minigpt-4
Maintainer: daanelson

1.3K
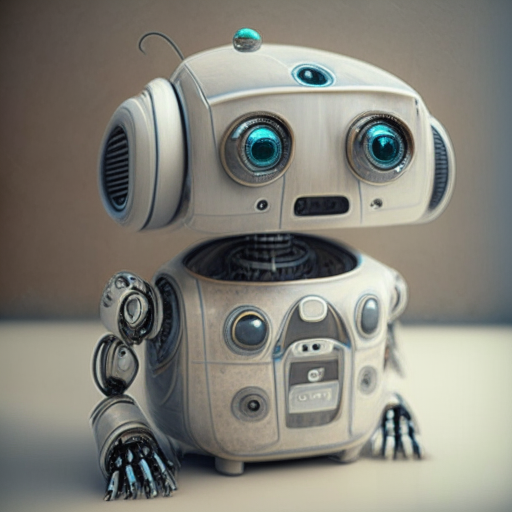
Property | Value |
---|---|
Model Link | View on Replicate |
API Spec | View on Replicate |
Github Link | View on Github |
Paper Link | View on Arxiv |
Create account to get full access
Model overview
minigpt-4
is a model that generates text in response to an input image and prompt. It was developed by Deyao Zhu, Jun Chen, Xiaoqian Shen, Xiang Li, and Mohamed Elhoseiny at King Abdullah University of Science and Technology. minigpt-4
aligns a frozen visual encoder from BLIP-2 with a frozen large language model, Vicuna, using just one projection layer. This allows the model to understand images and generate coherent, user-friendly text in response.
The model's capabilities are similar to those demonstrated in GPT-4, with the ability to perform a variety of vision-language tasks like image captioning, visual question answering, and story generation. It is a compact and efficient model that can run on a single A100 GPU, making it accessible for a wide range of users.
Model inputs and outputs
Inputs
- image: The image to discuss, provided as a URL.
- prompt: The text prompt to guide the model's generation.
- num_beams: The number of beams to use for beam search decoding.
- max_length: The maximum length of the prompt and output combined, in tokens.
- temperature: The temperature for generating tokens, where lower values result in more predictable outputs.
- max_new_tokens: The maximum number of new tokens to generate.
- repetition_penalty: The penalty for repeated words in the generated text, where values greater than 1 discourage repetition.
Outputs
- Output: The text generated by the model in response to the input image and prompt.
Capabilities
minigpt-4
demonstrates a range of vision-language capabilities, including image captioning, visual question answering, and story generation. For example, when provided an image of a wild animal and the prompt "Describe what you see in the image", the model can generate a detailed description of the animal's features and behavior. Similarly, when given an image and a prompt asking to "Write a short story about this image", the model can produce a coherent, imaginative narrative.
What can I use it for?
minigpt-4
could be useful for a variety of applications that involve generating text based on visual input, such as:
- Automated image captioning for social media or e-commerce
- Visual question answering for educational or assistive applications
- Story generation for creative writing or game development
- Generating text-based descriptions of product images
The model's compact size and efficient performance make it a potentially accessible option for developers and researchers looking to incorporate vision-language capabilities into their projects.
Things to try
One interesting aspect of minigpt-4
is its ability to generate text that is closely tied to the input image, rather than just producing generic responses. For example, if you provide an image of a cityscape and ask the model to "Describe what you see", it will generate a response that is specific to the details and features of that particular scene, rather than giving a generic description of a cityscape.
You can also experiment with providing the model with more open-ended prompts, like "Write a short story inspired by this image" or "Discuss the emotions conveyed in this image". This can lead to more creative and imaginative outputs that go beyond simple descriptive tasks.
This summary was produced with help from an AI and may contain inaccuracies - check out the links to read the original source documents!
Related Models
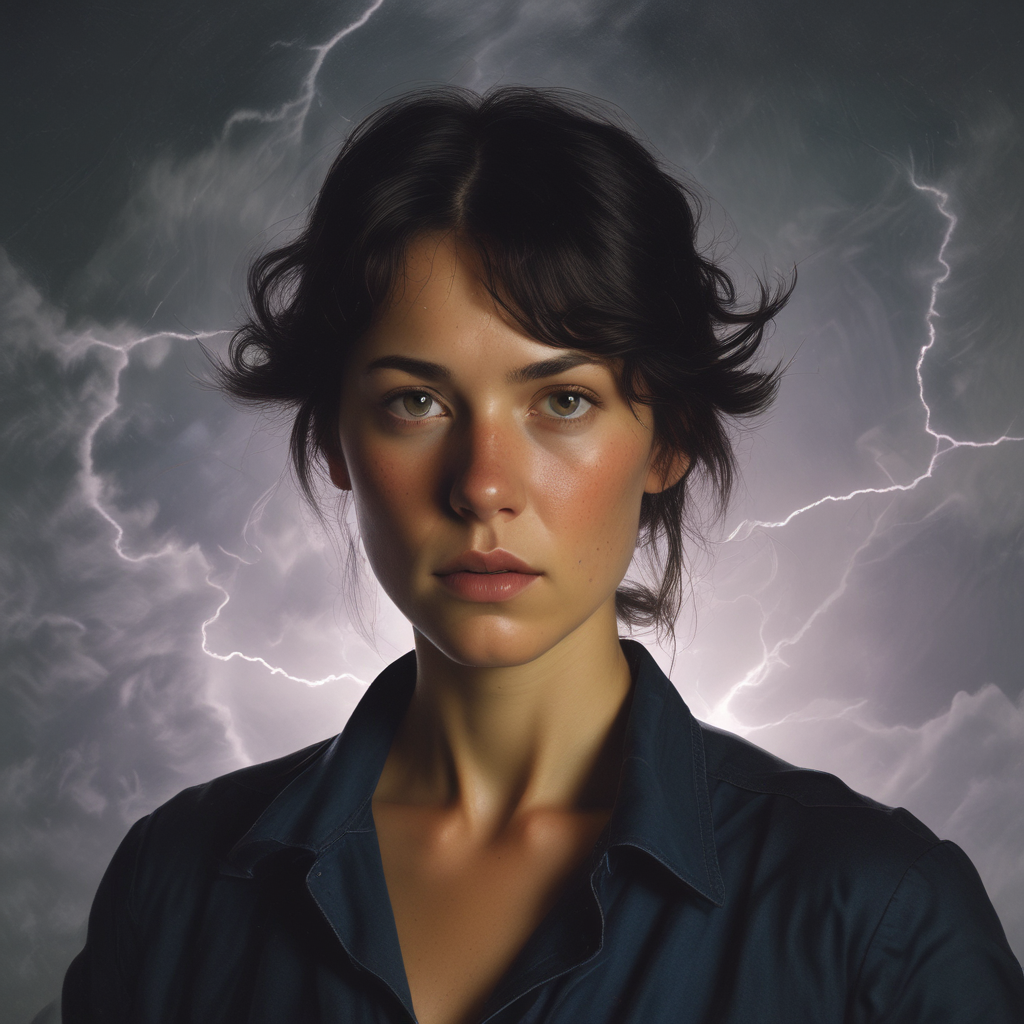
sdxl-lightning-4step

158.8K
sdxl-lightning-4step is a fast text-to-image model developed by ByteDance that can generate high-quality images in just 4 steps. It is similar to other fast diffusion models like AnimateDiff-Lightning and Instant-ID MultiControlNet, which also aim to speed up the image generation process. Unlike the original Stable Diffusion model, these fast models sacrifice some flexibility and control to achieve faster generation times. Model inputs and outputs The sdxl-lightning-4step model takes in a text prompt and various parameters to control the output image, such as the width, height, number of images, and guidance scale. The model can output up to 4 images at a time, with a recommended image size of 1024x1024 or 1280x1280 pixels. Inputs Prompt**: The text prompt describing the desired image Negative prompt**: A prompt that describes what the model should not generate Width**: The width of the output image Height**: The height of the output image Num outputs**: The number of images to generate (up to 4) Scheduler**: The algorithm used to sample the latent space Guidance scale**: The scale for classifier-free guidance, which controls the trade-off between fidelity to the prompt and sample diversity Num inference steps**: The number of denoising steps, with 4 recommended for best results Seed**: A random seed to control the output image Outputs Image(s)**: One or more images generated based on the input prompt and parameters Capabilities The sdxl-lightning-4step model is capable of generating a wide variety of images based on text prompts, from realistic scenes to imaginative and creative compositions. The model's 4-step generation process allows it to produce high-quality results quickly, making it suitable for applications that require fast image generation. What can I use it for? The sdxl-lightning-4step model could be useful for applications that need to generate images in real-time, such as video game asset generation, interactive storytelling, or augmented reality experiences. Businesses could also use the model to quickly generate product visualization, marketing imagery, or custom artwork based on client prompts. Creatives may find the model helpful for ideation, concept development, or rapid prototyping. Things to try One interesting thing to try with the sdxl-lightning-4step model is to experiment with the guidance scale parameter. By adjusting the guidance scale, you can control the balance between fidelity to the prompt and diversity of the output. Lower guidance scales may result in more unexpected and imaginative images, while higher scales will produce outputs that are closer to the specified prompt.
Updated Invalid Date
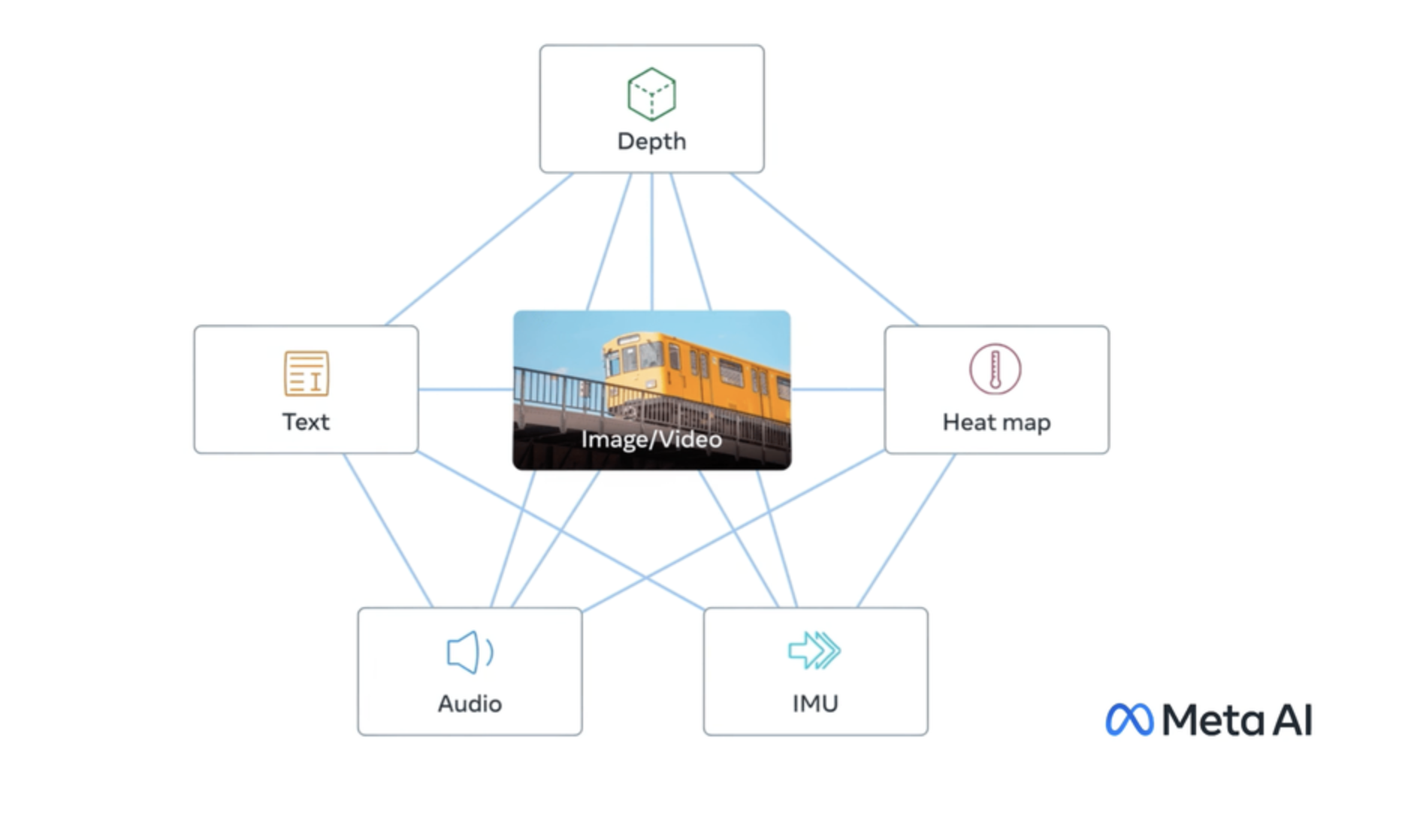
imagebind

2.0K
ImageBind is a model developed by researchers at FAIR, Meta AI that learns a joint embedding across six different modalities - images, text, audio, depth, thermal, and IMU data. This allows it to perform novel "emergent" applications like cross-modal retrieval, composing modalities with arithmetic, cross-modal detection and generation. The model outperforms many existing single-modality models on zero-shot classification tasks across a range of datasets, demonstrating its ability to effectively represent and relate information from diverse inputs. Model inputs and outputs ImageBind takes in data from various modalities - text, images, audio, depth, thermal, and IMU sensors. The inputs are preprocessed and transformed before being fed into the model. The model then outputs a single, unified embedding that captures the semantic relationships between the different modalities. Inputs Text**: Text input in the form of a string Vision**: Image data in the form of image file paths Audio**: Audio data in the form of audio file paths Outputs Embedding**: A high-dimensional vector representing the input data in a shared embedding space Capabilities ImageBind demonstrates impressive zero-shot classification performance on a range of datasets, including ImageNet, Kinetics-400, NYU-D, ESC, and LLVIP. This indicates that the model is able to effectively represent and relate information from diverse inputs, and can generalize to new tasks and datasets without extensive fine-tuning. What can I use it for? The cross-modal capabilities of ImageBind enable novel applications like cross-modal retrieval, where you can search for images using text queries or vice versa. The model can also be used to compose modalities with arithmetic, allowing you to generate new content by combining text, images, and audio in interesting ways. Additionally, ImageBind can be used for cross-modal detection and generation tasks, expanding the possibilities for multimodal AI systems. Things to try One interesting aspect of ImageBind is its ability to learn a shared embedding space across diverse modalities. This allows you to explore the relationships between different types of data, such as how textual descriptions relate to visual and audio representations of the same concepts. You could experiment with tasks like zero-shot classification, cross-modal retrieval, or even generating new content by combining modalities in novel ways.
Updated Invalid Date
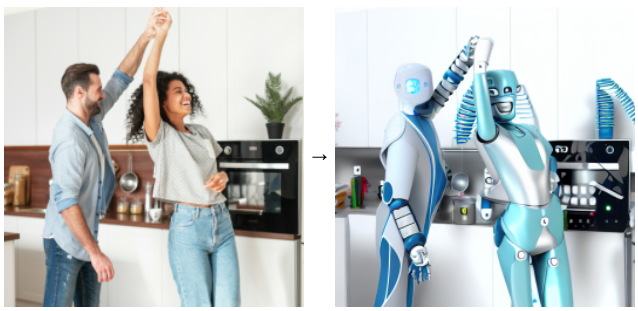
plug_and_play_image_translation

6
plug_and_play_image_translation is a model developed by daanelson that enables editing an image using features from diffusion models. It builds upon the capabilities of models like stable-diffusion and stable-diffusion-inpainting by allowing users to selectively apply diffusion features to an input image to achieve specific edits. Model inputs and outputs Inputs Input Image**: The image to edit. Generation Prompt**: A text prompt that can be used to generate an image instead of providing an input image. Translation Prompts**: A set of text prompts that will be used to guide the image translation process. Negative Prompt**: Text to control the level of deviation from the source image. Scale**: The unconditional guidance scale, which determines how much the model should deviate from the source image. Negative Prompt Alpha**: The strength of the negative prompt's effect, with lower values being stronger. Feature Injection Threshold**: The timestep at which to stop injecting the saved features into the translation diffusion process, controlling the level of structure preservation. Outputs A set of translated images, one for each of the provided translation prompts. Capabilities plug_and_play_image_translation allows users to selectively apply diffusion features from pre-trained models like stable-diffusion to an input image, enabling a range of editing capabilities. This can be used to make targeted changes to an image while preserving the overall structure and composition. What can I use it for? plug_and_play_image_translation can be used for a variety of image editing tasks, such as generating variations of an existing image, combining elements from different images, or making specific changes to an image while maintaining its overall appearance. The ability to control the level of structure preservation and deviation from the source image makes it a versatile tool for creative workflows. Things to try One interesting aspect of plug_and_play_image_translation is the ability to control the level of structure preservation through the feature_injection_threshold parameter. By adjusting this value, you can find a balance between preserving the original image's composition and introducing new elements from the diffusion features. Additionally, experimenting with different translation prompts and negative prompts can help you achieve a wide range of creative effects.
Updated Invalid Date
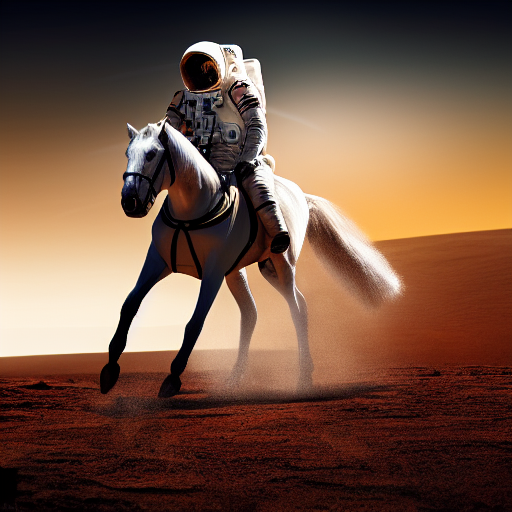
stable-diffusion

108.2K
Stable Diffusion is a latent text-to-image diffusion model capable of generating photo-realistic images given any text input. Developed by Stability AI, it is an impressive AI model that can create stunning visuals from simple text prompts. The model has several versions, with each newer version being trained for longer and producing higher-quality images than the previous ones. The main advantage of Stable Diffusion is its ability to generate highly detailed and realistic images from a wide range of textual descriptions. This makes it a powerful tool for creative applications, allowing users to visualize their ideas and concepts in a photorealistic way. The model has been trained on a large and diverse dataset, enabling it to handle a broad spectrum of subjects and styles. Model inputs and outputs Inputs Prompt**: The text prompt that describes the desired image. This can be a simple description or a more detailed, creative prompt. Seed**: An optional random seed value to control the randomness of the image generation process. Width and Height**: The desired dimensions of the generated image, which must be multiples of 64. Scheduler**: The algorithm used to generate the image, with options like DPMSolverMultistep. Num Outputs**: The number of images to generate (up to 4). Guidance Scale**: The scale for classifier-free guidance, which controls the trade-off between image quality and faithfulness to the input prompt. Negative Prompt**: Text that specifies things the model should avoid including in the generated image. Num Inference Steps**: The number of denoising steps to perform during the image generation process. Outputs Array of image URLs**: The generated images are returned as an array of URLs pointing to the created images. Capabilities Stable Diffusion is capable of generating a wide variety of photorealistic images from text prompts. It can create images of people, animals, landscapes, architecture, and more, with a high level of detail and accuracy. The model is particularly skilled at rendering complex scenes and capturing the essence of the input prompt. One of the key strengths of Stable Diffusion is its ability to handle diverse prompts, from simple descriptions to more creative and imaginative ideas. The model can generate images of fantastical creatures, surreal landscapes, and even abstract concepts with impressive results. What can I use it for? Stable Diffusion can be used for a variety of creative applications, such as: Visualizing ideas and concepts for art, design, or storytelling Generating images for use in marketing, advertising, or social media Aiding in the development of games, movies, or other visual media Exploring and experimenting with new ideas and artistic styles The model's versatility and high-quality output make it a valuable tool for anyone looking to bring their ideas to life through visual art. By combining the power of AI with human creativity, Stable Diffusion opens up new possibilities for visual expression and innovation. Things to try One interesting aspect of Stable Diffusion is its ability to generate images with a high level of detail and realism. Users can experiment with prompts that combine specific elements, such as "a steam-powered robot exploring a lush, alien jungle," to see how the model handles complex and imaginative scenes. Additionally, the model's support for different image sizes and resolutions allows users to explore the limits of its capabilities. By generating images at various scales, users can see how the model handles the level of detail and complexity required for different use cases, such as high-resolution artwork or smaller social media graphics. Overall, Stable Diffusion is a powerful and versatile AI model that offers endless possibilities for creative expression and exploration. By experimenting with different prompts, settings, and output formats, users can unlock the full potential of this cutting-edge text-to-image technology.
Updated Invalid Date