face-align-cog
Maintainer: cjwbw

4
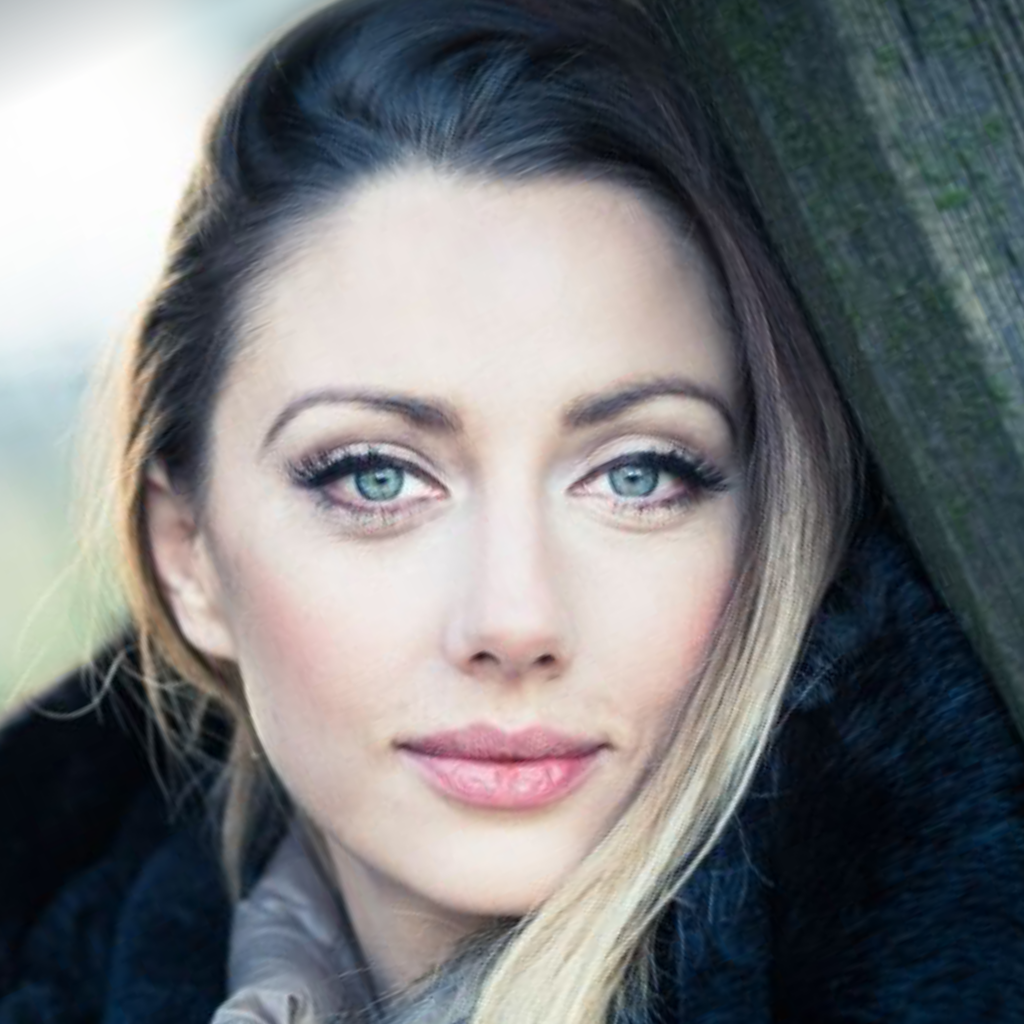
Property | Value |
---|---|
Run this model | Run on Replicate |
API spec | View on Replicate |
Github link | View on Github |
Paper link | No paper link provided |
Create account to get full access
Model overview
The face-align-cog
model is a Cog implementation of a face alignment code from the stylegan-encoder project. It is designed to preprocess input images by aligning and cropping faces, which is often a necessary step before using them with other models. The model is similar to other face processing tools like GFPGAN and style-your-hair, which focus on face restoration and hairstyle transfer respectively.
Model inputs and outputs
The face-align-cog
model takes a single input of an image URI and outputs a new image URI with the face aligned and cropped.
Inputs
- Image: The input source image.
Outputs
- Output: The image with the face aligned and cropped.
Capabilities
The face-align-cog
model can be used to preprocess input images by aligning and cropping the face. This can be useful when working with models that require well-aligned faces, such as face recognition or face generation models.
What can I use it for?
The face-align-cog
model can be used as a preprocessing step for a variety of computer vision tasks that involve faces, such as face recognition, face generation, or facial analysis. It could be integrated into a larger pipeline or used as a standalone tool to prepare images for use with other models.
Things to try
You could try using the face-align-cog
model to preprocess your own images before using them with other face-related models, such as the GFPGAN model for face restoration or the style-your-hair model for hairstyle transfer. This can help ensure that your input images are properly aligned and cropped, which can improve the performance of those downstream models.
This summary was produced with help from an AI and may contain inaccuracies - check out the links to read the original source documents!
Related Models
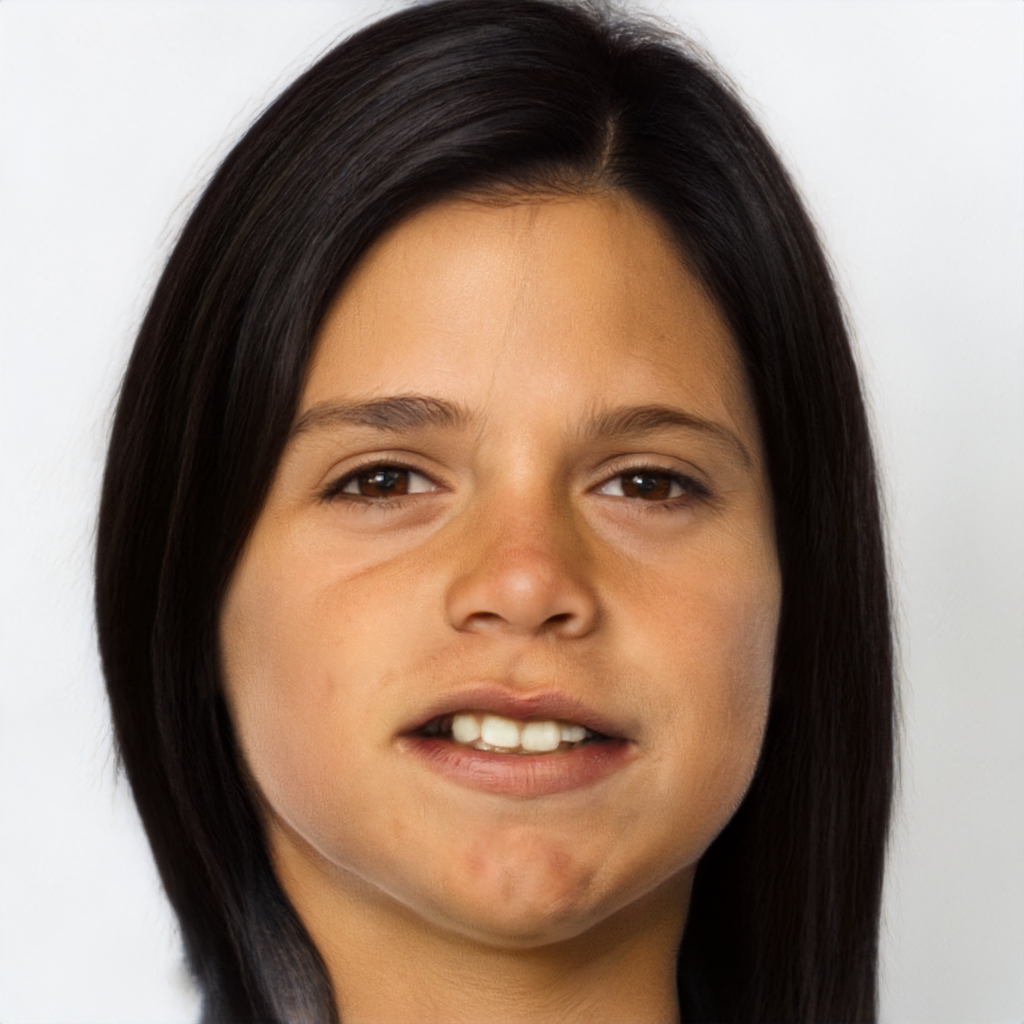
style-your-hair

9
The style-your-hair model, developed by the Replicate creator cjwbw, is a pose-invariant hairstyle transfer model that allows users to seamlessly transfer hairstyles between different facial poses. Unlike previous approaches that assumed aligned target and source images, this model utilizes a latent optimization technique and a local-style-matching loss to preserve the detailed textures of the target hairstyle even under significant pose differences. The model builds upon recent advances in hair modeling and leverages the capabilities of Stable Diffusion, a powerful text-to-image generation model, to produce high-quality hairstyle transfers. Similar models created by cjwbw include herge-style, anything-v4.0, and stable-diffusion-v2-inpainting. Model inputs and outputs The style-your-hair model takes two images as input: a source image containing a face and a target image containing the desired hairstyle. The model then seamlessly transfers the target hairstyle onto the source face, preserving the detailed texture and appearance of the target hairstyle even under significant pose differences. Inputs Source Image**: The image containing the face onto which the hairstyle will be transferred. Target Image**: The image containing the desired hairstyle to be transferred. Outputs Transferred Hairstyle Image**: The output image with the target hairstyle applied to the source face. Capabilities The style-your-hair model excels at transferring hairstyles between images with significant pose differences, a task that has historically been challenging. By leveraging a latent optimization technique and a local-style-matching loss, the model is able to preserve the detailed textures and appearance of the target hairstyle, resulting in high-quality, natural-looking transfers. What can I use it for? The style-your-hair model can be used in a variety of applications, such as virtual hair styling, entertainment, and fashion. For example, users could experiment with different hairstyles on their own photos or create unique hairstyles for virtual avatars. Businesses in the beauty and fashion industries could also leverage the model to offer personalized hair styling services or incorporate hairstyle transfer features into their products. Things to try One interesting aspect of the style-your-hair model is its ability to preserve the local-style details of the target hairstyle, even under significant pose differences. Users could experiment with transferring hairstyles between images with varying facial poses and angles, and observe how the model maintains the intricate textures and structure of the target hairstyle. Additionally, users could try combining the style-your-hair model with other Replicate models, such as anything-v3.0 or portraitplus, to explore more creative and personalized hair styling possibilities.
Updated Invalid Date
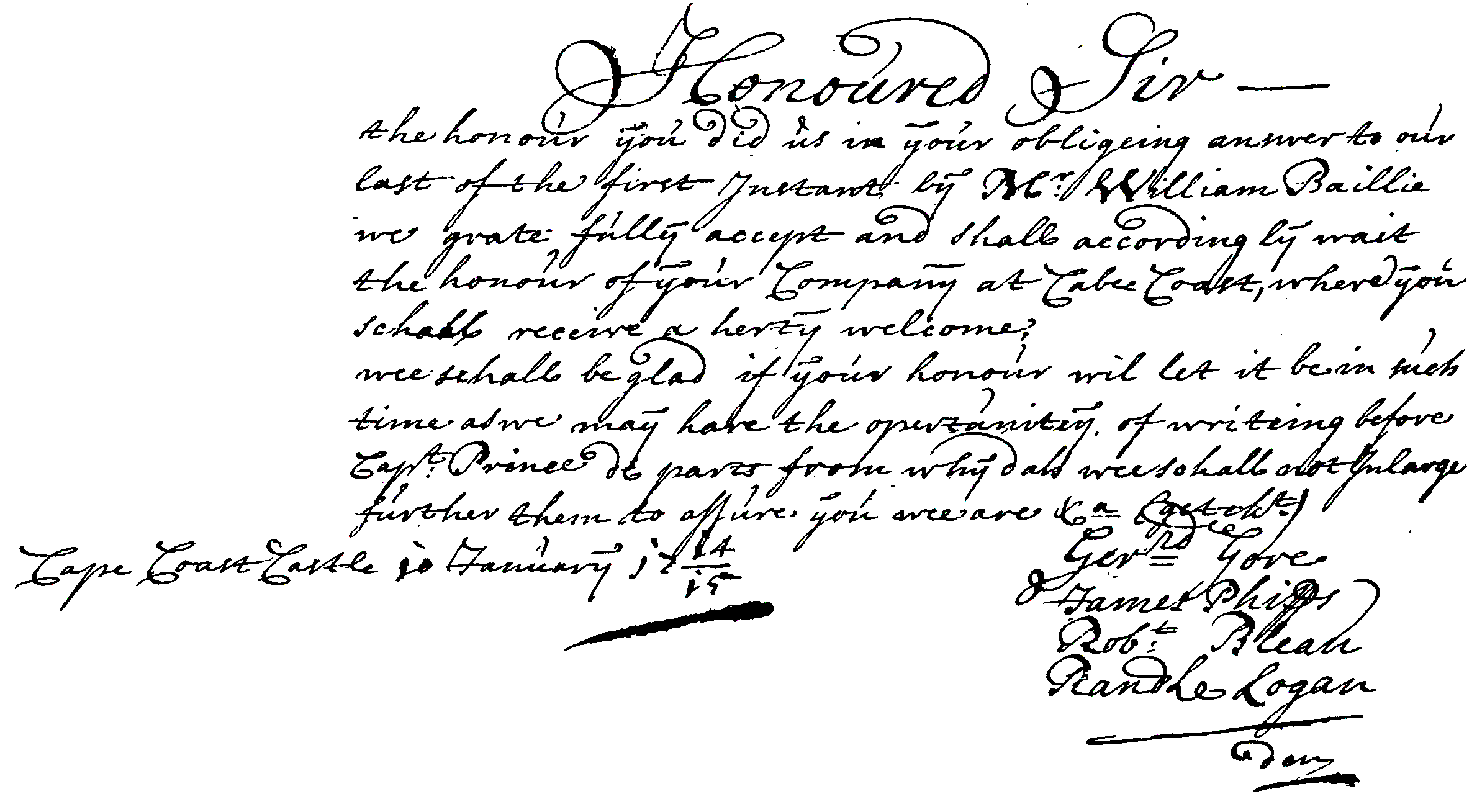
docentr

3
The docentr model is an end-to-end document image enhancement transformer developed by cjwbw. It is a PyTorch implementation of the paper "DocEnTr: An End-to-End Document Image Enhancement Transformer" and is built on top of the vit-pytorch vision transformers library. The model is designed to enhance and binarize degraded document images, as demonstrated in the provided examples. Model inputs and outputs The docentr model takes an image as input and produces an enhanced, binarized output image. The input image can be a degraded or low-quality document, and the model aims to improve its visual quality by performing tasks such as binarization, noise removal, and contrast enhancement. Inputs image**: The input image, which should be in a valid image format (e.g., PNG, JPEG). Outputs Output**: The enhanced, binarized output image. Capabilities The docentr model is capable of performing end-to-end document image enhancement, including binarization, noise removal, and contrast improvement. It can be used to improve the visual quality of degraded or low-quality document images, making them more readable and easier to process. The model has shown promising results on benchmark datasets such as DIBCO, H-DIBCO, and PALM. What can I use it for? The docentr model can be useful for a variety of applications that involve processing and analyzing document images, such as optical character recognition (OCR), document archiving, and image-based document retrieval. By enhancing the quality of the input images, the model can help improve the accuracy and reliability of downstream tasks. Additionally, the model's capabilities can be leveraged in projects related to document digitization, historical document restoration, and automated document processing workflows. Things to try You can experiment with the docentr model by testing it on your own degraded document images and observing the binarization and enhancement results. The model is also available as a pre-trained Replicate model, which you can use to quickly apply the image enhancement without training the model yourself. Additionally, you can explore the provided demo notebook to gain a better understanding of how to use the model and customize its configurations.
Updated Invalid Date
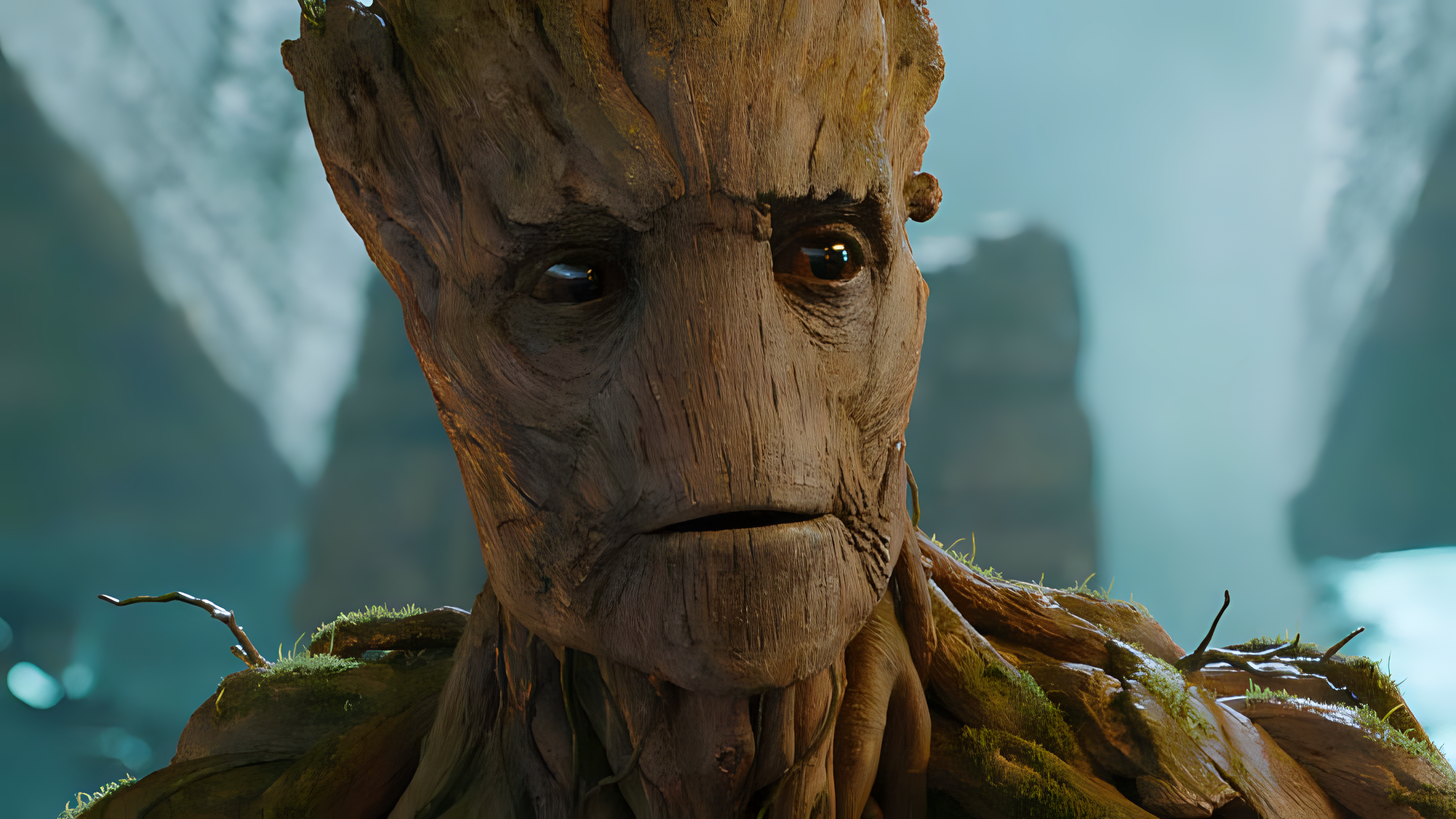
real-esrgan

1.7K
real-esrgan is an AI model developed by the creator cjwbw that focuses on real-world blind super-resolution. This means the model can upscale low-quality images without relying on a reference high-quality image. In contrast, similar models like real-esrgan and realesrgan also offer additional features like face correction, while seesr and supir incorporate semantic awareness and language models for enhanced image restoration. Model inputs and outputs real-esrgan takes an input image and an upscaling factor, and outputs a higher-resolution version of the input image. The model is designed to work well on a variety of real-world images, even those with significant noise or artifacts. Inputs Image**: The input image to be upscaled Outputs Output Image**: The upscaled version of the input image Capabilities real-esrgan excels at enlarging low-quality images while preserving details and reducing artifacts. This makes it useful for tasks such as enhancing photos, improving video resolution, and restoring old or damaged images. What can I use it for? real-esrgan can be used in a variety of applications where high-quality image enlargement is needed, such as photography, video editing, digital art, and image restoration. For example, you could use it to upscale low-resolution images for use in marketing materials, or to enhance old family photos. The model's ability to handle real-world images makes it a valuable tool for many image-related projects. Things to try One interesting aspect of real-esrgan is its ability to handle a wide range of input image types and qualities. Try experimenting with different types of images, such as natural scenes, portraits, or even text-heavy images, to see how the model performs. Additionally, you can try adjusting the upscaling factor to find the right balance between quality and file size for your specific use case.
Updated Invalid Date
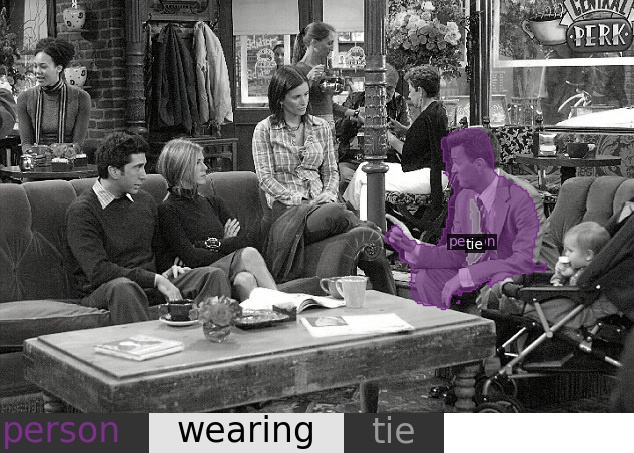
openpsg

1
openpsg is a powerful AI model for Panoptic Scene Graph Generation (PSG). Developed by researchers at Nanyang Technological University and SenseTime Research, openpsg aims to provide a comprehensive scene understanding by generating a scene graph representation that is grounded by pixel-accurate segmentation masks. This contrasts with classic Scene Graph Generation (SGG) datasets that use bounding boxes, which can result in coarse localization, inability to ground backgrounds, and trivial relationships. The openpsg model addresses these issues by using the COCO panoptic segmentation dataset to annotate relations based on segmentation masks rather than bounding boxes. It also carefully defines 56 predicates to avoid trivial or duplicated relationships. Similar models like gfpgan for face restoration, segmind-vega for accelerated Stable Diffusion, stable-diffusion for text-to-image generation, cogvlm for powerful visual language modeling, and real-esrgan for blind super-resolution, also tackle complex visual understanding tasks. Model inputs and outputs The openpsg model takes an input image and generates a scene graph representation of the content in the image. The scene graph consists of a set of nodes (objects) and edges (relationships) that comprehensively describe the scene. Inputs Image**: The input image to be analyzed. Num Rel**: The desired number of relationships to be generated in the scene graph, ranging from 1 to 20. Outputs Scene Graph**: An array of scene graph elements, where each element represents a relationship in the form of a subject, predicate, and object, all grounded by their corresponding segmentation masks in the input image. Capabilities openpsg excels at holistically understanding complex scenes by generating a detailed scene graph representation. Unlike classic SGG approaches that focus on objects and their relationships, openpsg considers both "things" (objects) and "stuff" (backgrounds) to provide a more comprehensive interpretation of the scene. What can I use it for? The openpsg model can be useful for a variety of applications that require a deep understanding of visual scenes, such as: Robotic Vision**: Enabling robots to better comprehend their surroundings and interact with objects and environments. Autonomous Driving**: Improving scene understanding for self-driving cars to navigate more safely and effectively. Visual Question Answering**: Enhancing the ability to answer questions about the contents and relationships in an image. Image Captioning**: Generating detailed captions that describe not just the objects, but also the interactions and spatial relationships in a scene. Things to try With the openpsg model, you can experiment with various types of images to see how it generates the scene graph representation. Try uploading photos of everyday scenes, like a living room or a park, and observe how the model identifies the objects, their attributes, and the relationships between them. You can also explore the potential of using the scene graph output for downstream tasks like visual reasoning or image-text matching.
Updated Invalid Date