audiogen
Maintainer: sepal

49
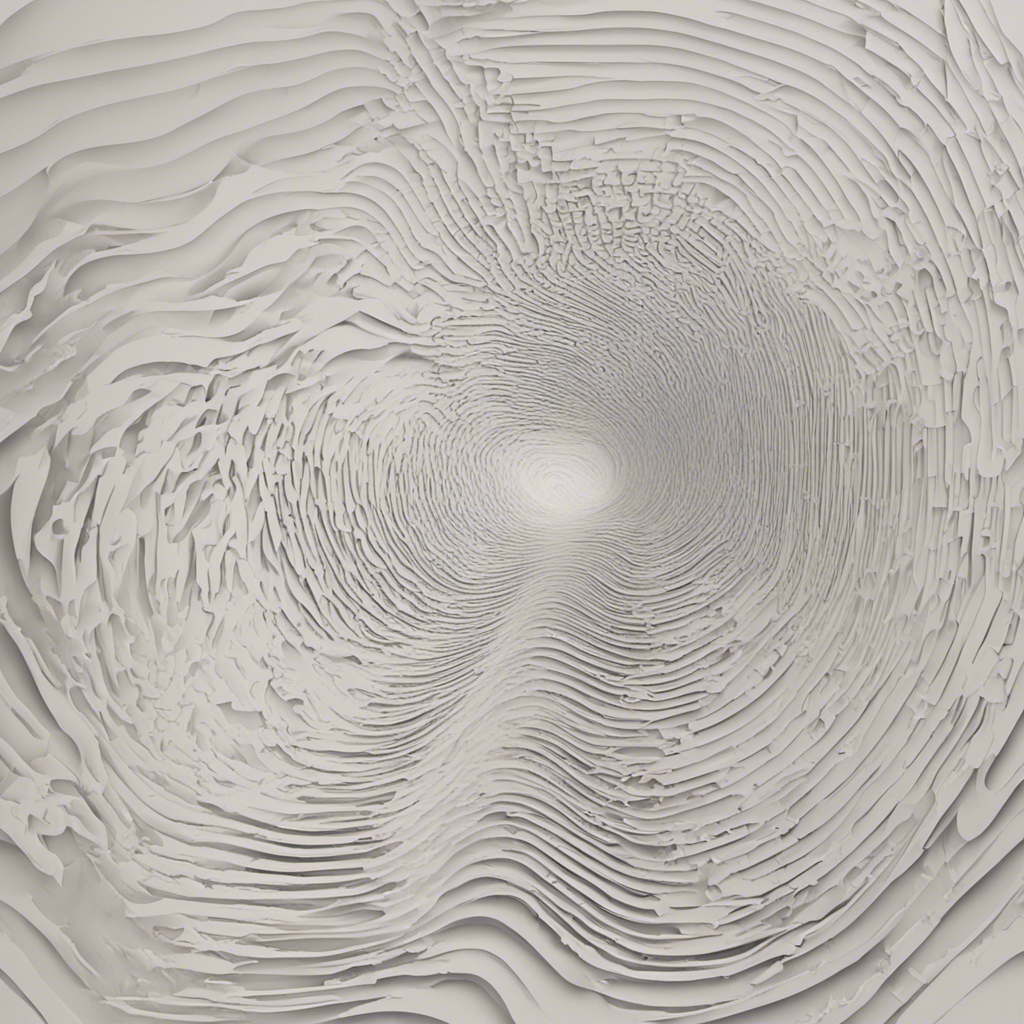
Property | Value |
---|---|
Run this model | Run on Replicate |
API spec | View on Replicate |
Github link | View on Github |
Paper link | View on Arxiv |
Create account to get full access
Model overview
audiogen
is a model developed by Sepal that can generate sounds from text prompts. It is similar to other audio-related models like musicgen from Meta, which generates music from prompts, and styletts2 from Adirik, which generates speech from text. audiogen
can be used to create a wide variety of sounds, from ambient noise to sound effects, based on the text prompt provided.
Model inputs and outputs
audiogen
takes a text prompt as the main input, along with several optional parameters to control the output, such as duration, temperature, and output format. The model then generates an audio file in the specified format that represents the sounds described by the prompt.
Inputs
- Prompt: A text description of the sounds to be generated
- Duration: The maximum duration of the generated audio (in seconds)
- Temperature: Controls the "conservativeness" of the sampling process, with higher values producing more diverse outputs
- Classifier Free Guidance: Increases the influence of the input prompt on the output
- Output Format: The desired output format for the generated audio (e.g., WAV)
Outputs
- Audio File: The generated audio file in the specified format
Capabilities
audiogen
can create a wide range of sounds based on text prompts, from simple ambient noise to more complex sound effects. For example, you could use it to generate the sound of a babbling brook, a thunderstorm, or even the roar of a lion. The model's ability to generate diverse and realistic-sounding audio makes it a useful tool for tasks like audio production, sound design, and even voice user interface development.
What can I use it for?
audiogen
could be used in a variety of projects that require audio generation, such as video game sound effects, podcast or audiobook background music, or even sound design for augmented reality or virtual reality applications. The model's versatility and ease of use make it a valuable tool for creators and developers working in these and other audio-related fields.
Things to try
One interesting aspect of audiogen
is its ability to generate sounds that are both realistic and evocative. By crafting prompts that tap into specific emotions or sensations, users can explore the model's potential to create immersive audio experiences. For example, you could try generating the sound of a cozy fireplace or the peaceful ambiance of a forest, and then incorporate these sounds into a multimedia project or relaxation app.
This summary was produced with help from an AI and may contain inaccuracies - check out the links to read the original source documents!
Related Models
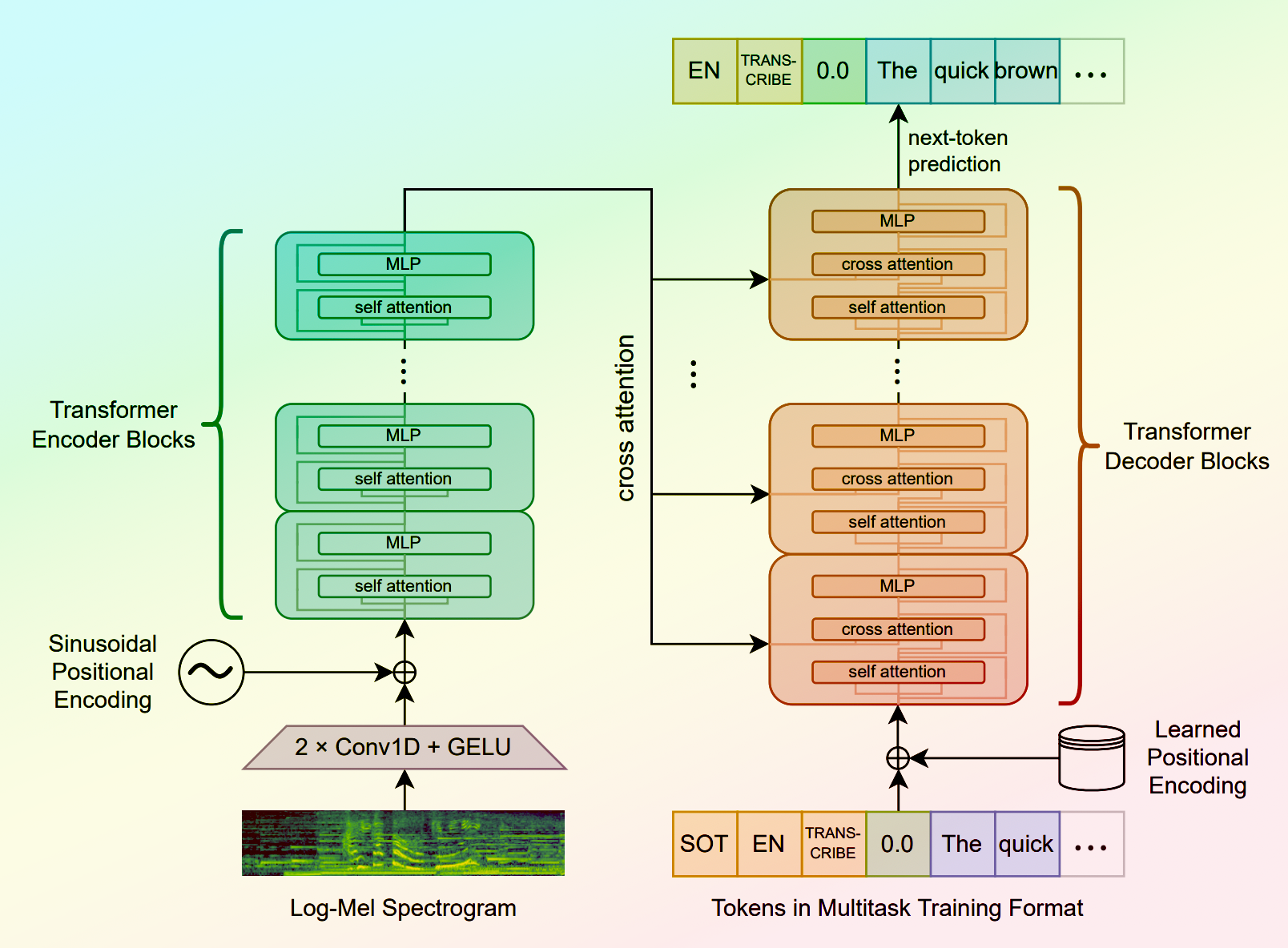
whisper

34.6K
Whisper is a general-purpose speech recognition model developed by OpenAI. It is capable of converting speech in audio to text, with the ability to translate the text to English if desired. Whisper is based on a large Transformer model trained on a diverse dataset of multilingual and multitask speech recognition data. This allows the model to handle a wide range of accents, background noises, and languages. Similar models like whisper-large-v3, incredibly-fast-whisper, and whisper-diarization offer various optimizations and additional features built on top of the core Whisper model. Model inputs and outputs Whisper takes an audio file as input and outputs a text transcription. The model can also translate the transcription to English if desired. The input audio can be in various formats, and the model supports a range of parameters to fine-tune the transcription, such as temperature, patience, and language. Inputs Audio**: The audio file to be transcribed Model**: The specific version of the Whisper model to use, currently only large-v3 is supported Language**: The language spoken in the audio, or None to perform language detection Translate**: A boolean flag to translate the transcription to English Transcription**: The format for the transcription output, such as "plain text" Initial Prompt**: An optional initial text prompt to provide to the model Suppress Tokens**: A list of token IDs to suppress during sampling Logprob Threshold**: The minimum average log probability threshold for a successful transcription No Speech Threshold**: The threshold for considering a segment as silence Condition on Previous Text**: Whether to provide the previous output as a prompt for the next window Compression Ratio Threshold**: The maximum compression ratio threshold for a successful transcription Temperature Increment on Fallback**: The temperature increase when the decoding fails to meet the specified thresholds Outputs Transcription**: The text transcription of the input audio Language**: The detected language of the audio (if language input is None) Tokens**: The token IDs corresponding to the transcription Timestamp**: The start and end timestamps for each word in the transcription Confidence**: The confidence score for each word in the transcription Capabilities Whisper is a powerful speech recognition model that can handle a wide range of accents, background noises, and languages. The model is capable of accurately transcribing audio and optionally translating the transcription to English. This makes Whisper useful for a variety of applications, such as real-time captioning, meeting transcription, and audio-to-text conversion. What can I use it for? Whisper can be used in various applications that require speech-to-text conversion, such as: Captioning and Subtitling**: Automatically generate captions or subtitles for videos, improving accessibility for viewers. Meeting Transcription**: Transcribe audio recordings of meetings, interviews, or conferences for easy review and sharing. Podcast Transcription**: Convert audio podcasts to text, making the content more searchable and accessible. Language Translation**: Transcribe audio in one language and translate the text to another, enabling cross-language communication. Voice Interfaces**: Integrate Whisper into voice-controlled applications, such as virtual assistants or smart home devices. Things to try One interesting aspect of Whisper is its ability to handle a wide range of languages and accents. You can experiment with the model's performance on audio samples in different languages or with various background noises to see how it handles different real-world scenarios. Additionally, you can explore the impact of the different input parameters, such as temperature, patience, and language detection, on the transcription quality and accuracy.
Updated Invalid Date
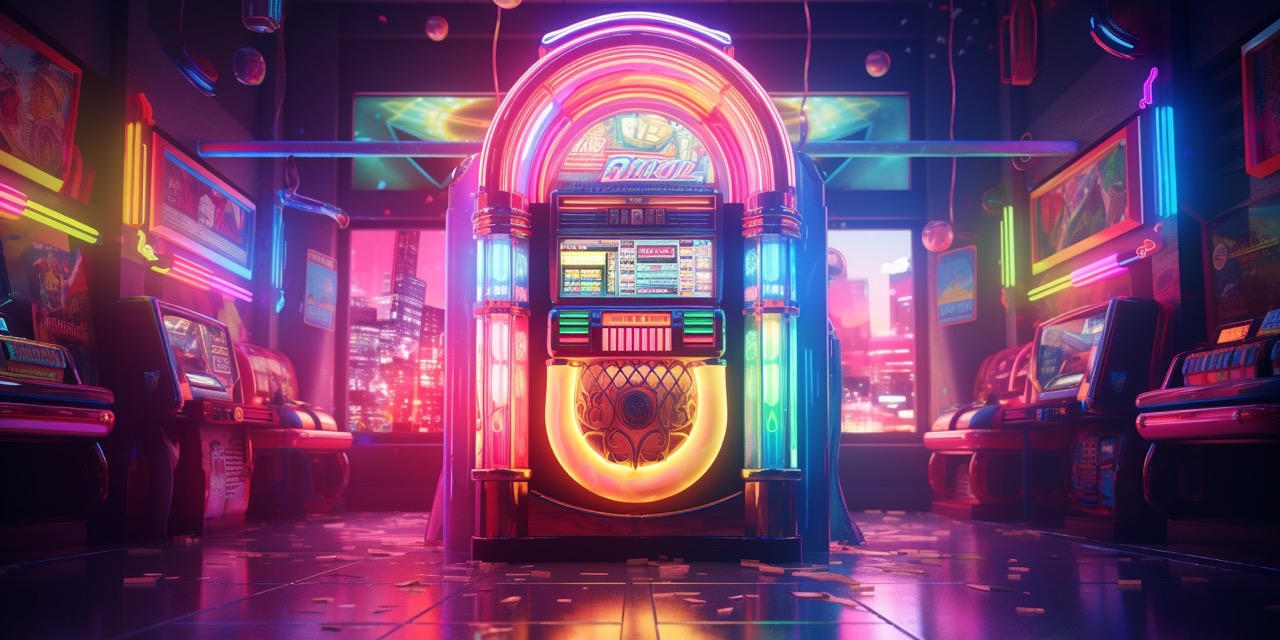
musicgen

2.0K
musicgen is a simple and controllable model for music generation developed by Meta. Unlike existing methods like MusicLM, musicgen doesn't require a self-supervised semantic representation and generates all 4 codebooks in one pass. By introducing a small delay between the codebooks, the authors show they can predict them in parallel, thus having only 50 auto-regressive steps per second of audio. musicgen was trained on 20K hours of licensed music, including an internal dataset of 10K high-quality music tracks and music data from ShutterStock and Pond5. Model inputs and outputs musicgen takes in a text prompt or melody and generates corresponding music. The model's inputs include a description of the desired music, an optional input audio file to influence the generated output, and various parameters to control the generation process like temperature, top-k, and top-p sampling. The output is a generated audio file in WAV format. Inputs Prompt**: A description of the music you want to generate. Input Audio**: An optional audio file that will influence the generated music. If "continuation" is set to true, the generated music will be a continuation of the input audio. Otherwise, it will mimic the input audio's melody. Duration**: The duration of the generated audio in seconds. Continuation Start/End**: The start and end times of the input audio to use for continuation. Various generation parameters**: Settings like temperature, top-k, top-p, etc. to control the diversity and quality of the generated output. Outputs Generated Audio**: A WAV file containing the generated music. Capabilities musicgen can generate a wide variety of music styles and genres based on the provided text prompt. For example, you could ask it to generate "tense, staccato strings with plucked dissonant strings, like a scary movie soundtrack" and it would produce corresponding music. The model can also continue or mimic the melody of an input audio file, allowing for more coherent and controlled music generation. What can I use it for? musicgen could be used for a variety of applications, such as: Background music generation**: Automatically generating custom music for videos, games, or other multimedia projects. Music composition assistance**: Helping musicians and composers come up with new musical ideas or sketches to build upon. Audio creation for content creators**: Allowing YouTubers, podcasters, and other content creators to easily add custom music to their projects. Things to try One interesting aspect of musicgen is its ability to generate music in parallel by predicting the different codebook components separately. This allows for faster generation compared to previous autoregressive music models. You could try experimenting with different generation parameters to find the right balance between generation speed, diversity, and quality for your use case. Additionally, the model's ability to continue or mimic input audio opens up possibilities for interactive music creation workflows, where users could iterate on an initial seed melody or prompt to refine the generated output.
Updated Invalid Date
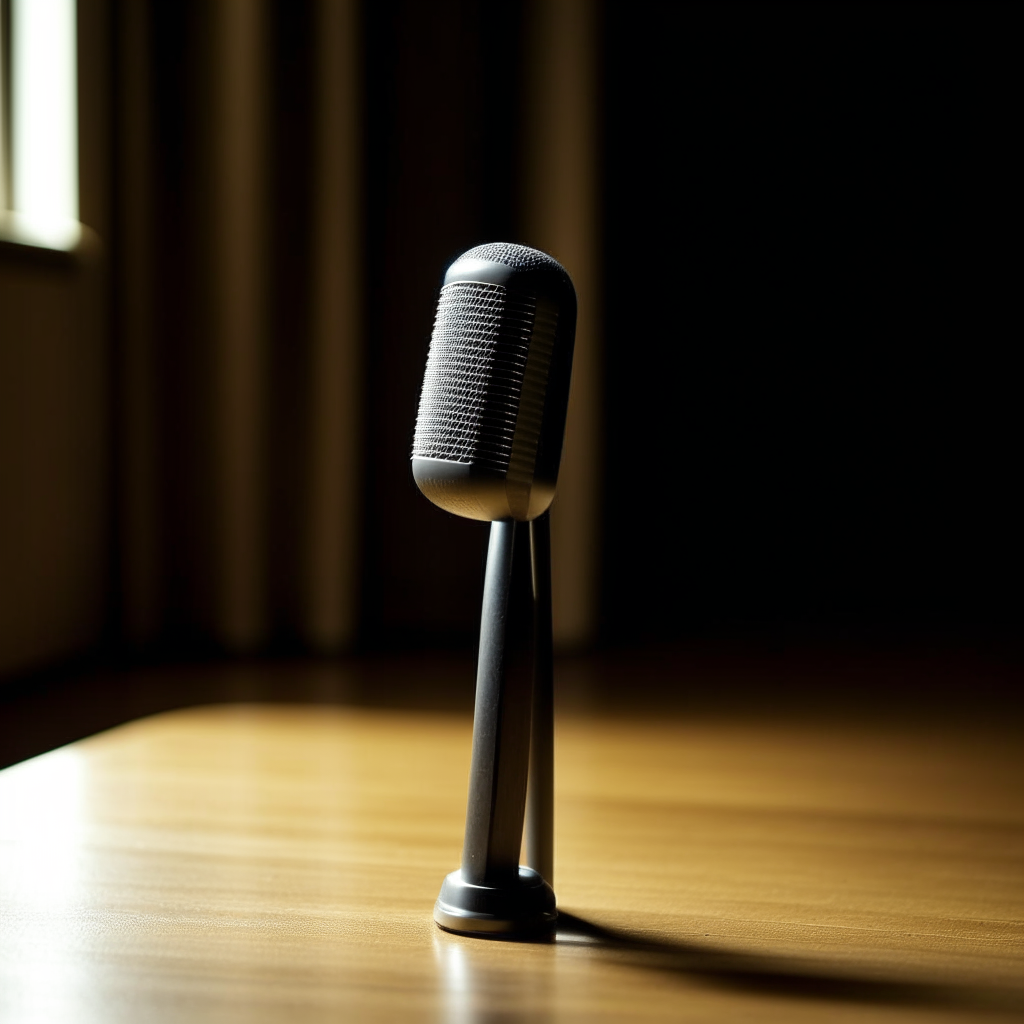
styletts2

4.2K
styletts2 is a text-to-speech (TTS) model developed by Yinghao Aaron Li, Cong Han, Vinay S. Raghavan, Gavin Mischler, and Nima Mesgarani. It leverages style diffusion and adversarial training with large speech language models (SLMs) to achieve human-level TTS synthesis. Unlike its predecessor, styletts2 models styles as a latent random variable through diffusion models, allowing it to generate the most suitable style for the text without requiring reference speech. It also employs large pre-trained SLMs, such as WavLM, as discriminators with a novel differentiable duration modeling for end-to-end training, resulting in improved speech naturalness. Model inputs and outputs styletts2 takes in text and generates high-quality speech audio. The model inputs and outputs are as follows: Inputs Text**: The text to be converted to speech. Beta**: A parameter that determines the prosody of the generated speech, with lower values sampling style based on previous or reference speech and higher values sampling more from the text. Alpha**: A parameter that determines the timbre of the generated speech, with lower values sampling style based on previous or reference speech and higher values sampling more from the text. Reference**: An optional reference speech audio to copy the style from. Diffusion Steps**: The number of diffusion steps to use in the generation process, with higher values resulting in better quality but longer generation time. Embedding Scale**: A scaling factor for the text embedding, which can be used to produce more pronounced emotion in the generated speech. Outputs Audio**: The generated speech audio in the form of a URI. Capabilities styletts2 is capable of generating human-level TTS synthesis on both single-speaker and multi-speaker datasets. It surpasses human recordings on the LJSpeech dataset and matches human performance on the VCTK dataset. When trained on the LibriTTS dataset, styletts2 also outperforms previous publicly available models for zero-shot speaker adaptation. What can I use it for? styletts2 can be used for a variety of applications that require high-quality text-to-speech generation, such as audiobook production, voice assistants, language learning tools, and more. The ability to control the prosody and timbre of the generated speech, as well as the option to use reference audio, makes styletts2 a versatile tool for creating personalized and expressive speech output. Things to try One interesting aspect of styletts2 is its ability to perform zero-shot speaker adaptation on the LibriTTS dataset. This means that the model can generate speech in the style of speakers it has not been explicitly trained on, by leveraging the diverse speech synthesis offered by the diffusion model. Developers could explore the limits of this zero-shot adaptation and experiment with fine-tuning the model on new speakers to further improve the quality and diversity of the generated speech.
Updated Invalid Date
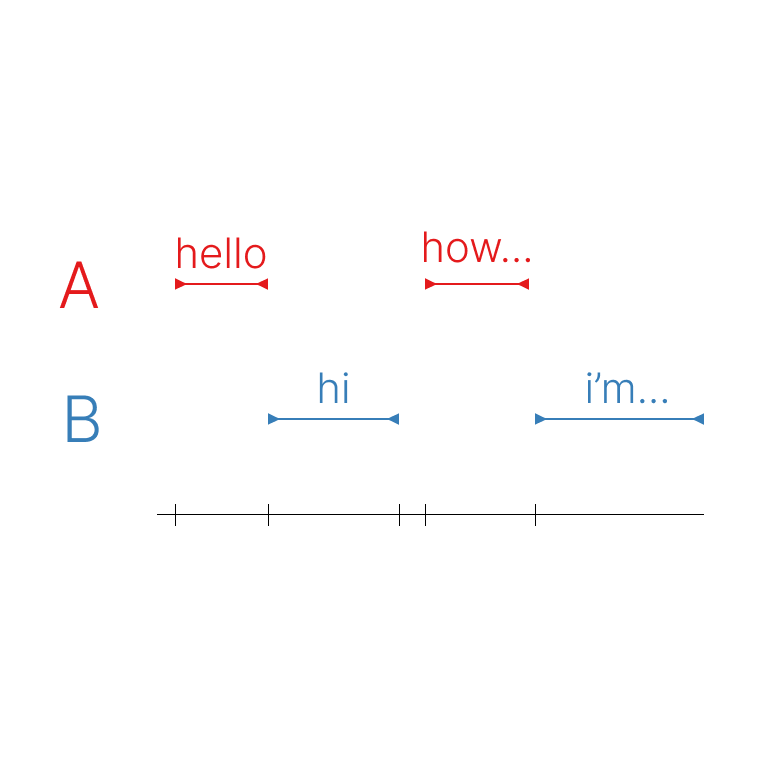
speaker-transcription

22
The speaker-transcription model is a powerful AI system that combines speaker diarization and speech transcription capabilities. It was developed by Meronym, a creator on the Replicate platform. This model builds upon two main components: the pyannote.audio speaker diarization pipeline and OpenAI's whisper model for general-purpose English speech transcription. The speaker-transcription model outperforms similar models like whisper-diarization and whisperx by providing more accurate speaker segmentation and identification, as well as high-quality transcription. It can be particularly useful for tasks that require both speaker information and verbatim transcripts, such as interview analysis, podcast processing, or meeting recordings. Model inputs and outputs The speaker-transcription model takes an audio file as input and can optionally accept a prompt string to guide the transcription. The model outputs a JSON file containing the transcribed segments, with each segment associated with a speaker label and timestamps. Inputs Audio**: An audio file in a supported format, such as MP3, AAC, FLAC, OGG, OPUS, or WAV. Prompt (optional)**: A text prompt that can be used to provide additional context for the transcription. Outputs JSON file**: A JSON file with the following structure: segments: A list of transcribed segments, each with a speaker label, start and stop timestamps, and the segment transcript. speakers: Information about the detected speakers, including the total count, labels for each speaker, and embeddings (a vector representation of each speaker's voice). Capabilities The speaker-transcription model excels at accurately identifying and labeling different speakers within an audio recording, while also providing high-quality transcripts of the spoken content. This makes it a valuable tool for a variety of applications, such as interview analysis, podcast processing, or meeting recordings. What can I use it for? The speaker-transcription model can be used for data augmentation and segmentation tasks, where the speaker information and timestamps can be used to improve the accuracy and effectiveness of transcription and captioning models. Additionally, the speaker embeddings generated by the model can be used for speaker recognition, allowing you to match voice profiles against a database of known speakers. Things to try One interesting aspect of the speaker-transcription model is the ability to use a prompt to guide the transcription. By providing additional context about the topic or subject matter, you can potentially improve the accuracy and relevance of the transcripts. Try experimenting with different prompts to see how they affect the output. Another useful feature is the generation of speaker embeddings, which can be used for speaker recognition and identification tasks. Consider exploring ways to leverage these embeddings, such as building a speaker verification system or clustering speakers in large audio datasets.
Updated Invalid Date