effnet-discogs
Maintainer: mtg

114
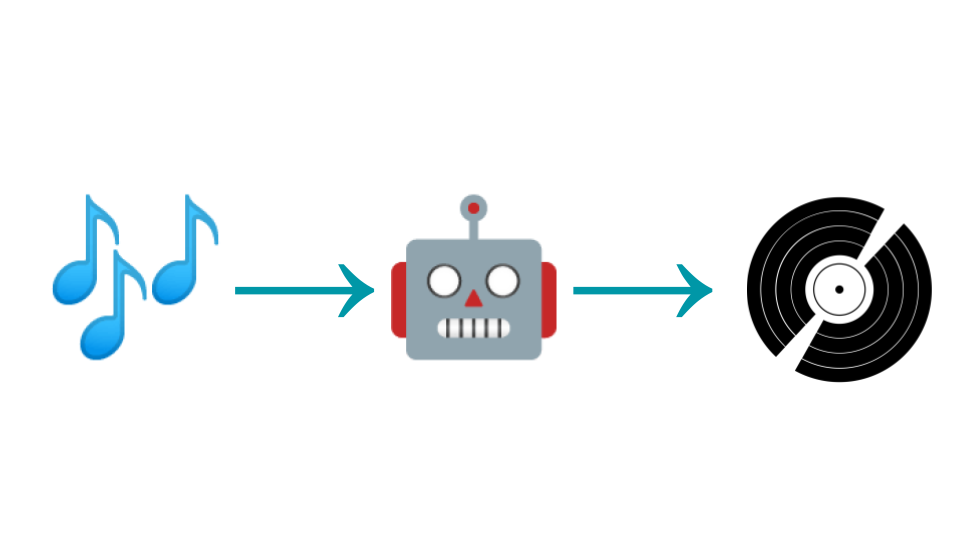
Property | Value |
---|---|
Run this model | Run on Replicate |
API spec | View on Replicate |
Github link | View on Github |
Paper link | No paper link provided |
Create account to get full access
Model overview
The effnet-discogs
model is an EfficientNet model trained for music style classification. It can identify 400 different music styles from the Discogs taxonomy. This model is part of a collection of music classification models developed by the Music Technology Group (MTG) at Universitat Pompeu Fabra.
The effnet-discogs
model is similar to other music classification models from MTG, such as music-classifiers, which can identify music genres, moods, and instrumentation, and music-approachability-engagement, which classifies music based on approachability and engagement.
Model inputs and outputs
The effnet-discogs
model takes either a YouTube URL or an audio file as input, and outputs a list of the top N most likely music styles from the Discogs taxonomy, along with their probabilities.
Inputs
- Url: A YouTube URL to process (overrides the audio input)
- Audio: An audio file to process
- Top N: The number of top music styles to return (default is 10)
- Output Format: The output format, which can be either a bar chart visualization or a JSON blob
Outputs
- Output: A URI (URL) pointing to the model's output, which can be either a visualization or a JSON blob
Capabilities
The effnet-discogs
model can accurately classify music into 400 different styles from the Discogs taxonomy. This can be useful for music discovery, recommendation, and analysis applications.
What can I use it for?
You can use the effnet-discogs
model to build music classification and discovery applications. For example, you could integrate it into a music streaming service to provide detailed genre and style information for the songs in your library. You could also use it to build a music discovery tool that recommends new artists and genres based on a user's listening history.
Things to try
Try using the effnet-discogs
model to classify a diverse set of music, including both popular and obscure genres. This can help you understand the model's capabilities and limitations, and identify potential areas for improvement or fine-tuning.
This summary was produced with help from an AI and may contain inaccuracies - check out the links to read the original source documents!
Related Models
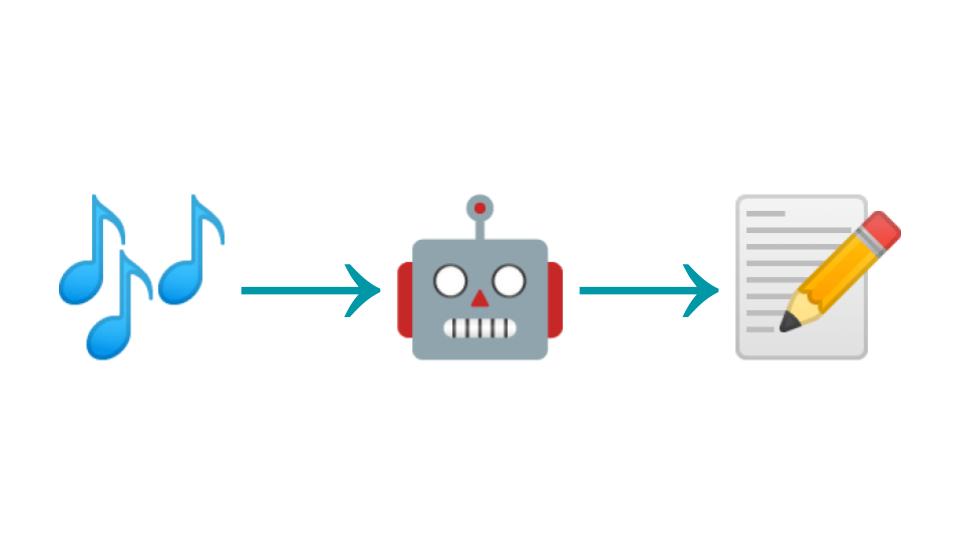
music-classifiers

9
The music-classifiers model is a set of transfer learning models developed by MTG for classifying music by genres, moods, and instrumentation. It is part of the Essentia replicate demos hosted on Replicate.ai's MTG site. The model is built on top of the open-source Essentia library and provides pre-trained models for a variety of music classification tasks. This model can be seen as complementary to other music-related models like music-approachability-engagement, music-arousal-valence, and the MusicGen and MusicGen-melody models from Meta/Facebook, which focus on music generation and analysis. Model inputs and outputs Inputs Url**: YouTube URL to process (overrides audio input) Audio**: Audio file to process model_type**: Model type (embeddings) Outputs Output**: URI representing the processed audio Capabilities The music-classifiers model can be used to classify music along several dimensions, including genre, mood, and instrumentation. This can be useful for applications like music recommendation, audio analysis, and music information retrieval. The model provides pre-trained embeddings that can be used as features for a variety of downstream music-related tasks. What can I use it for? Researchers and developers working on music-related applications can use the music-classifiers model to add music classification capabilities to their projects. For example, the model could be integrated into a music recommendation system to suggest songs based on genre or mood, or used in an audio analysis tool to automatically extract information about the instrumentation in a piece of music. The model's pre-trained embeddings can also be used as input features for custom machine learning models, enabling more sophisticated music analysis and classification tasks. Things to try One interesting thing to try with the music-classifiers model is to experiment with different music genres, moods, and instrumentation combinations to see how the model classifies and represents them. You could also try using the model's embeddings as input features for your own custom music classification models, and see how they perform compared to other approaches. Additionally, you could explore ways to combine the music-classifiers model with other music-related models, such as the MusicGen and MusicGen-melody models, to create more comprehensive music analysis and generation pipelines.
Updated Invalid Date
📈
music-approachability-engagement

6
The music-approachability-engagement model is a classification model developed by the MTG team that aims to assess the approachability and engagement of music. This model can be useful for research on AI-based music generation, as it provides insights into how humans perceive the "approachability" and "engagement" of generated music. The model is part of a suite of Essentia models hosted on Replicate. Similar models in this space include MusicGen from Meta, which can generate music from text or melody, and Stable Diffusion, a text-to-image diffusion model. These models showcase the capabilities of AI in music and creative domains, although they have different specific use cases compared to the music-approachability-engagement model. Model inputs and outputs Inputs URL**: A YouTube URL to process (overrides audio input) Audio**: An audio file to process Model type**: Specifies the downstream task, such as regression or classification (2-class or 3-class) Outputs Output**: A URI representing the processed output from the model Capabilities The music-approachability-engagement model can classify the "approachability" and "engagement" of music, which can be useful for understanding how humans perceive the accessibility and captivating nature of generated music. This type of insight can inform the development of more user-friendly and engaging AI-generated music. What can I use it for? Researchers in audio, machine learning, and artificial intelligence can use the music-approachability-engagement model to better understand the capabilities and limitations of current generative music models. By analyzing the model's classifications, researchers can gain insights into how to improve the perceptual qualities of AI-generated music. Additionally, amateur users interested in understanding generative music models can experiment with the model to see how it responds to different audio inputs. Things to try One interesting thing to try with the music-approachability-engagement model is to input a variety of music genres and styles, and observe how the model classifies the "approachability" and "engagement" of each. This could reveal patterns or biases in the model's understanding of music perception. Additionally, experimenting with different input audio and comparing the model's classifications could provide valuable feedback for improving the model's capabilities.
Updated Invalid Date
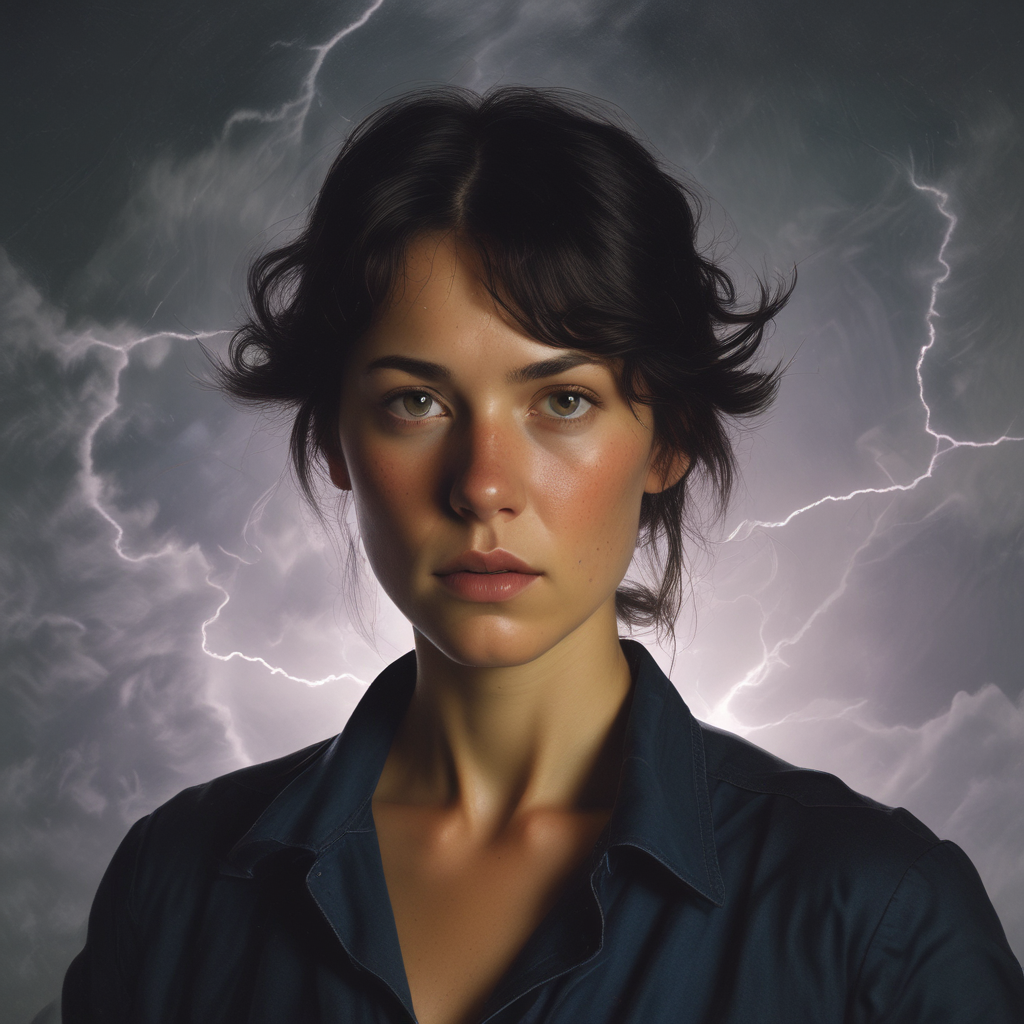
sdxl-lightning-4step

412.2K
sdxl-lightning-4step is a fast text-to-image model developed by ByteDance that can generate high-quality images in just 4 steps. It is similar to other fast diffusion models like AnimateDiff-Lightning and Instant-ID MultiControlNet, which also aim to speed up the image generation process. Unlike the original Stable Diffusion model, these fast models sacrifice some flexibility and control to achieve faster generation times. Model inputs and outputs The sdxl-lightning-4step model takes in a text prompt and various parameters to control the output image, such as the width, height, number of images, and guidance scale. The model can output up to 4 images at a time, with a recommended image size of 1024x1024 or 1280x1280 pixels. Inputs Prompt**: The text prompt describing the desired image Negative prompt**: A prompt that describes what the model should not generate Width**: The width of the output image Height**: The height of the output image Num outputs**: The number of images to generate (up to 4) Scheduler**: The algorithm used to sample the latent space Guidance scale**: The scale for classifier-free guidance, which controls the trade-off between fidelity to the prompt and sample diversity Num inference steps**: The number of denoising steps, with 4 recommended for best results Seed**: A random seed to control the output image Outputs Image(s)**: One or more images generated based on the input prompt and parameters Capabilities The sdxl-lightning-4step model is capable of generating a wide variety of images based on text prompts, from realistic scenes to imaginative and creative compositions. The model's 4-step generation process allows it to produce high-quality results quickly, making it suitable for applications that require fast image generation. What can I use it for? The sdxl-lightning-4step model could be useful for applications that need to generate images in real-time, such as video game asset generation, interactive storytelling, or augmented reality experiences. Businesses could also use the model to quickly generate product visualization, marketing imagery, or custom artwork based on client prompts. Creatives may find the model helpful for ideation, concept development, or rapid prototyping. Things to try One interesting thing to try with the sdxl-lightning-4step model is to experiment with the guidance scale parameter. By adjusting the guidance scale, you can control the balance between fidelity to the prompt and diversity of the output. Lower guidance scales may result in more unexpected and imaginative images, while higher scales will produce outputs that are closer to the specified prompt.
Updated Invalid Date
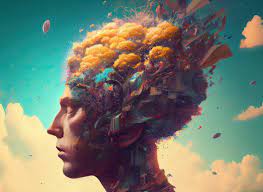
neurogen

2
The neurogen model is a Stable Diffusion-based AI model developed by replicategithubwc for generating "splurge art". It shares similarities with other AI models like dreamlike-photoreal, anime-pastel-dream, and nebul.redmond, which also focus on producing artistic, surreal, or dreamlike imagery. Model inputs and outputs The neurogen model takes in a text prompt, along with various parameters to control the output, such as the image size, the number of inference steps, and the guidance scale. The model then generates one or more images based on the provided inputs. Inputs Prompt**: The text prompt that describes the desired image Seed**: A random seed value to control the randomness of the generated image Width/Height**: The desired dimensions of the output image Num Outputs**: The number of images to generate Guidance Scale**: A parameter to control the influence of the text prompt on the generated image Negative Prompt**: Text to specify things the model should not include in the output Num Inference Steps**: The number of denoising steps to perform during the generation process Scheduler**: The algorithm used to control the diffusion process Outputs Image(s)**: One or more generated images that match the provided prompt and other input parameters Capabilities The neurogen model is capable of generating a wide variety of surreal, dreamlike, and artistic images based on the input prompt. It can produce images with a unique, visually striking aesthetic that sets it apart from more photorealistic AI image generation models. What can I use it for? The neurogen model could be useful for creators, artists, and designers who are looking to generate unique, abstract, or conceptual imagery for a variety of applications, such as album covers, book illustrations, marketing materials, or digital art. The model's ability to create visually striking and imaginative images may also make it useful for hobbyists or enthusiasts who enjoy exploring the creative potential of AI-generated art. Things to try One interesting aspect of the neurogen model is its ability to generate images with a strong sense of mood and atmosphere. By experimenting with different prompts and input parameters, users may be able to create images that evoke specific emotions or convey a particular narrative or mood. Additionally, the model's versatility in producing a range of artistic styles, from surreal to dreamlike, presents opportunities for users to explore and push the boundaries of AI-generated art.
Updated Invalid Date