Sepal
Models by this creator
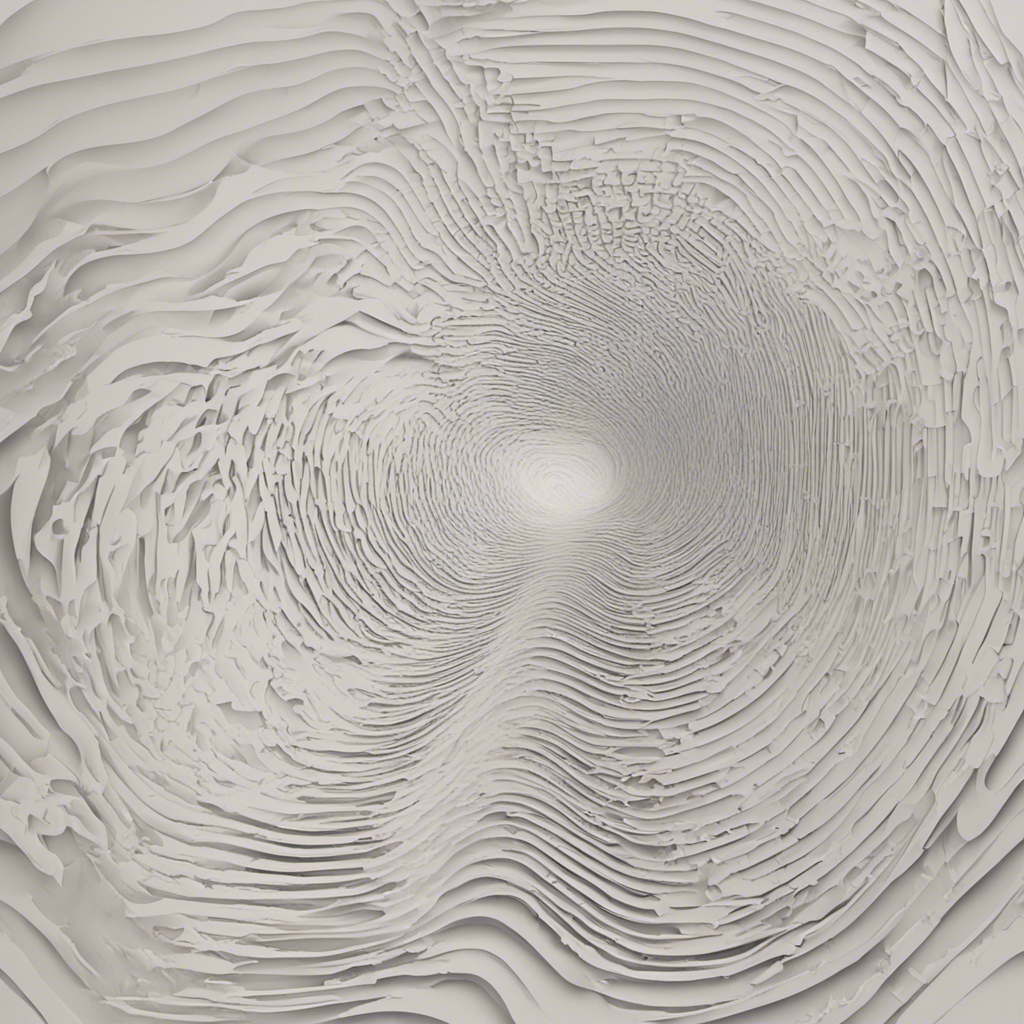
audiogen

42
audiogen is a model developed by Sepal that can generate sounds from text prompts. It is similar to other audio-related models like musicgen from Meta, which generates music from prompts, and styletts2 from Adirik, which generates speech from text. audiogen can be used to create a wide variety of sounds, from ambient noise to sound effects, based on the text prompt provided. Model inputs and outputs audiogen takes a text prompt as the main input, along with several optional parameters to control the output, such as duration, temperature, and output format. The model then generates an audio file in the specified format that represents the sounds described by the prompt. Inputs Prompt**: A text description of the sounds to be generated Duration**: The maximum duration of the generated audio (in seconds) Temperature**: Controls the "conservativeness" of the sampling process, with higher values producing more diverse outputs Classifier Free Guidance**: Increases the influence of the input prompt on the output Output Format**: The desired output format for the generated audio (e.g., WAV) Outputs Audio File**: The generated audio file in the specified format Capabilities audiogen can create a wide range of sounds based on text prompts, from simple ambient noise to more complex sound effects. For example, you could use it to generate the sound of a babbling brook, a thunderstorm, or even the roar of a lion. The model's ability to generate diverse and realistic-sounding audio makes it a useful tool for tasks like audio production, sound design, and even voice user interface development. What can I use it for? audiogen could be used in a variety of projects that require audio generation, such as video game sound effects, podcast or audiobook background music, or even sound design for augmented reality or virtual reality applications. The model's versatility and ease of use make it a valuable tool for creators and developers working in these and other audio-related fields. Things to try One interesting aspect of audiogen is its ability to generate sounds that are both realistic and evocative. By crafting prompts that tap into specific emotions or sensations, users can explore the model's potential to create immersive audio experiences. For example, you could try generating the sound of a cozy fireplace or the peaceful ambiance of a forest, and then incorporate these sounds into a multimedia project or relaxation app.
Updated 7/4/2024
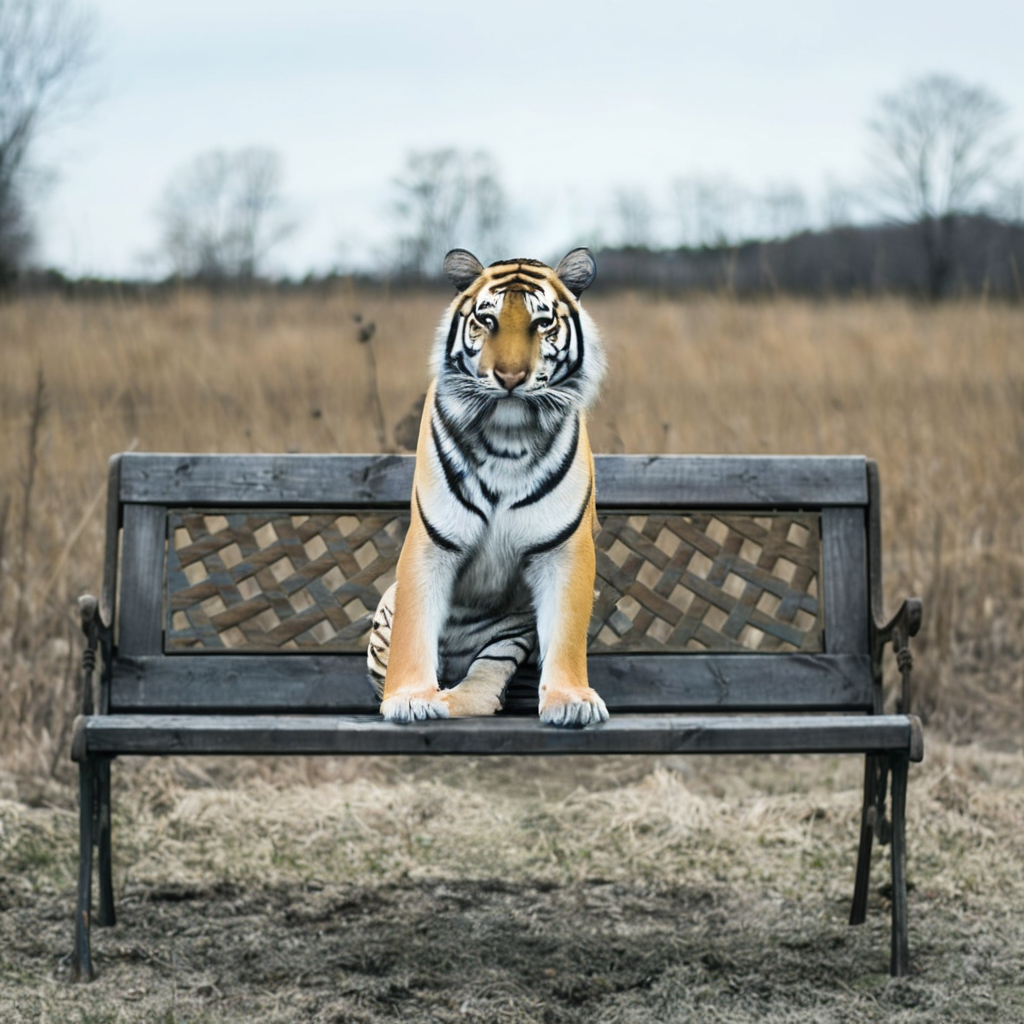
sdxl-inpainting

3
The sdxl-inpainting model is a version of Stable Diffusion XL that has been specifically trained on the task of inpainting. Developed by sepal, it is based on the Stable Diffusion XL model from Hugging Face. This model excels at filling in masked or missing parts of images, allowing for creative image editing and manipulation. Similar models include the sdxl-inpainting model by lucataco, the stable-diffusion-inpainting model by Stability AI, the inpainting-xl model by ikun-ai, and the sdxl-ad-inpaint model by catacolabs. Model inputs and outputs The sdxl-inpainting model takes in a variety of inputs to generate its output: Inputs Prompt**: The text prompt that describes the desired image. This can be anything from a simple description to a more complex, creative prompt. Negative Prompt**: An optional text prompt that describes what the model should not generate. Image**: An input image that the model will use as a starting point for the inpainting task. Mask**: A mask image that specifies which parts of the input image should be inpainted. Seed**: An optional random seed value to control the stochastic nature of the image generation. Guidance Scale**: A value that controls the strength of the text prompt on the generated image. Prompt Strength**: A value that controls the balance between the input image and the text prompt. Num Inference Steps**: The number of denoising steps the model will take to generate the output image. Outputs The model outputs a single image that has been inpainted based on the input prompt, image, and mask. Capabilities The sdxl-inpainting model excels at filling in missing or damaged parts of images based on a text prompt. For example, you could provide an image of a landscape and a prompt like "A majestic castle in the foreground", and the model would generate a new version of the image with a castle added. What can I use it for? The sdxl-inpainting model can be used for a variety of creative and practical applications. For example, you could use it to: Edit existing images by filling in missing or damaged areas Create new images by combining an existing image with a text prompt Experiment with different prompts and masks to see what the model can generate Incorporate the model into creative tools or applications Things to try One interesting thing to try with the sdxl-inpainting model is to use it to generate images with varying levels of detail or realism. By adjusting the Guidance Scale and Prompt Strength, you can create images that range from photorealistic to more abstract and stylized. You could also try combining the model with other image manipulation tools to create even more complex and unique outputs.
Updated 7/4/2024