speaker-diarization
Maintainer: lucataco

9
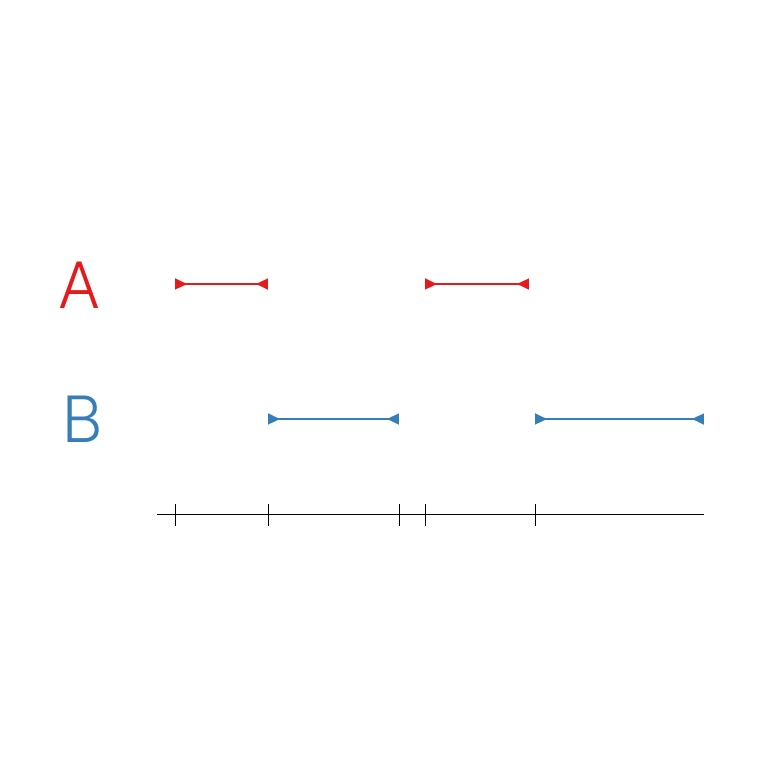
Property | Value |
---|---|
Run this model | Run on Replicate |
API spec | View on Replicate |
Github link | View on Github |
Paper link | No paper link provided |
Create account to get full access
Model overview
The speaker-diarization
model is an AI-powered tool that can segment an audio recording based on who is speaking. It uses a pre-trained speaker diarization pipeline from the pyannote.audio
package, which is an open-source toolkit for speaker diarization based on PyTorch. The model is capable of identifying individual speakers within an audio recording and providing information about the start and stop times of each speaker's segment, as well as speaker embeddings that can be used for speaker recognition. This model is similar to other audio-related models created by lucataco, such as whisperspeech-small, xtts-v2, and magnet.
Model inputs and outputs
The speaker-diarization
model takes a single input: an audio file in a variety of supported formats, including MP3, AAC, FLAC, OGG, OPUS, and WAV. The model processes the audio and outputs a JSON file containing information about the identified speakers, including the start and stop times of each speaker's segment, the number of detected speakers, and speaker embeddings that can be used for speaker recognition.
Inputs
- Audio: An audio file in a supported format (e.g., MP3, AAC, FLAC, OGG, OPUS, WAV)
Outputs
- Output.json: A JSON file containing the following information:
segments
: A list of objects, each representing a detected speaker segment, with the speaker label, start time, and end timespeakers
: An object containing the number of detected speakers, their labels, and the speaker embeddings for each speaker
Capabilities
The speaker-diarization
model can effectively segment an audio recording and identify the individual speakers. This can be useful for a variety of applications, such as transcription and captioning tasks, as well as speaker recognition. The model's ability to generate speaker embeddings can be particularly valuable for building speaker recognition systems.
What can I use it for?
The speaker-diarization
model can be used for a variety of data augmentation and segmentation tasks, such as processing interview recordings, podcast episodes, or meeting recordings. The speaker segmentation and embedding information provided by the model can be used to enhance transcription and captioning tasks, as well as to implement speaker recognition systems that can identify specific speakers within an audio recording.
Things to try
One interesting thing to try with the speaker-diarization
model is to experiment with the speaker embeddings it generates. These embeddings can be used to build speaker recognition systems that can identify specific speakers within an audio recording. You could try matching the speaker embeddings against a database of known speakers, or using them as input features for a machine learning model that can classify speakers.
Another thing to try is to use the speaker segmentation information provided by the model to enhance transcription and captioning tasks. By knowing where each speaker's segments begin and end, you can potentially improve the accuracy of the transcription or captioning, especially in cases where there is overlapping speech.
This summary was produced with help from an AI and may contain inaccuracies - check out the links to read the original source documents!
Related Models
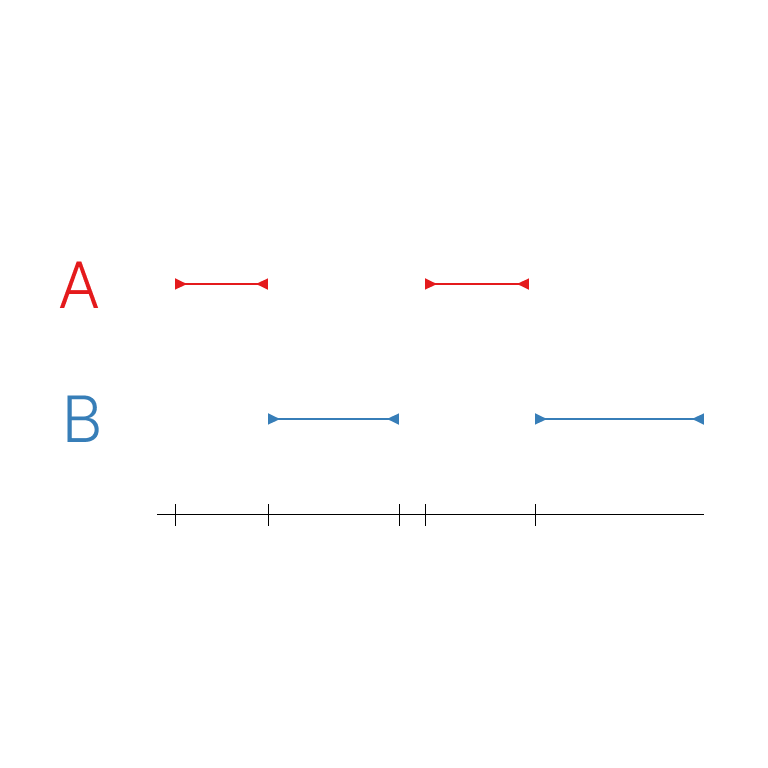
speaker-diarization

42
The speaker-diarization model from Replicate creator meronym is a tool that segments an audio recording based on who is speaking. It is built using the open-source pyannote.audio library, which provides a set of trainable end-to-end neural building blocks for speaker diarization. This model is similar to other speaker diarization models available, such as speaker-diarization and whisper-diarization, which also leverage the pyannote.audio library. However, the speaker-diarization model from meronym specifically uses a pre-trained pipeline that combines speaker segmentation, embedding, and clustering to identify individual speakers within the audio. Model inputs and outputs Inputs audio**: The audio file to be processed, in a supported format such as MP3, AAC, FLAC, OGG, OPUS, or WAV. Outputs The model outputs a JSON file with the following structure: segments: A list of diarization segments, each with a speaker label, start time, and end time. speakers: An object containing the number of detected speakers, their labels, and 192-dimensional speaker embedding vectors. Capabilities The speaker-diarization model is capable of automatically identifying individual speakers within an audio recording, even in cases where there is overlapping speech. It can handle a variety of audio formats and sample rates, and provides both segmentation information and speaker embeddings as output. What can I use it for? This model can be useful for a variety of applications, such as: Data Augmentation**: The speaker diarization output can be used to enhance transcription and captioning tasks by providing speaker-level segmentation. Speaker Recognition**: The speaker embeddings generated by the model can be used to match against a database of known speakers, enabling speaker identification and verification. Meeting and Interview Analysis**: The speaker diarization output can be used to analyze meeting recordings or interviews, providing insights into speaker participation, turn-taking, and interaction patterns. Things to try One interesting aspect of the speaker-diarization model is its ability to handle overlapping speech. You could experiment with audio files that contain multiple speakers talking simultaneously, and observe how the model segments and labels the different speakers. Additionally, you could explore the use of the speaker embeddings for tasks like speaker clustering or identification, and see how the model's performance compares to other approaches.
Updated Invalid Date
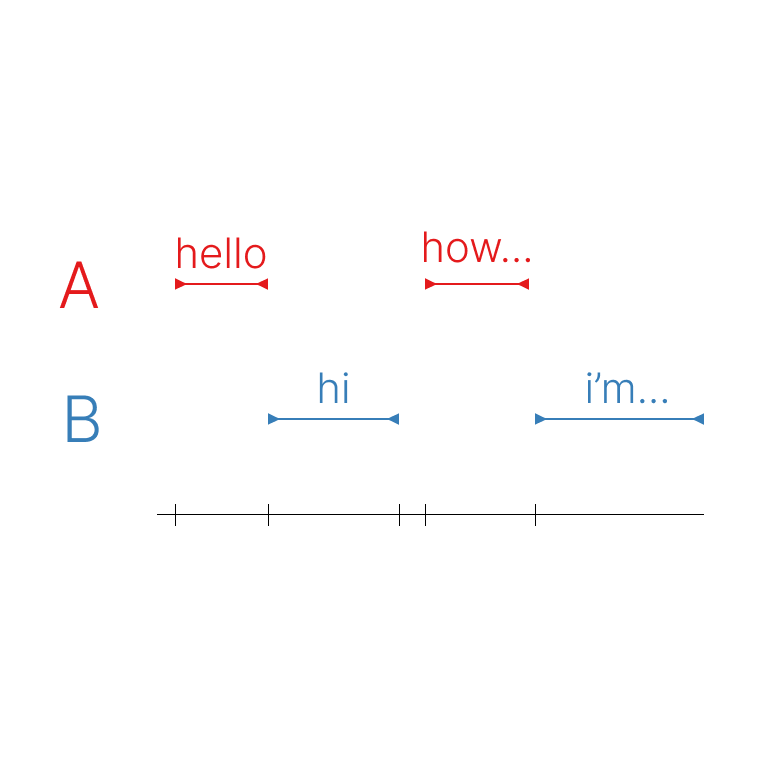
speaker-transcription

21
The speaker-transcription model is a powerful AI system that combines speaker diarization and speech transcription capabilities. It was developed by Meronym, a creator on the Replicate platform. This model builds upon two main components: the pyannote.audio speaker diarization pipeline and OpenAI's whisper model for general-purpose English speech transcription. The speaker-transcription model outperforms similar models like whisper-diarization and whisperx by providing more accurate speaker segmentation and identification, as well as high-quality transcription. It can be particularly useful for tasks that require both speaker information and verbatim transcripts, such as interview analysis, podcast processing, or meeting recordings. Model inputs and outputs The speaker-transcription model takes an audio file as input and can optionally accept a prompt string to guide the transcription. The model outputs a JSON file containing the transcribed segments, with each segment associated with a speaker label and timestamps. Inputs Audio**: An audio file in a supported format, such as MP3, AAC, FLAC, OGG, OPUS, or WAV. Prompt (optional)**: A text prompt that can be used to provide additional context for the transcription. Outputs JSON file**: A JSON file with the following structure: segments: A list of transcribed segments, each with a speaker label, start and stop timestamps, and the segment transcript. speakers: Information about the detected speakers, including the total count, labels for each speaker, and embeddings (a vector representation of each speaker's voice). Capabilities The speaker-transcription model excels at accurately identifying and labeling different speakers within an audio recording, while also providing high-quality transcripts of the spoken content. This makes it a valuable tool for a variety of applications, such as interview analysis, podcast processing, or meeting recordings. What can I use it for? The speaker-transcription model can be used for data augmentation and segmentation tasks, where the speaker information and timestamps can be used to improve the accuracy and effectiveness of transcription and captioning models. Additionally, the speaker embeddings generated by the model can be used for speaker recognition, allowing you to match voice profiles against a database of known speakers. Things to try One interesting aspect of the speaker-transcription model is the ability to use a prompt to guide the transcription. By providing additional context about the topic or subject matter, you can potentially improve the accuracy and relevance of the transcripts. Try experimenting with different prompts to see how they affect the output. Another useful feature is the generation of speaker embeddings, which can be used for speaker recognition and identification tasks. Consider exploring ways to leverage these embeddings, such as building a speaker verification system or clustering speakers in large audio datasets.
Updated Invalid Date
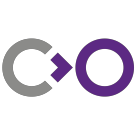
whisperspeech-small

1
whisperspeech-small is an open-source text-to-speech system built by inverting the Whisper speech recognition model. It was developed by lucataco, a contributor at Replicate. This model can be used to generate audio from text, allowing users to create their own text-to-speech applications. whisperspeech-small is similar to other open-source text-to-speech models like whisper-diarization, whisperx, and voicecraft, which leverage the capabilities of the Whisper speech recognition model in different ways. Model Inputs and Outputs whisperspeech-small takes a text prompt as input and generates an audio file as output. The model can handle various languages, and users can optionally provide a speaker audio file for zero-shot voice cloning. Inputs Prompt**: The text to be synthesized into speech Speaker**: URL of an audio file for zero-shot voice cloning (optional) Language**: The language of the text to be synthesized Outputs Audio File**: The generated speech audio file Capabilities whisperspeech-small can generate high-quality speech audio from text in a variety of languages. The model uses the Whisper speech recognition architecture to generate the audio, which results in natural-sounding speech. The zero-shot voice cloning feature also allows users to customize the voice used for the synthesized speech. What Can I Use It For? whisperspeech-small can be used to create text-to-speech applications, such as audiobook narration, language learning tools, or accessibility features for websites and applications. The model's ability to generate speech in multiple languages makes it useful for international or multilingual projects. Additionally, the zero-shot voice cloning feature allows for more personalized or branded text-to-speech outputs. Things to Try One interesting thing to try with whisperspeech-small is using the zero-shot voice cloning feature to generate speech that matches the voice of a specific person or character. This could be useful for creating audiobooks, podcasts, or interactive voice experiences. Another idea is to experiment with different text prompts and language settings to see how the model handles a variety of input content.
Updated Invalid Date
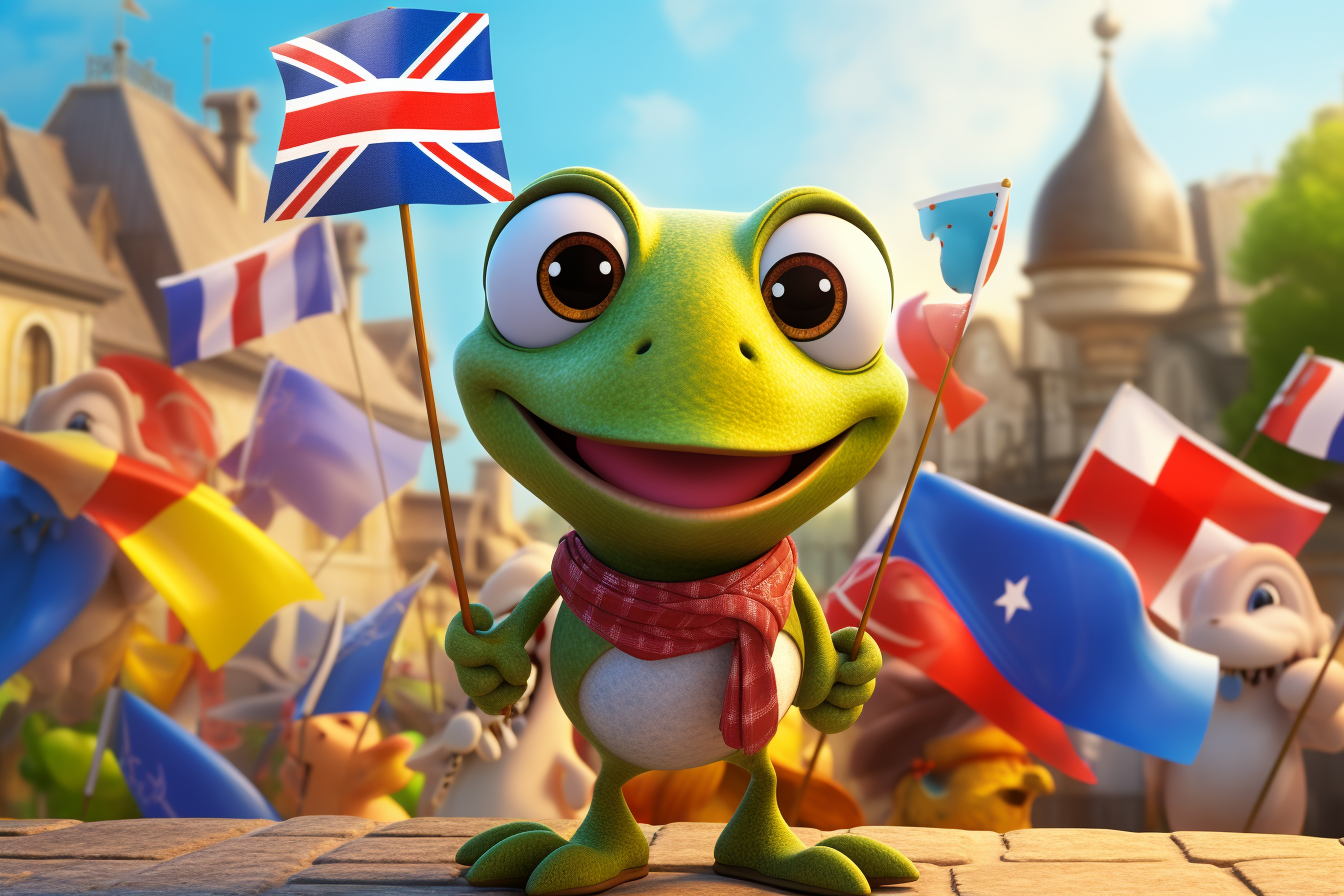
xtts-v2

316
The xtts-v2 model is a multilingual text-to-speech voice cloning system developed by lucataco, the maintainer of this Cog implementation. This model is part of the Coqui TTS project, an open-source text-to-speech library. The xtts-v2 model is similar to other text-to-speech models like whisperspeech-small, styletts2, and qwen1.5-110b, which also generate speech from text. Model inputs and outputs The xtts-v2 model takes three main inputs: text to synthesize, a speaker audio file, and the output language. It then produces a synthesized audio file of the input text spoken in the voice of the provided speaker. Inputs Text**: The text to be synthesized Speaker**: The original speaker audio file (wav, mp3, m4a, ogg, or flv) Language**: The output language for the synthesized speech Outputs Output**: The synthesized audio file Capabilities The xtts-v2 model can generate high-quality multilingual text-to-speech audio by cloning the voice of a provided speaker. This can be useful for a variety of applications, such as creating personalized audio content, improving accessibility, or enhancing virtual assistants. What can I use it for? The xtts-v2 model can be used to create personalized audio content, such as audiobooks, podcasts, or video narrations. It could also be used to improve accessibility by generating audio versions of written content for users with visual impairments or other disabilities. Additionally, the model could be integrated into virtual assistants or chatbots to provide a more natural, human-like voice interface. Things to try One interesting thing to try with the xtts-v2 model is to experiment with different speaker audio files to see how the synthesized voice changes. You could also try using the model to generate audio in various languages and compare the results. Additionally, you could explore ways to integrate the model into your own applications or projects to enhance the user experience.
Updated Invalid Date