flux-dev-de-distill
Maintainer: nyanko7

77
🏅
Property | Value |
---|---|
Run this model | Run on HuggingFace |
API spec | View on HuggingFace |
Github link | No Github link provided |
Paper link | No paper link provided |
Create account to get full access
Model overview
flux-dev-de-distill
is an experiment by maintainer nyanko7 to "de-distill" guidance from the flux.1-dev
model. The model was trained to remove the original distilled guidance and create true classifier-free guidance reworks. This model is not compatible with the diffusers pipeline, so users will need to use the provided inference script or manually add guidance during the iteration loop.
The model was trained on 150K Unsplash images for 6K steps with a global batch size of 32, using a frozen teacher model. Examples show the model producing improved results compared to the distilled CFG approach.
Similar models include the SDXL-Lightning model from ByteDance, which is a fast text-to-image model, and the CLIP-Guided Diffusion model from afiaka87, which generates images from text by guiding a denoising diffusion model.
Model inputs and outputs
Inputs
- Text prompts to describe the desired image
Outputs
- Generated images based on the input text prompt
Capabilities
The flux-dev-de-distill
model is capable of generating high-quality images from text prompts, improving upon the distilled CFG approach used in the original flux.1-dev
model. The model was trained to produce true classifier-free guidance, which can lead to enhanced prompt following and more coherent outputs.
What can I use it for?
The flux-dev-de-distill
model is intended for research and creative applications, such as generating artwork, designing visuals, and exploring the potential of text-to-image diffusion models. While the model is open-source, the maintainer has specified a non-commercial license that restricts certain use cases.
Things to try
One interesting aspect of the flux-dev-de-distill
model is its use of true classifier-free guidance, which aims to improve upon the distilled CFG approach. Users could experiment with different prompts and compare the outputs to the original flux.1-dev
model to see how the de-distillation process affects the model's performance and coherence.
This summary was produced with help from an AI and may contain inaccuracies - check out the links to read the original source documents!
Related Models
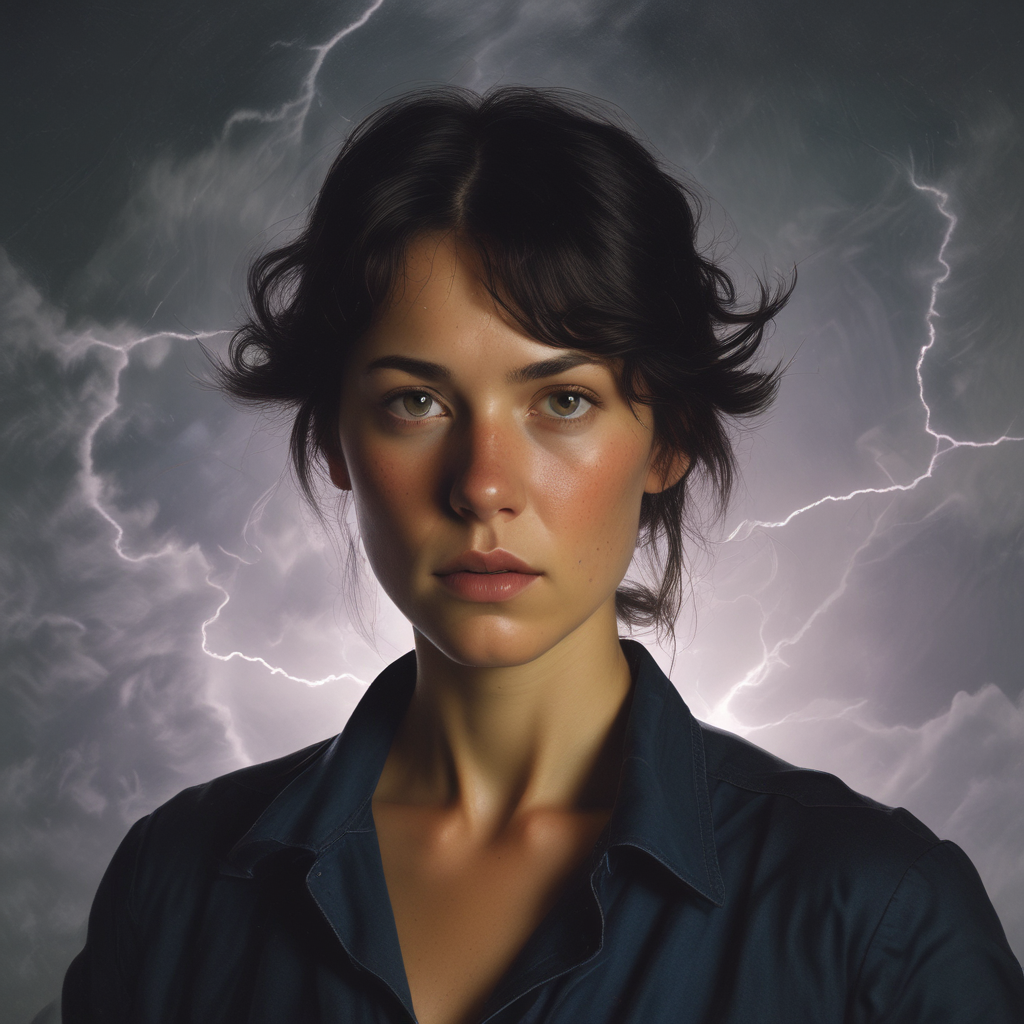
sdxl-lightning-4step

453.2K
sdxl-lightning-4step is a fast text-to-image model developed by ByteDance that can generate high-quality images in just 4 steps. It is similar to other fast diffusion models like AnimateDiff-Lightning and Instant-ID MultiControlNet, which also aim to speed up the image generation process. Unlike the original Stable Diffusion model, these fast models sacrifice some flexibility and control to achieve faster generation times. Model inputs and outputs The sdxl-lightning-4step model takes in a text prompt and various parameters to control the output image, such as the width, height, number of images, and guidance scale. The model can output up to 4 images at a time, with a recommended image size of 1024x1024 or 1280x1280 pixels. Inputs Prompt**: The text prompt describing the desired image Negative prompt**: A prompt that describes what the model should not generate Width**: The width of the output image Height**: The height of the output image Num outputs**: The number of images to generate (up to 4) Scheduler**: The algorithm used to sample the latent space Guidance scale**: The scale for classifier-free guidance, which controls the trade-off between fidelity to the prompt and sample diversity Num inference steps**: The number of denoising steps, with 4 recommended for best results Seed**: A random seed to control the output image Outputs Image(s)**: One or more images generated based on the input prompt and parameters Capabilities The sdxl-lightning-4step model is capable of generating a wide variety of images based on text prompts, from realistic scenes to imaginative and creative compositions. The model's 4-step generation process allows it to produce high-quality results quickly, making it suitable for applications that require fast image generation. What can I use it for? The sdxl-lightning-4step model could be useful for applications that need to generate images in real-time, such as video game asset generation, interactive storytelling, or augmented reality experiences. Businesses could also use the model to quickly generate product visualization, marketing imagery, or custom artwork based on client prompts. Creatives may find the model helpful for ideation, concept development, or rapid prototyping. Things to try One interesting thing to try with the sdxl-lightning-4step model is to experiment with the guidance scale parameter. By adjusting the guidance scale, you can control the balance between fidelity to the prompt and diversity of the output. Lower guidance scales may result in more unexpected and imaginative images, while higher scales will produce outputs that are closer to the specified prompt.
Updated Invalid Date
🌀
distil-medium.en

109
The distil-medium.en model is a distilled version of the Whisper medium.en model proposed in the paper Robust Knowledge Distillation via Large-Scale Pseudo Labelling. It is 6 times faster, 49% smaller, and performs within 1% word error rate (WER) on out-of-distribution evaluation sets compared to the original Whisper medium.en model. This makes it an efficient alternative for English speech recognition tasks. The model is part of the Distil-Whisper repository, which contains several distilled variants of the Whisper model. The distil-large-v2 model is another example, which surpasses the performance of the original Whisper large-v2 model. Model inputs and outputs Inputs Audio data**: The model takes audio data as input, in the form of log-Mel spectrograms. Outputs Transcription text**: The model outputs transcribed text in the same language as the input audio. Capabilities The distil-medium.en model demonstrates strong performance on English speech recognition tasks, achieving a short-form WER of 11.1% and a long-form WER of 12.4% on out-of-distribution evaluation sets. It is significantly more efficient than the original Whisper medium.en model, running 6.8 times faster with 49% fewer parameters. What can I use it for? The distil-medium.en model is well-suited for a variety of English speech recognition applications, such as transcribing audio recordings, live captioning, and voice-to-text conversion. Its efficiency makes it a practical choice for real-world deployment, particularly in scenarios where latency and model size are important considerations. Things to try You can use the distil-medium.en model with the Hugging Face Transformers library to perform short-form transcription of audio samples. The model can also be used for long-form transcription by leveraging the chunking capabilities of the pipeline class, allowing it to handle audio files of arbitrary length. Additionally, the Distil-Whisper repository provides training code that you can use to distill the Whisper model on other languages, expanding the model's capabilities beyond English. If you're interested in distilling Whisper for your language, be sure to check out the training code.
Updated Invalid Date
🔎
distilbert-base-uncased-go-emotions-student

64
The distilbert-base-uncased-go-emotions-student model is a distilled version of a zero-shot classification pipeline trained on the unlabeled GoEmotions dataset. The maintainer explains that this model was trained with mixed precision for 10 epochs using a script for distilling an NLI-based zero-shot model into a more efficient student model. While the original GoEmotions dataset allows for multi-label classification, the teacher model used single-label classification to create pseudo-labels for the student. Similar models include distilbert-base-multilingual-cased-sentiments-student, which was distilled from a zero-shot classification pipeline on the Multilingual Sentiment dataset, and roberta-base-go_emotions, a model trained directly on the GoEmotions dataset. Model Inputs and Outputs Inputs Text**: The model takes text input, such as a sentence or short paragraph. Outputs Emotion Labels**: The model outputs a list of predicted emotion labels and their corresponding scores. The model predicts the probability of the input text expressing emotions like anger, disgust, fear, joy, sadness, and surprise. Capabilities The distilbert-base-uncased-go-emotions-student model can be used for zero-shot emotion classification on text data. While it may not perform as well as a fully supervised model, it can provide a quick and efficient way to gauge the emotional tone of text without the need for labeled training data. What Can I Use It For? This model could be useful for a variety of text-based applications, such as: Analyzing customer feedback or social media posts to understand the emotional sentiment expressed Categorizing movie or book reviews based on the emotions they convey Monitoring online discussions or forums for signs of emotional distress or conflict Things to Try One interesting aspect of this model is that it was distilled from a zero-shot classification pipeline. This means the model was trained without any labeled data, relying instead on pseudo-labels generated by a teacher model. It would be interesting to experiment with different approaches to distillation or to explore how the performance of this student model compares to a fully supervised model trained directly on the GoEmotions dataset. Verifying all URLs: All URLs provided in the links are contained within the prompt.
Updated Invalid Date
🏋️
FLUX.1-dev

3.5K
The FLUX.1 [dev] is a 12 billion parameter rectified flow transformer developed by black-forest-labs that can generate images from text descriptions. It is part of the FLUX.1 model family, which includes the state-of-the-art FLUX.1 [pro] model as well as the efficient FLUX.1 [schnell] and the base flux-dev and flux-pro models. These models offer cutting-edge output quality, competitive prompt following, and various training approaches like guidance distillation and latent adversarial diffusion distillation. Model inputs and outputs The FLUX.1 [dev] model takes text prompts as input and generates corresponding images as output. The text prompts can describe a wide range of subjects, and the model is able to produce high-quality, diverse images that match the input descriptions. Inputs Text prompt**: A textual description of the desired image Outputs Generated image**: An image generated by the model based on the input text prompt Capabilities The FLUX.1 [dev] model is capable of generating visually compelling images from text descriptions. It matches the performance of closed-source alternatives in terms of output quality and prompt following, making it a powerful tool for artists, designers, and researchers. The model's open weights also allow for further scientific exploration and the development of innovative workflows. What can I use it for? The FLUX.1 [dev] model can be used for a variety of applications, such as: Personal creative projects**: Generate unique images to use in art, design, or other creative endeavors. Scientific research**: Experiment with the model's capabilities and contribute to the advancement of AI-powered image generation. Commercial applications**: Incorporate the model into various products and services, as permitted by the flux-1-dev-non-commercial-license. Things to try One interesting aspect of the FLUX.1 [dev] model is its ability to generate outputs that can be used for various purposes, as long as they comply with the specified limitations and out-of-scope uses. Experiment with different types of prompts to see the model's versatility and explore its potential applications.
Updated Invalid Date