real-basicvsr-video-superresolution
Maintainer: pollinations

8
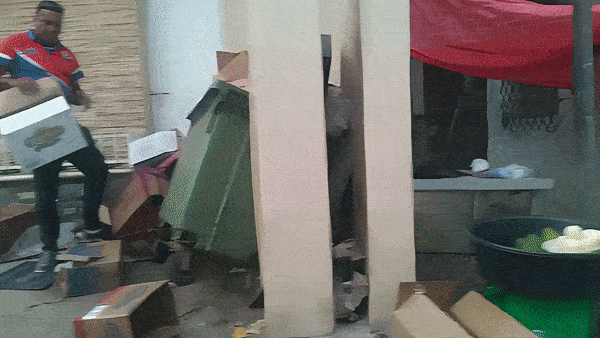
Property | Value |
---|---|
Model Link | View on Replicate |
API Spec | View on Replicate |
Github Link | View on Github |
Paper Link | View on Arxiv |
Get summaries of the top AI models delivered straight to your inbox:
Model overview
The real-basicvsr-video-superresolution
model, created by pollinations, is a video super-resolution model that aims to address the challenges of real-world video super-resolution. It is part of the MMEditing open-source toolbox, which provides state-of-the-art methods for various image and video editing tasks. The model is designed to enhance low-resolution video frames while preserving realistic details and textures, making it suitable for a wide range of applications, from video production to video surveillance.
Similar models in the MMEditing toolbox include SeeSR, which focuses on semantics-aware real-world image super-resolution, Swin2SR, a high-performance image super-resolution model, and RefVSR, which uses a reference video frame to super-resolve an input low-resolution video frame.
Model inputs and outputs
The real-basicvsr-video-superresolution
model takes a low-resolution video as input and generates a high-resolution version of the same video as output. The input video can be of various resolutions and frame rates, and the model will upscale it to a higher quality while preserving the original temporal information.
Inputs
- Video: The low-resolution input video to be super-resolved.
Outputs
- Output Video: The high-resolution video generated by the model, with improved details and texture.
Capabilities
The real-basicvsr-video-superresolution
model is designed to address the challenges of real-world video super-resolution, where the input video may have various degradations such as noise, blur, and compression artifacts. The model leverages the capabilities of the BasicVSR++ architecture, which was introduced in the CVPR 2022 paper "Towards Real-World Video Super-Resolution: A New Benchmark and a State-of-the-Art Model". By incorporating insights from this research, the real-basicvsr-video-superresolution
model is able to produce high-quality, realistic video outputs even from low-quality input footage.
What can I use it for?
The real-basicvsr-video-superresolution
model can be used in a variety of applications where high-quality video is needed, such as video production, video surveillance, and video streaming. For example, it could be used to upscale security camera footage to improve visibility and detail, or to enhance the resolution of old family videos for a more immersive viewing experience. Additionally, the model could be integrated into video editing workflows to improve the quality of low-res footage or to create high-resolution versions of existing videos.
Things to try
One interesting aspect of the real-basicvsr-video-superresolution
model is its ability to handle a wide range of input video resolutions and frame rates. This makes it a versatile tool that can be applied to a variety of real-world video sources, from low-quality smartphone footage to professional-grade video. Users could experiment with feeding the model different types of input videos, such as those with varying levels of noise, blur, or compression, and observe how the model responds and the quality of the output. Additionally, users could try combining the real-basicvsr-video-superresolution
model with other video processing techniques, such as video stabilization or color grading, to further enhance the final output.
This summary was produced with help from an AI and may contain inaccuracies - check out the links to read the original source documents!
Related Models
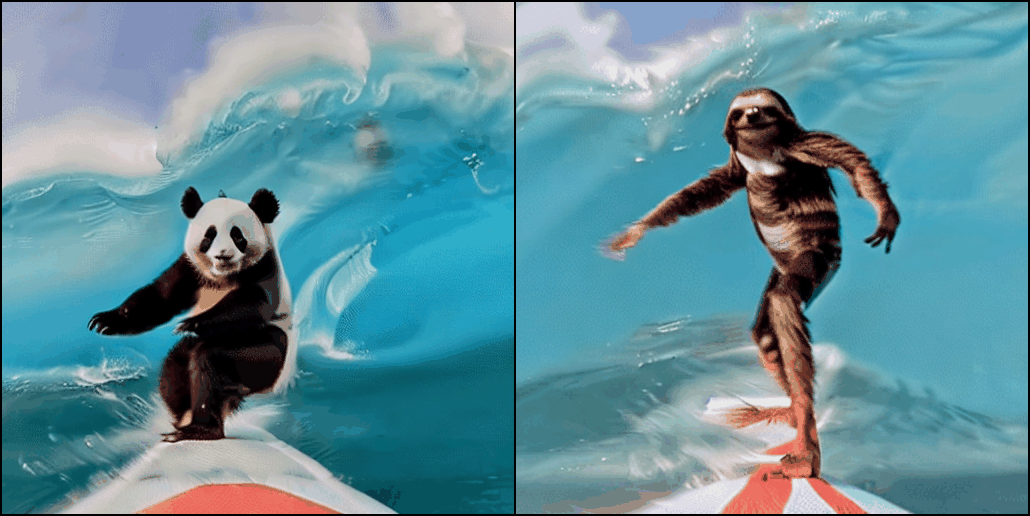
tune-a-video

2
Tune-A-Video is an AI model developed by the team at Pollinations, known for creating innovative AI models like AMT, BARK, Music-Gen, and Lucid Sonic Dreams XL. Tune-A-Video is a one-shot tuning approach that allows users to fine-tune text-to-image diffusion models, like Stable Diffusion, for text-to-video generation. Model inputs and outputs Tune-A-Video takes in a source video, a source prompt describing the video, and target prompts that you want to change the video to. It then fine-tunes the text-to-image diffusion model to generate a new video matching the target prompts. The output is a video with the requested changes. Inputs Video**: The input video you want to modify Source Prompt**: A prompt describing the original video Target Prompts**: Prompts describing the desired changes to the video Outputs Output Video**: The modified video matching the target prompts Capabilities Tune-A-Video enables users to quickly adapt text-to-image models like Stable Diffusion for text-to-video generation with just a single example video. This allows for the creation of custom video content tailored to specific prompts, without the need for lengthy fine-tuning on large video datasets. What can I use it for? With Tune-A-Video, you can generate custom videos for a variety of applications, such as creating personalized content, developing educational materials, or producing marketing videos. The ability to fine-tune the model with a single example video makes it particularly useful for rapid prototyping and iterating on video ideas. Things to try Some interesting things to try with Tune-A-Video include: Generating videos of your favorite characters or objects in different scenarios Modifying existing videos to change the style, setting, or actions Experimenting with prompts to see how the model can transform the video in unique ways Combining Tune-A-Video with other AI models like BARK for audio-visual content creation By leveraging the power of one-shot tuning, Tune-A-Video opens up new possibilities for personalized and creative video generation.
Updated Invalid Date
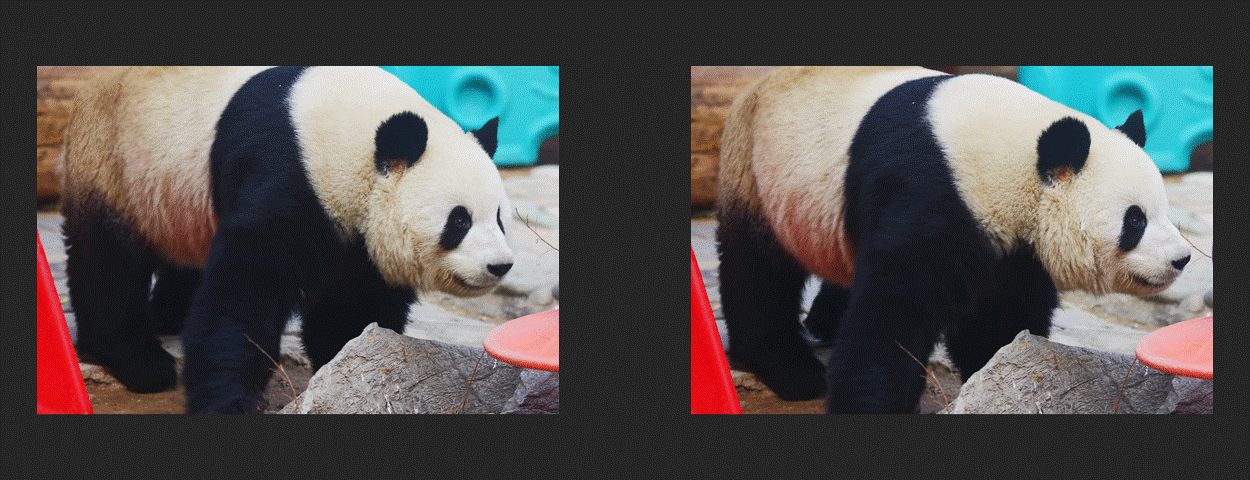
amt

213
AMT is a lightweight, fast, and accurate algorithm for Frame Interpolation developed by researchers at Nankai University. It aims to provide practical solutions for video generation from a few given frames (at least two frames). AMT is similar to models like rembg-enhance, stable-video-diffusion, gfpgan, and stable-diffusion-inpainting in its focus on image and video processing tasks. However, AMT is specifically designed for efficient frame interpolation, which can be useful for a variety of video-related applications. Model inputs and outputs The AMT model takes in a set of input frames (at least two) and generates intermediate frames to create a smoother, more fluid video. The model is capable of handling both fixed and arbitrary frame rates, making it suitable for a range of video processing needs. Inputs Video**: The input video or set of images to be interpolated. Model Type**: The specific version of the AMT model to use, such as amt-l or amt-s. Output Video Fps**: The desired output frame rate for the interpolated video. Recursive Interpolation Passes**: The number of times to recursively interpolate the frames to achieve the desired output. Outputs Output**: The interpolated video with the specified frame rate. Capabilities AMT is designed to be a highly efficient and accurate frame interpolation model. It can generate smooth, high-quality intermediate frames between input frames, resulting in more fluid and natural-looking videos. The model's performance has been demonstrated on various datasets, including Vimeo90k and GoPro. What can I use it for? The AMT model can be useful for a variety of video-related applications, such as video generation, slow-motion creation, and frame rate upscaling. For example, you could use AMT to generate high-quality slow-motion footage from your existing videos, or to create smooth transitions between video frames for more visually appealing content. Things to try One interesting thing to try with AMT is to experiment with the different model types and the number of recursive interpolation passes. By adjusting these settings, you can find the right balance between output quality and computational efficiency for your specific use case. Additionally, you can try combining AMT with other video processing techniques, such as AnimateDiff-Lightning, to achieve even more advanced video effects.
Updated Invalid Date
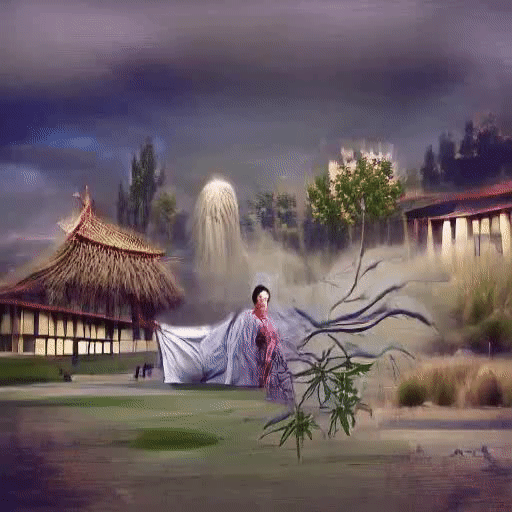
adampi

5
The adampi model, developed by the team at Pollinations, is a powerful AI tool that can create 3D photos from single in-the-wild 2D images. This model is based on the Adaptive Multiplane Images (AdaMPI) technique, which was recently published in the SIGGRAPH 2022 paper "Single-View View Synthesis in the Wild with Learned Adaptive Multiplane Images". The adampi model is capable of handling diverse scene layouts and producing high-quality 3D content from a single input image. Model inputs and outputs The adampi model takes a single 2D image as input and generates a 3D photo as output. This allows users to transform ordinary 2D photos into immersive 3D experiences, adding depth and perspective to the original image. Inputs Image**: A 2D image in standard image format (e.g. JPEG, PNG) Outputs 3D Photo**: A 3D representation of the input image, which can be viewed and interacted with from different perspectives. Capabilities The adampi model is designed to tackle the challenge of synthesizing novel views for in-the-wild photographs, where scenes can have complex 3D geometry. By leveraging the Adaptive Multiplane Images (AdaMPI) representation, the model is able to adjust the initial plane positions and predict depth-aware color and density for each plane, allowing it to produce high-quality 3D content from a single input image. What can I use it for? The adampi model can be used to create immersive 3D experiences from ordinary 2D photos, opening up new possibilities for photographers, content creators, and virtual reality applications. For example, you could use the model to transform family photos, travel snapshots, or artwork into 3D scenes that can be viewed and explored from different angles. This could enhance the viewing experience, add depth and perspective, and even enable new creative possibilities. Things to try One interesting aspect of the adampi model is its ability to handle diverse scene layouts in the wild. Try experimenting with a variety of input images, from landscapes and cityscapes to portraits and still lifes, and see how the model adapts to the different scene geometries. You could also explore the depth-aware color and density predictions, and how they contribute to the final 3D output.
Updated Invalid Date
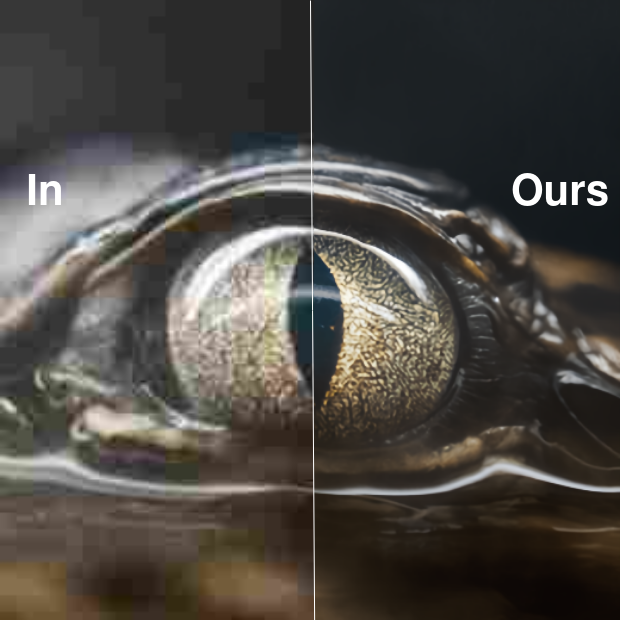
swin2sr

3.5K
swin2sr is a state-of-the-art AI model for photorealistic image super-resolution and restoration, developed by the mv-lab research team. It builds upon the success of the SwinIR model by incorporating the novel Swin Transformer V2 architecture, which improves training convergence and performance, especially for compressed image super-resolution tasks. The model outperforms other leading solutions in classical, lightweight, and real-world image super-resolution, JPEG compression artifact reduction, and compressed input super-resolution. It was a top-5 solution in the "AIM 2022 Challenge on Super-Resolution of Compressed Image and Video". Similar models in the image restoration and enhancement space include supir, stable-diffusion, instructir, gfpgan, and seesr. Model inputs and outputs swin2sr takes low-quality, low-resolution JPEG compressed images as input and generates high-quality, high-resolution images as output. The model can upscale the input by a factor of 2, 4, or other scales, depending on the task. Inputs Low-quality, low-resolution JPEG compressed images Outputs High-quality, high-resolution images with reduced compression artifacts and enhanced visual details Capabilities swin2sr can effectively tackle various image restoration and enhancement tasks, including: Classical image super-resolution Lightweight image super-resolution Real-world image super-resolution JPEG compression artifact reduction Compressed input super-resolution The model's excellent performance is achieved through the use of the Swin Transformer V2 architecture, which improves training stability and data efficiency compared to previous transformer-based approaches like SwinIR. What can I use it for? swin2sr can be particularly useful in applications where image quality and resolution are crucial, such as: Enhancing images for high-resolution displays and printing Improving image quality for streaming services and video conferencing Restoring old or damaged photos Generating high-quality images for virtual reality and gaming The model's ability to handle compressed input super-resolution makes it a valuable tool for efficient image and video transmission and storage in bandwidth-limited systems. Things to try One interesting aspect of swin2sr is its potential to be used in combination with other image processing and generation models, such as instructir or stable-diffusion. By integrating swin2sr into a workflow that starts with text-to-image generation or semantic-aware image manipulation, users can achieve even more impressive and realistic results. Additionally, the model's versatility in handling various image restoration tasks makes it a valuable tool for researchers and developers working on computational photography, low-level vision, and image signal processing applications.
Updated Invalid Date