real-esrgan-a40
Maintainer: anotherjesse

204
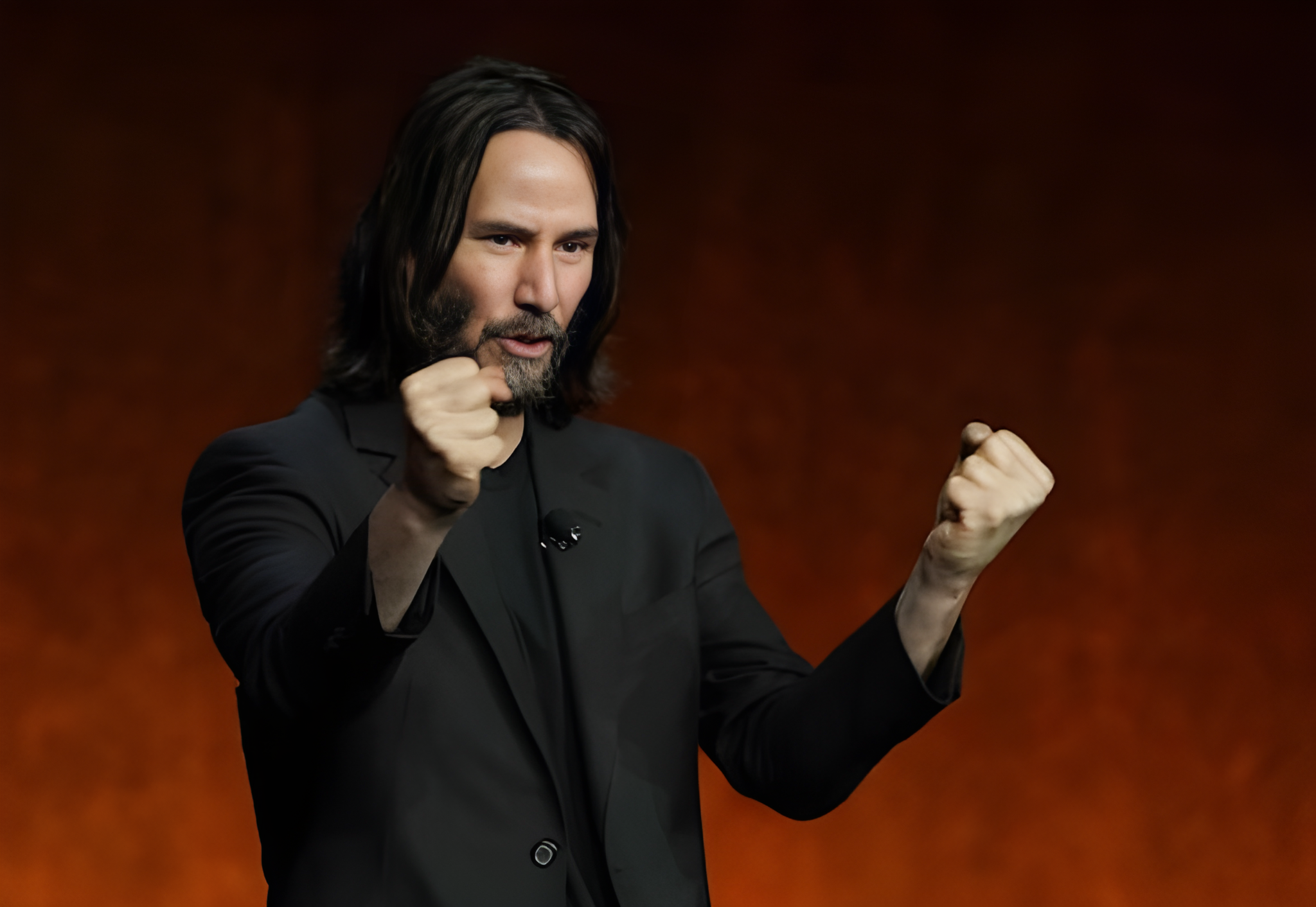
Property | Value |
---|---|
Run this model | Run on Replicate |
API spec | View on Replicate |
Github link | No Github link provided |
Paper link | No paper link provided |
Create account to get full access
Model overview
real-esrgan-a40
is a variant of the Real-ESRGAN model, which is a powerful image upscaling and enhancement tool. It was created by anotherjesse, a prolific AI model developer. Like the original Real-ESRGAN, real-esrgan-a40
can upscale images while preserving details and reducing noise. It also has the ability to enhance facial features using the GFPGAN face enhancement model.
Model inputs and outputs
real-esrgan-a40
takes an input image and a scale factor, and outputs an upscaled and enhanced version of the image. The model supports adjustable upscaling, with a scale factor ranging from 0 to 10, allowing you to control the level of magnification. It also has a "face enhance" option, which can be used to improve the appearance of faces in the output image.
Inputs
- image: The input image to be upscaled and enhanced
- scale: The factor to scale the image by, between 0 and 10
- face_enhance: A boolean flag to enable GFPGAN face enhancement
Outputs
- Output: The upscaled and enhanced version of the input image
Capabilities
real-esrgan-a40
is capable of significantly improving the quality of low-resolution images through its upscaling and enhancement capabilities. It can produce visually stunning results, especially when dealing with images that contain human faces. The model's ability to adjust the scale factor and enable face enhancement provides users with a high degree of control over the output.
What can I use it for?
real-esrgan-a40
can be used in a variety of applications, such as enhancing images for social media, improving the quality of old photographs, or generating high-resolution images for print and digital media. It could also be integrated into image editing workflows or used to upscale and enhance images generated by other AI models, such as real-esrgan or llava-lies.
Things to try
One interesting aspect of real-esrgan-a40
is its ability to enhance facial features. You could try using the "face enhance" option to improve the appearance of portraits or other images with human faces. Additionally, experimenting with different scale factors can produce a range of upscaling results, from subtle improvements to dramatic enlargements.
This summary was produced with help from an AI and may contain inaccuracies - check out the links to read the original source documents!
Related Models
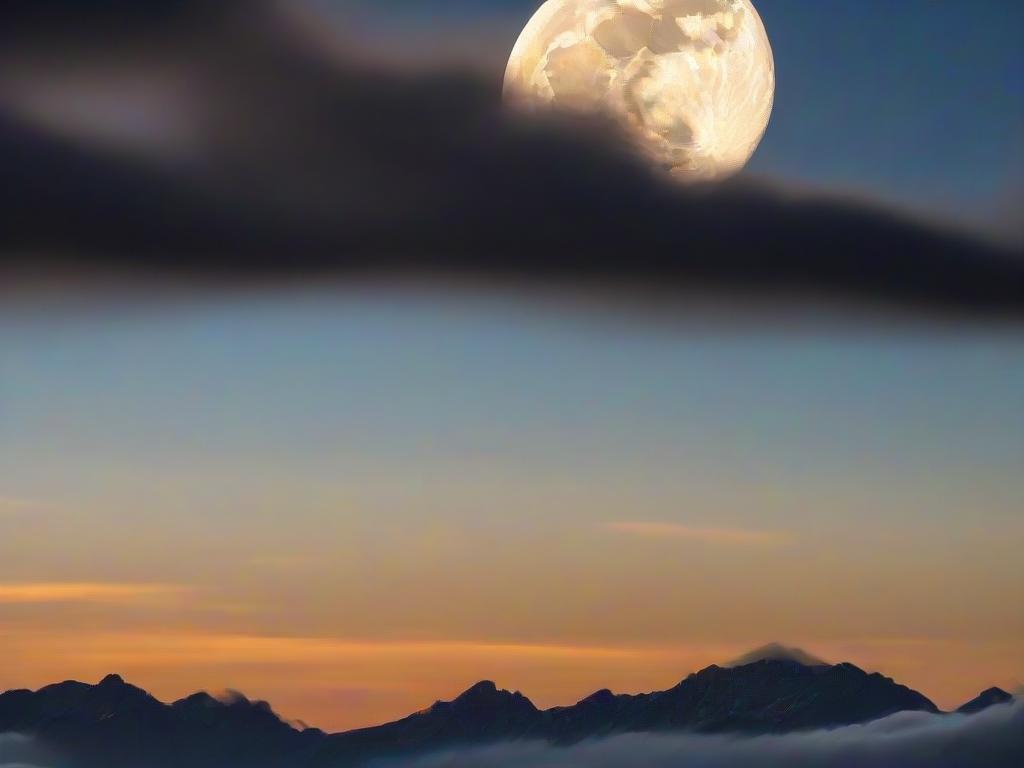
sdxl-recur

1
The sdxl-recur model is an exploration of image-to-image zooming and recursive generation of images, built on top of the SDXL model. This model allows for the generation of images through a process of progressive zooming and refinement, starting from an initial image or prompt. It is similar to other SDXL-based models like image-merge-sdxl, sdxl-custom-model, masactrl-sdxl, and sdxl, all of which build upon the core SDXL architecture. Model inputs and outputs The sdxl-recur model accepts a variety of inputs, including a prompt, an optional starting image, zoom factor, number of steps, and number of frames. The model then generates a series of images that progressively zoom in on the initial prompt or image. The outputs are an array of generated image URLs. Inputs Prompt**: The input text prompt that describes the desired image. Image**: An optional starting image that the model can use as a reference. Zoom**: The zoom factor to apply to the image during the recursive generation process. Steps**: The number of denoising steps to perform per image. Frames**: The number of frames to generate in the recursive process. Width/Height**: The desired width and height of the output images. Scheduler**: The scheduler algorithm to use for the diffusion process. Guidance Scale**: The scale for classifier-free guidance, which controls the balance between the prompt and the model's own generation. Prompt Strength**: The strength of the input prompt when using image-to-image or inpainting. Outputs The model generates an array of image URLs representing the recursively zoomed and refined images. Capabilities The sdxl-recur model is capable of generating images based on a text prompt, or starting from an existing image and recursively zooming and refining the output. This allows for the exploration of increasingly detailed and complex visual concepts, starting from a high-level prompt or initial image. What can I use it for? The sdxl-recur model could be useful for a variety of creative and artistic applications, such as generating concept art, visual storytelling, or exploring abstract and surreal imagery. The recursive zooming and refinement process could also be applied to tasks like product visualization, architectural design, or scientific visualization, where the ability to generate increasingly detailed and focused images could be valuable. Things to try One interesting aspect of the sdxl-recur model is the ability to start with an existing image and recursively zoom in, generating increasingly detailed and refined versions of the original. This could be useful for tasks like image enhancement, object detection, or content-aware image editing. Additionally, experimenting with different prompts, zoom factors, and other input parameters could lead to the discovery of unexpected and unique visual outputs.
Updated Invalid Date
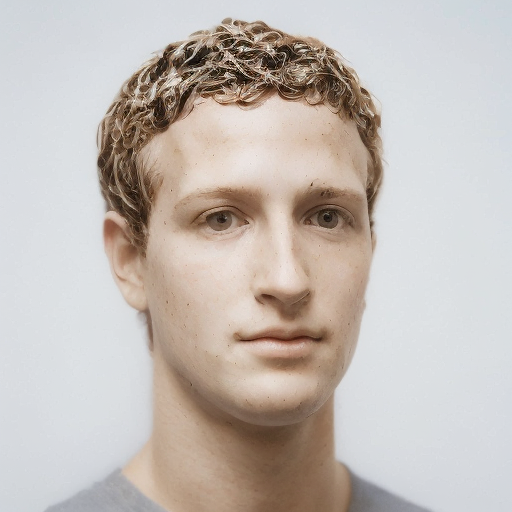
llava-lies

2
llava-lies is a model developed by Replicate AI contributor anotherjesse. It is related to the LLaVA (Large Language and Vision Assistant) family of models, which are large language and vision models aimed at achieving GPT-4-level capabilities. The llava-lies model specifically focuses on injecting randomness into generated images. Model inputs and outputs The llava-lies model takes in the following inputs: Inputs Image**: The input image to generate from Prompt**: The prompt to use for text generation Image Seed**: The seed to use for image generation Temperature**: Adjusts the randomness of the outputs, with higher values resulting in more random generation Max Tokens**: The maximum number of tokens to generate The output of the model is an array of generated text. Capabilities The llava-lies model is capable of generating text based on a given prompt and input image, with the ability to control the randomness of the output through the temperature parameter. This could be useful for tasks like creative writing, image captioning, or generating descriptive text to accompany images. What can I use it for? The llava-lies model could be used in a variety of applications that require generating text based on visual inputs, such as: Automated image captioning for social media or e-commerce Generating creative story ideas or plot points based on visual prompts Enhancing product descriptions with visually-inspired text Exploring the creative potential of combining language and vision models Things to try One interesting aspect of the llava-lies model is its ability to inject randomness into the image generation process. This could be used to explore the boundaries of creative expression, generating a diverse range of interpretations or ideas based on a single visual prompt. Experimenting with different temperature settings and image seeds could yield unexpected and thought-provoking results.
Updated Invalid Date
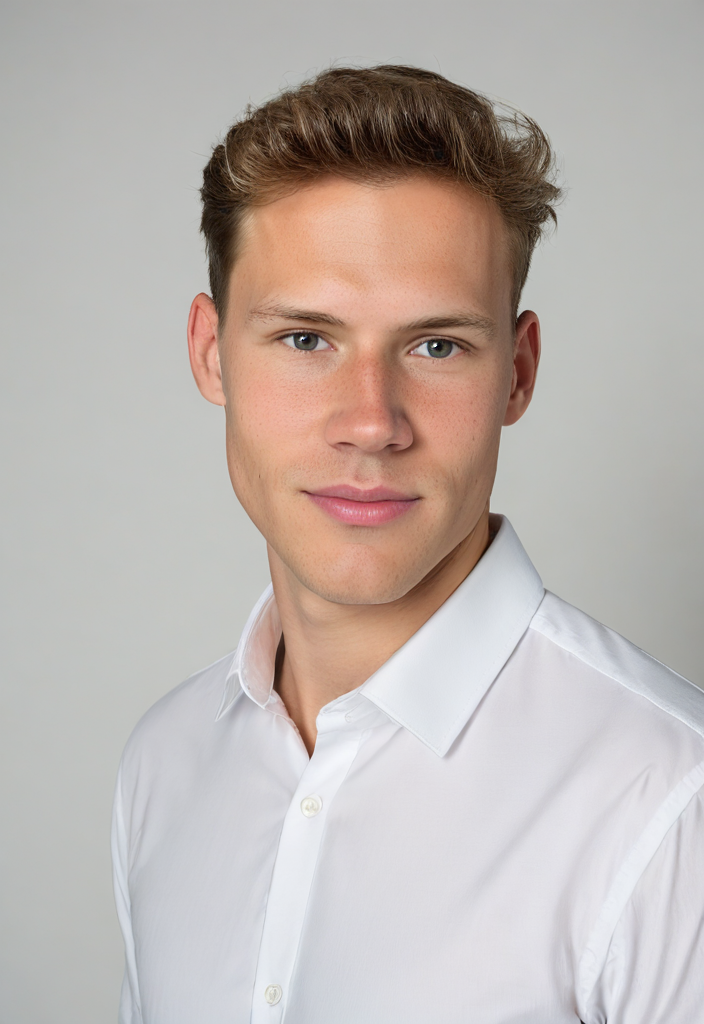
real-esrgan-v2

485
The real-esrgan-v2 model is an AI-powered image upscaling tool created by maintainer juergengunz. It builds upon the popular Real-ESRGAN model, which is known for its ability to enhance images with AI-driven face correction. Similar models include real-esrgan by nightmareai, ultimate-portrait-upscale by juergengunz, and real-esrgan by lucataco. Model inputs and outputs The real-esrgan-v2 model takes an image as input and provides an upscaled and enhanced version of that image as output. Users can control various parameters like the scale factor and whether to enhance the eyes, face, or mouth. Inputs image**: The input image to be upscaled scale**: The factor to scale the image by, up to 2x enhance_eyes**: Whether to enhance the eyes in the image face_enhance**: Whether to enhance the face in the image enhance_mouth**: Whether to enhance the mouth in the image Outputs Output**: The upscaled and enhanced output image Capabilities The real-esrgan-v2 model is capable of significantly improving the quality and detail of images through its powerful upscaling and enhancement capabilities. It can produce visually stunning results, especially for portraits and other images with prominent facial features. What can I use it for? The real-esrgan-v2 model can be useful for a variety of applications, such as enhancing low-resolution images for use in marketing materials, creating high-quality images for social media, or improving the visual quality of images used in presentations or publications. Businesses could potentially use it to improve the visual impact of their digital content. Photographers and digital artists may also find it helpful for enhancing their work. Things to try One interesting aspect of the real-esrgan-v2 model is its ability to selectively enhance specific facial features like the eyes and mouth. This could be useful for creating more dramatic or striking portraits, or for emphasizing particular aspects of a subject's appearance. Experimenting with the different enhancement options could lead to some unique and creative results.
Updated Invalid Date
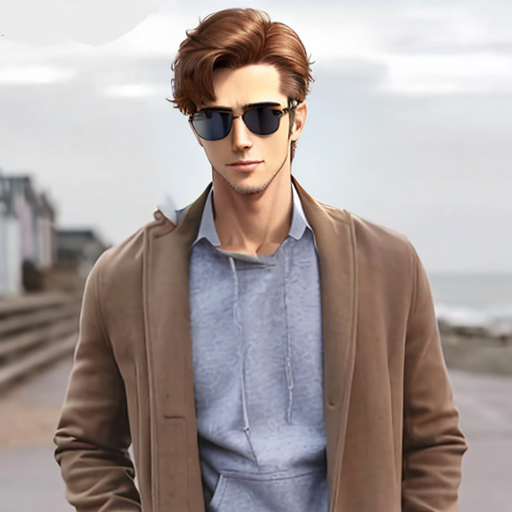
controlnet-inpaint-test

89
controlnet-inpaint-test is a Stable Diffusion-based AI model created by Replicate user anotherjesse. This model is designed for inpainting tasks, allowing users to generate new content within a specified mask area of an image. It builds upon the capabilities of the ControlNet family of models, which leverage additional control signals to guide the image generation process. Similar models include controlnet-x-ip-adapter-realistic-vision-v5, multi-control, multi-controlnet-x-consistency-decoder-x-realestic-vision-v5, controlnet-x-majic-mix-realistic-x-ip-adapter, and controlnet-1.1-x-realistic-vision-v2.0, all of which explore various aspects of the ControlNet architecture and its applications. Model inputs and outputs controlnet-inpaint-test takes a set of inputs to guide the image generation process, including a mask, prompt, control image, and various hyperparameters. The model then outputs one or more images that match the provided prompt and control signals. Inputs Mask**: The area of the image to be inpainted. Prompt**: The text description of the desired output image. Control Image**: An optional image to guide the generation process. Seed**: A random seed value to control the output. Width/Height**: The dimensions of the output image. Num Outputs**: The number of images to generate. Scheduler**: The denoising scheduler to use. Guidance Scale**: The scale for classifier-free guidance. Num Inference Steps**: The number of denoising steps. Disable Safety Check**: An option to disable the safety check. Outputs Output Images**: One or more generated images that match the provided prompt and control signals. Capabilities controlnet-inpaint-test demonstrates the ability to generate new content within a specified mask area of an image, while maintaining coherence with the surrounding context. This can be useful for tasks such as object removal, scene editing, and image repair. What can I use it for? The controlnet-inpaint-test model can be utilized for a variety of image editing and manipulation tasks. For example, you could use it to remove unwanted elements from a photograph, replace damaged or occluded areas of an image, or combine different visual elements into a single cohesive scene. Additionally, the model's ability to generate new content based on a prompt and control image could be leveraged for creative projects, such as concept art or product visualization. Things to try One interesting aspect of controlnet-inpaint-test is its ability to blend the generated content seamlessly with the surrounding image. By carefully selecting the control image and mask, you can explore ways to create visually striking and plausible compositions. Additionally, experimenting with different prompts and hyperparameters can yield a wide range of creative outputs, from photorealistic to more fantastical imagery.
Updated Invalid Date