vid2openpose
Maintainer: lucataco

1
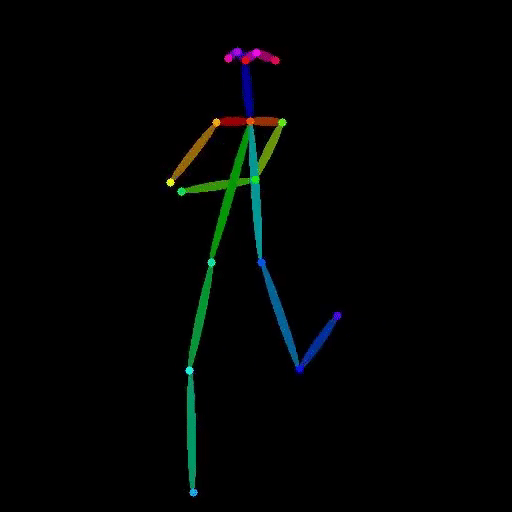
Property | Value |
---|---|
Model Link | View on Replicate |
API Spec | View on Replicate |
Github Link | View on Github |
Paper Link | No paper link provided |
Create account to get full access
Model overview
vid2openpose
is a Cog model developed by lucataco that can take a video as input and generate an output video with OpenPose-style skeletal pose estimation overlaid on the original frames. This model is similar to other AI models like DeepSeek-VL, open-dalle-v1.1, and ProteusV0.1 created by lucataco, which focus on various computer vision and language understanding capabilities.
Model inputs and outputs
The vid2openpose
model takes a single input of a video file. The output is a new video file with the OpenPose-style skeletal pose estimation overlaid on the original frames.
Inputs
- Video: The input video file to be processed.
Outputs
- Output Video: The resulting video with the OpenPose-style skeletal pose estimation overlaid.
Capabilities
The vid2openpose
model is capable of taking an input video and generating a new video with real-time skeletal pose estimation using the OpenPose algorithm. This can be useful for a variety of applications, such as motion capture, animation, and human pose analysis.
What can I use it for?
The vid2openpose
model can be used for a variety of applications, such as:
- Motion capture: The skeletal pose estimation can be used to capture the motion of actors or athletes for use in animation or video games.
- Human pose analysis: The skeletal pose estimation can be used to analyze the movements and posture of people in various situations, such as fitness or rehabilitation.
- Animation: The skeletal pose estimation can be used as a starting point for animating characters in videos or films.
Things to try
One interesting thing to try with the vid2openpose
model is to use it to analyze the movements of athletes or dancers, and then use that data to create new animations or visualizations. Another idea is to use the model to create interactive experiences where users can control a virtual character by moving in front of a camera.
This summary was produced with help from an AI and may contain inaccuracies - check out the links to read the original source documents!
Related Models
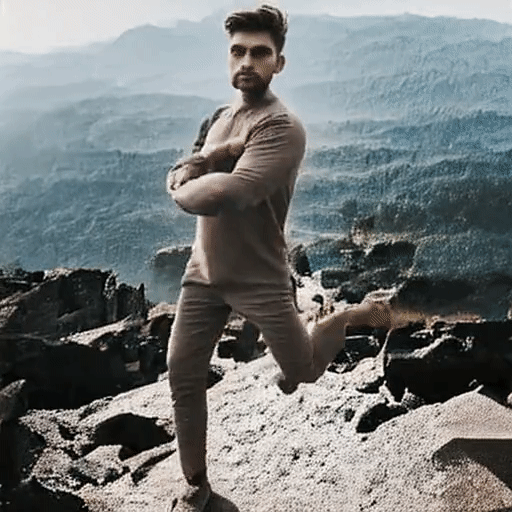
magic-animate-openpose

8
The magic-animate-openpose model is an implementation of the magic-animate model that uses OpenPose input instead of DensePose. Developed by Luca Taco, this model allows you to animate human figures in images using an input video. It is similar to other models like vid2openpose, vid2densepose, and the original magic-animate model. Model inputs and outputs The magic-animate-openpose model takes in an image and a video as inputs. The image is the base image that will be animated, and the video provides the motion information to drive the animation. The model outputs an animated version of the input image. Inputs Image**: The input image to be animated Video**: The motion video that will drive the animation Outputs Animated Image**: The input image with the motion from the video applied to it Capabilities The magic-animate-openpose model can take an image of a human figure and animate it using the motion from an input video. This allows you to create dynamic, animated versions of static images. The model uses OpenPose to extract the pose information from the input video, which it then applies to the target image. What can I use it for? You can use the magic-animate-openpose model to create fun and engaging animated content. This could be useful for social media, video production, or even creative projects. By combining a static image with motion from a video, you can bring characters and figures to life in new and interesting ways. Things to try One interesting thing to try with the magic-animate-openpose model is to use it in combination with other models like real-esrgan-video to upscale and enhance the quality of the animated output. You could also experiment with using different types of input videos to see how the animation is affected, or try animating various types of figures beyond just humans.
Updated Invalid Date
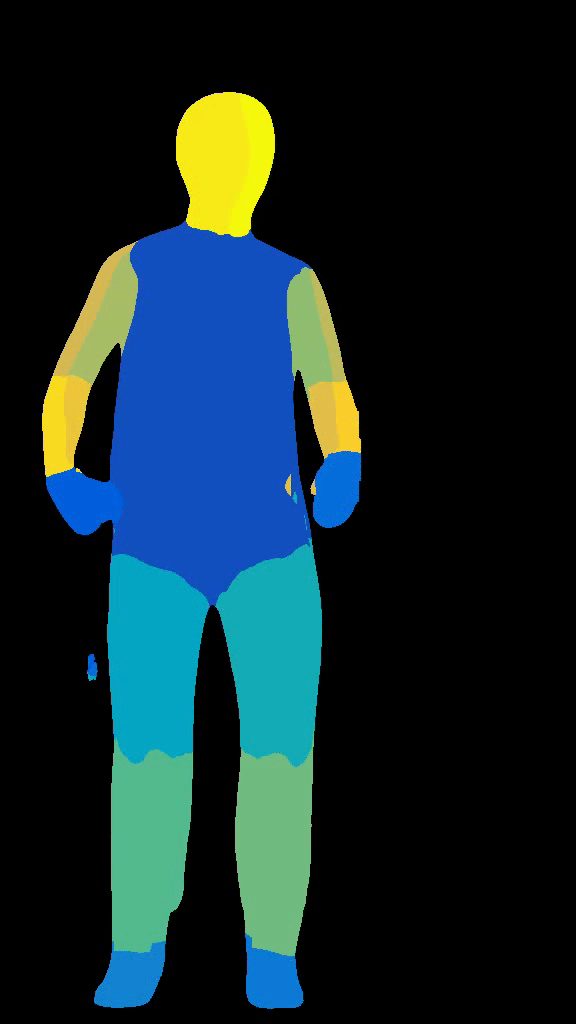
vid2densepose

4
The vid2densepose model is a powerful tool designed for applying the DensePose model to videos, generating detailed "Part Index" visualizations for each frame. This tool is particularly useful for enhancing animations, especially when used in conjunction with MagicAnimate, a model for temporally consistent human image animation. The vid2densepose model was created by lucataco, a developer known for creating various AI-powered video processing tools. Model inputs and outputs The vid2densepose model takes a video as input and outputs a new video file with the DensePose information overlaid in a vivid, color-coded format. This output can then be used as input to other models, such as MagicAnimate, to create advanced human animation projects. Inputs Input Video**: The input video file that you want to process with the DensePose model. Outputs Output Video**: The processed video file with the DensePose information overlaid in a color-coded format. Capabilities The vid2densepose model can take a video input and generate a new video output that displays detailed "Part Index" visualizations for each frame. This information can be used to enhance animations, create novel visual effects, or provide a rich dataset for further computer vision research. What can I use it for? The vid2densepose model is particularly useful for creators and animators who want to incorporate detailed human pose information into their projects. By using the output of vid2densepose as input to MagicAnimate, you can create temporally consistent and visually stunning human animations. Additionally, the DensePose data could be used for various computer vision tasks, such as human motion analysis, body part segmentation, or virtual clothing applications. Things to try One interesting thing to try with the vid2densepose model is to combine it with other video processing tools, such as Real-ESRGAN Video Upscaler or AniPortrait: Audio-Driven Synthesis of Photorealistic Portrait Animation. By stacking these models together, you can create highly detailed and visually compelling animated sequences.
Updated Invalid Date
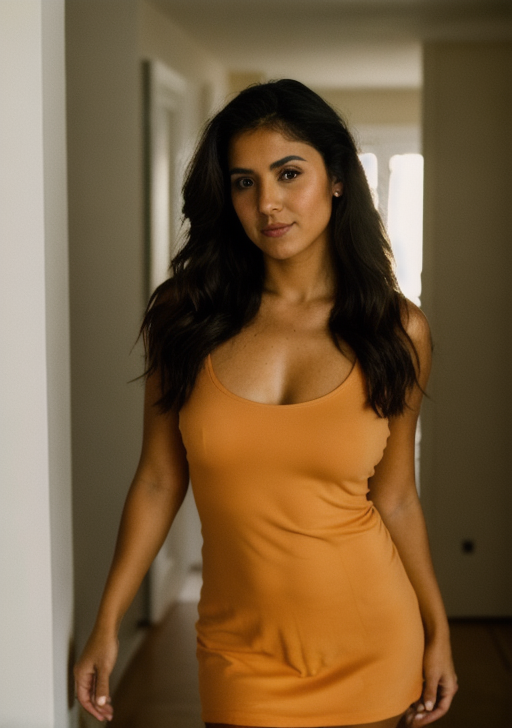
realistic-vision-v5-openpose

5
The realistic-vision-v5-openpose model is an implementation of the SG161222/Realistic_Vision_V5.0_noVAE model with OpenPose, created by lucataco. This model aims to generate realistic images based on a text prompt, while incorporating OpenPose to leverage pose information. It is similar to other Realistic Vision models developed by lucataco, each with its own unique capabilities and use cases. Model inputs and outputs The realistic-vision-v5-openpose model takes a text prompt, an input image, and various configurable parameters as inputs. The text prompt describes the desired output image, while the input image provides pose information to guide the generation. The model outputs a generated image that matches the given prompt and leverages the pose information from the input. Inputs Image**: The input pose image to guide the generation Prompt**: The text description of the desired output image Seed**: The random seed value (0 for random, up to 2147483647) Steps**: The number of inference steps (0-100) Width**: The desired width of the output image (0-1920) Height**: The desired height of the output image (0-1920) Guidance**: The guidance scale (3.5-7) Scheduler**: The scheduler algorithm to use for inference Outputs Output image**: The generated image that matches the input prompt and leverages the pose information Capabilities The realistic-vision-v5-openpose model is capable of generating highly realistic images based on text prompts, while incorporating pose information from an input image. This allows for the creation of visually striking and anatomically accurate portraits, scenes, and other content. The model's attention to detail and ability to capture the nuances of human form and expression make it a powerful tool for a variety of applications, from art and design to visual storytelling and beyond. What can I use it for? The realistic-vision-v5-openpose model can be used for a wide range of creative and professional applications. Artists and designers can leverage the model to generate unique, high-quality images for use in illustrations, concept art, and other visual media. Content creators can use the model to enhance their video productions, adding realistic character animations and poses to their scenes. Researchers and developers can explore the model's capabilities for applications in areas like virtual reality, augmented reality, and human-computer interaction. Things to try One interesting aspect of the realistic-vision-v5-openpose model is its ability to generate images that seamlessly combine realistic elements with more abstract or stylized components. By experimenting with different input prompts and pose images, users can explore the model's capacity to blend realism and imagination, creating visually striking and emotionally evocative artworks. Additionally, users may want to try varying the model's configuration parameters, such as the guidance scale or the scheduler, to observe the impact on the generated output and discover new creative possibilities.
Updated Invalid Date
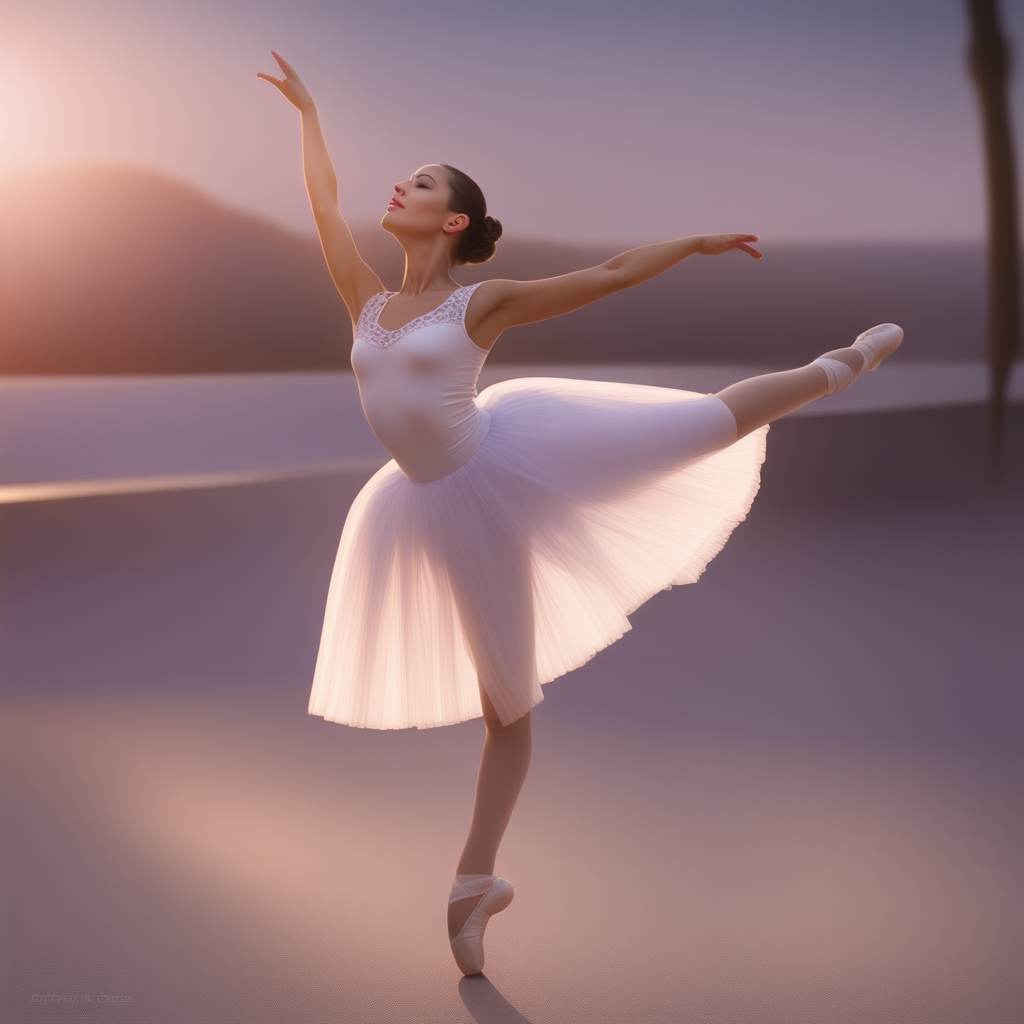
sdxl-controlnet-openpose

21
The sdxl-controlnet-openpose is an AI model developed by lucataco that combines the SDXL (Stable Diffusion XL) model with the ControlNet module to generate images based on an input prompt and a reference OpenPose image. This model is similar to other ControlNet-based models like sdxl-controlnet, sdxl-controlnet-depth, and sdxl-controlnet-lora, which use different control signals such as Canny edges, depth maps, and LoRA. Model inputs and outputs The sdxl-controlnet-openpose model takes in an input image and a text prompt, and generates an output image that combines the visual elements from the input image and the textual elements from the prompt. The input image should contain an OpenPose-style pose estimation, which the model uses as a control signal to guide the image generation process. Inputs Image**: The input image containing the OpenPose-style pose estimation. Prompt**: The text prompt describing the desired image. Guidance Scale**: A parameter that controls the influence of the text prompt on the generated image. High Noise Frac**: A parameter that controls the level of noise in the generated image. Negative Prompt**: A text prompt that describes elements that should not be included in the generated image. Num Inference Steps**: The number of denoising steps to perform during the image generation process. Outputs Output Image**: The generated image that combines the visual elements from the input image and the textual elements from the prompt. Capabilities The sdxl-controlnet-openpose model can generate high-quality, photorealistic images based on a text prompt and a reference OpenPose image. This can be useful for creating images of specific scenes or characters, such as a "latina ballerina in a romantic sunset" as demonstrated in the example. The model can also be used to generate images for a variety of other applications, such as character design, fashion design, or visual storytelling. What can I use it for? The sdxl-controlnet-openpose model can be used for a variety of creative and commercial applications, such as: Generating images for use in video games, films, or other media Designing characters or costumes for cosplay or other creative projects Visualizing ideas or concepts for design or marketing purposes Enhancing existing images with new elements or effects Additionally, the model can be used in conjunction with other ControlNet-based models, such as sdxl-controlnet or sdxl-controlnet-depth, to create even more versatile and compelling images. Things to try One interesting thing to try with the sdxl-controlnet-openpose model is to experiment with different input images and prompts to see the range of outputs it can generate. For example, you could try using the model to generate images of different types of dancers or athletes, or to create unique and surreal scenes by combining the OpenPose control signal with more abstract or imaginative prompts. Another interesting approach might be to use the model in a iterative or collaborative way, where the generated image is used as a starting point for further refinement or elaboration, either manually or through the use of other AI-powered tools.
Updated Invalid Date