glm-4v-9b
Maintainer: cuuupid

3.2K
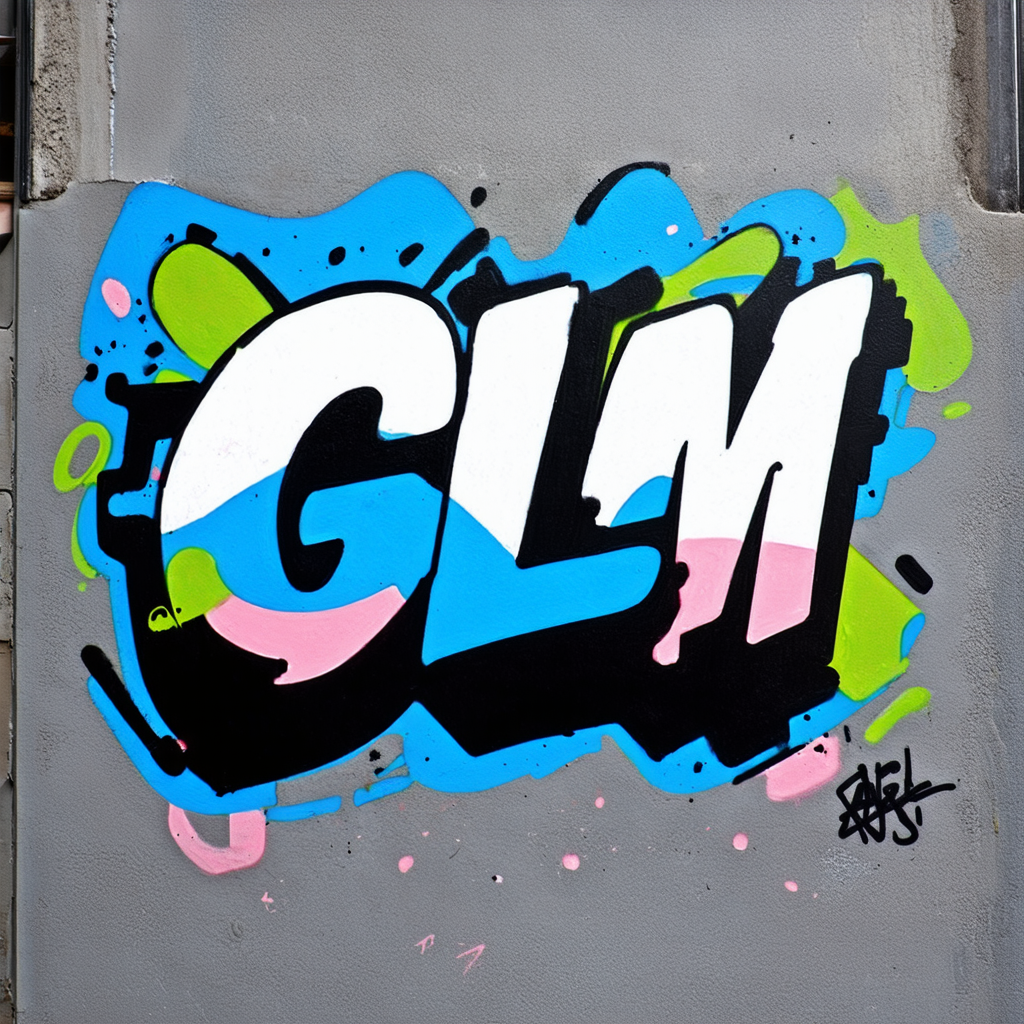
Property | Value |
---|---|
Run this model | Run on Replicate |
API spec | View on Replicate |
Github link | View on Github |
Paper link | No paper link provided |
Create account to get full access
Model overview
glm-4v-9b
is a powerful multimodal language model developed by Tsinghua University that demonstrates state-of-the-art performance on several benchmarks, including optical character recognition (OCR). It is part of the GLM-4 series of models, which includes the base glm-4-9b
model as well as the glm-4-9b-chat
and glm-4-9b-chat-1m
chat-oriented models. The glm-4v-9b
model specifically adds visual understanding capabilities, allowing it to excel at tasks like image description, visual question answering, and multimodal reasoning.
Compared to similar models like sdxl-lightning-4step and cogvlm, the glm-4v-9b
model stands out for its strong performance across a wide range of multimodal benchmarks, as well as its support for both Chinese and English languages. It has been shown to outperform models like GPT-4, Gemini 1.0 Pro, and Claude 3 Opus on these tasks.
Model inputs and outputs
Inputs
- Image: An image to be used as input for the model
- Prompt: A text prompt describing the task or query for the model
Outputs
- Output: The model's response, which could be a textual description of the input image, an answer to a visual question, or the result of a multimodal reasoning task.
Capabilities
The glm-4v-9b
model demonstrates strong multimodal understanding and generation capabilities. It can generate detailed, coherent descriptions of input images, answer questions about the visual content, and perform tasks like visual reasoning and optical character recognition. For example, the model can analyze a complex chart or diagram and provide a summary of the key information and insights.
What can I use it for?
The glm-4v-9b
model could be a valuable tool for a variety of applications that require multimodal intelligence, such as:
- Intelligent image captioning and visual question answering for social media, e-commerce, or creative applications
- Multimodal document understanding and analysis for business intelligence or research tasks
- Multimodal conversational AI assistants that can engage in visual and textual dialogue
The model's strong performance and broad capabilities make it a compelling option for developers and researchers looking to push the boundaries of what's possible with language models and multimodal AI.
Things to try
One interesting thing to try with the glm-4v-9b
model is exploring its ability to perform multimodal reasoning tasks. For example, you could provide the model with an image and a textual prompt that requires analyzing the visual information and drawing inferences. This could involve tasks like answering questions about the relationships between objects in the image, identifying anomalies or inconsistencies, or generating hypothetical scenarios based on the visual content.
Another area to explore is the model's potential for multimodal content generation. You could experiment with providing the model with a combination of image and text inputs, and see how it can generate new, creative content that seamlessly integrates the visual and textual elements.
This summary was produced with help from an AI and may contain inaccuracies - check out the links to read the original source documents!
Related Models
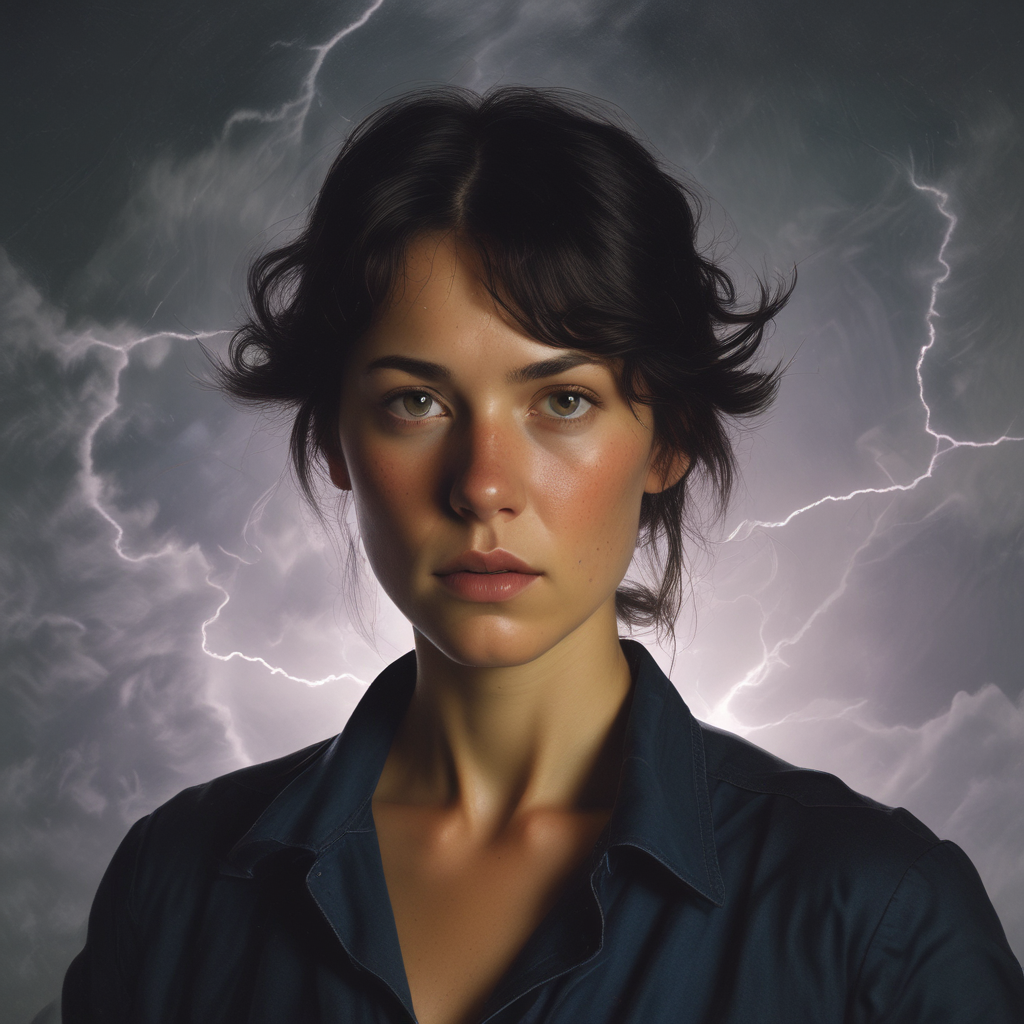
sdxl-lightning-4step

450.8K
sdxl-lightning-4step is a fast text-to-image model developed by ByteDance that can generate high-quality images in just 4 steps. It is similar to other fast diffusion models like AnimateDiff-Lightning and Instant-ID MultiControlNet, which also aim to speed up the image generation process. Unlike the original Stable Diffusion model, these fast models sacrifice some flexibility and control to achieve faster generation times. Model inputs and outputs The sdxl-lightning-4step model takes in a text prompt and various parameters to control the output image, such as the width, height, number of images, and guidance scale. The model can output up to 4 images at a time, with a recommended image size of 1024x1024 or 1280x1280 pixels. Inputs Prompt**: The text prompt describing the desired image Negative prompt**: A prompt that describes what the model should not generate Width**: The width of the output image Height**: The height of the output image Num outputs**: The number of images to generate (up to 4) Scheduler**: The algorithm used to sample the latent space Guidance scale**: The scale for classifier-free guidance, which controls the trade-off between fidelity to the prompt and sample diversity Num inference steps**: The number of denoising steps, with 4 recommended for best results Seed**: A random seed to control the output image Outputs Image(s)**: One or more images generated based on the input prompt and parameters Capabilities The sdxl-lightning-4step model is capable of generating a wide variety of images based on text prompts, from realistic scenes to imaginative and creative compositions. The model's 4-step generation process allows it to produce high-quality results quickly, making it suitable for applications that require fast image generation. What can I use it for? The sdxl-lightning-4step model could be useful for applications that need to generate images in real-time, such as video game asset generation, interactive storytelling, or augmented reality experiences. Businesses could also use the model to quickly generate product visualization, marketing imagery, or custom artwork based on client prompts. Creatives may find the model helpful for ideation, concept development, or rapid prototyping. Things to try One interesting thing to try with the sdxl-lightning-4step model is to experiment with the guidance scale parameter. By adjusting the guidance scale, you can control the balance between fidelity to the prompt and diversity of the output. Lower guidance scales may result in more unexpected and imaginative images, while higher scales will produce outputs that are closer to the specified prompt.
Updated Invalid Date
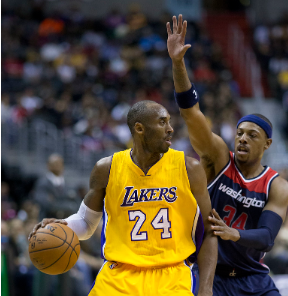
cogvlm

584
CogVLM is a powerful open-source visual language model developed by the maintainer cjwbw. It comprises a vision transformer encoder, an MLP adapter, a pretrained large language model (GPT), and a visual expert module. CogVLM-17B has 10 billion vision parameters and 7 billion language parameters, and it achieves state-of-the-art performance on 10 classic cross-modal benchmarks, including NoCaps, Flicker30k captioning, RefCOCO, and more. It can also engage in conversational interactions about images. Similar models include segmind-vega, an open-source distilled Stable Diffusion model with 100% speedup, animagine-xl-3.1, an anime-themed text-to-image Stable Diffusion model, cog-a1111-ui, a collection of anime Stable Diffusion models, and videocrafter, a text-to-video and image-to-video generation and editing model. Model inputs and outputs CogVLM is a powerful visual language model that can accept both text and image inputs. It can generate detailed image descriptions, answer various types of visual questions, and even engage in multi-turn conversations about images. Inputs Image**: The input image that CogVLM will process and generate a response for. Query**: The text prompt or question that CogVLM will use to generate a response related to the input image. Outputs Text response**: The generated text response from CogVLM based on the input image and query. Capabilities CogVLM is capable of accurately describing images in detail with very few hallucinations. It can understand and answer various types of visual questions, and it has a visual grounding version that can ground the generated text to specific regions of the input image. CogVLM sometimes captures more detailed content than GPT-4V(ision). What can I use it for? With its powerful visual and language understanding capabilities, CogVLM can be used for a variety of applications, such as image captioning, visual question answering, image-based dialogue systems, and more. Developers and researchers can leverage CogVLM to build advanced multimodal AI systems that can effectively process and understand both visual and textual information. Things to try One interesting aspect of CogVLM is its ability to engage in multi-turn conversations about images. You can try providing a series of related queries about a single image and observe how the model responds and maintains context throughout the conversation. Additionally, you can experiment with different prompting strategies to see how CogVLM performs on various visual understanding tasks, such as detailed image description, visual reasoning, and visual grounding.
Updated Invalid Date
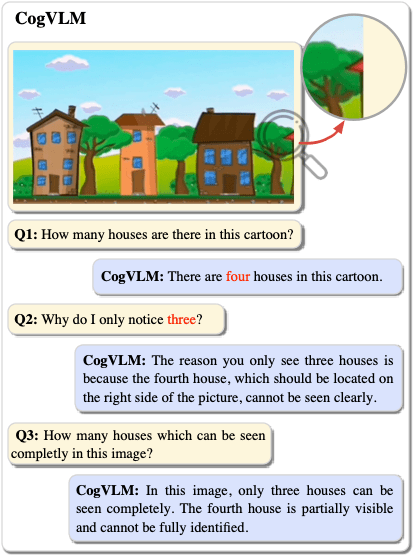
cogvlm

12
cogvlm is a powerful open-source visual language model (VLM) developed by the team at Tsinghua University. Compared to similar visual-language models like cogvlm and llava-13b, cogvlm stands out with its state-of-the-art performance on a wide range of cross-modal benchmarks, including NoCaps, Flickr30k captioning, and various visual question answering tasks. The model has 10 billion visual parameters and 7 billion language parameters, allowing it to understand and generate detailed descriptions of images. Unlike some previous VLMs that struggled with hallucination, cogvlm is known for its ability to provide accurate and factual information about the visual content. Model Inputs and Outputs Inputs Image**: An image in a standard image format (e.g. JPEG, PNG) provided as a URL. Prompt**: A text prompt describing the task or question to be answered about the image. Outputs Output**: An array of strings, where each string represents the model's response to the provided prompt and image. Capabilities cogvlm excels at a variety of visual understanding and reasoning tasks. It can provide detailed descriptions of images, answer complex visual questions, and even perform visual grounding - identifying and localizing specific objects or elements in an image based on a textual description. For example, when shown an image of a park scene and asked "Can you describe what you see in the image?", cogvlm might respond with a detailed paragraph capturing the key elements, such as the lush green grass, the winding gravel path, the trees in the distance, and the clear blue sky overhead. Similarly, if presented with an image of a kitchen and the prompt "Where is the microwave located in the image?", cogvlm would be able to identify the microwave's location and provide the precise bounding box coordinates. What Can I Use It For? The broad capabilities of cogvlm make it a versatile tool for a wide range of applications. Developers and researchers could leverage the model for tasks such as: Automated image captioning and visual question answering for media or educational content Visual interface agents that can understand and interact with graphical user interfaces Multimodal search and retrieval systems that can match images to relevant textual information Visual data analysis and reporting, where the model can extract insights from visual data By tapping into cogvlm's powerful visual understanding, these applications can offer more natural and intuitive experiences for users. Things to Try One interesting way to explore cogvlm's capabilities is to try various types of visual prompts and see how the model responds. For example, you could provide complex scenes with multiple objects and ask the model to identify and localize specific elements. Or you could give it abstract or artistic images and see how it interprets and describes the visual content. Another interesting avenue to explore is the model's ability to handle visual grounding tasks. By providing textual descriptions of objects or elements in an image, you can test how accurately cogvlm can pinpoint their locations and extents. Ultimately, the breadth of cogvlm's visual understanding makes it a valuable tool for a wide range of applications. As you experiment with the model, be sure to share your findings and insights with the broader AI community.
Updated Invalid Date
🤷
glm-4v-9b

154
glm-4v-9b is a large language model developed by THUDM, a leading AI research group. It is part of the GLM (General Language Model) family, which aims to create open, bilingual language models capable of strong performance across a wide range of tasks. The glm-4v-9b model builds upon the successes of earlier GLM models, incorporating advanced techniques like autoregressive blank infilling and hybrid pretraining objectives. This allows it to achieve impressive results on benchmarks like MMBench-EN-Test, MMBench-CN-Test, and SEEDBench_IMG, outperforming models like GPT-4-turbo-2024-04-09, Gemini 1.0, and Qwen-VL-Max. Compared to similar large language models, glm-4v-9b stands out for its strong multilingual and multimodal capabilities. It can seamlessly handle both English and Chinese, and has been trained to integrate visual information with text, making it well-suited for tasks like image captioning and visual question answering. Model Inputs and Outputs Inputs Text**: The model can accept text input in the form of a conversation, with the user's message formatted as {"role": "user", "content": "query"}. Images**: Along with text, the model can also take image inputs, which are passed through the tokenizer using the image field in the input template. Outputs Text Response**: The model will generate a text response to the provided input, which can be retrieved by decoding the model's output tokens. Conversation History**: The model maintains a conversation history, which can be passed back into the model to continue the dialogue in a coherent manner. Capabilities The glm-4v-9b model has demonstrated strong performance on a wide range of benchmarks, particularly those testing multilingual and multimodal capabilities. For example, it achieves high scores on the MMBench-EN-Test (81.1), MMBench-CN-Test (79.4), and SEEDBench_IMG (76.8) tasks, showcasing its ability to understand and generate text in both English and Chinese, as well as integrate visual information. Additionally, the model has shown promising results on tasks like MMLU (58.7), AI2D (81.1), and OCRBench (786), indicating its potential for applications in areas like question answering, image understanding, and optical character recognition. What Can I Use It For? The glm-4v-9b model's strong multilingual and multimodal capabilities make it a versatile tool for a variety of applications. Some potential use cases include: Intelligent Assistants**: The model's ability to engage in natural language conversations, while also understanding and generating content related to images, makes it well-suited for building advanced virtual assistants that can handle a wide range of user requests. Multimodal Content Generation**: Leveraging the model's text-image integration capabilities, developers can create applications that generate multimedia content, such as image captions, visual narratives, or even animated stories. Multilingual Language Understanding**: Organizations operating in diverse language environments can use glm-4v-9b to build applications that can seamlessly handle both English and Chinese, enabling improved cross-cultural communication and collaboration. Research and Development**: As an open-source model, glm-4v-9b can be a valuable resource for AI researchers and developers looking to explore the latest advancements in large language models and multimodal learning. Things to Try One key feature of the glm-4v-9b model is its ability to effectively utilize both textual and visual information. Developers and researchers can experiment with incorporating image data into their applications, exploring how the model's multimodal capabilities can enhance tasks like image captioning, visual question answering, or even image-guided text generation. Another avenue to explore is the model's strong multilingual performance. Users can try interacting with the model in both English and Chinese, and observe how it maintains coherence and contextual understanding across languages. This can lead to insights on building truly global AI systems that can bridge language barriers. Finally, the model's impressive benchmark scores suggest that it could be a valuable starting point for fine-tuning or further pretraining on domain-specific datasets. Developers can experiment with adapting the model to their particular use cases, unlocking new capabilities and expanding the model's utility.
Updated Invalid Date