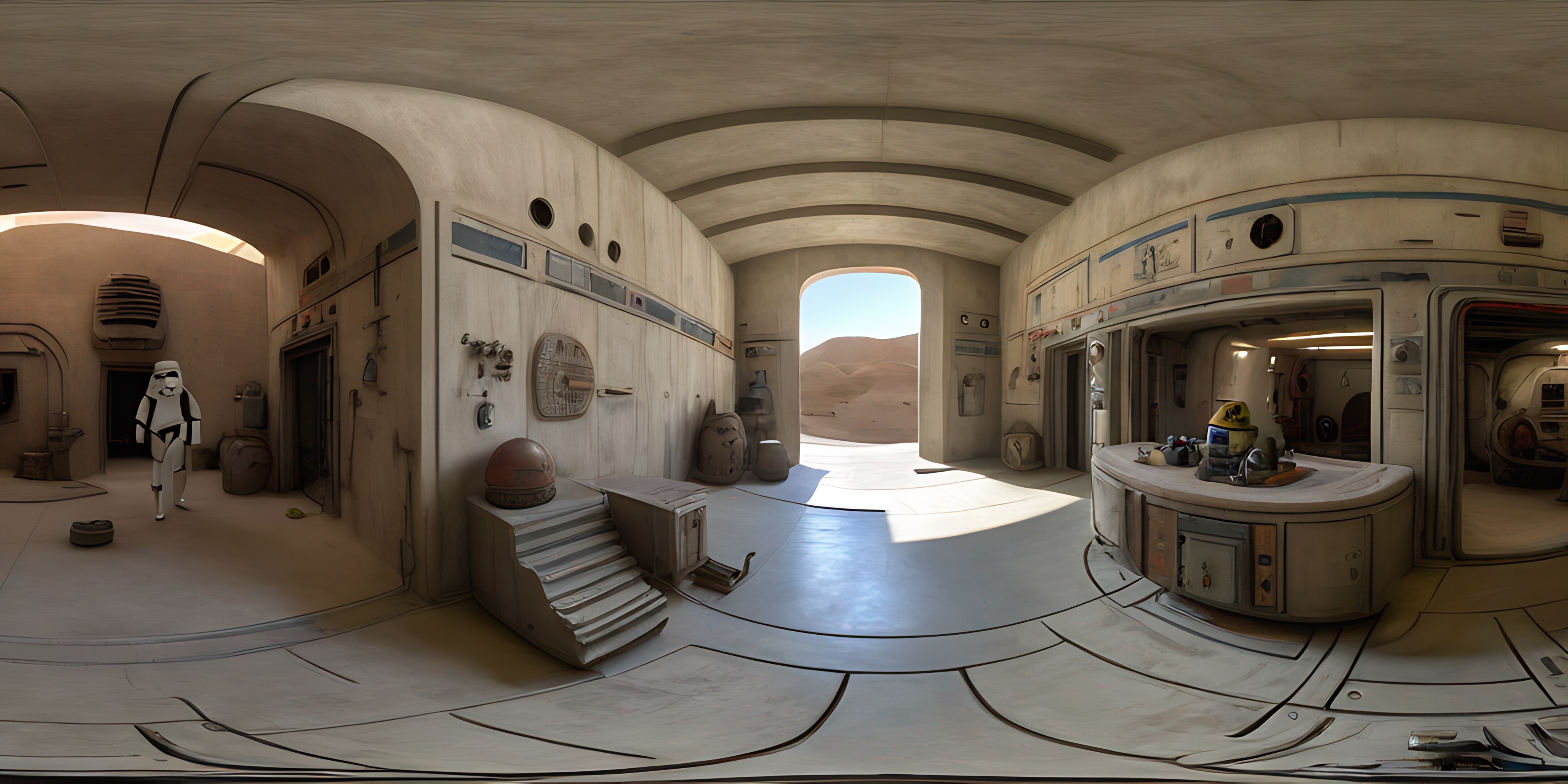
sdxl-panorama

1
The sdxl-panorama model is a version of the Stable Diffusion XL (SDXL) model that has been fine-tuned for panoramic image generation. This model builds on the capabilities of similar SDXL-based models, such as sdxl-recur, sdxl-controlnet-lora, sdxl-outpainting-lora, sdxl-black-light, and sdxl-deep-down, each of which focuses on a specific aspect of image generation. Model inputs and outputs The sdxl-panorama model takes a variety of inputs, including a prompt, image, seed, and various parameters to control the output. It generates panoramic images based on the provided input. Inputs Prompt**: The text prompt that describes the desired image. Image**: An input image for img2img or inpaint mode. Mask**: An input mask for inpaint mode, where black areas will be preserved and white areas will be inpainted. Seed**: A random seed to control the output. Width and Height**: The desired dimensions of the output image. Refine**: The refine style to use. Scheduler**: The scheduler to use for the diffusion process. LoRA Scale**: The LoRA additive scale, which is only applicable on trained models. Num Outputs**: The number of images to output. Refine Steps**: The number of steps to refine, which defaults to num_inference_steps. Guidance Scale**: The scale for classifier-free guidance. Apply Watermark**: A boolean to determine whether to apply a watermark to the output image. High Noise Frac**: The fraction of noise to use for the expert_ensemble_refiner. Negative Prompt**: An optional negative prompt to guide the image generation. Prompt Strength**: The prompt strength when using img2img or inpaint mode. Num Inference Steps**: The number of denoising steps to perform. Outputs Output Images**: The generated panoramic images. Capabilities The sdxl-panorama model is capable of generating high-quality panoramic images based on the provided inputs. It can produce detailed and visually striking landscapes, cityscapes, and other panoramic scenes. The model can also be used for image inpainting and manipulation, allowing users to refine and enhance existing images. What can I use it for? The sdxl-panorama model can be useful for a variety of applications, such as creating panoramic images for virtual tours, film and video production, architectural visualization, and landscape photography. The model's ability to generate and manipulate panoramic images can be particularly valuable for businesses and creators looking to showcase their products, services, or artistic visions in an immersive and engaging way. Things to try One interesting aspect of the sdxl-panorama model is its ability to generate seamless and coherent panoramic images from a variety of input prompts and images. You could try experimenting with different types of scenes, architectural styles, or natural landscapes to see how the model handles the challenges of panoramic image generation. Additionally, you could explore the model's inpainting capabilities by providing partial images or masked areas and observing how it fills in the missing details.
Updated Invalid Date
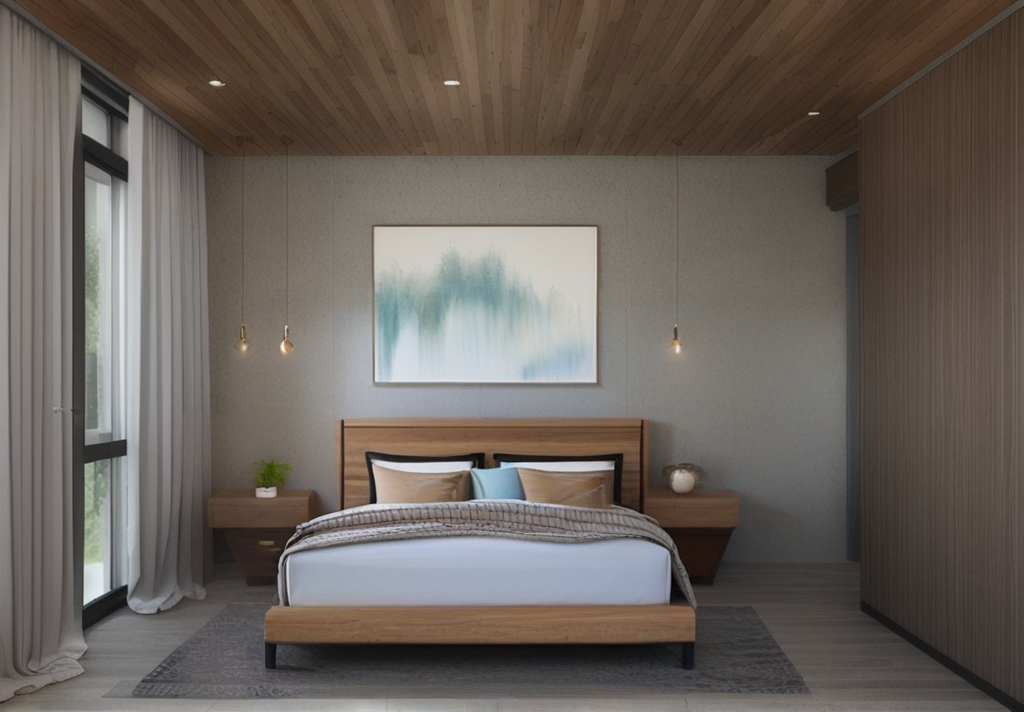
sdxl-inpainting

798
The sdxl-inpainting model is an implementation of the Stable Diffusion XL Inpainting model developed by the Hugging Face Diffusers team. This model allows you to fill in masked parts of images using the power of Stable Diffusion. It is similar to other inpainting models like the stable-diffusion-inpainting model from Stability AI, but with some additional capabilities. Model inputs and outputs The sdxl-inpainting model takes in an input image, a mask image, and a prompt to guide the inpainting process. It outputs one or more inpainted images that match the prompt. The model also allows you to control various parameters like the number of denoising steps, guidance scale, and random seed. Inputs Image**: The input image that you want to inpaint. Mask**: A mask image that specifies the areas to be inpainted. Prompt**: The text prompt that describes the desired output image. Negative Prompt**: A prompt that describes what should not be present in the output image. Seed**: A random seed to control the generation process. Steps**: The number of denoising steps to perform. Strength**: The strength of the inpainting, where 1.0 corresponds to full destruction of the input image. Guidance Scale**: The guidance scale, which controls how strongly the model follows the prompt. Scheduler**: The scheduler to use for the diffusion process. Num Outputs**: The number of output images to generate. Outputs Output Images**: One or more inpainted images that match the provided prompt. Capabilities The sdxl-inpainting model can be used to fill in missing or damaged areas of an image, while maintaining the overall style and composition. This can be useful for tasks like object removal, image restoration, and creative image manipulation. The model's ability to generate high-quality inpainted results makes it a powerful tool for a variety of applications. What can I use it for? The sdxl-inpainting model can be used for a wide range of applications, such as: Image Restoration**: Repairing damaged or corrupted images by filling in missing or degraded areas. Object Removal**: Removing unwanted objects from images, such as logos, people, or other distracting elements. Creative Image Manipulation**: Exploring new visual concepts by selectively modifying or enhancing parts of an image. Product Photography**: Removing backgrounds or other distractions from product images to create clean, professional-looking shots. The model's flexibility and high-quality output make it a valuable tool for both professional and personal use cases. Things to try One interesting thing to try with the sdxl-inpainting model is experimenting with different prompts to see how the model handles various types of content. You could try inpainting scenes, objects, or even abstract patterns. Additionally, you can play with the model's parameters, such as the strength and guidance scale, to see how they affect the output. Another interesting approach is to use the sdxl-inpainting model in conjunction with other AI models, such as the dreamshaper-xl-lightning model or the pasd-magnify model, to create more sophisticated image manipulation workflows.
Updated Invalid Date
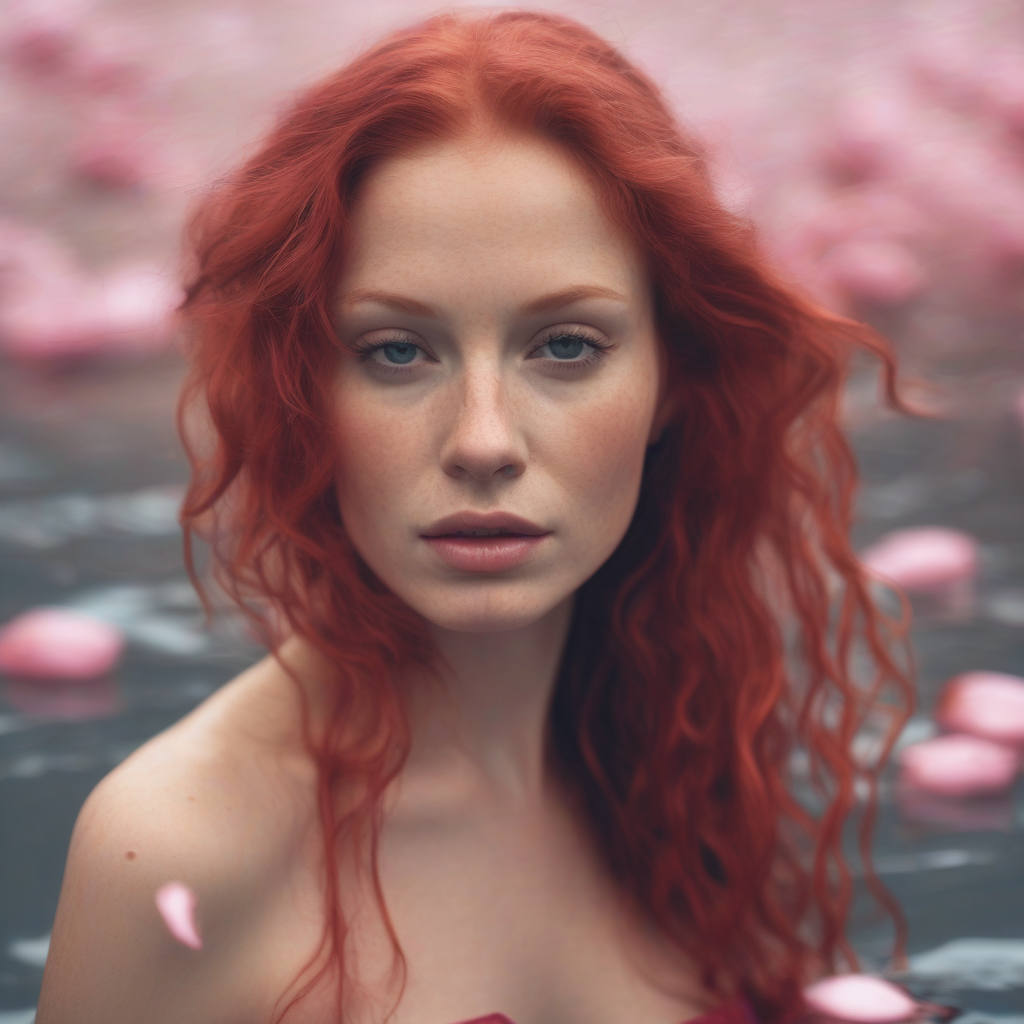
sdxl-img-blend

42
The sdxl-img-blend model is an implementation of an SDXL Image Blending model using Compel as a Cog model. Developed by lucataco, this model is part of the SDXL family of models, which also includes SDXL Inpainting, SDXL Panoramic, SDXL, SDXL_Niji_Special Edition, and SDXL CLIP Interrogator. Model inputs and outputs The sdxl-img-blend model takes two input images and blends them together using various parameters such as strength, guidance scale, and number of inference steps. The output is a single image that combines the features of the two input images. Inputs image1: The first input image image2: The second input image strength1: The strength of the first input image strength2: The strength of the second input image guidance_scale: The scale for classifier-free guidance num_inference_steps: The number of denoising steps scheduler: The scheduler to use for the diffusion process seed: The seed for the random number generator Outputs output: The blended image Capabilities The sdxl-img-blend model can be used to create unique and visually interesting images by blending two input images. The model allows for fine-tuning of the blending process through the various input parameters, enabling users to experiment and find the perfect balance between the two images. What can I use it for? The sdxl-img-blend model can be used for a variety of creative projects, such as generating cover art, designing social media posts, or creating unique digital artwork. The ability to blend images in this way can be especially useful for artists, designers, and content creators who are looking to add a touch of creativity and visual interest to their projects. Things to try One interesting thing to try with the sdxl-img-blend model is experimenting with different combinations of input images. By adjusting the strength and other parameters, you can create a wide range of blended images, from subtle and harmonious to more abstract and surreal. Additionally, you can try using the model to blend images of different styles, such as a realistic photograph and a stylized illustration, to see how the model handles the contrast and creates a unique result.
Updated Invalid Date
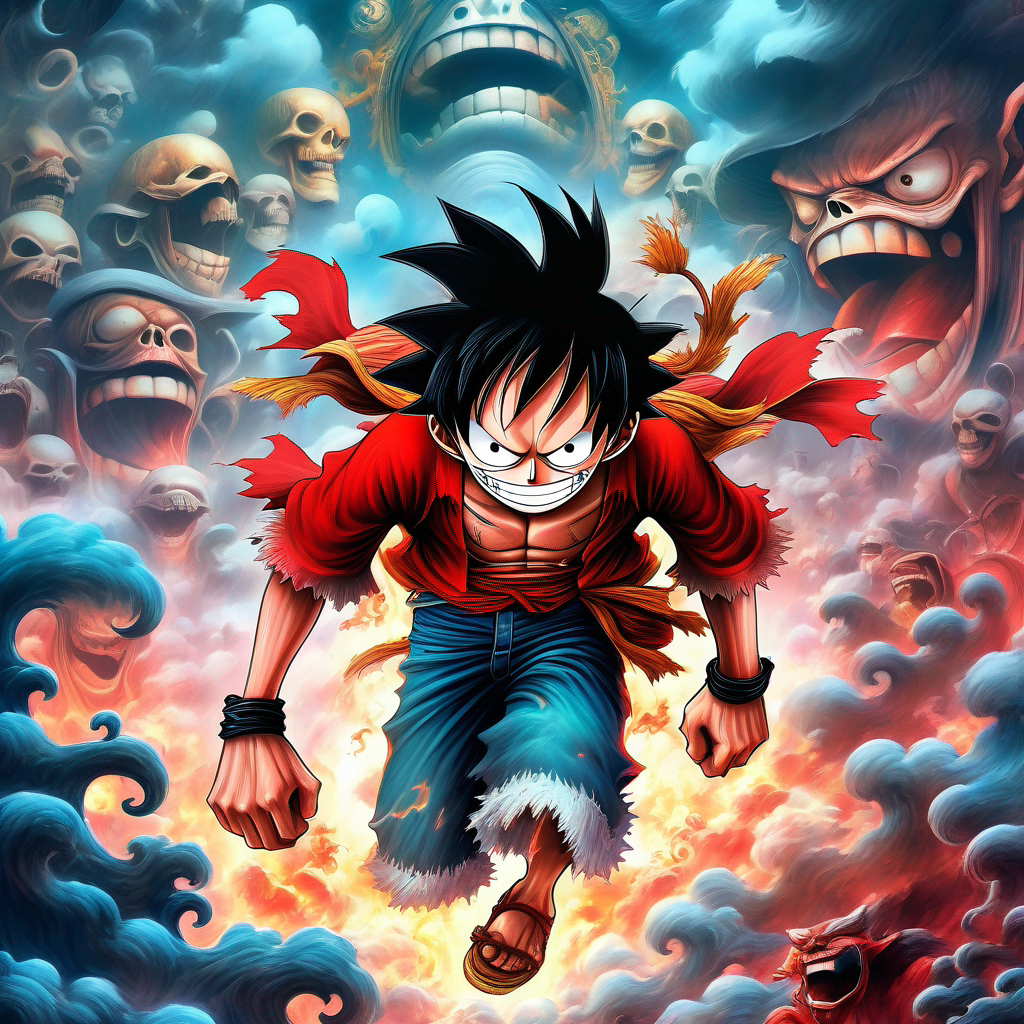
sdxl-niji-se

48
The sdxl-niji-se model is a variant of the SDXL (Stable Diffusion XL) text-to-image model developed by the Replicate creator lucataco. It is a specialized version of the SDXL model, known as the "Niji Special Edition", which aims to produce anime-themed images. The sdxl-niji-se model can be compared to other anime-focused models like animagine-xl-3.1 and more general-purpose SDXL models like dreamshaper-xl-turbo and pixart-xl-2. Model inputs and outputs The sdxl-niji-se model takes a text prompt as input and generates one or more images as output. The input prompt can describe a wide range of scenes and subjects, and the model will attempt to produce corresponding anime-style images. The outputs are high-resolution images that can be further refined or edited as needed. Inputs Prompt**: A text description of the desired image Seed**: An optional random seed value to control image randomness Width/Height**: The desired dimensions of the output image Scheduler**: The algorithm used to generate the image Num Outputs**: The number of images to generate Guidance Scale**: The strength of the text guidance during generation Apply Watermark**: Whether to apply a watermark to the generated image Negative Prompt**: Text to avoid or exclude from the generated image Outputs Image(s)**: One or more high-resolution images generated from the input prompt Capabilities The sdxl-niji-se model is capable of generating a wide variety of anime-themed images based on text prompts. It can create characters, scenes, and illustrations in a consistent anime art style. The model is particularly adept at producing dynamic, vibrant images with detailed characters and backgrounds. What can I use it for? The sdxl-niji-se model could be useful for a variety of creative projects, such as: Generating concept art or character designs for anime, manga, or other media Visualizing stories or narratives in an anime-inspired style Creating illustrations, backgrounds, or assets for games, animations, or other multimedia projects Experimentation and exploration of anime-themed imagery and aesthetics Companies or individuals working in the anime, manga, or animation industries may find the sdxl-niji-se model particularly useful for generating visual content and assets. Things to try Some interesting things to try with the sdxl-niji-se model include: Experimenting with different prompts to see the range of anime-style images the model can generate Combining the model with other tools or techniques, such as image editing or 3D rendering, to create more complex or refined outputs Exploring the model's capabilities for generating specific types of anime characters, scenes, or narratives Comparing the sdxl-niji-se model to other anime-focused text-to-image models to understand its unique strengths and characteristics By engaging with the sdxl-niji-se model in creative and thoughtful ways, users can unlock new possibilities for their anime-inspired projects and push the boundaries of what is possible with this powerful text-to-image technology.
Updated Invalid Date