marker
Maintainer: cuuupid

2
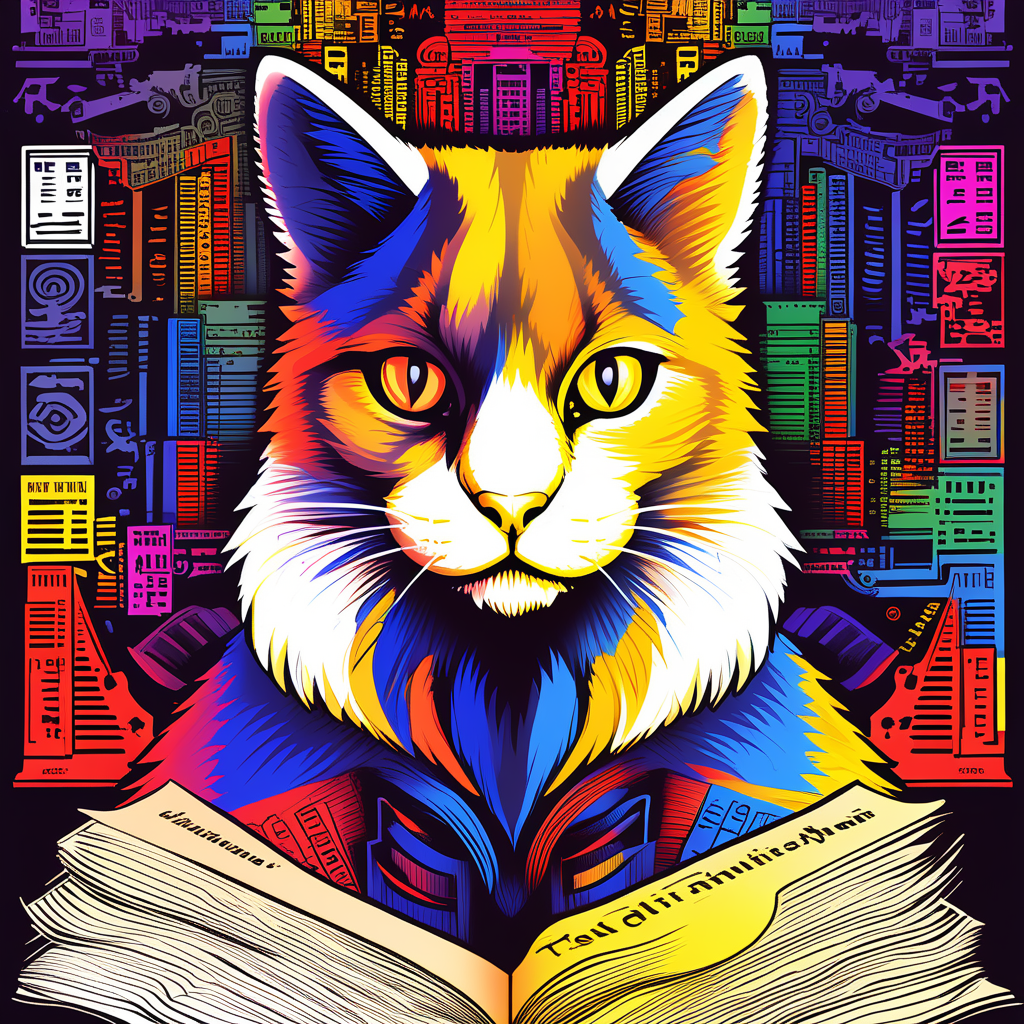
Property | Value |
---|---|
Run this model | Run on Replicate |
API spec | View on Replicate |
Github link | View on Github |
Paper link | No paper link provided |
Create account to get full access
Model overview
Marker
is an AI model created by cuuupid that converts scanned or electronic documents to Markdown format. It is designed to be faster and more accurate than similar models like ocr-surya and nougat. Marker
uses a pipeline of deep learning models to extract text, detect page layout, clean and format each block, and combine the blocks into a final Markdown document. It is optimized for speed and has low hallucination risk compared to autoregressive language models.
Model inputs and outputs
Marker
takes a variety of document formats as input, including PDF, EPUB, and MOBI, and converts them to Markdown. It can handle a range of PDF documents, including books and scientific papers, and can remove headers, footers, and other artifacts. The model can also convert most equations to LaTeX format and format code blocks and tables.
Inputs
- Document: The input file, which can be a PDF, EPUB, MOBI, XPS, or FB2 document.
- Language: The language of the document, which is used for OCR and other processing.
- DPI: The DPI to use for OCR.
- Max Pages: The maximum number of pages to parse.
- Enable Editor: Whether to enable the editor model for additional processing.
- Parallel Factor: The parallel factor to use for OCR.
Outputs
- Markdown: The converted Markdown text of the input document.
Capabilities
Marker
is designed to be fast and accurate, with low hallucination risk compared to other models. It can handle a variety of document types and languages, and it includes features like equation conversion, code block formatting, and table formatting. The model is built on a pipeline of deep learning models, including a layout segmenter, column detector, and postprocessor, which allows it to be more robust and accurate than models that rely solely on autoregressive language generation.
What can I use it for?
Marker
is a powerful tool for converting PDFs, EPUBs, and other document formats to Markdown. This can be useful for a variety of applications, such as:
- Archiving and preserving digital documents: By converting documents to Markdown, you can ensure that they are easily searchable and preservable for the long term.
- Technical writing and documentation:
Marker
can be used to convert technical documents, such as scientific papers or programming tutorials, to Markdown, making them easier to edit, version control, and publish. - Content creation and publishing: The Markdown output of
Marker
can be easily integrated into content management systems or other publishing platforms, allowing for more efficient and streamlined content creation workflows.
Things to try
One interesting feature of Marker
is its ability to handle a variety of document types and languages. You could try using it to convert documents in languages other than English, or to process more complex document types like technical manuals or legal documents. Additionally, you could experiment with the different configuration options, such as the DPI, parallel factor, and editor model, to see how they impact the speed and accuracy of the conversion process.
This summary was produced with help from an AI and may contain inaccuracies - check out the links to read the original source documents!
Related Models
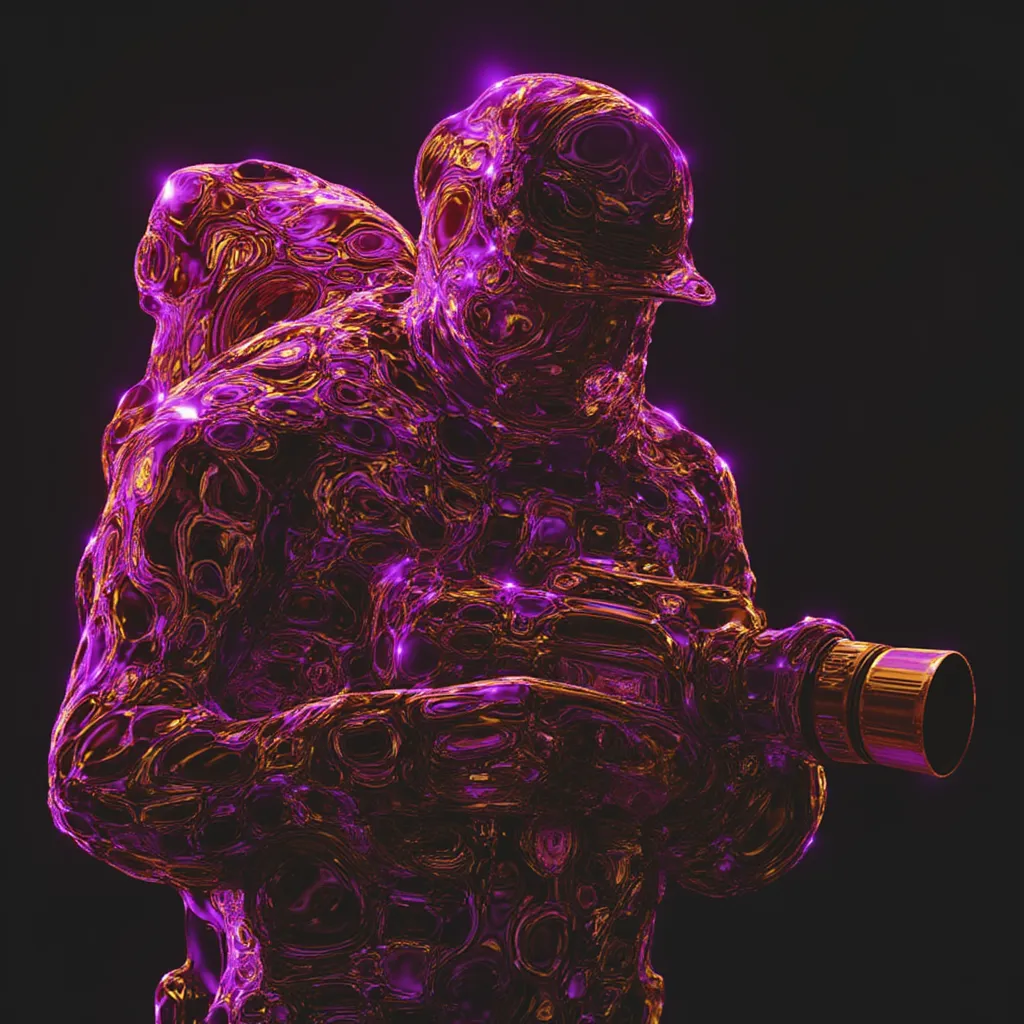
cogvideox-5b

1
cogvideox-5b is a powerful AI model developed by cuuupid that can generate high-quality videos from a text prompt. It is similar to other text-to-video models like video-crafter, cogvideo, and damo-text-to-video, but with its own unique capabilities and approach. Model inputs and outputs cogvideox-5b takes in a text prompt, guidance scale, number of output videos, and a seed for reproducibility. It then generates one or more high-quality videos based on the input prompt. The outputs are video files that can be downloaded and used for a variety of purposes. Inputs Prompt**: The text prompt that describes the video you want to generate Guidance**: The scale for classifier-free guidance, which can improve adherence to the prompt Num Outputs**: The number of output videos to generate Seed**: A seed value for reproducibility Outputs Video files**: The generated videos based on the input prompt Capabilities cogvideox-5b is capable of generating a wide range of high-quality videos from text prompts. It can create videos with realistic scenes, characters, and animations that closely match the provided prompt. The model leverages advanced techniques in text-to-video generation to produce visually striking and compelling output. What can I use it for? You can use cogvideox-5b to create videos for a variety of purposes, such as: Generating promotional or marketing videos for your business Creating educational or explainer videos Producing narrative or cinematic videos for films or animations Generating concept videos for product development or design Things to try Some ideas for things to try with cogvideox-5b include: Experimenting with different prompts to see the range of videos the model can generate Trying out different guidance scale and step settings to find the optimal balance of quality and consistency Generating multiple output videos from the same prompt to see the variations in the results Combining cogvideox-5b with other AI models or tools for more complex video production workflows
Updated Invalid Date
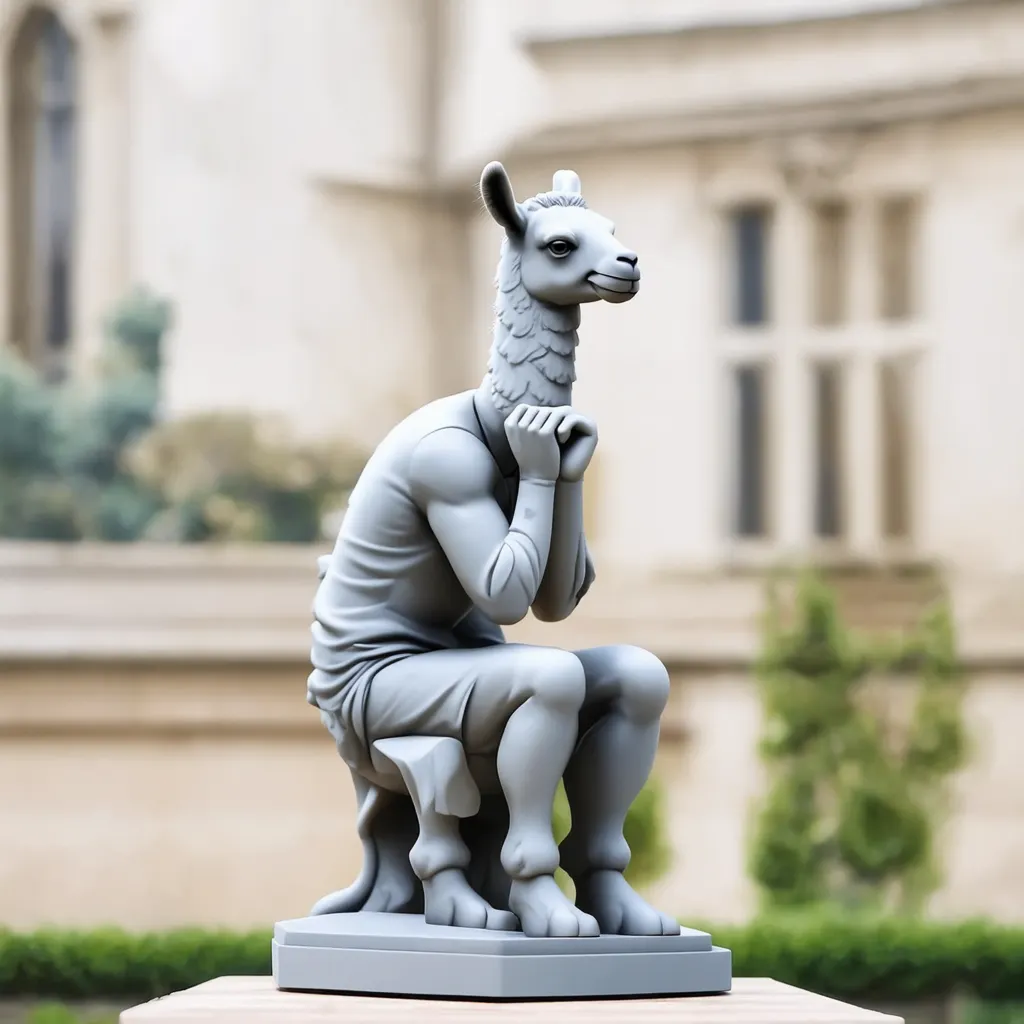
gte-qwen2-7b-instruct

48
The gte-qwen2-7b-instruct is the latest model in the General Text Embedding (GTE) family from Alibaba NLP. It is a large language model based on the Qwen2-7B model, with an embedding dimension of 3584 and a maximum input length of 32,000 tokens. The model has been fine-tuned for improved performance on the Massive Text Embedding Benchmark (MTEB), ranking first in both English and Chinese evaluations. Compared to the previous gte-Qwen1.5-7B-instruct model, the gte-qwen2-7b-instruct utilizes the upgraded Qwen2-7B base model, which incorporates several key advancements like bidirectional attention mechanisms and comprehensive training across a vast, multilingual text corpus. This results in consistent performance enhancements over the previous model. The GTE model series from Alibaba NLP also includes other variants like GTE-large-zh, GTE-base-en-v1.5, and gte-Qwen1.5-7B-instruct, catering to different language requirements and model sizes. Model inputs and outputs Inputs Text**: An array of strings representing the texts to be embedded. Outputs Output**: An array of numbers representing the embedding vector for the input text. Capabilities The gte-qwen2-7b-instruct model excels at general text embedding tasks, consistently ranking at the top of the MTEB and C-MTEB benchmarks. It demonstrates strong performance across a variety of languages and domains, making it a versatile choice for applications that require high-quality text representations. What can I use it for? The gte-qwen2-7b-instruct model can be leveraged for a wide range of applications that benefit from powerful text embeddings, such as: Information retrieval and search Text classification and clustering Semantic similarity detection Recommendation systems Data augmentation and generation The model's impressive performance on the MTEB and C-MTEB benchmarks suggests it could be particularly useful for tasks that require cross-lingual or multilingual text understanding. Things to try One interesting aspect of the gte-qwen2-7b-instruct model is its integration of bidirectional attention mechanisms, which can enhance its contextual understanding. Experimenting with different prompts or input formats to leverage this capability could yield interesting insights. Additionally, the model's large size and comprehensive training corpus make it well-suited for transfer learning or fine-tuning on domain-specific tasks. Exploring how the model's embeddings perform on various downstream applications could uncover new use cases and opportunities.
Updated Invalid Date
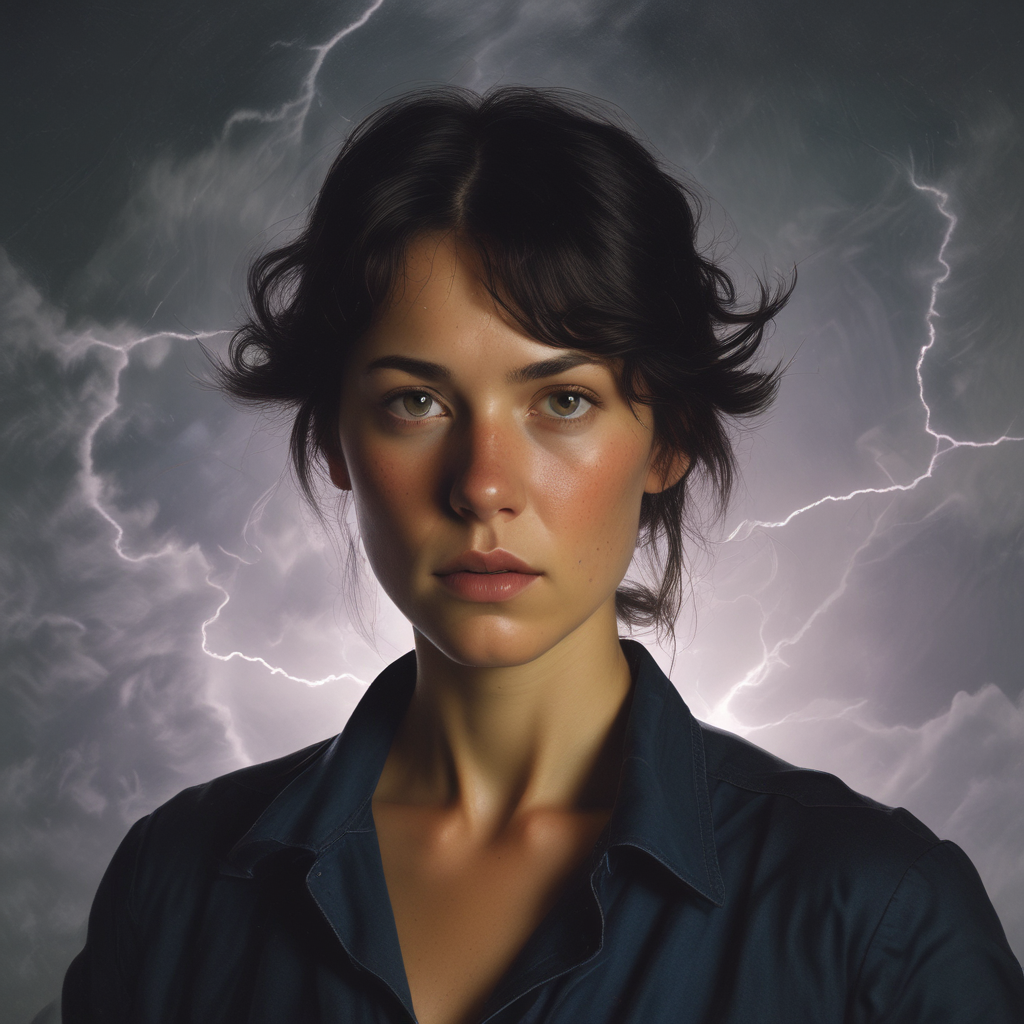
sdxl-lightning-4step

453.2K
sdxl-lightning-4step is a fast text-to-image model developed by ByteDance that can generate high-quality images in just 4 steps. It is similar to other fast diffusion models like AnimateDiff-Lightning and Instant-ID MultiControlNet, which also aim to speed up the image generation process. Unlike the original Stable Diffusion model, these fast models sacrifice some flexibility and control to achieve faster generation times. Model inputs and outputs The sdxl-lightning-4step model takes in a text prompt and various parameters to control the output image, such as the width, height, number of images, and guidance scale. The model can output up to 4 images at a time, with a recommended image size of 1024x1024 or 1280x1280 pixels. Inputs Prompt**: The text prompt describing the desired image Negative prompt**: A prompt that describes what the model should not generate Width**: The width of the output image Height**: The height of the output image Num outputs**: The number of images to generate (up to 4) Scheduler**: The algorithm used to sample the latent space Guidance scale**: The scale for classifier-free guidance, which controls the trade-off between fidelity to the prompt and sample diversity Num inference steps**: The number of denoising steps, with 4 recommended for best results Seed**: A random seed to control the output image Outputs Image(s)**: One or more images generated based on the input prompt and parameters Capabilities The sdxl-lightning-4step model is capable of generating a wide variety of images based on text prompts, from realistic scenes to imaginative and creative compositions. The model's 4-step generation process allows it to produce high-quality results quickly, making it suitable for applications that require fast image generation. What can I use it for? The sdxl-lightning-4step model could be useful for applications that need to generate images in real-time, such as video game asset generation, interactive storytelling, or augmented reality experiences. Businesses could also use the model to quickly generate product visualization, marketing imagery, or custom artwork based on client prompts. Creatives may find the model helpful for ideation, concept development, or rapid prototyping. Things to try One interesting thing to try with the sdxl-lightning-4step model is to experiment with the guidance scale parameter. By adjusting the guidance scale, you can control the balance between fidelity to the prompt and diversity of the output. Lower guidance scales may result in more unexpected and imaginative images, while higher scales will produce outputs that are closer to the specified prompt.
Updated Invalid Date
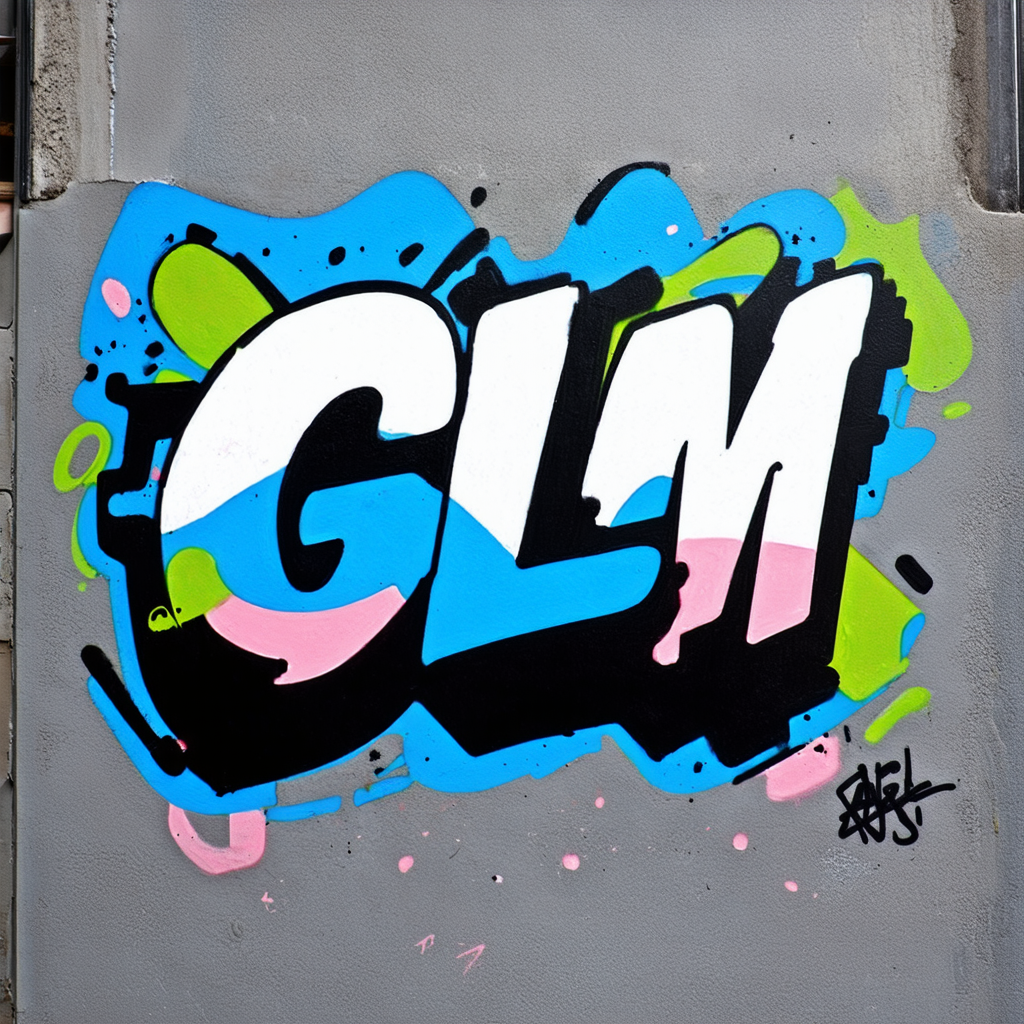
glm-4v-9b

3.2K
glm-4v-9b is a powerful multimodal language model developed by Tsinghua University that demonstrates state-of-the-art performance on several benchmarks, including optical character recognition (OCR). It is part of the GLM-4 series of models, which includes the base glm-4-9b model as well as the glm-4-9b-chat and glm-4-9b-chat-1m chat-oriented models. The glm-4v-9b model specifically adds visual understanding capabilities, allowing it to excel at tasks like image description, visual question answering, and multimodal reasoning. Compared to similar models like sdxl-lightning-4step and cogvlm, the glm-4v-9b model stands out for its strong performance across a wide range of multimodal benchmarks, as well as its support for both Chinese and English languages. It has been shown to outperform models like GPT-4, Gemini 1.0 Pro, and Claude 3 Opus on these tasks. Model inputs and outputs Inputs Image**: An image to be used as input for the model Prompt**: A text prompt describing the task or query for the model Outputs Output**: The model's response, which could be a textual description of the input image, an answer to a visual question, or the result of a multimodal reasoning task. Capabilities The glm-4v-9b model demonstrates strong multimodal understanding and generation capabilities. It can generate detailed, coherent descriptions of input images, answer questions about the visual content, and perform tasks like visual reasoning and optical character recognition. For example, the model can analyze a complex chart or diagram and provide a summary of the key information and insights. What can I use it for? The glm-4v-9b model could be a valuable tool for a variety of applications that require multimodal intelligence, such as: Intelligent image captioning and visual question answering for social media, e-commerce, or creative applications Multimodal document understanding and analysis for business intelligence or research tasks Multimodal conversational AI assistants that can engage in visual and textual dialogue The model's strong performance and broad capabilities make it a compelling option for developers and researchers looking to push the boundaries of what's possible with language models and multimodal AI. Things to try One interesting thing to try with the glm-4v-9b model is exploring its ability to perform multimodal reasoning tasks. For example, you could provide the model with an image and a textual prompt that requires analyzing the visual information and drawing inferences. This could involve tasks like answering questions about the relationships between objects in the image, identifying anomalies or inconsistencies, or generating hypothetical scenarios based on the visual content. Another area to explore is the model's potential for multimodal content generation. You could experiment with providing the model with a combination of image and text inputs, and see how it can generate new, creative content that seamlessly integrates the visual and textual elements.
Updated Invalid Date