Papercut_SDXL
Maintainer: TheLastBen

50
🔮
Property | Value |
---|---|
Run this model | Run on HuggingFace |
API spec | View on HuggingFace |
Github link | No Github link provided |
Paper link | No paper link provided |
Create account to get full access
Model overview
Papercut_SDXL
is a text-to-image AI model developed by TheLastBen. It is trained using the fast-stable-diffusion SDXL trainer, which aims to create images with a distinct "papercut" style.
Model inputs and outputs
Papercut_SDXL
takes text prompts as input and generates corresponding images. The model can produce a variety of scenes and subjects, as demonstrated by the sample images provided.
Inputs
- Text prompts that start with "papercut -subject/scene-"
Outputs
- Images with a unique "papercut" visual style
Capabilities
Papercut_SDXL
can generate images with a distinctive papercut aesthetic. The model is capable of producing a range of scenes and subjects, from abstract compositions to more realistic depictions.
What can I use it for?
The Papercut_SDXL
model could be useful for creating unique, stylized images for a variety of applications, such as art, design, or content creation. Its distinctive visual style could make it a valuable tool for those seeking to incorporate a distinctive, handcrafted look into their projects.
Things to try
Experiment with different text prompts to see the range of images the Papercut_SDXL
model can produce. Try combining the model with other text-to-image systems or post-processing techniques to further refine the output.
This summary was produced with help from an AI and may contain inaccuracies - check out the links to read the original source documents!
Related Models
🔮
Papercut_SDXL

50
Papercut_SDXL is a text-to-image AI model developed by TheLastBen. It is trained using the fast-stable-diffusion SDXL trainer, which aims to create images with a distinct "papercut" style. Model inputs and outputs Papercut_SDXL takes text prompts as input and generates corresponding images. The model can produce a variety of scenes and subjects, as demonstrated by the sample images provided. Inputs Text prompts that start with "papercut -subject/scene-" Outputs Images with a unique "papercut" visual style Capabilities Papercut_SDXL can generate images with a distinctive papercut aesthetic. The model is capable of producing a range of scenes and subjects, from abstract compositions to more realistic depictions. What can I use it for? The Papercut_SDXL model could be useful for creating unique, stylized images for a variety of applications, such as art, design, or content creation. Its distinctive visual style could make it a valuable tool for those seeking to incorporate a distinctive, handcrafted look into their projects. Things to try Experiment with different text prompts to see the range of images the Papercut_SDXL model can produce. Try combining the model with other text-to-image systems or post-processing techniques to further refine the output.
Updated Invalid Date
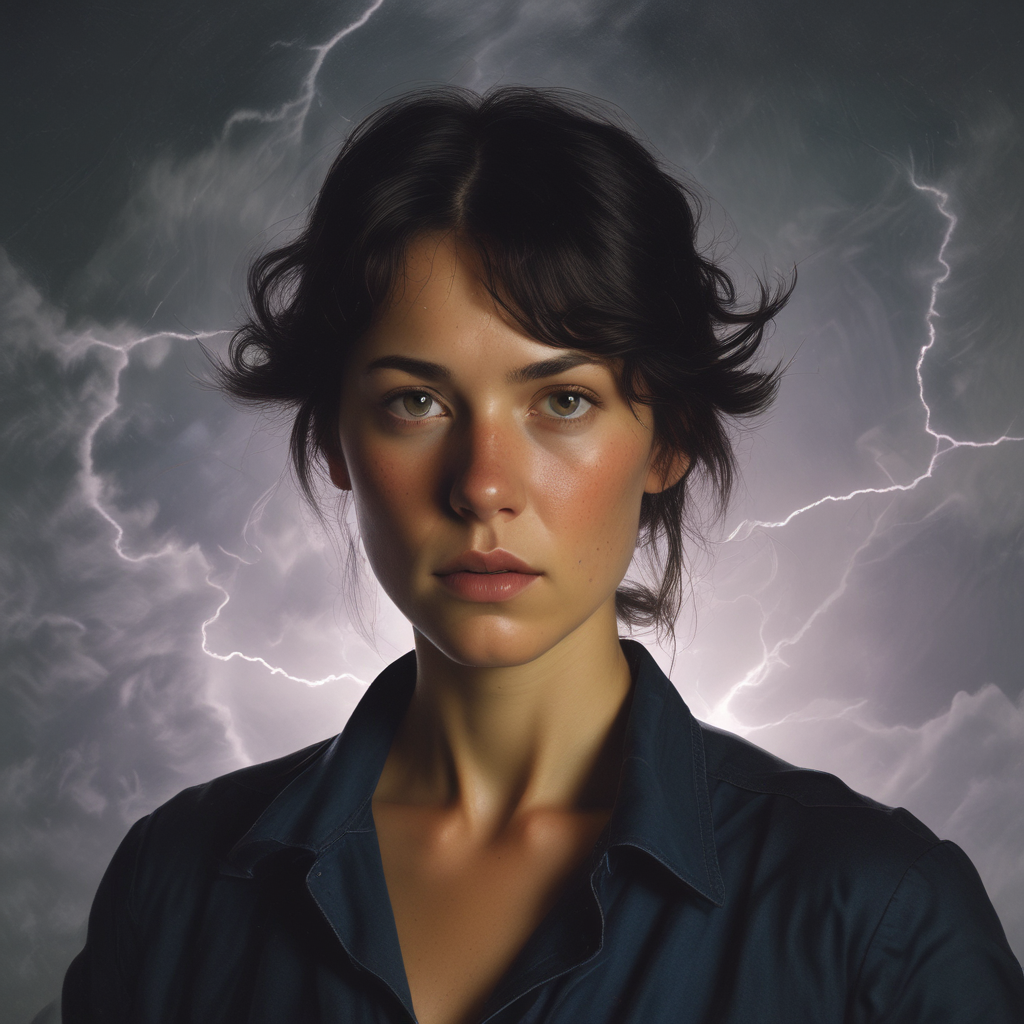
sdxl-lightning-4step

414.6K
sdxl-lightning-4step is a fast text-to-image model developed by ByteDance that can generate high-quality images in just 4 steps. It is similar to other fast diffusion models like AnimateDiff-Lightning and Instant-ID MultiControlNet, which also aim to speed up the image generation process. Unlike the original Stable Diffusion model, these fast models sacrifice some flexibility and control to achieve faster generation times. Model inputs and outputs The sdxl-lightning-4step model takes in a text prompt and various parameters to control the output image, such as the width, height, number of images, and guidance scale. The model can output up to 4 images at a time, with a recommended image size of 1024x1024 or 1280x1280 pixels. Inputs Prompt**: The text prompt describing the desired image Negative prompt**: A prompt that describes what the model should not generate Width**: The width of the output image Height**: The height of the output image Num outputs**: The number of images to generate (up to 4) Scheduler**: The algorithm used to sample the latent space Guidance scale**: The scale for classifier-free guidance, which controls the trade-off between fidelity to the prompt and sample diversity Num inference steps**: The number of denoising steps, with 4 recommended for best results Seed**: A random seed to control the output image Outputs Image(s)**: One or more images generated based on the input prompt and parameters Capabilities The sdxl-lightning-4step model is capable of generating a wide variety of images based on text prompts, from realistic scenes to imaginative and creative compositions. The model's 4-step generation process allows it to produce high-quality results quickly, making it suitable for applications that require fast image generation. What can I use it for? The sdxl-lightning-4step model could be useful for applications that need to generate images in real-time, such as video game asset generation, interactive storytelling, or augmented reality experiences. Businesses could also use the model to quickly generate product visualization, marketing imagery, or custom artwork based on client prompts. Creatives may find the model helpful for ideation, concept development, or rapid prototyping. Things to try One interesting thing to try with the sdxl-lightning-4step model is to experiment with the guidance scale parameter. By adjusting the guidance scale, you can control the balance between fidelity to the prompt and diversity of the output. Lower guidance scales may result in more unexpected and imaginative images, while higher scales will produce outputs that are closer to the specified prompt.
Updated Invalid Date
💬
hrrzg-style-768px

89
The hrrzg-style-768px model is a Stable Diffusion model trained by TheLastBen on the work of photographer Fred Herzog. This model can generate images in the distinct style of Herzog's photography, with a focus on detailed, cinematic street scenes and portraiture. Similar models include the herge-style model trained on the Hergé style, and the Evt_V3 model for a more general anime-inspired aesthetic. Model inputs and outputs The hrrzg-style-768px model takes text prompts as input and generates high-resolution images in the style of Fred Herzog's photography. The model was trained on a dataset of 30 images at 768x768 resolution, with a focus on detailed street scenes and portraiture. Inputs Text prompts describing the desired image, including elements like subject, setting, and style Outputs High-resolution images (896x768 and above) in the distinct aesthetic of Fred Herzog's photography, with a cinematic, detailed look Capabilities The hrrzg-style-768px model can generate striking, realistic images in the style of Fred Herzog's work. It excels at producing detailed, moody street scenes and portraits with a cinematic quality. The model can capture the essence of Herzog's style, including the use of natural lighting, muted colors, and a focus on candid, gritty urban environments. What can I use it for? The hrrzg-style-768px model could be valuable for artists, photographers, and designers looking to recreate or be inspired by the aesthetic of Fred Herzog's photography. It could be used to generate images for use in media, advertising, or creative projects, or as a starting point for further artistic exploration and editing. The model's capabilities in portraiture and street scenes make it a versatile tool for a variety of applications. Things to try One interesting avenue to explore with the hrrzg-style-768px model would be combining its distinct aesthetic with other visual styles or subjects. Experimenting with prompts that blend the Herzog look with elements from other genres, like science fiction or fantasy, could yield unique and striking results. Additionally, users could try fine-tuning the model further on their own datasets to create highly personalized variations on the Fred Herzog style.
Updated Invalid Date
👁️
SD2-768-Papercut

66
The SD2-768-Papercut model is a Textual Inversion Embedding for Stable Diffusion 2.0 created by ShadoWxShinigamI. It is based on ShadoWxShinigamI's previous MJv4-Paper Cut Model, and is designed to be fully customizable with prompts. The model was trained using 106 manually captioned images at 768x768 resolution over 150 steps, with 8 Textual Inversion vectors. Model inputs and outputs Inputs Textual prompts that can be customized for a variety of subjects, including ships, dogs, lions, people, landscapes, and buildings. Outputs High-quality, photorealistic images generated in the style of the training data, which includes a variety of scenes and subject matter. Capabilities The SD2-768-Papercut model is capable of generating detailed, visually striking images across a range of subject matter. The examples provided show its versatility in depicting ships, animals, people, and landscapes with a distinctive photographic quality. The model's ability to handle a variety of prompt inputs without extensive engineering suggests it could be a useful tool for content creators or designers looking to quickly generate concept art or visual references. What can I use it for? The SD2-768-Papercut model could be used for a variety of projects that require high-quality, customizable images. Some potential use cases include: Generating visual concepts or references for game, film, or product design Creating unique images for blog posts, social media, or marketing materials Exploring different artistic styles or photographic techniques through prompt experimentation Things to try One interesting aspect of the SD2-768-Papercut model is its ability to generate visually cohesive images from diverse prompt inputs. For example, the model can depict a wide range of subjects, from natural landscapes to man-made objects, while maintaining a consistent level of detail and photorealism. This suggests the model has learned robust representations of visual elements that can be flexibly combined to create novel compositions. Prompt engineers or artists could experiment with the model by trying to push the boundaries of its capabilities, such as by combining multiple subjects in a single image, or by introducing more abstract or fantastical elements into the prompts. The model's performance on these types of prompts could reveal interesting insights about its inner workings and potential areas for further development.
Updated Invalid Date