dreambooth-batch
Maintainer: anotherjesse

1.0K
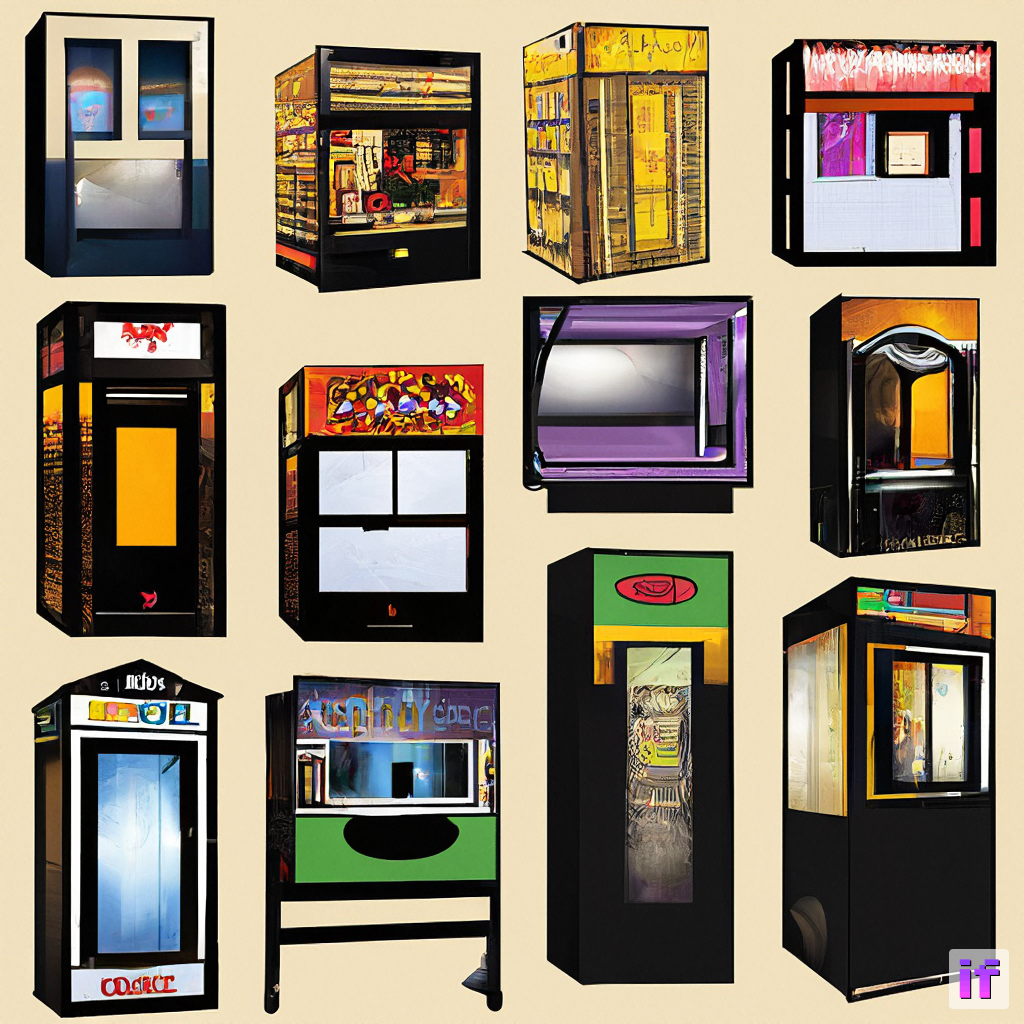
Property | Value |
---|---|
Run this model | Run on Replicate |
API spec | View on Replicate |
Github link | View on Github |
Paper link | No paper link provided |
Create account to get full access
Model overview
dreambooth-batch
is a batch inference model for Stable Diffusion's DreamBooth training process, developed by Replicate. It is based on the cog-stable-diffusion model, which utilizes the Diffusers library. This model allows for efficient batch generation of images based on DreamBooth-trained models, enabling users to quickly create personalized content.
Model inputs and outputs
The dreambooth-batch
model takes two key inputs: a set of images and a URL pointing to the trained DreamBooth model weights. The images are used to generate new content based on the DreamBooth model, while the weights file provides the necessary information for the model to perform the image generation.
Inputs
- Images: A JSON input containing the images to be used for generation
- Weights: A URL pointing to the trained DreamBooth model weights
Outputs
- Output Images: An array of generated image URLs
Capabilities
The dreambooth-batch
model excels at generating personalized content based on DreamBooth-trained models. It allows users to quickly create images of their own concepts or characters, leveraging the capabilities of Stable Diffusion's text-to-image generation.
What can I use it for?
The dreambooth-batch
model can be used to generate custom content for a variety of applications, such as:
- Creating personalized illustrations, avatars, or characters for games, apps, or websites
- Generating images for marketing, advertising, or social media campaigns
- Producing unique stock imagery or visual assets for commercial use
By using the DreamBooth training process and the efficient batch inference capabilities of dreambooth-batch
, users can easily create high-quality, personalized content that aligns with their specific needs or brand.
Things to try
One key feature of the dreambooth-batch
model is its ability to handle batch processing of images. This can be particularly useful for users who need to generate large volumes of content quickly, such as for animation or video production. Additionally, the model's integration with the Diffusers library allows for seamless integration with other Stable Diffusion-based models, such as Real-ESRGAN for image upscaling and enhancement.
This summary was produced with help from an AI and may contain inaccuracies - check out the links to read the original source documents!
Related Models
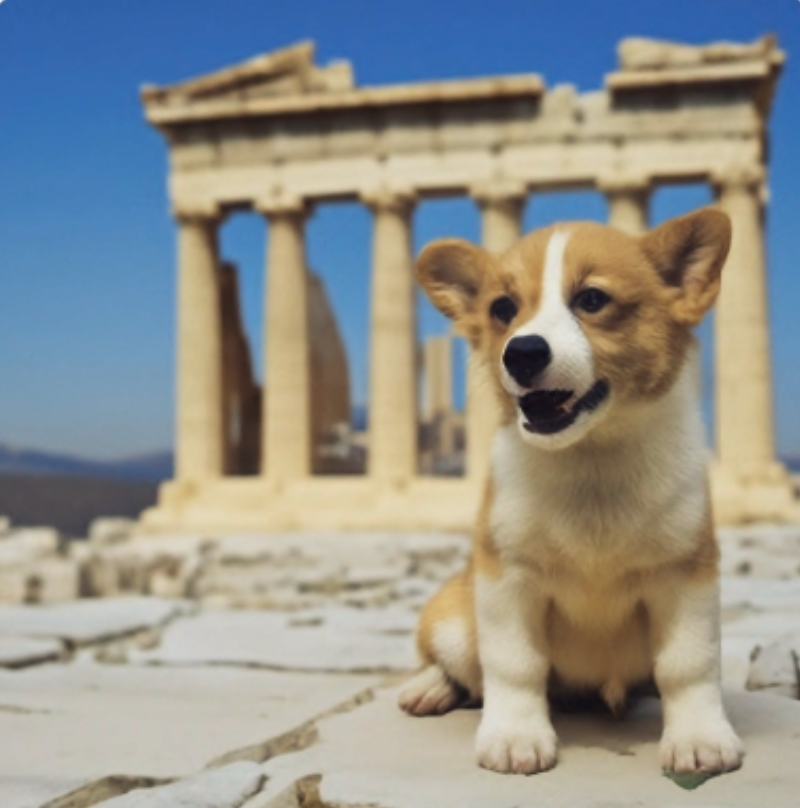
dreambooth

295
dreambooth is a deep learning model developed by researchers from Google Research and Boston University in 2022. It is used to fine-tune existing text-to-image models, such as Stable Diffusion, allowing them to generate more personalized and customized outputs. By training the model on a small set of images, dreambooth can learn to associate a unique identifier with a specific subject, enabling the generation of new images that feature that subject in various contexts. Model inputs and outputs dreambooth takes a set of training images as input, along with prompts that describe the subject and class of those images. The model then outputs trained weights that can be used to generate custom variants of the base text-to-image model, such as Stable Diffusion. Inputs instance_data: A ZIP file containing the training images of the subject you want to specialize the model for. instance_prompt: A prompt that describes the subject of the training images, in the format "a [identifier] [class noun]". class_prompt: A prompt that describes the broader class of the training images, in the format "a [class noun]". class_data (optional): A ZIP file containing training images for the broader class, to help the model maintain generalization. Outputs Trained weights that can be used to generate images with the customized subject. Capabilities dreambooth allows you to fine-tune a pre-trained text-to-image model, such as Stable Diffusion, to specialize in generating images of a specific subject. By training on a small set of images, the model can learn to associate a unique identifier with that subject, enabling the generation of new images that feature the subject in various contexts. What can I use it for? You can use dreambooth to create your own custom variants of text-to-image models, allowing you to generate images that feature specific subjects, characters, or objects. This can be useful for a variety of applications, such as: Generating personalized content for marketing or e-commerce Creating custom assets for video games, films, or other media Exploring creative and artistic use cases by training the model on your own unique subjects Things to try One interesting aspect of dreambooth is its ability to maintain the generalization of the base text-to-image model, even as it specializes in a specific subject. By incorporating the class_prompt and optional class_data, the model can learn to generate a variety of images within the broader class, while still retaining the customized subject. Try experimenting with different prompts and training data to see how this balance can be achieved.
Updated Invalid Date
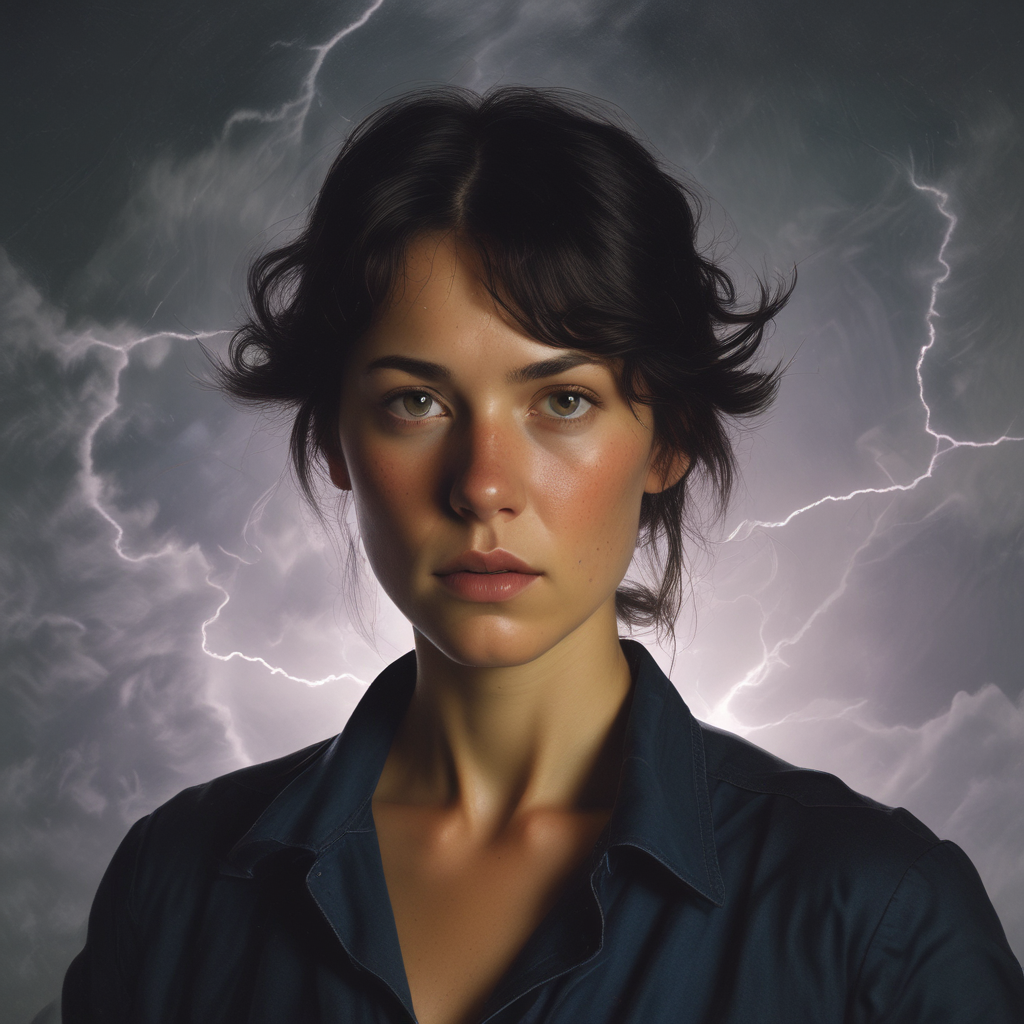
sdxl-lightning-4step

412.2K
sdxl-lightning-4step is a fast text-to-image model developed by ByteDance that can generate high-quality images in just 4 steps. It is similar to other fast diffusion models like AnimateDiff-Lightning and Instant-ID MultiControlNet, which also aim to speed up the image generation process. Unlike the original Stable Diffusion model, these fast models sacrifice some flexibility and control to achieve faster generation times. Model inputs and outputs The sdxl-lightning-4step model takes in a text prompt and various parameters to control the output image, such as the width, height, number of images, and guidance scale. The model can output up to 4 images at a time, with a recommended image size of 1024x1024 or 1280x1280 pixels. Inputs Prompt**: The text prompt describing the desired image Negative prompt**: A prompt that describes what the model should not generate Width**: The width of the output image Height**: The height of the output image Num outputs**: The number of images to generate (up to 4) Scheduler**: The algorithm used to sample the latent space Guidance scale**: The scale for classifier-free guidance, which controls the trade-off between fidelity to the prompt and sample diversity Num inference steps**: The number of denoising steps, with 4 recommended for best results Seed**: A random seed to control the output image Outputs Image(s)**: One or more images generated based on the input prompt and parameters Capabilities The sdxl-lightning-4step model is capable of generating a wide variety of images based on text prompts, from realistic scenes to imaginative and creative compositions. The model's 4-step generation process allows it to produce high-quality results quickly, making it suitable for applications that require fast image generation. What can I use it for? The sdxl-lightning-4step model could be useful for applications that need to generate images in real-time, such as video game asset generation, interactive storytelling, or augmented reality experiences. Businesses could also use the model to quickly generate product visualization, marketing imagery, or custom artwork based on client prompts. Creatives may find the model helpful for ideation, concept development, or rapid prototyping. Things to try One interesting thing to try with the sdxl-lightning-4step model is to experiment with the guidance scale parameter. By adjusting the guidance scale, you can control the balance between fidelity to the prompt and diversity of the output. Lower guidance scales may result in more unexpected and imaginative images, while higher scales will produce outputs that are closer to the specified prompt.
Updated Invalid Date
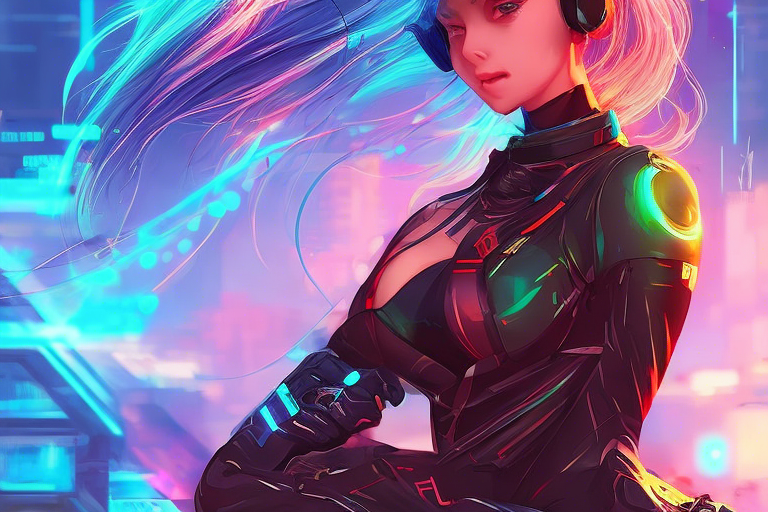
sdv2-preview

28
sdv2-preview is a preview of Stable Diffusion 2.0, a latent diffusion model capable of generating photorealistic images from text prompts. It was created by anotherjesse and builds upon the original Stable Diffusion model. The sdv2-preview model uses a downsampling-factor 8 autoencoder with an 865M UNet and OpenCLIP ViT-H/14 text encoder, producing 768x768 px outputs. It is trained from scratch and can be sampled with higher guidance scales than the original Stable Diffusion. Model inputs and outputs The sdv2-preview model takes a text prompt as input and generates one or more corresponding images as output. The text prompt can describe any scene, object, or concept, and the model will attempt to create a photorealistic visualization of it. Inputs Prompt**: A text description of the desired image content. Seed**: An optional random seed to control the stochastic generation process. Width/Height**: The desired dimensions of the output image, up to 1024x768 or 768x1024. Num Outputs**: The number of images to generate (up to 10). Guidance Scale**: A value that controls the trade-off between fidelity to the prompt and creativity in the generation process. Num Inference Steps**: The number of denoising steps used in the diffusion process. Outputs Images**: One or more photorealistic images corresponding to the input prompt. Capabilities The sdv2-preview model is capable of generating a wide variety of photorealistic images from text prompts, including landscapes, portraits, abstract concepts, and fantastical scenes. It has been trained on a large, diverse dataset and can handle complex prompts with multiple elements. What can I use it for? The sdv2-preview model can be used for a variety of creative and practical applications, such as: Generating concept art or illustrations for creative projects. Prototyping product designs or visualizing ideas. Creating unique and personalized images for marketing or social media. Exploring creative prompts and ideas without the need for traditional artistic skills. Things to try Some interesting things to try with the sdv2-preview model include: Experimenting with different types of prompts, from the specific to the abstract. Combining the model with other tools, such as image editing software or 3D modeling tools, to create more complex and integrated visuals. Exploring the model's capabilities for specific use cases, such as product design, character creation, or scientific visualization. Comparing the output of sdv2-preview to similar models, such as the original Stable Diffusion or the Stable Diffusion 2-1-unclip model, to understand the model's unique strengths and characteristics.
Updated Invalid Date
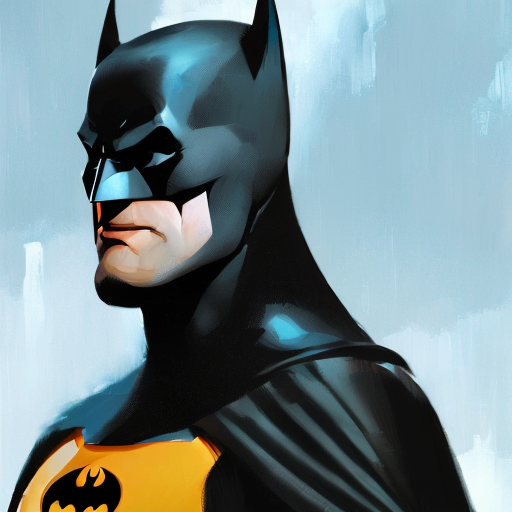
wavyfusion

3
wavyfusion is a Dreambooth-trained AI model that can generate diverse images, ranging from photographs to paintings. It was created by the Replicate user cjwbw, who has also developed similar models like analog-diffusion, portraitplus, and contributed to models like dreambooth and dreambooth-batch. These models demonstrate the versatility of Dreambooth, a technique that allows training custom Stable Diffusion models on a small set of images. Model inputs and outputs wavyfusion is a text-to-image AI model that generates images based on a provided prompt. The model takes in a variety of inputs, including the prompt, seed, image size, number of outputs, and more. The outputs are a set of generated images that match the given prompt. Inputs Prompt**: The text description of the desired image Seed**: A random seed value to control the image generation Width/Height**: The desired dimensions of the output image Num Outputs**: The number of images to generate Scheduler**: The algorithm used to generate the images Guidance Scale**: The scale for classifier-free guidance Negative Prompt**: Specify things to not see in the output Prompt Strength**: The strength of the prompt when using an initial image Num Inference Steps**: The number of denoising steps to take Outputs A set of generated images that match the provided prompt Capabilities wavyfusion can generate a wide variety of images, from realistic photographs to creative and artistic renderings. The model's diverse training dataset allows it to produce images in many different styles and genres, making it a versatile tool for various creative applications. What can I use it for? With wavyfusion's capabilities, you can use it for all sorts of creative projects, such as: Generating concept art or illustrations for stories, games, or other media Producing unique and personalized images for marketing, branding, or social media Experimenting with different art styles and techniques Visualizing ideas or concepts that are difficult to express through traditional means Things to try One interesting aspect of wavyfusion is its ability to blend different artistic styles and techniques. Try experimenting with prompts that combine elements from various genres, such as "a surrealist portrait of a person in the style of impressionist painting". This can lead to unexpected and visually striking results.
Updated Invalid Date