refvsr-cvpr2022
Maintainer: codeslake

14
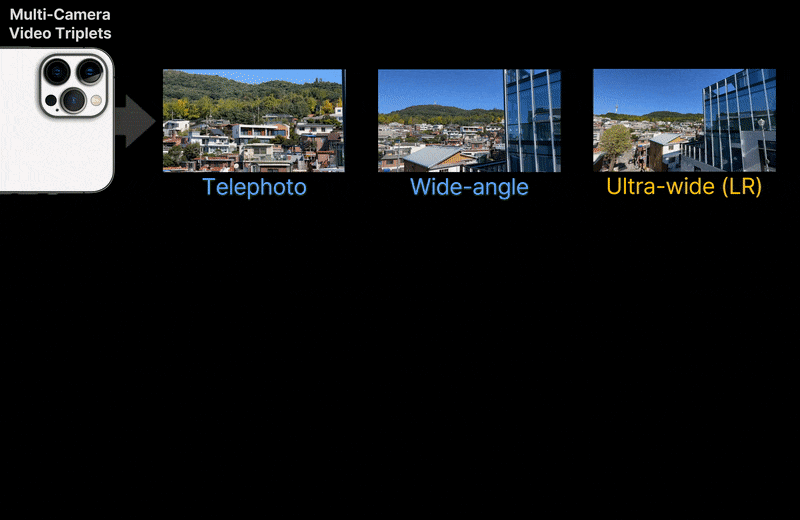
Property | Value |
---|---|
Run this model | Run on Replicate |
API spec | View on Replicate |
Github link | View on Github |
Paper link | View on Arxiv |
Create account to get full access
Model overview
refvsr-cvpr2022
is a reference-based video super-resolution model developed by codeslake and presented at CVPR 2022. It improves on existing video super-resolution approaches by using a reference wide-angle video frame to super-resolve an ultra-wide low-resolution video frame. This is useful for scenarios where a wide-angle and ultra-wide camera are available, like in action cameras or smartphones. Similar models include lcm-video2video, which uses a latent consistency model for fast video-to-video translation, and arbsr, which can perform scale-arbitrary super-resolution.
Model inputs and outputs
refvsr-cvpr2022
takes two inputs: a low-resolution ultra-wide video frame (LR
) and a reference wide-angle video frame (Ref
). It outputs the super-resolved ultra-wide video frame (SR_output
), using the reference frame to guide the super-resolution process.
Inputs
- LR: Low-resolution ultra-wide video frame to super-resolve
- Ref: Reference wide-angle video frame
Outputs
- SR_output: Super-resolved ultra-wide video frame
Capabilities
refvsr-cvpr2022
can perform high-quality 4x video super-resolution, significantly improving the resolution of ultra-wide video frames by leveraging a wide-angle reference frame. This enables applications like high-resolution action cameras or smartphone videos without the need for expensive ultra-wide sensors.
What can I use it for?
The refvsr-cvpr2022
model can be used to enhance the quality of ultra-wide video footage, such as from action cameras or smartphones, by super-resolving the frames using a reference wide-angle video. This can be useful for content creators, video production companies, or anyone looking to improve the resolution of their ultra-wide video without expensive hardware upgrades.
Things to try
One interesting thing to try with refvsr-cvpr2022
is exploring the impact of the reference frame quality and field of view on the super-resolution performance. The model's ability to leverage the reference frame is a key aspect, so experimenting with different reference video conditions could yield insights into the model's capabilities and limitations.
This summary was produced with help from an AI and may contain inaccuracies - check out the links to read the original source documents!
Related Models
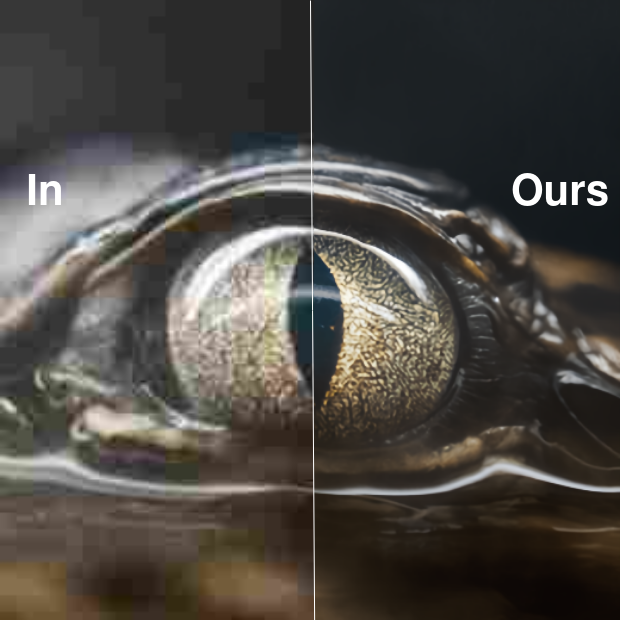
swin2sr

3.5K
swin2sr is a state-of-the-art AI model for photorealistic image super-resolution and restoration, developed by the mv-lab research team. It builds upon the success of the SwinIR model by incorporating the novel Swin Transformer V2 architecture, which improves training convergence and performance, especially for compressed image super-resolution tasks. The model outperforms other leading solutions in classical, lightweight, and real-world image super-resolution, JPEG compression artifact reduction, and compressed input super-resolution. It was a top-5 solution in the "AIM 2022 Challenge on Super-Resolution of Compressed Image and Video". Similar models in the image restoration and enhancement space include supir, stable-diffusion, instructir, gfpgan, and seesr. Model inputs and outputs swin2sr takes low-quality, low-resolution JPEG compressed images as input and generates high-quality, high-resolution images as output. The model can upscale the input by a factor of 2, 4, or other scales, depending on the task. Inputs Low-quality, low-resolution JPEG compressed images Outputs High-quality, high-resolution images with reduced compression artifacts and enhanced visual details Capabilities swin2sr can effectively tackle various image restoration and enhancement tasks, including: Classical image super-resolution Lightweight image super-resolution Real-world image super-resolution JPEG compression artifact reduction Compressed input super-resolution The model's excellent performance is achieved through the use of the Swin Transformer V2 architecture, which improves training stability and data efficiency compared to previous transformer-based approaches like SwinIR. What can I use it for? swin2sr can be particularly useful in applications where image quality and resolution are crucial, such as: Enhancing images for high-resolution displays and printing Improving image quality for streaming services and video conferencing Restoring old or damaged photos Generating high-quality images for virtual reality and gaming The model's ability to handle compressed input super-resolution makes it a valuable tool for efficient image and video transmission and storage in bandwidth-limited systems. Things to try One interesting aspect of swin2sr is its potential to be used in combination with other image processing and generation models, such as instructir or stable-diffusion. By integrating swin2sr into a workflow that starts with text-to-image generation or semantic-aware image manipulation, users can achieve even more impressive and realistic results. Additionally, the model's versatility in handling various image restoration tasks makes it a valuable tool for researchers and developers working on computational photography, low-level vision, and image signal processing applications.
Updated Invalid Date
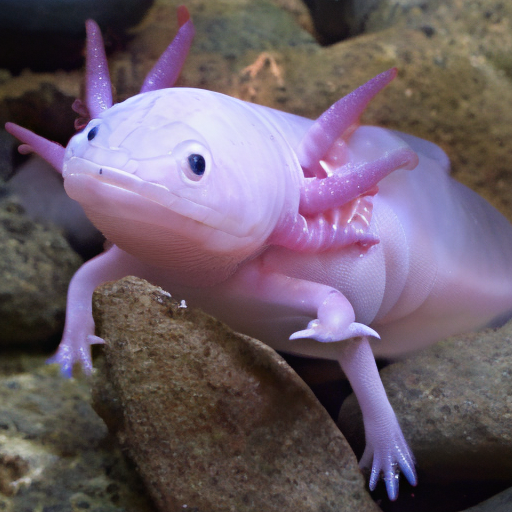
seesr

36
seesr is an AI model developed by cswry that aims to perform semantics-aware real-world image super-resolution. It builds upon the Stable Diffusion model and incorporates additional components to enhance the quality of real-world image upscaling. Unlike similar models like supir, supir-v0q, supir-v0f, real-esrgan, and gfpgan, seesr focuses on leveraging semantic information to improve the fidelity and perception of the upscaled images. Model inputs and outputs seesr takes in a low-resolution real-world image and generates a high-resolution version of the same image, aiming to preserve the semantic content and visual quality. The model can handle a variety of input images, from natural scenes to portraits and close-up shots. Inputs Image**: The input low-resolution real-world image Outputs Output image**: The high-resolution version of the input image, with improved fidelity and perception Capabilities seesr demonstrates the ability to perform semantics-aware real-world image super-resolution, preserving the semantic content and visual quality of the input images. It can handle a diverse range of real-world scenes, from buildings and landscapes to people and animals, and produces high-quality upscaled results. What can I use it for? seesr can be used for a variety of applications that require high-resolution real-world images, such as photo editing, digital art, and content creation. Its semantic awareness allows for more faithful and visually pleasing upscaling, making it a valuable tool for professionals and enthusiasts alike. Additionally, the model can be utilized in applications where high-quality image assets are needed, such as virtual reality, gaming, and architectural visualization. Things to try One interesting aspect of seesr is its ability to balance the trade-off between fidelity and perception in the upscaled images. Users can experiment with the various parameters, such as the number of inference steps and the guidance scale, to find the right balance for their specific use cases. Additionally, users can try manually specifying prompts to further enhance the quality of the results, as the automatic prompt extraction by the DAPE component may not always be perfect.
Updated Invalid Date
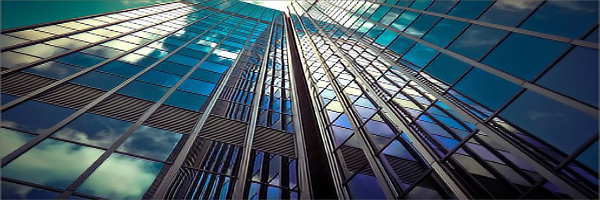
arbsr

21
The arbsr model, developed by Longguang Wang, is a plug-in module that extends a baseline super-resolution (SR) network to a scale-arbitrary SR network with a small additional cost. This allows the model to perform non-integer and asymmetric scale factor SR, while maintaining state-of-the-art performance for integer scale factors. This is useful for real-world applications where arbitrary zoom levels are required, beyond the typical integer scale factors. The arbsr model is related to other SR models like GFPGAN, ESRGAN, SuPeR, and HCFlow-SR, which focus on various aspects of image restoration and enhancement. Model inputs and outputs Inputs image**: The input image to be super-resolved target_width**: The desired width of the output image, which can be 1-4 times the input width target_height**: The desired height of the output image, which can be 1-4 times the input width Outputs Output**: The super-resolved image at the desired target size Capabilities The arbsr model is capable of performing scale-arbitrary super-resolution, including non-integer and asymmetric scale factors. This allows for more flexible and customizable image enlargement compared to typical integer-only scale factors. What can I use it for? The arbsr model can be useful for a variety of real-world applications where arbitrary zoom levels are required, such as image editing, content creation, and digital asset management. By enabling non-integer and asymmetric scale factor SR, the model provides more flexibility and control over the final image resolution, allowing users to zoom in on specific details or adapt the image size to their specific needs. Things to try One interesting aspect of the arbsr model is its ability to handle continuous scale factors, which can be explored using the interactive viewer provided by the maintainer. This allows you to experiment with different zoom levels and observe the model's performance in real-time.
Updated Invalid Date
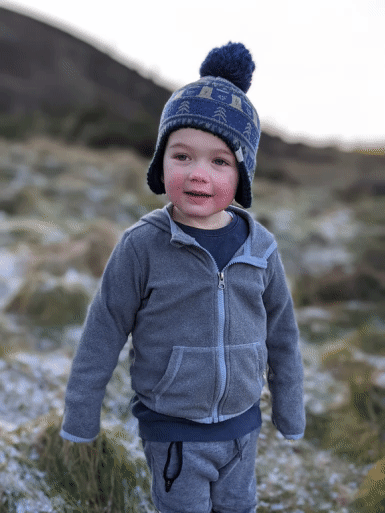
frame-interpolation

259
The frame-interpolation model, developed by the Google Research team, is a high-quality frame interpolation neural network that can transform near-duplicate photos into slow-motion footage. It uses a unified single-network approach without relying on additional pre-trained networks like optical flow or depth estimation, yet achieves state-of-the-art results. The model is trainable from frame triplets alone and uses a multi-scale feature extractor with shared convolution weights across scales. The frame-interpolation model is similar to the FILM: Frame Interpolation for Large Motion model, which also focuses on frame interpolation for large scene motion. Other related models include stable-diffusion, a latent text-to-image diffusion model, video-to-frames and frames-to-video, which split a video into frames and convert frames to a video, respectively, and lcm-animation, a fast animation model using a latent consistency model. Model inputs and outputs The frame-interpolation model takes two input frames and the number of times to interpolate between them. The output is a URI pointing to the interpolated frames, including the input frames, with the number of output frames determined by the "Times To Interpolate" parameter. Inputs Frame1**: The first input frame Frame2**: The second input frame Times To Interpolate**: Controls the number of times the frame interpolator is invoked. When set to 1, the output will be the sub-frame at t=0.5; when set to > 1, the output will be an interpolation video with (2^times_to_interpolate + 1) frames, at 30 fps. Outputs Output**: A URI pointing to the interpolated frames, including the input frames. Capabilities The frame-interpolation model can transform near-duplicate photos into slow-motion footage that looks as if it was shot with a video camera. It is capable of handling large scene motion and achieving state-of-the-art results without relying on additional pre-trained networks. What can I use it for? The frame-interpolation model can be used to create high-quality slow-motion videos from a set of near-duplicate photos. This can be particularly useful for capturing dynamic scenes or events where a video camera was not available. The model's ability to handle large scene motion makes it well-suited for a variety of applications, such as creating cinematic-quality videos, enhancing surveillance footage, or generating visual effects for film and video production. Things to try With the frame-interpolation model, you can experiment with different levels of interpolation by adjusting the "Times To Interpolate" parameter. This allows you to control the number of in-between frames generated, enabling you to create slow-motion footage with varying degrees of smoothness and detail. Additionally, you can try the model on a variety of input image pairs to see how it handles different types of motion and scene complexity.
Updated Invalid Date