AI Models
Browse and discover AI models across various categories.
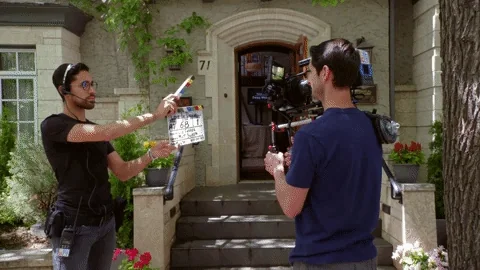
llava-next-video

2.5K
llava-next-video is a large language and vision model developed by the team led by Chunyuan Li that can process and understand video content. It is part of the LLaVA-NeXT family of models, which aims to build powerful multimodal AI systems that can excel across a wide range of visual and language tasks. Unlike similar models like whisperx-video-transcribe and insanely-fast-whisper-with-video that focus on video transcription, llava-next-video can understand and reason about video content at a high level, going beyond just transcription. Model inputs and outputs llava-next-video takes a video file as input and a prompt that describes what the user wants to know about the video. The model can then generate a textual response that answers the prompt, drawing insights and understanding from the video content. Inputs Video**: The input video file that the model will process and reason about Prompt**: A natural language prompt that describes what the user wants to know about the video Outputs Text response**: A textual response generated by the model that answers the given prompt based on its understanding of the video Capabilities llava-next-video can perform a variety of tasks related to video understanding, such as: Answering questions about the content and events in a video Summarizing the key points or storyline of a video Describing the actions, objects, and people shown in a video Providing insights and analysis on the meaning or significance of a video The model is trained on a large and diverse dataset of videos, allowing it to develop robust capabilities for understanding visual information and reasoning about it in natural language. What can I use it for? llava-next-video could be useful for a variety of applications, such as: Building intelligent video assistants that can help users find information and insights in video content Automating the summarization and analysis of video content for businesses or media organizations Integrating video understanding capabilities into chatbots or virtual assistants to make them more multimodal and capable Developing educational or training applications that leverage video content in interactive and insightful ways Things to try One interesting thing to try with llava-next-video is to ask it open-ended questions about a video that go beyond just describing the content. For example, you could ask the model to analyze the emotional tone of a video, speculate on the motivations of the characters, or draw connections between the video and broader cultural or social themes. The model's ability to understand and reason about video content at a deeper level can lead to surprising and insightful responses.
Updated 10/5/2024
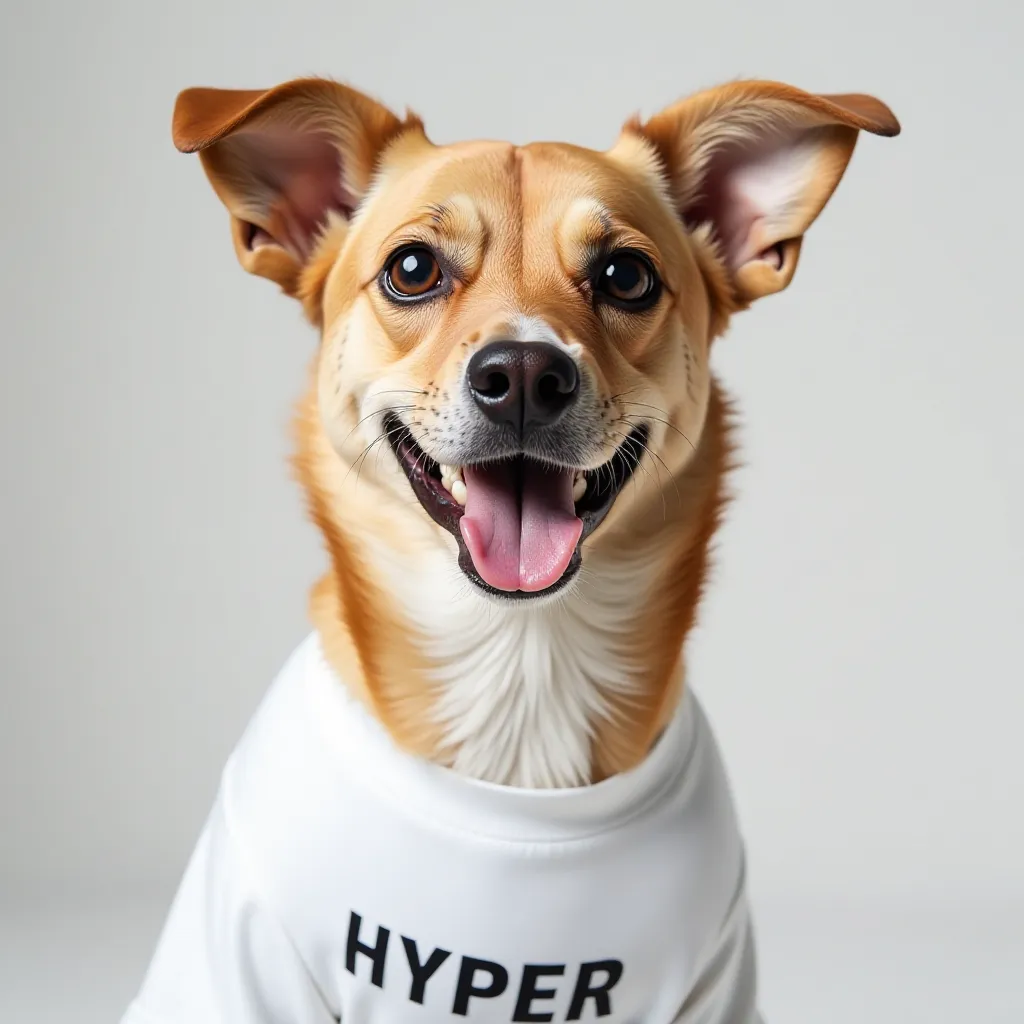
New!hyper-flux-8step

943
hyper-flux-8step is a text-to-image AI model developed by ByteDance. It is a variant of the ByteDance/Hyper-SD FLUX.1-dev model, which is a diffusion-based model trained to generate high-quality images from textual descriptions. The hyper-flux-8step version uses an 8-step inference process, compared to the 16-step process of the original Hyper FLUX model. This makes it faster to run while still producing compelling images. The model is similar to other AI text-to-image models like sdxl-lightning-4step and hyper-flux-16step, all of which are developed by ByteDance. These models offer varying trade-offs between speed, quality, and resource requirements. Model inputs and outputs The hyper-flux-8step model takes a text prompt as input and generates one or more corresponding images as output. The input prompt can describe a wide variety of subjects, scenes, and styles, and the model will attempt to create visuals that match the description. Inputs Prompt**: A text description of the image you want the model to generate. Seed**: A random seed value to ensure reproducible generation. Width/Height**: The desired width and height of the generated image, if using a custom aspect ratio. Num Outputs**: The number of images to generate (up to 4). Aspect Ratio**: The aspect ratio of the generated image, such as 1:1 or custom. Output Format**: The file format for the generated images, such as WEBP or PNG. Guidance Scale**: A parameter that controls the strength of the text-to-image guidance. Num Inference Steps**: The number of steps to use in the diffusion process (8 in this case). Disable Safety Checker**: An option to disable the model's safety checks for inappropriate content. Outputs One or more image files in the requested format, corresponding to the provided prompt. Capabilities The hyper-flux-8step model is capable of generating a wide variety of high-quality images from textual descriptions. It can create realistic scenes, fantastical creatures, abstract art, and more. The 8-step inference process makes it faster to use compared to the 16-step version, while still producing compelling results. What can I use it for? You can use hyper-flux-8step to generate custom images for a variety of applications, such as: Illustrations for articles, blog posts, or social media Concept art for games, films, or other creative projects Product visualizations or mockups Unique artwork and designs for personal or commercial use The speed and quality of the generated images make it a useful tool for rapid prototyping, ideation, and content creation. Things to try Some interesting things you could try with the hyper-flux-8step model include: Generating images with specific art styles or aesthetics by including relevant keywords in the prompt. Experimenting with different aspect ratios and image sizes to see how the model handles different output formats. Trying out the [disable_safety_checker] option to see how it affects the generated images (while being mindful of potential issues). Combining the hyper-flux-8step model with other AI tools or workflows to create more complex visual content. The key is to explore the model's capabilities and see how it can fit into your creative or business needs.
Updated 10/5/2024
🏅
New!whisper-large-v3-turbo

489
The whisper-large-v3-turbo model is a finetuned version of the pruned Whisper large-v3 model. It is the exact same model, except that the number of decoding layers have been reduced from 32 to 4, making the model significantly faster while only experiencing a minor quality degradation. The Whisper model was proposed by Alec Radford et al. from OpenAI and demonstrates strong generalization across many datasets and domains in a zero-shot setting. Model inputs and outputs The whisper-large-v3-turbo model is designed for automatic speech recognition (ASR) and speech translation. It takes audio samples as input and outputs text transcriptions. Inputs Audio samples**: The model accepts arbitrary length audio inputs, which it can process efficiently using a chunked inference algorithm. Outputs Text transcriptions**: The model outputs text transcriptions of the input audio, either in the same language as the audio (for ASR) or in a different language (for speech translation). Timestamps**: The model can optionally provide timestamps for each transcribed sentence or word. Capabilities The whisper-large-v3-turbo model exhibits improved robustness to accents, background noise, and technical language compared to many existing ASR systems. It also demonstrates strong zero-shot translation capabilities, allowing it to transcribe audio in one language and output the text in a different language. What can I use it for? The whisper-large-v3-turbo model is primarily intended for AI researchers studying the capabilities, biases, and limitations of large language models. However, it can also be a useful ASR solution for developers, especially for English speech recognition tasks. The speed and accuracy of the model suggest that others may be able to build applications on top of it that allow for near-real-time speech recognition and translation. Things to try One key capability to explore with the whisper-large-v3-turbo model is its ability to handle long-form audio. By using the chunked inference algorithm provided in the Transformers library, the model can efficiently transcribe audio files of arbitrary length. Developers could experiment with using this feature to build applications that provide accurate transcriptions of podcasts, interviews, or other long-form audio content. Another interesting aspect to investigate is the model's performance on non-English languages and its zero-shot translation capabilities. Users could try transcribing audio in different languages and evaluating the quality of the translations to English, as well as exploring ways to fine-tune the model for specific language pairs or domains.
Updated 10/5/2024
🏅
whisper-large-v3-turbo

489
The whisper-large-v3-turbo model is a finetuned version of the Whisper large-v3 model, a state-of-the-art automatic speech recognition (ASR) and speech translation model proposed by Alec Radford et al. from OpenAI. Trained on over 5 million hours of labeled data, Whisper demonstrates strong generalization to many datasets and domains without the need for fine-tuning. The whisper-large-v3-turbo model has a reduced number of decoding layers from 32 to 4, resulting in a faster model but with a minor quality degradation. Model inputs and outputs The whisper-large-v3-turbo model takes audio samples as input and generates transcribed text as output. It can be used for both speech recognition, where the output is in the same language as the input audio, as well as speech translation, where the output is in a different language. Inputs Audio samples**: The model accepts raw audio waveforms sampled at 16kHz or 44.1kHz. Outputs Transcribed text**: The model generates text transcriptions of the input audio. Timestamps (optional)**: The model can also generate timestamps indicating the start and end time of each transcribed segment. Capabilities The Whisper models demonstrate strong performance on speech recognition and translation tasks, exhibiting improved robustness to accents, background noise, and technical language compared to many existing ASR systems. The models can also perform zero-shot translation from multiple languages into English. What can I use it for? The whisper-large-v3-turbo model can be useful for a variety of applications, such as: Transcription and translation**: The model can be used to transcribe audio in various languages and translate it to English or other target languages. Accessibility tools**: The model's transcription capabilities can be leveraged to improve accessibility, such as live captioning or subtitling for audio/video content. Voice interaction and assistants**: The model's ASR and translation abilities can be integrated into voice-based interfaces and digital assistants. Things to try One interesting aspect of the Whisper models is their ability to automatically determine the language of the input audio and perform the appropriate task (recognition or translation) without any additional prompting. You can experiment with this by providing audio samples in different languages and observing how the model handles the task. Additionally, the models support returning word-level timestamps, which can be useful for applications that require precise alignment between the transcribed text and the audio. Try using the return_timestamps="word" parameter to see the word-level timing information.
Updated 10/5/2024
📉
NVLM-D-72B

366
NVLM-D-72B is a frontier-class multimodal large language model (LLM) developed by NVIDIA. It achieves state-of-the-art results on vision-language tasks, rivaling leading proprietary models like GPT-4o and open-access models like Llama 3-V 405B and InternVL2. Remarkably, NVLM-D-72B shows improved text-only performance over its LLM backbone after multimodal training. Model Inputs and Outputs NVLM-D-72B is a decoder-only multimodal LLM that can take both text and images as inputs. The model outputs are primarily text, allowing it to excel at vision-language tasks like visual question answering, image captioning, and image-text retrieval. Inputs Text**: The model can take text inputs of up to 8,000 characters. Images**: The model can accept image inputs in addition to text. Outputs Text**: The model generates text outputs, which can be used for a variety of vision-language tasks. Capabilities NVLM-D-72B demonstrates strong performance on a range of multimodal benchmarks, including MMMU, MathVista, OCRBench, AI2D, ChartQA, DocVQA, TextVQA, RealWorldQA, and VQAv2. It outperforms many leading models in these areas, making it a powerful tool for vision-language applications. What can I use it for? NVLM-D-72B is well-suited for a variety of vision-language applications, such as: Visual Question Answering**: The model can answer questions about the content and context of an image. Image Captioning**: The model can generate detailed captions describing the contents of an image. Image-Text Retrieval**: The model can match images with relevant textual descriptions and vice versa. Multimodal Reasoning**: The model can combine information from text and images to perform advanced reasoning tasks. Things to try One key insight about NVLM-D-72B is its ability to maintain and even improve on its text-only performance after multimodal training. This suggests that the model has learned to effectively integrate visual and textual information, making it a powerful tool for a wide range of vision-language applications.
Updated 10/5/2024
🖼️
MiniCPM-Embedding

209
MiniCPM-Embedding is a bilingual and cross-lingual text embedding model developed by ModelBest Inc. and THUNLP. It is trained based on MiniCPM-2B-sft-bf16 and incorporates bidirectional attention and Weighted Mean Pooling. The model was trained on approximately 6 million examples, including open-source, synthetic, and proprietary data, to achieve exceptional Chinese and English retrieval capabilities as well as outstanding cross-lingual retrieval between the two languages. Model inputs and outputs Inputs Instruction: {{ instruction }} Query: {{ query }} - MiniCPM-Embedding supports query-side instructions in this format. Query: {{ query }} - MiniCPM-Embedding also works in instruction-free mode. Outputs The model generates text outputs in response to the provided input queries. Capabilities MiniCPM-Embedding features exceptional capabilities in Chinese and English text retrieval, as well as outstanding cross-lingual retrieval between the two languages. This makes it a powerful tool for tasks that require understanding and retrieving information across multiple languages. What can I use it for? With its strong bilingual and cross-lingual text embedding abilities, MiniCPM-Embedding can be useful for a variety of applications, such as: Cross-lingual information retrieval Multilingual question answering Bilingual document classification and clustering Multilingual text summarization Things to try Explore the other models in the RAG toolkit series, such as MiniCPM-Reranker and MiniCPM3-RAG-LoRA, to see how they can be used in conjunction with MiniCPM-Embedding for more advanced retrieval and ranking tasks.
Updated 10/5/2024
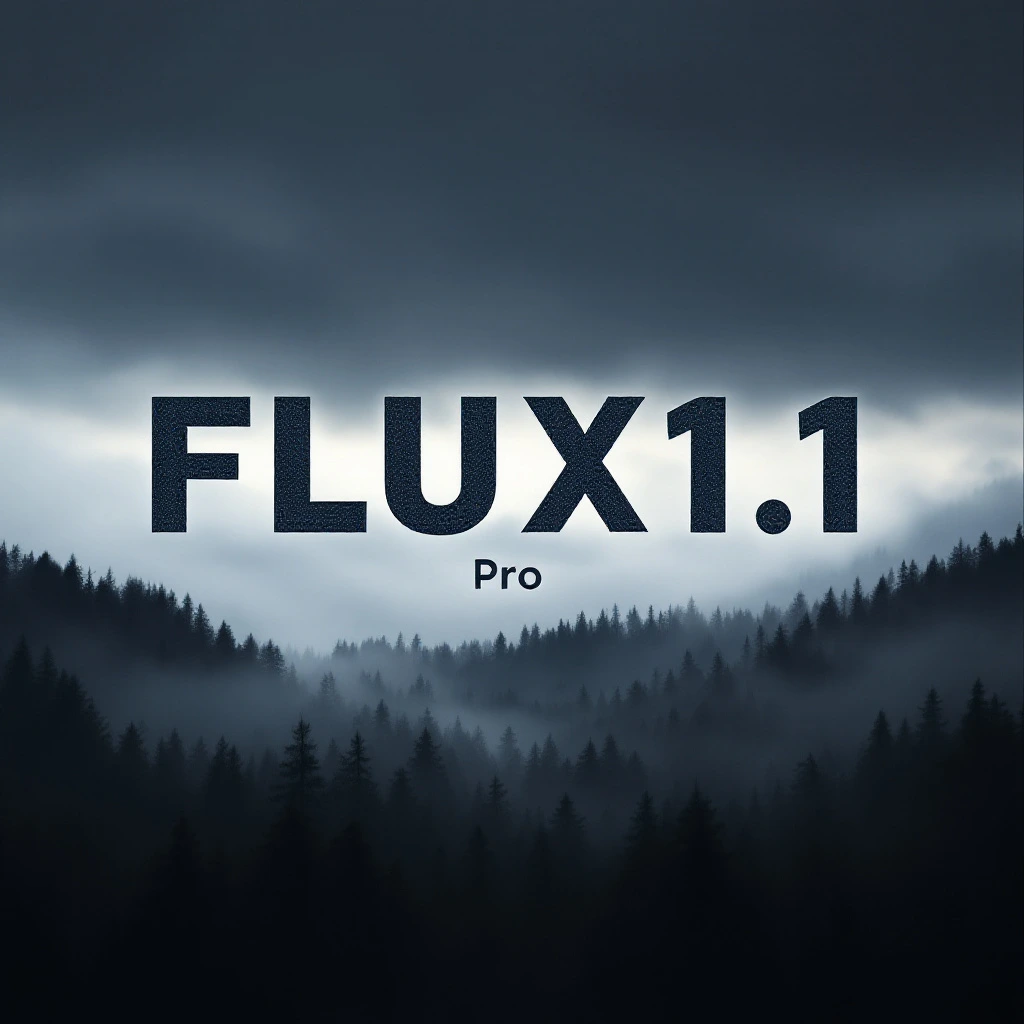
New!flux-1.1-pro

181
The flux-1.1-pro model is a powerful text-to-image AI model developed by black-forest-labs. It builds upon the capabilities of the flux-pro model, offering even faster generation and improved image quality, prompt adherence, and output diversity. Compared to similar models like flux-schnell, flux-dev, and [FLUX.1 [schnell]](https://aimodels.fyi/models/replicate/flux1-schnell-black-forest-labs), the flux-1.1-pro model strikes a balance between speed, quality, and creativity. Model inputs and outputs The flux-1.1-pro model takes a text prompt as input and generates a corresponding image. The input schema includes parameters for setting the image size, aspect ratio, output format, and safety tolerance. The model outputs a single image file in the specified format, which can be used for a variety of creative and practical applications. Inputs Prompt**: The text prompt describing the desired image Seed**: A random seed for reproducible generation Width**: The width of the generated image (only used with custom aspect ratio) Height**: The height of the generated image (only used with custom aspect ratio) Aspect Ratio**: The aspect ratio of the generated image Output Format**: The file format of the output image Output Quality**: The quality level of the output image (not relevant for PNG) Safety Tolerance**: The level of content filtering for the generated image Outputs Image**: A single image file in the specified format Capabilities The flux-1.1-pro model excels at generating high-quality, diverse images that closely match the provided text prompt. It leverages advanced machine learning techniques to capture intricate details, maintain visual coherence, and deliver a wide range of creative outputs. Compared to the previous flux-pro model, the flux-1.1-pro offers faster generation and improved prompt adherence, making it an ideal choice for a wide range of text-to-image applications. What can I use it for? The flux-1.1-pro model is a versatile tool that can be used for a variety of creative and practical applications. Artists and designers can use it to generate concept art, storyboards, and illustrations. Marketers and content creators can leverage it to produce visual assets for social media, advertisements, and presentations. Educators and researchers can explore its capabilities for data visualization, educational materials, and prototyping. The model's versatility and high-quality outputs make it a valuable asset for anyone working with visual content. Things to try One interesting aspect of the flux-1.1-pro model is its ability to generate diverse outputs from the same prompt. By adjusting the seed parameter, you can create multiple variations of a single concept, enabling you to explore different creative directions and find the perfect image for your needs. Additionally, experimenting with the prompt upsampling feature can lead to more creative and unexpected results, allowing you to push the boundaries of what's possible with text-to-image generation.
Updated 10/5/2024
🐍
Emu3-Gen

101
Emu3 is a powerful multimodal AI model developed by the Beijing Academy of Artificial Intelligence (BAAI). Unlike traditional models that require separate architectures for different tasks, Emu3 is trained solely on next-token prediction, allowing it to excel at both generation and perception across a wide range of modalities. The model outperforms several well-established task-specific models, including SDXL, LLaVA-1.6, and OpenSora-1.2, while eliminating the need for diffusion or compositional architectures. Model inputs and outputs Emu3 is a versatile model that can process and generate a variety of multimodal data, including images, text, and videos. The model takes in sequences of discrete tokens and generates the next token in the sequence, allowing it to perform tasks such as image generation, text-to-image translation, and video prediction. Inputs Sequences of discrete tokens representing images, text, or videos Outputs The next token in the input sequence, which can be used to generate new content or extend existing content Capabilities Emu3 demonstrates impressive capabilities in both generation and perception tasks. It can generate high-quality images by simply predicting the next vision token, and it also shows strong vision-language understanding abilities to provide coherent text responses without relying on a CLIP or a pretrained language model. Additionally, Emu3 can generate videos by predicting the next token in a video sequence, and it can also extend existing videos to predict what will happen next. What can I use it for? The broad capabilities of Emu3 make it a valuable tool for a wide range of applications, including: Content creation: Generating high-quality images, text, and videos to support various creative projects Multimodal AI: Developing advanced AI systems that can understand and interact with multimodal data Personalization: Tailoring content and experiences to individual users based on their preferences and behavior Automation: Streamlining tasks that involve the processing or generation of multimodal data Things to try One of the key insights of Emu3 is its ability to learn from a mixture of multimodal sequences, rather than relying on task-specific architectures. This allows the model to develop a more holistic understanding of the relationships between different modalities, which can be leveraged in a variety of ways. For example, you could explore how Emu3 performs on cross-modal tasks, such as generating images from text prompts or translating text into other languages while preserving the original meaning and style.
Updated 10/5/2024
🐍
Emu3-Gen

101
Emu3 is a powerful multimodal AI model developed by the Beijing Academy of Artificial Intelligence (BAAI). Unlike traditional models that require separate architectures for different tasks, Emu3 is trained solely on next-token prediction, allowing it to excel at both generation and perception across a wide range of modalities. The model outperforms several well-established task-specific models, including SDXL, LLaVA-1.6, and OpenSora-1.2, while eliminating the need for diffusion or compositional architectures. Model inputs and outputs Emu3 is a versatile model that can process and generate a variety of multimodal data, including images, text, and videos. The model takes in sequences of discrete tokens and generates the next token in the sequence, allowing it to perform tasks such as image generation, text-to-image translation, and video prediction. Inputs Sequences of discrete tokens representing images, text, or videos Outputs The next token in the input sequence, which can be used to generate new content or extend existing content Capabilities Emu3 demonstrates impressive capabilities in both generation and perception tasks. It can generate high-quality images by simply predicting the next vision token, and it also shows strong vision-language understanding abilities to provide coherent text responses without relying on a CLIP or a pretrained language model. Additionally, Emu3 can generate videos by predicting the next token in a video sequence, and it can also extend existing videos to predict what will happen next. What can I use it for? The broad capabilities of Emu3 make it a valuable tool for a wide range of applications, including: Content creation: Generating high-quality images, text, and videos to support various creative projects Multimodal AI: Developing advanced AI systems that can understand and interact with multimodal data Personalization: Tailoring content and experiences to individual users based on their preferences and behavior Automation: Streamlining tasks that involve the processing or generation of multimodal data Things to try One of the key insights of Emu3 is its ability to learn from a mixture of multimodal sequences, rather than relying on task-specific architectures. This allows the model to develop a more holistic understanding of the relationships between different modalities, which can be leveraged in a variety of ways. For example, you could explore how Emu3 performs on cross-modal tasks, such as generating images from text prompts or translating text into other languages while preserving the original meaning and style.
Updated 10/5/2024
📉
AMD-Llama-135m

92
The AMD-Llama-135m is a 135M parameter language model based on the LLaMA architecture, created by AMD. It was trained on a dataset consisting of SlimPajama and Project Gutenberg, totalling around 670B training tokens. The model can be smoothly loaded as a LlamaForCausalLM with the Hugging Face Transformers library, and uses the same tokenizer as the LLaMA2 model. Similar models include the Llama-3.1-Minitron-4B-Width-Base from NVIDIA, a pruned and distilled version of the Llama-3.1-8B model, as well as the llama3-llava-next-8b from LMMS Lab, which fine-tunes the LLaMA-3 model on multimodal instruction-following data. Model inputs and outputs Inputs Text**: The AMD-Llama-135m model takes in text inputs, which can be in the form of a string. Outputs Text**: The model generates text outputs, which can be used for a variety of natural language processing tasks such as language generation, summarization, and question answering. Capabilities The AMD-Llama-135m model is a powerful text-to-text model that can be used for a variety of natural language processing tasks. Its capabilities include: Language Generation**: The model can generate coherent and fluent text on a wide range of topics, making it useful for applications like creative writing, dialogue systems, and content generation. Text Summarization**: The model can summarize long text passages, capturing the key points and essential information. Question Answering**: The model can answer questions based on the provided context, making it useful for building question-answering systems. What can I use it for? The AMD-Llama-135m model can be used for a variety of applications, including: Content Generation**: The model can be used to generate blog posts, articles, product descriptions, and other types of content, saving time and effort for content creators. Dialogue Systems**: The model can be used to build chatbots and virtual assistants that can engage in natural conversations with users. Language Learning**: The model can be used to generate language practice exercises, provide feedback on user-generated text, and assist with language learning tasks. Things to try One interesting thing to try with the AMD-Llama-135m model is to use it as a draft model for speculative decoding of the LLaMA2 and CodeLlama models. Since the model uses the same tokenizer as LLaMA2, it can be a useful starting point for exploring the capabilities of these related models. Another thing to try is to fine-tune the model on specific datasets or tasks to improve its performance for your particular use case. The model's modular architecture and open-source nature make it a flexible starting point for a wide range of natural language processing applications.
Updated 10/5/2024
🏅
New!flux-dev-de-distill

81
flux-dev-de-distill is an experiment by maintainer nyanko7 to "de-distill" guidance from the flux.1-dev model. The model was trained to remove the original distilled guidance and create true classifier-free guidance reworks. This model is not compatible with the diffusers pipeline, so users will need to use the provided inference script or manually add guidance during the iteration loop. The model was trained on 150K Unsplash images for 6K steps with a global batch size of 32, using a frozen teacher model. Examples show the model producing improved results compared to the distilled CFG approach. Similar models include the SDXL-Lightning model from ByteDance, which is a fast text-to-image model, and the CLIP-Guided Diffusion model from afiaka87, which generates images from text by guiding a denoising diffusion model. Model inputs and outputs Inputs Text prompts to describe the desired image Outputs Generated images based on the input text prompt Capabilities The flux-dev-de-distill model is capable of generating high-quality images from text prompts, improving upon the distilled CFG approach used in the original flux.1-dev model. The model was trained to produce true classifier-free guidance, which can lead to enhanced prompt following and more coherent outputs. What can I use it for? The flux-dev-de-distill model is intended for research and creative applications, such as generating artwork, designing visuals, and exploring the potential of text-to-image diffusion models. While the model is open-source, the maintainer has specified a non-commercial license that restricts certain use cases. Things to try One interesting aspect of the flux-dev-de-distill model is its use of true classifier-free guidance, which aims to improve upon the distilled CFG approach. Users could experiment with different prompts and compare the outputs to the original flux.1-dev model to see how the de-distillation process affects the model's performance and coherence.
Updated 10/5/2024
🎯
colqwen2-v0.1

76
colqwen2-v0.1 is a model based on a novel model architecture and training strategy called ColPali, which is designed to efficiently index documents from their visual features. It is an extension of the Qwen2-VL-2B model that generates ColBERT-style multi-vector representations of text and images. This version is the untrained base version to guarantee deterministic projection layer initialization. The model was introduced in the paper ColPali: Efficient Document Retrieval with Vision Language Models and first released in this repository. It was developed by the team at vidore. Model inputs and outputs Inputs Images**: The model takes dynamic image resolutions as input and does not resize them, maintaining their aspect ratio. Text**: The model can take text inputs, such as queries, to be used alongside the image inputs. Outputs The model outputs multi-vector representations of the text and images, which can be used for efficient document retrieval. Capabilities colqwen2-v0.1 is designed to efficiently index documents from their visual features. It can generate multi-vector representations of text and images using the ColBERT strategy, which enables improved performance compared to previous models like BiPali. What can I use it for? The colqwen2-v0.1 model can be used for a variety of document retrieval tasks, such as searching for relevant documents based on visual features. It could be particularly useful for applications that deal with large document repositories, such as academic paper search engines or enterprise knowledge management systems. Things to try One interesting aspect of colqwen2-v0.1 is its ability to handle dynamic image resolutions without resizing them. This can be useful for preserving the original aspect ratio and visual information of the documents being indexed. You could experiment with different image resolutions and observe how the model's performance changes. Additionally, you could explore the model's performance on a variety of document types beyond just PDFs, such as scanned images or screenshots, to see how it generalizes to different visual input formats.
Updated 10/5/2024