idm-vton
Maintainer: cuuupid

312
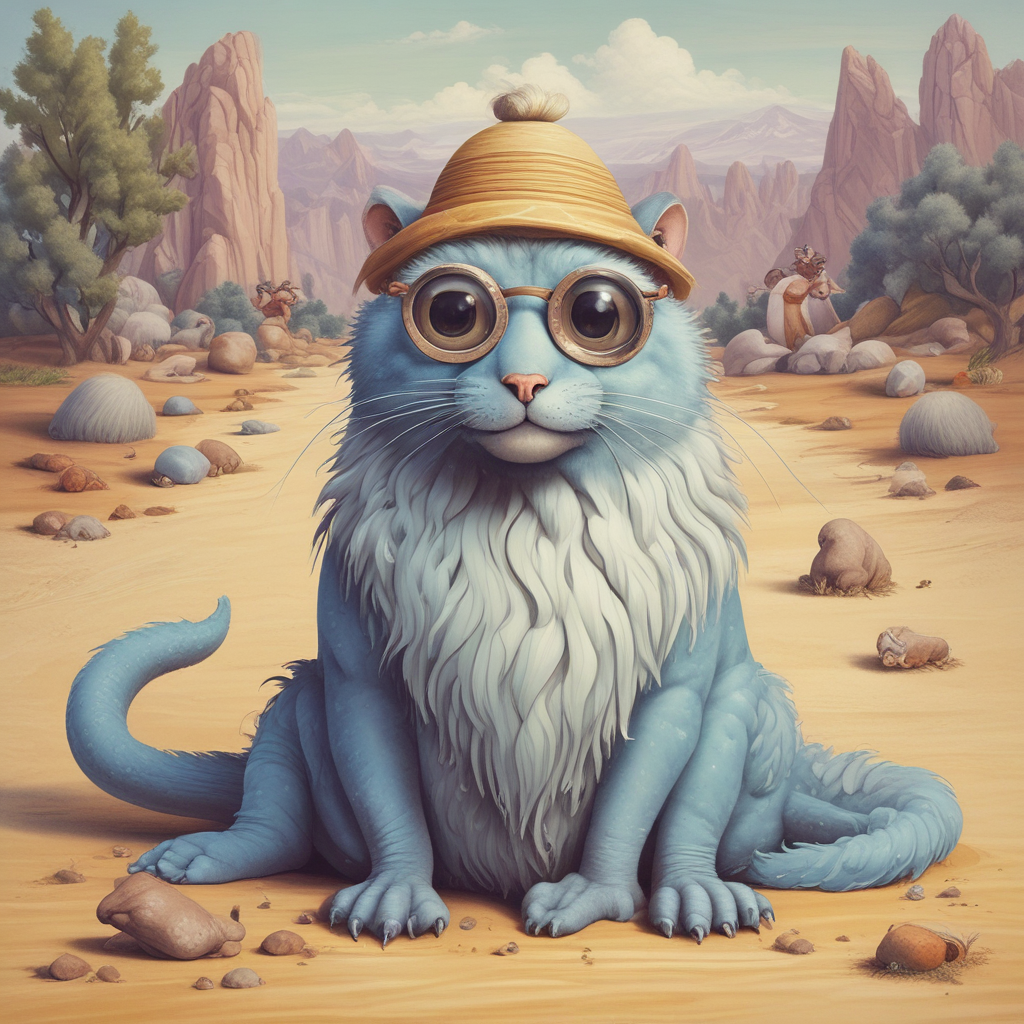
Property | Value |
---|---|
Run this model | Run on Replicate |
API spec | View on Replicate |
Github link | View on Github |
Paper link | View on Arxiv |
Create account to get full access
Model overview
The idm-vton
model, developed by the researcher cuuupid, is a state-of-the-art clothing virtual try-on system designed to work in the wild. It outperforms similar models like instant-id, absolutereality-v1.8.1, and reliberate-v3 in terms of realism and authenticity.
Model inputs and outputs
The idm-vton
model takes in several input images and parameters to generate a realistic image of a person wearing a particular garment. The inputs include the garment image, a mask image, the human image, and optional parameters like crop, seed, and steps. The model outputs a single image of the person wearing the garment.
Inputs
- Garm Img: The image of the garment, which should match the specified category (e.g., upper body, lower body, or dresses).
- Mask Img: An optional mask image that can be used to speed up the process.
- Human Img: The image of the person who will be wearing the garment.
- Category: The category of the garment, which can be "upper_body", "lower_body", or "dresses".
- Crop: A boolean indicating whether to use cropping on the input images.
- Seed: An integer that sets the random seed for reproducibility.
- Steps: The number of diffusion steps to use for generating the output image.
Outputs
- Output: A single image of the person wearing the specified garment.
Capabilities
The idm-vton
model is capable of generating highly realistic and authentic virtual try-on images, even in challenging "in the wild" scenarios. It outperforms previous methods by using advanced diffusion models and techniques to seamlessly blend the garment with the person's body and background.
What can I use it for?
The idm-vton
model can be used for a variety of applications, such as e-commerce clothing websites, virtual fashion shows, and personal styling tools. By allowing users to visualize how a garment would look on them, the model can help increase conversion rates, reduce return rates, and enhance the overall shopping experience.
Things to try
One interesting aspect of the idm-vton
model is its ability to work with a wide range of garment types and styles. Try experimenting with different categories of clothing, such as formal dresses, casual t-shirts, or even accessories like hats or scarves. Additionally, you can play with the input parameters, such as the number of diffusion steps or the seed, to see how they affect the output.
This summary was produced with help from an AI and may contain inaccuracies - check out the links to read the original source documents!
Related Models
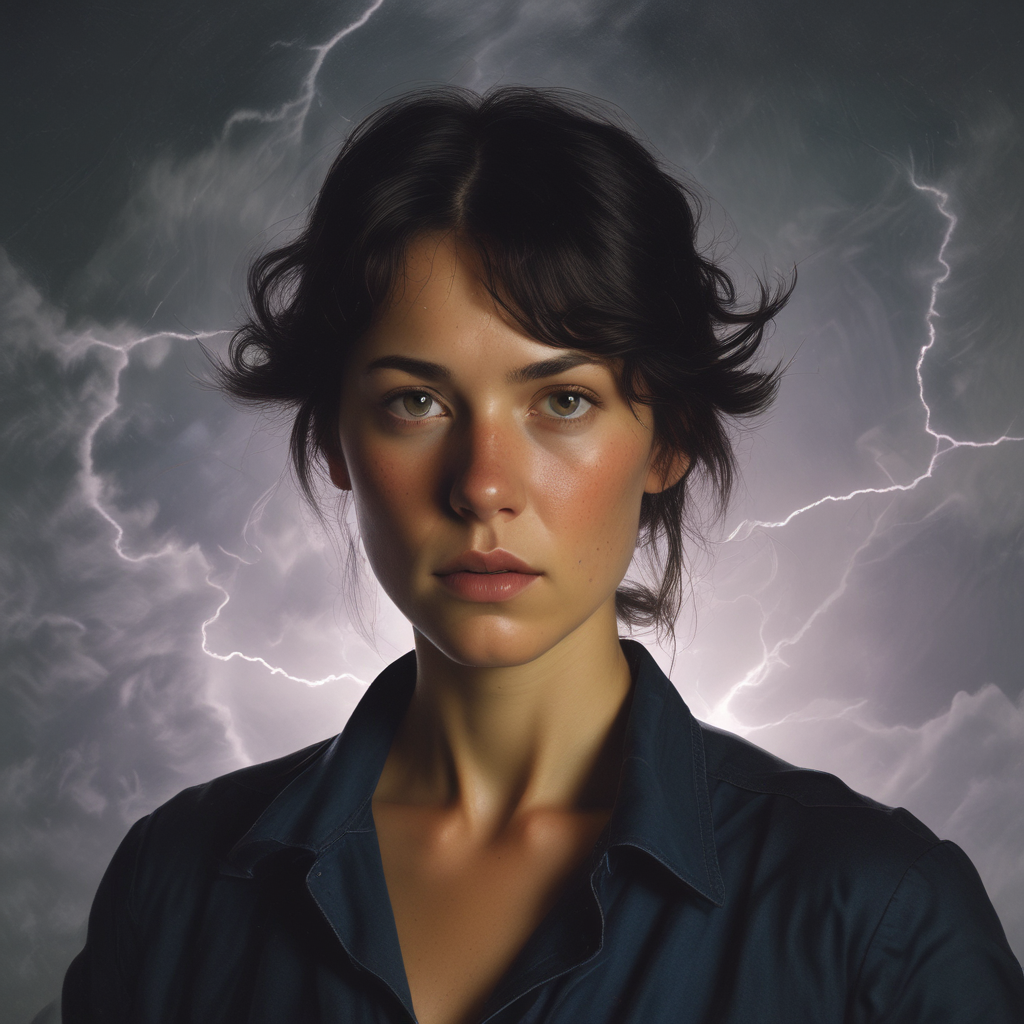
sdxl-lightning-4step

414.6K
sdxl-lightning-4step is a fast text-to-image model developed by ByteDance that can generate high-quality images in just 4 steps. It is similar to other fast diffusion models like AnimateDiff-Lightning and Instant-ID MultiControlNet, which also aim to speed up the image generation process. Unlike the original Stable Diffusion model, these fast models sacrifice some flexibility and control to achieve faster generation times. Model inputs and outputs The sdxl-lightning-4step model takes in a text prompt and various parameters to control the output image, such as the width, height, number of images, and guidance scale. The model can output up to 4 images at a time, with a recommended image size of 1024x1024 or 1280x1280 pixels. Inputs Prompt**: The text prompt describing the desired image Negative prompt**: A prompt that describes what the model should not generate Width**: The width of the output image Height**: The height of the output image Num outputs**: The number of images to generate (up to 4) Scheduler**: The algorithm used to sample the latent space Guidance scale**: The scale for classifier-free guidance, which controls the trade-off between fidelity to the prompt and sample diversity Num inference steps**: The number of denoising steps, with 4 recommended for best results Seed**: A random seed to control the output image Outputs Image(s)**: One or more images generated based on the input prompt and parameters Capabilities The sdxl-lightning-4step model is capable of generating a wide variety of images based on text prompts, from realistic scenes to imaginative and creative compositions. The model's 4-step generation process allows it to produce high-quality results quickly, making it suitable for applications that require fast image generation. What can I use it for? The sdxl-lightning-4step model could be useful for applications that need to generate images in real-time, such as video game asset generation, interactive storytelling, or augmented reality experiences. Businesses could also use the model to quickly generate product visualization, marketing imagery, or custom artwork based on client prompts. Creatives may find the model helpful for ideation, concept development, or rapid prototyping. Things to try One interesting thing to try with the sdxl-lightning-4step model is to experiment with the guidance scale parameter. By adjusting the guidance scale, you can control the balance between fidelity to the prompt and diversity of the output. Lower guidance scales may result in more unexpected and imaginative images, while higher scales will produce outputs that are closer to the specified prompt.
Updated Invalid Date
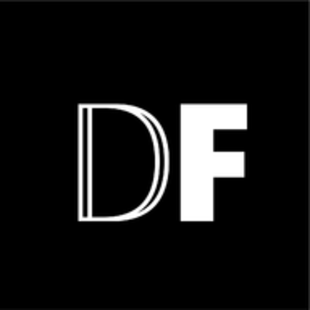
deepfashionsdxl

1
The deepfashionsdxl model is a high-resolution image generation AI developed by omniedgeio. It is similar to other SDXL-based models like sdxl-lightning-4step and fashion-design, which are also focused on generating high-quality images. The deepfashionsdxl model is particularly well-suited for fashion-related image generation tasks. Model inputs and outputs The deepfashionsdxl model takes a variety of inputs, including a text prompt, an optional input image, and various parameters to control the output. The model can generate high-resolution images up to 1024x1024 pixels in size. Inputs Prompt**: The text prompt that describes the desired image. Image**: An optional input image that can be used as a starting point for the generation process. Width/Height**: The desired width and height of the output image. Num Outputs**: The number of images to generate (up to 4). Strength**: The strength of the denoising process when using an input image. Guidance Scale**: The scale for the classifier-free guidance. Num Inference Steps**: The number of denoising steps to perform. Outputs Image**: One or more high-resolution images generated based on the provided inputs. Capabilities The deepfashionsdxl model is capable of generating high-quality, photorealistic images related to fashion and clothing. It can create images of models wearing various outfits, accessories, and fashion-forward designs. The model is particularly good at capturing intricate details and textures, making it well-suited for fashion-focused applications. What can I use it for? The deepfashionsdxl model could be useful for a variety of fashion-related applications, such as generating product images for e-commerce websites, visualizing clothing designs, or creating fashion editorials and lookbooks. It could also be used to generate concept art or inspiration for fashion designers and stylists. Additionally, the model's ability to generate high-resolution images makes it a valuable tool for creating marketing materials, social media content, and other visual assets related to the fashion industry. Things to try With the deepfashionsdxl model, you could experiment with different clothing styles, accessories, and fashion trends to see how the model interprets and generates these elements. You could also try using the model to create unique and unexpected fashion combinations or to explore the boundaries of what is possible in fashion-focused image generation.
Updated Invalid Date
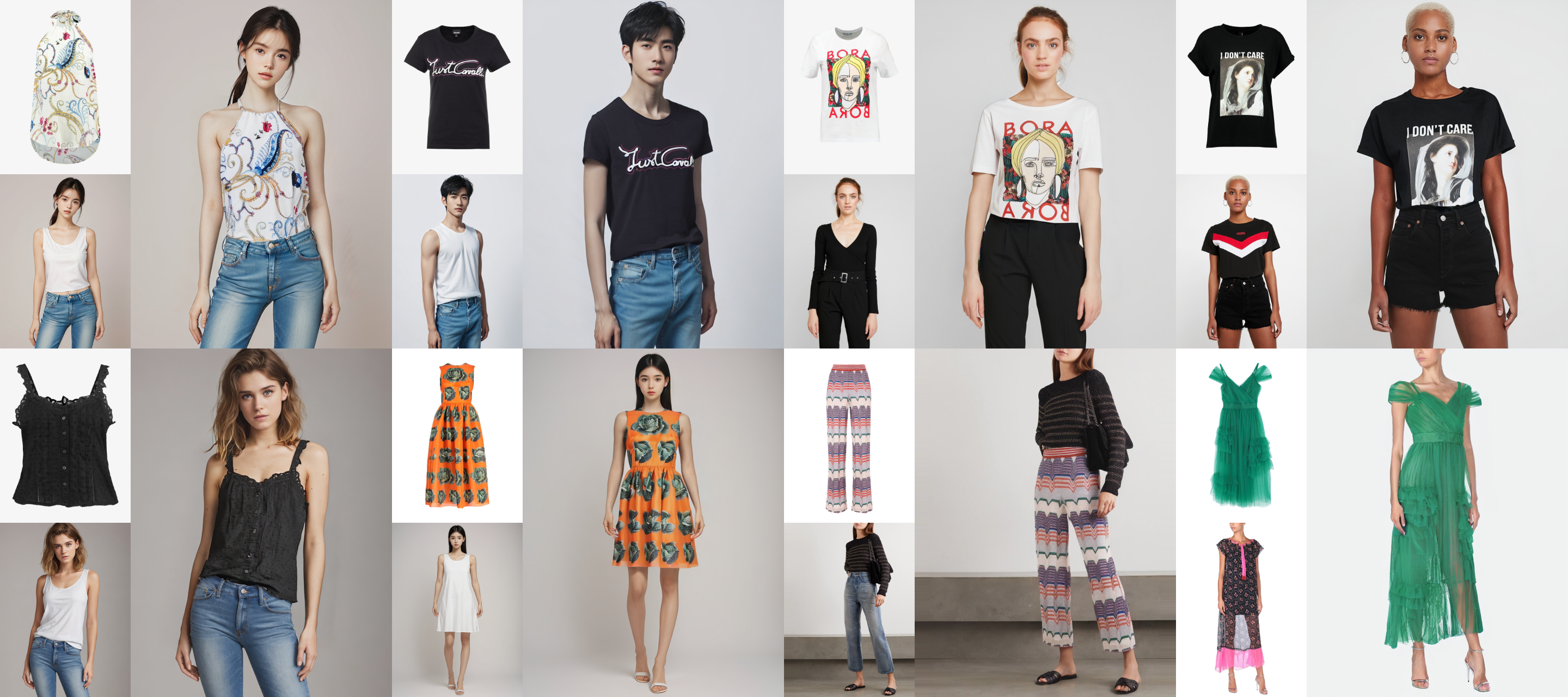
oot_diffusion

15
oot_diffusion is a virtual dressing room model created by viktorfa. It allows users to visualize how garments would look on a model. This can be useful for online clothing shopping or fashion design. Similar models include idm-vton, which provides virtual clothing try-on, and gfpgan, which restores old or AI-generated faces. Model inputs and outputs The oot_diffusion model takes several inputs to generate an image of a model wearing a specific garment. These include a seed value, the number of inference steps, an image of the model, an image of the garment, and a guidance scale. Inputs Seed**: An integer value used to initialize the random number generator. Steps**: The number of inference steps to perform, between 1 and 40. Model Image**: A clear picture of the model. Garment Image**: A clear picture of the upper body garment. Guidance Scale**: A value between 1 and 5 that controls the influence of the prompt on the generated image. Outputs An array of image URLs representing the generated outputs. Capabilities The oot_diffusion model can generate realistic images of a model wearing a specific garment. This can be useful for virtual clothing try-on, fashion design, and online shopping. What can I use it for? You can use oot_diffusion to visualize how clothing would look on a model, which can be helpful for online clothing shopping or fashion design. For example, you could use it to try on different outfits before making a purchase, or to experiment with different garment designs. Things to try With oot_diffusion, you can experiment with different input values to see how they affect the generated output. Try adjusting the seed, number of steps, or guidance scale to see how the resulting image changes. You could also try using different model and garment images to see how the model can adapt to different inputs.
Updated Invalid Date
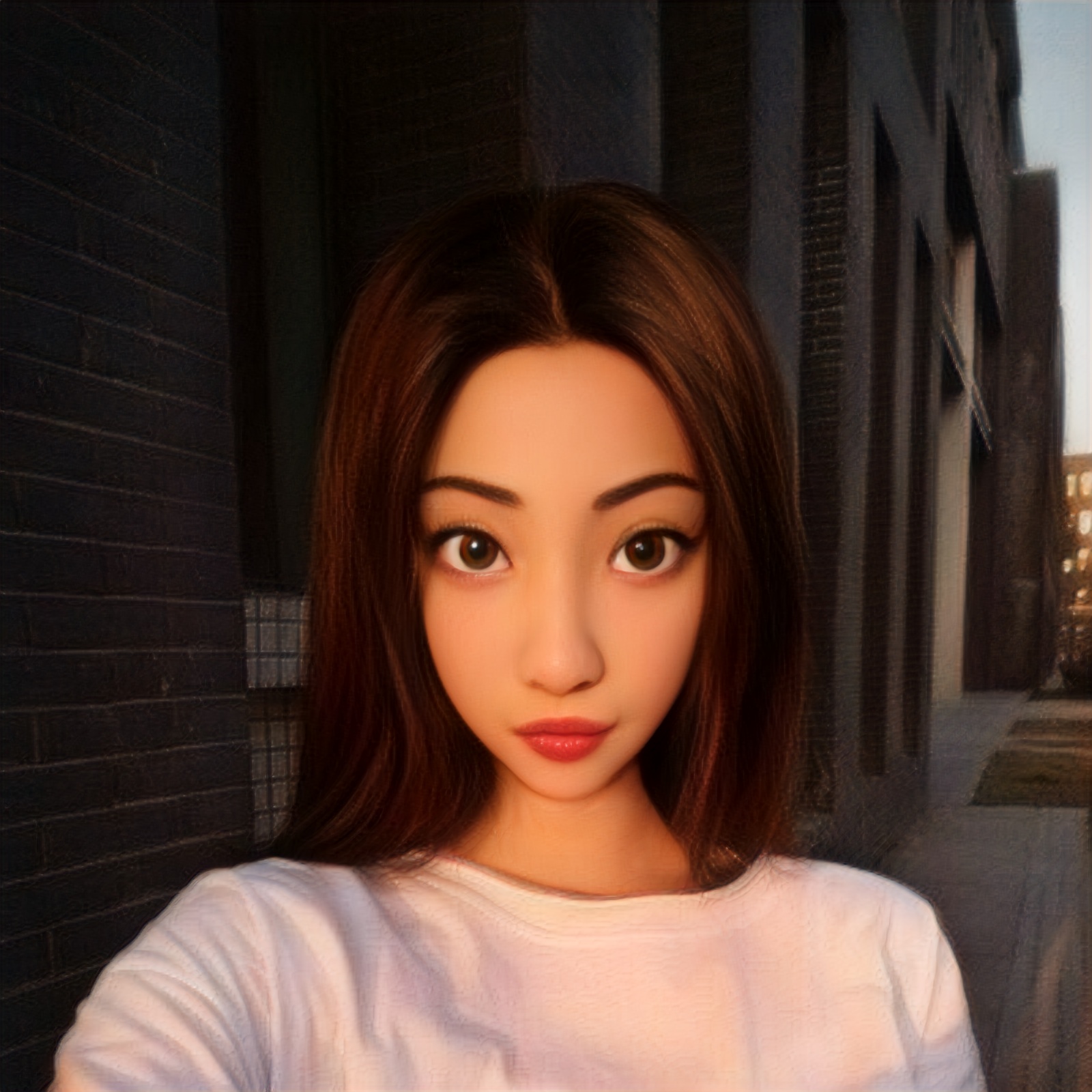
vtoonify

99
vtoonify is a model developed by 412392713 that enables high-quality artistic portrait video style transfer. It builds upon the powerful StyleGAN framework and leverages mid- and high-resolution layers to render detailed artistic portraits. Unlike previous image-oriented toonification models, vtoonify can handle non-aligned faces in videos of variable size, contributing to complete face regions with natural motions in the output. vtoonify is compatible with existing StyleGAN-based image toonification models like Toonify and DualStyleGAN, and inherits their appealing features for flexible style control on color and intensity. The model can be used to transfer the style of various reference images and adjust the style degree within a single model. Model inputs and outputs Inputs Image**: An input image or video to be stylized Padding**: The amount of padding (in pixels) to apply around the face region Style Type**: The type of artistic style to apply, such as cartoon, caricature, or comic Style Degree**: The degree or intensity of the applied style Outputs Stylized Image/Video**: The input image or video transformed with the specified artistic style Capabilities vtoonify is capable of generating high-resolution, temporally-consistent artistic portraits from input videos. It can handle non-aligned faces and preserve natural motions, unlike previous image-oriented toonification models. The model also provides flexible control over the style type and degree, allowing users to fine-tune the artistic output to their preferences. What can I use it for? vtoonify can be used to create visually striking and unique portrait videos for a variety of applications, such as: Video production and animation: Enhancing live-action footage with artistic styles to create animated or cartoon-like effects Social media and content creation: Applying stylized filters to portrait videos for more engaging and shareable content Artistic expression: Exploring different artistic styles and degrees of toonification to create unique, personalized portrait videos Things to try Some interesting things to try with vtoonify include: Experimenting with different style types (e.g., cartoon, caricature, comic) to find the one that best suits your content or artistic vision Adjusting the style degree to find the right balance between realism and stylization Applying vtoonify to footage of yourself or friends and family to create unique, personalized portrait videos Combining vtoonify with other AI-powered video editing tools to create more complex, multi-layered visual effects Overall, vtoonify offers a powerful and flexible way to transform portrait videos into unique, artistic masterpieces.
Updated Invalid Date